Abstract
Purpose
The value of the genomic profiling by targeted gene-sequencing on radiation therapy response prediction was evaluated through integrated analysis including clinical information. Radiation response prediction model was constructed based on the analyzed findings.
Materials and Methods
Patients who had the tumor sequenced using institutional cancer panel after informed consent and received radiotherapy for the measurable disease served as the target cohort. Patients with irradiated tumor locally controlled for more than 6 months after radiotherapy were defined as the durable local control (DLC) group, otherwise, non-durable local control (NDLC) group. Significant genomic factors and domain knowledge were used to develop the Bayesian network model to predict radiotherapy response.
Results
Altogether, 88 patients were collected for analysis. Of those, 41 (43.6%) and 47 (54.4%) patients were classified as the NDLC and DLC group, respectively. Somatic mutations of NOTCH2 and BCL were enriched in the NDLC group, whereas, mutations of CHEK2, MSH2, and NOTCH1 were more frequently found in the DLC group. Altered DNA repair pathway was associated with better local failure–free survival (hazard ratio, 0.40; 95% confidence interval, 0.19 to 0.86; p=0.014). Smoking somatic signature was found more frequently in the DLC group. Area under the receiver operating characteristic curve of the Bayesian network model predicting probability of 6-month local control was 0.83.
Conclusion
Durable radiation response was associated with alterations of DNA repair pathway and smoking somatic signature. Bayesian network model could provide helpful insights for high precision radiotherapy. However, these findings should be verified in prospective cohort for further individualization.
Genome sequencing is often used for tumor samples to help find the genomic alterations suitable for targeted therapy [1,2]. With increased use of institutional panel sequencing, there are challenges to interpret clinical and/or potential value of reported mutation. For druggable targets, utility-based ranking system such as the European Society for Medical Oncology scale for clinical actionability of molecular targets [3] or the American College of Medical Genetics guidelines [4] are introduced to prioritize alterations.
Radiation therapy (RT) is another crucial component for cancer treatment. Several syndromes developed from germline variations have been reported to be related with radiation sensitivity. However, these variations have not been linked to the radiation response of the tumor [5,6]. To our knowledge, only limited number of studies have investigated the association between the genomic profile of the patient-derived tumor and the clinical RT response. Genomic profile as well as clinical factors such as diagnosis, RT dose or fractions are well acknowledged factors related with RT response [7]. The clinical value of the genomic profiling needs to be evaluated accompanying clinical information. Thus, the model integrating clinical and genomic factors is unmet need in predicting RT response.
All exons and selected introns from tumor were sequenced with an institutional targeted next-generation sequencing panel, named FiRST cancer panel, to identify somatic mutations, copy number alterations, and structural variations of tumor samples obtained from informed-consent patients. Of those, we evaluated RT response in patients with measurable disease. Clinical data and genomic alterations were integrated by using machine learning framework to develop radiation response prediction model.
Among patients whose tumors were sequenced using the FiRST cancer gene panel ver. 3.1, patients who received radiotherapy for a measurable lesion were analyzed in the current study. Measurable tumor was defined according to the new response evaluation criteria in solid tumor, the revised RECIST guideline ver. 1.1 [8]. Baseline characteristics including age, sex, and diagnosis are collected. The site and type (metastatic vs. primary) of the sequenced specimen and match of specimen collection site against RT site was also collected. As for the radiotherapy, intent of treatment, dose/fractions, biologically effective dose (BED), treatment site, modality, and the completeness of the prescribed treatment were reviewed.
To evaluate the RT response, we tracked the changes of the RT-treated tumor in follow-up medical images as well as patient symptoms. Response to RT was defined as radiologic response or symptom relief greater than partial response of the treated target. For example, for metastatic bony lesions, revised RECIST guideline was used for bony lesions with measurable extraosseous extension. For lesions not deemed measurable, symptomatic response was employed for response assessment. Those not meeting the criteria were considered no response. The duration of local control was calculated from the first day of RT to tumor progression. The durable local control (DLC) group was defined as group of patients whose irradiated tumor was locally controlled for more than 6-months. The remaining patients were defined as the non-DLC (NDLC) group.
The FiRST cancer panel ver. 3.1, employed in current study, was developed to identify exon of 183 genes, introns of 23 fusion genes, the TERT promoter region, eight micro-satellite-instability markers, and 45 drug-target lesions. Detailed list of target genes is found in previous studies [9,10].
Formalin-fixed paraffin-embedded tumor tissue was used to extract DNA, which was sequenced by NextSeq 550Dx (Illumina Inc., San Diego, CA). After quality assurance protocol, the FASTQ file was generated and aligned to the hg19 reference genome with the Burrows-Wheeler Aligner-men (v0.7.17) [11] and the Genomic Analysis Toolkit Best Practice [12]. For single nucleotide variant (SNV) and insertion and deletion (InDel) detection, GATK UnifiedGenotyper (v4.0.6.0), SNVer (v0.5.3), and LoFreq (v2.1.0) were employed. The Delly (v0.7.8) and Manta (v1.4.0) were used to identify structural variation (SV), and the THetA2 (v0.7) and CNVKit (v0.9.3) were used to reveal copy number variation (CNV). Filtering parameters are as follows: variant allele frequency ≥ 5%, reads supporting for alternative allele ≥ 10, and reads supporting each strand for alternative allele > 5 for SNV and InDel; copy number ≥ 6 and copy number ≤ 1 for CNV; split reads supporting for alternative allele ≥ 10 for translocation. By applying the threshold (> 1%) of frequency in variants found in multiple databases, the germline variants were filtered out. Afterwards, single nucleotide polymorphism/InDel variants were annotated by the Catalogue of Somatic Mutations in Cancer (COSMIC) database version 91, and those variants not retrieved from the COSMIC were further filtered out.
