Abstract
Objectives
Methods
Results
Conclusion
Notes
CONFLICT OF INTEREST
There are no financial or other issues that might lead to conflict of interest.
FUNDING
This research was funded by 2024 Yeungnam University Research Grant and the National Research Foundation of Korea (NRF) grant funded by the Korea government (MSIT) (grant number: 2021R1A2C1007869).
DATA AVAILABILITY
This data that supports the findings of this study are openly available in KNHANES at https://knhanes.kdca.go.kr/knhanes/main.do.
REFERENCES






























Fig. 1.
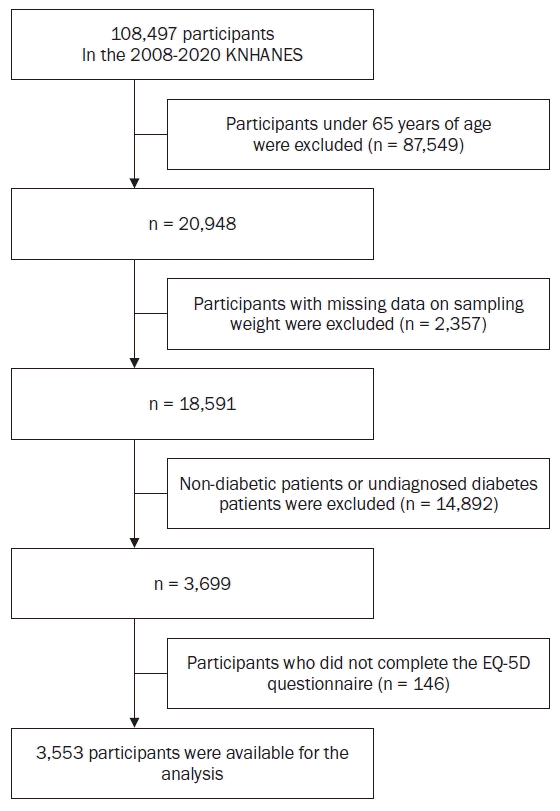
Table 1.
No. of comorbidity |
P-value | ||||
---|---|---|---|---|---|
0 (n = 573) | 1 (n = 1,421) | 2 (n = 1,135) | ≥ 3 (n = 424) | ||
Age (range, year) | < 0.001 | ||||
65 to < 70 | 178 (31.06) | 414 (29.14) | 376 (33.13) | 132 (31.13) | |
70 to < 75 | 190 (33.16) | 412 (28.99) | 353 (31.10) | 158 (37.26) | |
≥ 75 | 205 (35.78) | 595 (41.87) | 406 (35.77) | 134 (31.61) | |
Age (year) | 72.47 ± 0.20 | 73.11 ± 0.13 | 72.39 ± 0.14 | 72.28 ± 0.23 | 0.036 |
Sex | < 0.001 | ||||
Male | 284 (49.56) | 619 (43.56) | 437 (38.50) | 183 (43.16) | |
Female | 289 (50.44) | 802 (56.44) | 698 (61.50) | 241 (56.84) | |
Education level | 0.032 | ||||
Elementary school graduation or less | 355 (62.28) | 942 (66.71) | 694 (61.53) | 245 (58.19) | |
Middle school graduation | 81 (14.21) | 182 (12.89) | 168 (14.89) | 70 (16.63) | |
High school graduation or higher | 134 (23.51) | 288 (20.40) | 266 (23.58) | 106 (25.18) | |
Monthly household income (KRW) | 0.032 | ||||
Low | 172 (30.60) | 399 (28.58) | 224 (19.93) | 69 (16.31) | |
Mid-low | 144 (25.62) | 332 (23.78) | 290 (25.80) | 123 (29.08) | |
Mid-high | 110 (19.57) | 351 (25.15) | 326 (29.00) | 117 (27.66) | |
High | 136 (24.21) | 314 (22.49) | 284 (25.27) | 114 (26.95) | |
Employed, yes | 176 (30.93) | 421 (29.77) | 293 (25.98) | 82 (19.34) | < 0.001 |
Living status, alone | 116 (20.24) | 344 (24.21) | 269 (23.70) | 107 (25.24) | 0.212 |
Obesity status1) | < 0.001 | ||||
Underweight | 17 (2.98) | 26 (1.84) | 7 (0.62) | 4 (0.95) | |
Normal | 243 (42.63) | 454 (32.20) | 291 (25.84) | 110 (26.25) | |
Overweight | 135 (23.68) | 340 (24.12) | 282 (25.05) | 115 (27.45) | |
Obese | 175 (30.71) | 590 (41.84) | 546 (48.49) | 190 (45.35) | |
Physical activity2) | 28.56 ± 1.62 | 22.56 ± 1.02 | 18.59 ± 1.14 | 17.06 ± 1.87 | < 0.001 |
Smoking status | < 0.001 | ||||
Non-smoker | 311 (55.14) | 825 (58.68) | 706 (62.59) | 246 (58.57) | |
Former smoker | 157 (27.84) | 421 (29.94) | 320 (28.37) | 130 (30.95) | |
Current smoker | 96 (17.02) | 160 (11.38) | 102 (9.04) | 44 (10.48) | |
Alcohol consumption | 0.938 | ||||
Non-drinker | 315 (55.65) | 787 (56.05) | 639 (56.60) | 242 (57.48) | |
Drinker | 251 (44.35) | 617 (43.95) | 490 (43.40) | 179 (42.52) | |
Diabetes care | 0.002 | ||||
Non-care | 61 (10.65) | 121 (8.52) | 70 (6.17) | 25 (5.90) | |
Oral hypoglycemic agents/insulin treatments | 419 (73.12) | 1112 (78.25) | 924 (81.41) | 337 (79.48) | |
Diet/exercise or oral agents/insulin treatments combination | 93 (16.23) | 188 (13.23) | 141 (12.42) | 62 (14.62) | |
Diabetes duration (year) | 11.49 ± 0.39 | 11.02 ± 0.25 | 10.5 ± 0.28 | 11.5 ± 0.46 | 0.417 |
Table 2.