To derive somatic signatures, we adopted ‘deconstructSigs’ ver. 1.8.0 [13] using the COSMIC somatic signatures as reference. As per previous study [2], we derived eight signatures from COSMIC somatic signatures: aging, APOBEC, smoking, BRCA1/2, mismatch repair (MMR), ultraviolet (UV), POLE, and temozolomide (TMZ).
We employed the Bayesian network model to develop an RT response prediction model. The node indicates RT parameters, SV, CNV, and diagnosis. The edge indicates causal or effect relationship. The conditional dependencies of variables are represented with a directed acyclic graph and joint probabilities. Bayesian network specifies the full joint probability distribution as follows:
, where Xj(i) denotes the parents of Xi. Each node indicates one of a set of n dimensional variables {x1, x2,…, xn}. P denotes this conditional probability distribution for variable Xi. The Bayesian network structure is constructed to predict the probability of the DLC at 6-month via augmented Naive Bayes method. Three Bayesian network models─clinical, genomic, and clinico-genomic integrated model were generated. Mean area under the receiver operating characteristic curve (AUC) values and mean area under the precision-recall curve (AUPRC) were computed through 5-fold cross validation method.
In the clinico-genomic integrated model, target optimization tree algorithm was used to find the best evidence to maximize the DLC rate at 6 months. The target dynamic profile and embedded target optimization functions were utilized to serve the purpose. These functions are intended to search the optimum combination of variables that have a nonlinear relationship with the target and correlations between them.
Further, we performed direct effect contribution analysis to identify which type of data (clinical vs. genomic) serves as dominating factor on the local control prediction. While varying the x-value, a mean value analysis infers the corresponding mean values of the target node. Then, direct effect is the derivatives of their respective effect curves, which were computed at their a priori mean values as follows:
The standardized direct effect normalizes the direct effect by taking into account the ratio between the standard deviation of the variables (x) and the Target Node (y).
Finally, contribution is computed as follows:
All Bayesian network development, evaluation, optimization analysis, and estimation of direct effect contribution were performed using the BayesiaLab 8 (Bayesia S.A.S., Change, France).
Logistic regression was used for enrichment analysis. Chi-square tests were used to compare somatic signatures between the DLC and NDLC groups. Univariate and multivariate Cox proportional hazard models were established to investigate factors associated with local failure–free survival that was defined as the duration of local control as mentioned above. Kaplan-Meier survival analyses and log-rank tests were also performed using STATA 16 (StataCorp LLC, College Station, TX). To compare AUC among clinical, genomic, and clinico-genomic models, the DeLong’s pairwise comparison method was used [16]. To compare AUPRC, we adopted bootstrapping method with iterations of 1,000 and estimated confidence interval. The PRISM 8 (GraphPad Software, San Diego, CA) was used to perform t-test and depict bar-graphs.
Among 178 patients enrolled in the protocol for tumor sequencing, there were 88 patients (49.44%) who underwent RT. Tumor samples were collected and sequenced between March 2013 to March 2019. Among 88 patients, breast cancer was the leading diagnosis in the study population (n=28, 32%), followed by sarcorma (n=16, 18%), melanoma (n=9, 10%), and colorectal cancer (n=5, 6%) (Fig. 1A). As for the local control end-point, 46.6% (n=41) were grouped to the NDLC group, whereas 53.4% (n=47) were assigned to the DLC group, respectively. The most common RT-treated site was bone (Fig. 1B), and the leading objective of RT was palliation (Fig. 1C). Distribution of various factors were balanced between two groups except for the performance status (Table 1). Patients with deteriorated performance measured in higher Eastern Cooperative Oncology Group grade were more frequent in the NDLC group compared to the DLC group (p=0.003).
Distribution of somatically altered genes found in the COSMIC database is summarized in oncoplot (Fig. 2), regarding several oncogenic pathways: TP53, NOTCH, receptor tyrosine kinase–RAS, DNA repair, phosphoinositide 3-kinase, NRF2, cell cycle, and androgen receptor. Alterations within NOTCH2 (25/41, p=0.017) and BCL2 (7/41, p=0.018) gene were enriched in the NDLC group. Meanwhile, alterations in TSHR (9/47, p=0.014), MSH2 (11/47, p=0.014), NOTCH1 (16/47, p=0.031), and CHEK2 (15/47, p=0.049) were enriched in the DLC group.
In addition to the oncogenic pathways, we further investigated several COSMIC signatures: Aging, APOBEC, smoking, BRCA1/2, MMR, UV, POLE, and TMZ. Distribution of altered somatic signatures found in patients in terms of oncogenic pathways and the COSMIC signature are summarized in S1 Table. Alteration of smoking signature and altered DNA repair pathway were more frequent in the DLC group compared to the NDLC group (46.8% vs. 24.4%, p=0.045, Fig. 3B and 76.6% vs. 53.7%, p=0.027, Fig. 3C, respectively). Univariate and multivariate survival analysis in terms of local failure–free survival was conducted (S2 Table). Multivariate Cox proportional regression model revealed that the completion of RT (hazard ratio [HR], 0.21; 95% confidence interval [CI], 0.06 to 0.74; p=0.014) and alteration of DNA repair pathway were significant factors for better local failure–free survival (HR, 0.40; 95% CI, 0.19 to 0.86; p=0.018) (Fig. 3D). Although altered smoking signature showed numerically better local failure–free survival, the difference was not statistically significant (HR, 0.65; 95% CI, 0.32 to 1.31; p=0.232). Logistic regression analysis was conducted to investigate related factors in the DLC subgroup (S3 Table). Altered smoking signature (odds ratio [OR], 2.73; 95% CI, 1.09 to 6.81; p=0.031), performance status (OR, 0.37; 95% CI, 0.19 to 0.74; p=0.005), and altered DNA pathway (OR, 2.83; 95% CI, 1.13 to 7.04; p=0.026) were statistically significant factors.
There was no clear pattern of distribution of SV’s including insertion, inversion, duplication, deletion, translocation, and total number of SV counts (Fig. 4A). When distribution of various SV’s were compared between the groups, duplication event was more frequent in the DLC group (p=0.040) (Fig. 4B). Among duplicated genes, PUS1, POLE, FANCD2, IGFR1, and ZNF726 were duplicated for the DLC group, meanwhile, FANCD2 and NRG1 were duplicated for the NDLC group. In the circos plots, patterns of SV and CNV for the NDLC group (Fig. 4C) and the DLC group (Fig. 4D) were depicted, and differential findings in each group were also described. For example, NOTCH3 deletion was observed in the NDLC group, meanwhile, BRCA, RAD21, and ATR deletion was found in the DLC group. Copy number amplification of NOTCH2, ERBB2, CDK12, and TERT was found in the NDLC group, whereas, MYC, NOTCH3 in the DLC group. Furthermore, we investigated the fusion event per diagnosis in both groups. PDGFRB gene fusion in colorectal cancer was only found in the NDLC group, whereas melanoma with ALK gene fusion was only found in the DLC group. In particular, NTRK3 gene fusion was found in sarcoma and inflammatory myofibroblastic tumor, only in the DLC group. Overall, compared to the NDLC group, more fusion events were observed in the DLC group (Fig. 4E and F).
To integrate these results with clinical factors, we sought a model that can consider both genomic and clinical information to predict local control after RT. Finally, we constructed the Bayesian network model with significant somatic signatures, SV, CNV, diagnosis, and RT-related factors (Fig. 5A). Regarding somatic signatures, the alteration of DNA repair pathway and smoking signature was adopted. Regarding domain knowledge in radiation oncology, the model integrated RT parameters including RT site, RT dose (BED10), the intent of RT, and the completion of RT, all of which are considered to be important for local control. This model used both clinical and genomic information to predict the probability of local control at 6-month. Additionally, we built a model using clinical information only (S4A Fig.) and genomic information only (S4B Fig.), respectively. Model were compared in terms of AUC and AUPRC calculated from 10-fold validation. There was no statistically significant differences between clinico-genomic and clinical models with receiver operating characteristic curve comparison (p=0.530) (Fig. 5B), between genomic and clinico-genomic model (p=0.385), and clinical and genomic model (p=0.325), respectively. Regarding the precision-recall curve, clinico-genomic model showed the highest AUPRC of 0.879, followed by clinical model of 0.861, and genomic model of 0.834 (Fig. 5C). AUPRC difference between the employed models was statistically significant for clinico-genomic model and genomic model (AUPRC difference, 0.044; 95% bootstrap CI, 0.010 to 0.091), whereas not significant between clinico-genomic model and clinical model (AUPRC difference, 0.017; 95% bootstrap CI, −0.009 to 0.053) and clinical model and genomic model (AUPRC difference, 0.027; 95% bootstrap CI, −0.005 to 0.059).
There was a difference of the priority between clinical information and genomic information in each cancer for predicting the probability of local control (Fig. 5D). For example, to predict local control after RT, impact of clinical information was dominant in adenoid cystic carcinoma, meanwhile genomic information was more important in the hypopharyngeal cancer and choroid plexus carcinoma. However, application of derived model in clinical practice should be done with caution as it was developed from very limited number of patients.
The other usage of the model could be target optimization. This model can seek optimal combinations of clinical and genomic parameters to achieve high local control after RT. For example, when a patient with pancreatic cancer undergoes full course RT with dose of BED10 > 60 Gy and tumor demonstrates no DNA repair pathway mutation, then maximized local control could be expected if tumor carries smoking signature and CDKN2B amplification (S4D Fig.).
With the Bayesian network model, we can estimate the probability of local control at 6 months in various genomic and clinical parameter settings. For example, when palliative RT was given to painful bone metastases in patients with renal cell carcinoma, we can estimate local control probability at 6-month per prescribed RT dose in BED10. It would be very unlikely to be controlled for RT dose of BED10 < 40 Gy (S5A Fig.), approximately 39.6% control could be expected for RT dose of BED10 ≤ 60 Gy (S5B Fig.), and over 99.8% probability for RT dose of BED10 > 60 Gy (S5C Fig.), respectively. Low probability of local control by low dose reflects the real world situation and the clinical domain knowledge.
We developed a computational model to predict RT response of a measurable lesion using clinical and genomic information. Prediction was based on an individual characteristic including diagnosis, treatment parameters, somatic mutation, and structural/copy number alterations. Although quite crude in current stage, recommendation to undergo RT could be personalized based on clinical and genomic information. This in turn could collectively optimize the treatment for an individual patient, and serve as patient selection tool for clinical trials.
In clinical setting, tumor histology is an important factor for predicting radiation response [17]. Breast and prostate primaries are considered as radiosensitive tumors. In contrast, non-small lung cancer, melanoma, renal cell carcinoma, and sarcoma are considered as radioresistant tumors. In terms of local control, radiation dose, the completion of RT, and the intent of RT are important for radiation oncologists. Given that many factors are involved in RT response, our model has a clear advantage of integrating both genomic and clinical factors.