Table 3.
No. of comorbidity |
P-value1) |
P for trend2) |
||||
---|---|---|---|---|---|---|
0 (n = 573) | 1 (n = 1,421) | 2 (n = 1,135) | ≥ 3 (n = 424) | |||
Mobility3) | 0.79 ± 0.01ab | 0.79 ± 0.01a | 0.76 ± 0.01ab | 0.75 ± 0.02b | 0.009 | 0.003 |
Self-care3) | 0.92 ± 0.01 | 0.93 ± 0.01 | 0.91 ± 0.01 | 0.89 ± 0.01 | 0.047 | 0.020 |
Usual activity3) | 0.88 ± 0.01a | 0.87 ± 0.01a | 0.85 ± 0.01a | 0.80 ± 0.02b | < 0.001 | < 0.001 |
Pain/discomfort3) | 0.77 ± 0.02ab | 0.79 ± 0.01a | 0.74 ± 0.01b | 0.71 ± 0.02b | < 0.001 | < 0.001 |
Anxiety/depression3) | 0.93 ± 0.01a | 0.92 ± 0.01ab | 0.90 ± 0.01b | 0.88 ± 0.02b | 0.004 | < 0.001 |
EQ-5D index4) | 0.87 ± 0.01ab | 0.87 ± 0.01a | 0.85 ± 0.01bc | 0.83 ± 0.01c | < 0.001 | < 0.001 |
Mean ± SD.
Values are adjusted for age, sex, education level, monthly household income, smoking status, alcohol consumption, physical activity, body mass index and duration of diabetes.
Table 4.
No. of comorbidity |
P-value1) | ||||
---|---|---|---|---|---|
0 (n = 573) | 1 (n = 1,421) | 2 (n = 1,135) | ≥ 3 (n = 424) | ||
Nutrient | |||||
Carbohydrate (% of total energy)2) | 72.46 ± 0.54a | 72.02 ± 0.35a | 71.10 ± 0.40ab | 70.08 ± 0.65b | 0.009 |
Protein (% of total energy)2) | 13.02 ± 0.19 | 12.98 ± 0.11 | 13.20 ± 0.14 | 13.58 ± 0.22 | 0.088 |
Fat (% of total energy)2) | 12.61 ± 0.33b | 13.13 ± 0.25ab | 13.99 ± 0.28a | 14.43 ± 0.50a | < 0.001 |
Average nutrient intake | |||||
Energy (kcal)2) | 1,545.75 ± 29.30 | 1,613.28 ± 19.70 | 1,618.10 ± 21.49 | 1,530.15 ± 33.64 | 0.037 |
Vitamin A (R.E./R.A.E.)3),4) | 497.17 ± 46.34 | 466.96 ± 19.54 | 444.93 ± 22.47 | 487.64 ± 36.60 | 0.646 |
Vitamin B1 (mg)3) | 1.12 ± 0.06 | 1.14 ± 0.06 | 1.13 ± 0.06 | 1.15 ± 0.07 | 0.790 |
Vitamin B2 (mg)3) | 0.94 ± 0.05b | 0.96 ± 0.04b | 1.03 ± 0.04a | 1.07 ± 0.05a | < 0.001 |
Vitamin C (mg)3) | 74.13 ± 4.05 | 67.80 ± 2.43 | 65.63 ± 2.33 | 62.67 ± 3.27 | 0.150 |
Niacin (mg)3) | 11.13 ± 0.27 | 10.93 ± 0.13 | 10.73 ± 0.15 | 10.98 ± 0.29 | 0.563 |
Calcium (mg)3) | 429.89 ± 20.75 | 409.30 ± 8.56 | 417.88 ± 8.02 | 451.07 ± 15.86 | 0.120 |
Phosphorus (mg)3) | 895.70 ± 14.24 | 881.91 ± 7.92 | 879.61 ± 8.02 | 911.37 ± 17.53 | 0.304 |
Iron (mg)3) | 12.79 ± 0.63 | 12.12 ± 0.30 | 11.86 ± 0.33 | 11.53 ± 0.43 | 0.377 |
Sodium (mg)3) | 3,344.19 ± 108.99a | 3,041.86 ± 56.42ab | 3,115.73 ± 67.43ab | 2,886.98 ± 83.35b | 0.008 |
Potassium (mg)3) | 2,458.85 ± 44.68 | 2,449.37 ± 31.43 | 2,526.06 ± 36.39 | 2,489.72 ± 49.31 | 0.428 |
Fiber (g)3) | 17.89 ± 0.63b | 18.30 ± 0.37b | 21.70 ± 0.51a | 20.27 ± 0.68ab | < 0.001 |