The Bayesian network is the probabilistic graphical model [18] that can be developed from multi-dimensional data and human domain knowledge. This statistical framework can provide causal inference and optimization. Further, the Bayesian network model can integrate complex data, optimize the target variable, and perform contribution analysis. Specific domain knowledge elicited by expertise can also be incorporated in the model. Luo et al. [19] proposed hierarchical relationships based on the Bayesian network to predict tumor local control in 68 non-small cell lung cancer patients before and during RT. Furthermore, Luo et al. [20] developed more advanced Bayesian network model to predict radiation pneumonitis after RT. Various types of datasets including single nucleotide polymorphisms, micro RNAs, cytokines, radiation dosimetric data were used in the model. These factors were traditionally reported to be associated with radiation pneumonitis. In line with the aforementioned studies, current study also showed that clinical domain knowledge and heterogenous dataset could be integrated in the Bayesian network machine learning framework.
Clinico-genomic model may have additional implication over clinical model alone. First, we benchmarked the performance of derived clinical, genomic, and clinico-genomic models. We found that clinico-genomic model is superior to other models regarding AUPRC, which is considered more reliable evaluation metrics in an unbalanced dataset [21]. In a similar study by Oh et al. [22], the Bayesian network model based on clinical, dosimetric, and various blood borne information demonstrated a slightly higher performance, compared to the model based on clinical and dosimetric information. Thus, systemic approach for individual would be feasible using the clinico-genomic model. Second, the model output is the probability that can be easily interpreted by clinicians. The Cox proportional hazard model, which is commonly used in clinical research, cannot estimate the survival probability at any particular time. Meanwhile, the Bayesian network model can provide clinicians with the probability in real time. This makes it possible to cope with many challenging clinical scenarios. Third, we further investigated the impact of given information on specific cancer type. We found the diversity of clinical and genomic information that contributed to the probability of local control. These results could be helpful in decision making process. Similarly, Penson et al. [23] used targeted panel DNA sequencing data and clinical information including age and gender to predict cancer histology with machine learning algorithm. By using the random forest classer, authors showed that most informative individual features for predicting 22 tumor types with random forest classifier. Thus, a selective use of machine learning can provide clinical implications to the oncologists.
There are several limitations. The number of study population was quite limited and harbored cancer type was various. In particular, the interpretation of contribution analysis should be done with utmost caution, as the number of patients allocated to each cancer type was extremely small resulting in very limited generalizability. Many patients in this study were heavily treated with various systemic treatments including chemotherapy and hormonal therapy. As detail of the systemic treatment was not introduced in the model, it might serve as underlying confounding factor. However, we prioritized the domain knowledge in radiation oncology as clinical information. Because, first, response to prior systemic treatment was evaluated. More importantly, local control of irradiated lesion is more likely to be influenced by the local treatment given, namely RT in current clinical setting. Although there is a possibility of collinearity between clinical variables and diagnosis or genomic profile, the current Bayesian network model was constructed based on augmented Naive Bayes algorithm assuming that a variable is dependent on its target class and other variables. It is our understanding that the Bayesian network does not require strict independence of random variables. In conclusion, durable radiation response was associated with alterations of DNA repair pathway and smoking somatic signature. Bayesian network model enriched with genomic information could provide helpful insights for high precision radiotherapy. However, these findings should be verified comprehensively in more homogeneous and prospective cohort for further individualization.
Electronic Supplementary Material
Supplementary materials are available at Cancer Research and Treatment website (https://www.e-crt.org).
Notes
Ethical Statement
Informed consent was waived and study protocol was approved by the institutional review board (IRB No. H-2105-142-1220).
Author Contributions
Conceived and designed the analysis: Jang BS, Kim TY, Chie EK.
Collected the data: Chang JH, Jeon SH, Song MG, Lee KH, Im SA, Kim JI, Kim TY, Chie EK.
Contributed data or analysis tools: Chang JH, Jeon SH, Song MG, Lee KH, Im SA, Kim JI, Kim TY, Chie EK.
Performed the analysis: Jang BS, Kim TY.
Wrote the paper: Jang BS.
ACKNOWLEDGMENTS
This work was supported by the National Research Foundation of Korea (NRF) grants funded by the Ministry of Science and ICT, Republic of Korea (NRF-2020R1F1A1073616). The biospecimens and data used in this study were provided by the Biobank of Seoul National University Hospital, a member of Korea Biobank Network.
The SNUH FiRST Cancer Panel v3.1 used in this study was supported by the grant of the Korea Health Technology R&D Project through the Korea Health Industry Development Institute (KHIDI), funded by the Ministry of Health and Welfare, Republic of Korea [grant number: HI14C1277].
References
2. Zehir A, Benayed R, Shah RH, Syed A, Middha S, Kim HR, et al. Mutational landscape of metastatic cancer revealed from prospective clinical sequencing of 10,000 patients. Nat Med. 2017; 23:703–13.
3. Mateo J, Chakravarty D, Dienstmann R, Jezdic S, Gonzalez-Perez A, Lopez-Bigas N, et al. A framework to rank genomic alterations as targets for cancer precision medicine: the ESMO Scale for Clinical Actionability of molecular Targets (ESCAT). Ann Oncol. 2018; 29:1895–902.


4. Li MM, Datto M, Duncavage EJ, Kulkarni S, Lindeman NI, Roy S, et al. Standards and guidelines for the interpretation and reporting of sequence variants in cancer: a joint consensus recommendation of the Association for Molecular Pathology, American Society of Clinical Oncology, and College of American Pathologists. J Mol Diagn. 2017; 19:4–23.


5. Pollard JM, Gatti RA. Clinical radiation sensitivity with DNA repair disorders: an overview. Int J Radiat Oncol Biol Phys. 2009; 74:1323–31.


6. Bergom C, West CM, Higginson DS, Abazeed ME, Arun B, Bentzen SM, et al. The implications of genetic testing on radiation therapy decisions: a guide for radiation oncologists. Int J Radiat Oncol Biol Phys. 2019; 105:698–712.


7. Forker LJ, Choudhury A, Kiltie AE. Biomarkers of tumour radiosensitivity and predicting benefit from radiotherapy. Clin Oncol (R Coll Radiol). 2015; 27:561–9.


8. Eisenhauer EA, Therasse P, Bogaerts J, Schwartz LH, Sargent D, Ford R, et al. New response evaluation criteria in solid tumours: revised RECIST guideline (version 1.1). Eur J Cancer. 2009; 45:228–47.


9. Suh J, Jeong CW, Choi S, Ku JH, Kim HH, Kim K, et al. Sharing the initial experience of pan-cancer panel analysis in high-risk renal cell carcinoma in the Korean population. BMC Urol. 2020; 20:125.


10. Park C, Kim M, Kim MJ, Kim H, Ock CY, Keam B, et al. Clinical application of next-generation sequencing-based panel to BRAF wild-type advanced melanoma identifies key oncogenic alterations and therapeutic strategies. Mol Cancer Ther. 2020; 19:937–44.
11. Li H. Exploring single-sample SNP and INDEL calling with whole-genome de novo assembly. Bioinformatics. 2012; 28:1838–44.


12. Van der Auwera GA, Carneiro MO, Hartl C, Poplin R, Del Angel G, Levy-Moonshine A, et al. From FastQ data to high confidence variant calls: the Genome Analysis Toolkit best practices pipeline. Curr Protoc Bioinformatics. 2013; 43:11.0.1–11.10.33.
13. Rosenthal R, McGranahan N, Herrero J, Taylor BS, Swanton C. DeconstructSigs: delineating mutational processes in single tumors distinguishes DNA repair deficiencies and patterns of carcinoma evolution. Genome Biol. 2016; 17:31.


14. Mayakonda A, Lin DC, Assenov Y, Plass C, Koeffler HP. Maftools: efficient and comprehensive analysis of somatic variants in cancer. Genome Res. 2018; 28:1747–56.


15. Gu Z, Gu L, Eils R, Schlesner M, Brors B. circlize Implements and enhances circular visualization in R. Bioinformatics. 2014; 30:2811–2.


16. DeLong ER, DeLong DM, Clarke-Pearson DL. Comparing the areas under two or more correlated receiver operating characteristic curves: a nonparametric approach. Biometrics. 1988; 44:837–45.


17. Laufer I, Rubin DG, Lis E, Cox BW, Stubblefield MD, Yamada Y, et al. The NOMS framework: approach to the treatment of spinal metastatic tumors. Oncologist. 2013; 18:744–51.


18. Husmeier D, Dybowski R, Roberts S. Probabilistic modeling in bioinformatics and medical informatics. London: Springer;London: 2005.
19. Luo Y, McShan D, Ray D, Matuszak M, Jolly S, Lawrence T, et al. Development of a fully cross-validated Bayesian network approach for local control prediction in lung cancer. IEEE Trans Radiat Plasma Med Sci. 2019; 3:232–41.


20. Luo Y, El Naqa I, McShan DL, Ray D, Lohse I, Matuszak MM, et al. Unraveling biophysical interactions of radiation pneumonitis in non-small-cell lung cancer via Bayesian network analysis. Radiother Oncol. 2017; 123:85–92.


21. Ozenne B, Subtil F, Maucort-Boulch D. The precision-recall curve overcame the optimism of the receiver operating characteristic curve in rare diseases. J Clin Epidemiol. 2015; 68:855–9.


Fig. 1
(A) Distribution of primary cancer in the study cohort. (B) Dot plot representing radiation therapy (RT) site. (C) Dot plot showing the intent of RT. A dot represent one percent. CW, chest wall; MUO, metastasis of unknown origin.
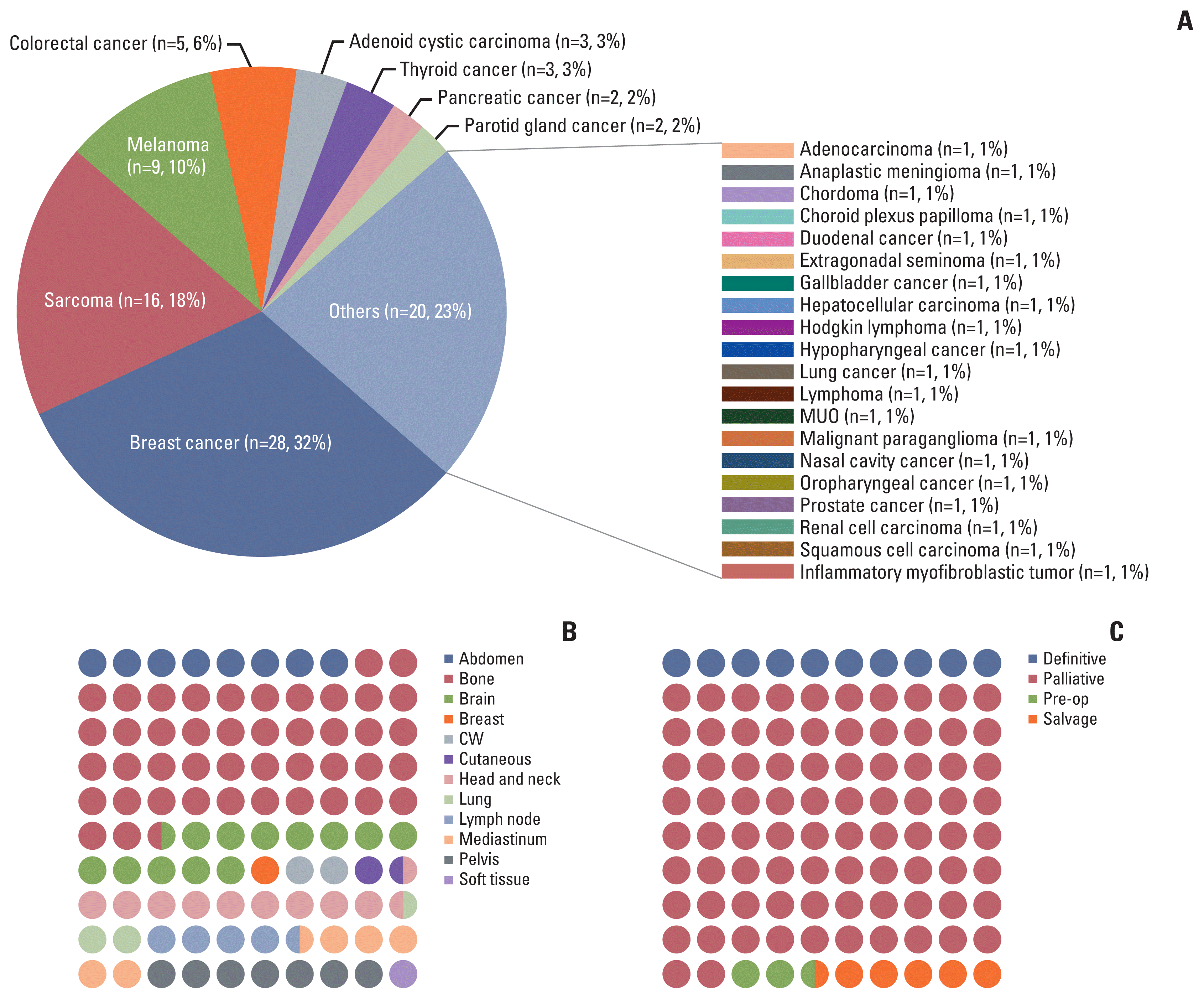
Fig. 2
Oncoplot showing the gene alterations found in Catalogue of Somatic Mutations in Cancer (COSMIC) database ver. 91. Amp, amplification; CNV, copy number variation; Del, deletion; DLC, durable local control group; ECOG, Eastern Cooperative Oncology Group performance status; LFS, local failure–free survival; NDLC, non-durable local control group; RT, radiation therapy.
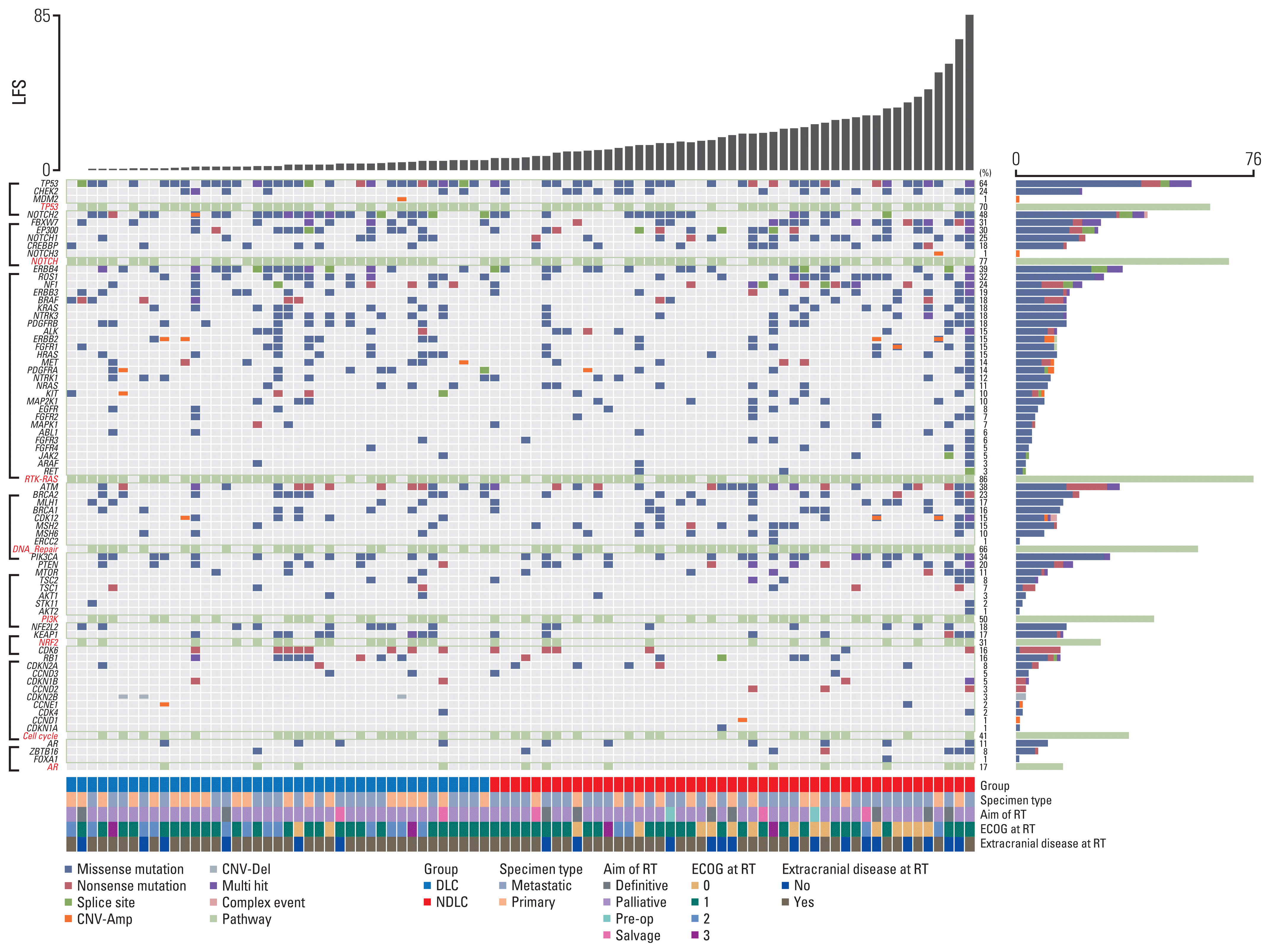
Fig. 3
(A) Bar plot showing the enrichment of gene mutation. The number of altered patient/a total number of patients are presented within bar plot. Bar plots representing percentage of patients having altered somatic signature (B) and pathway (C). p-value was estimated by Fisher exact test. (D) Kaplan-Meier curve depicting local failure–free survival between the altered and the non-altered DNA repair pathway. p-value was computed by log-rank test. AR, androgen receptor; DLC, durable local control group; MMR, mismatch repair; NDLC, non-durable local control group; PI3K, phosphoinositide 3-kinase; TMZ, temozolomide; UV, ultraviolet.
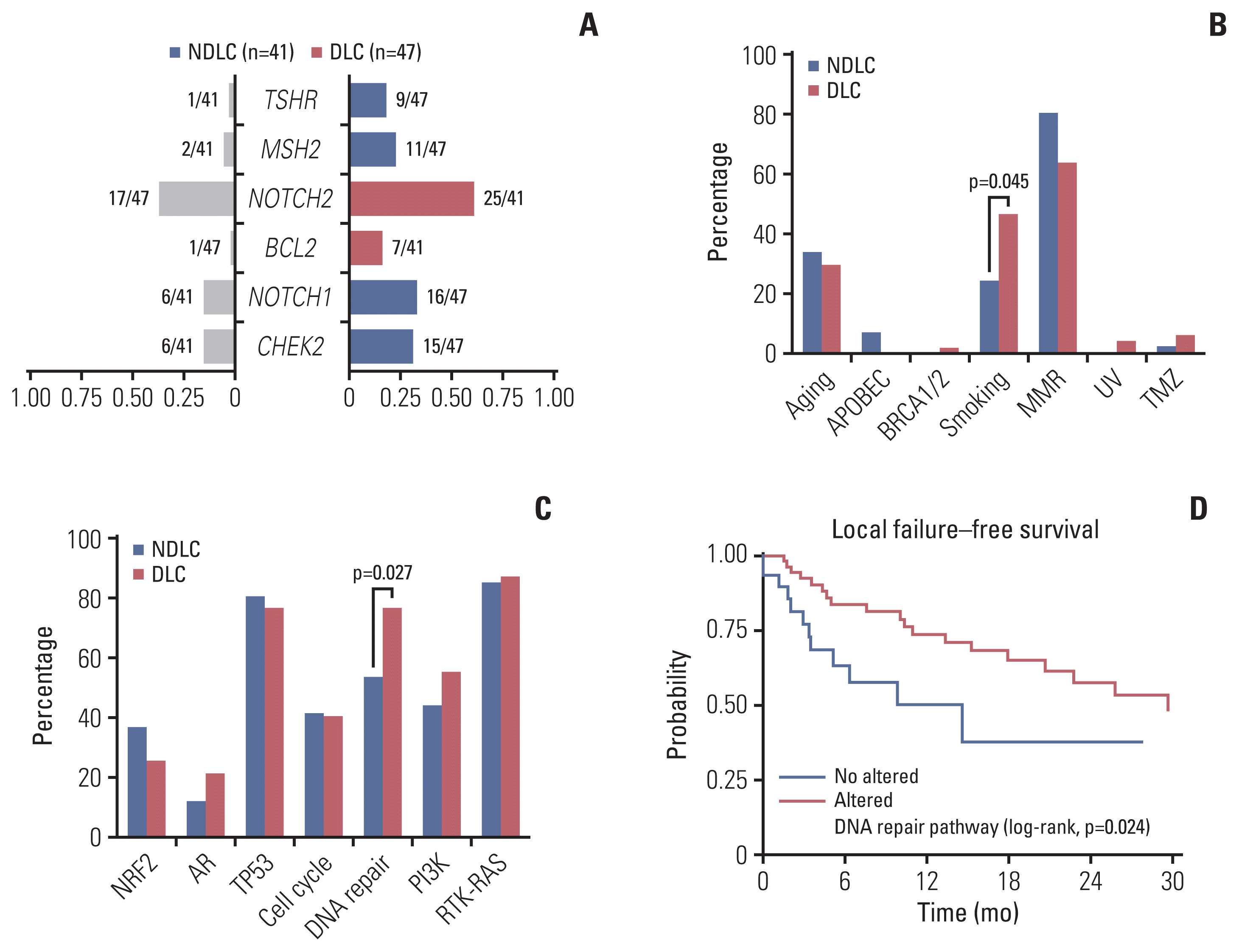
Fig. 4
(A) Cumulative bar plots showing the structural variation. Patients are sorted in ascending order in x-axis. (B) Bar plot comparing the number of structural variation events between the NDLC and the DLC groups. Circos plots depicting structural variation and copy number variations in the NDLC (C) and the DLC (D) groups. Heat map showing the number of patients having kinase fusion event in the NDLC (E) and the DLC (F) groups. Amp, amplification; CNV, copy number variation; Del or DEL, deletion; DLC, durable local control group; Dup or DUP, duplication; INS, insertion; INV, inversion; NDLC, non-durable local control group; SV, structural variation; TRA, translocation.
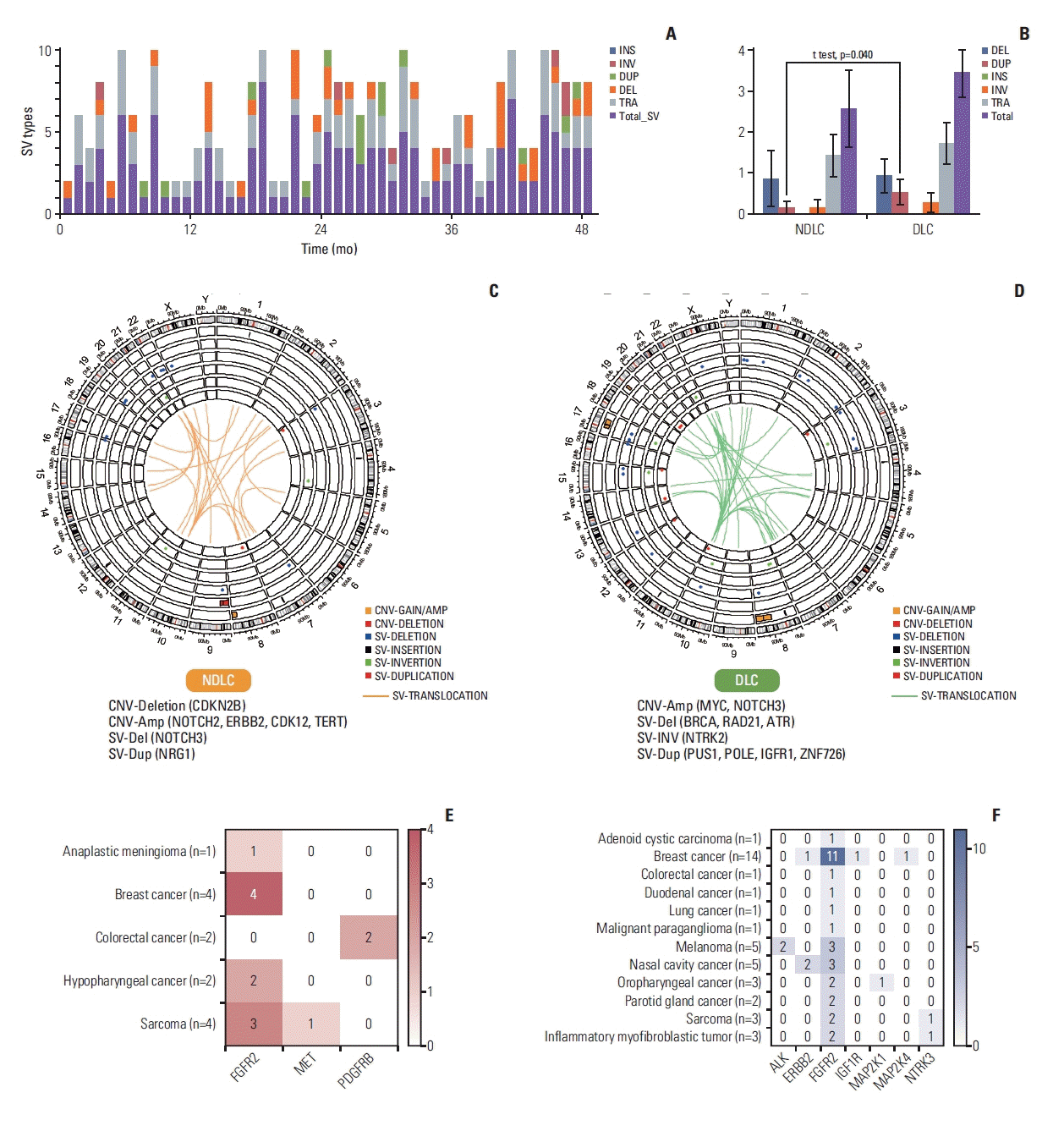
Fig. 5
(A) The Bayesian network model integrating genomic information and clinical domain knowledge. The final prediction is the probability of local control at 6 months after local RT (yellow circle). A receiver operating characteristic curve (B) and precision-recall curve (C) comparing clinico-genomic, clinical, and genomic Bayesian network models. AUC values are also presented in the plots. (D) Regarding probability prediction, contribution of genomic and clinical information in each cancer are represented. AUC, area under the curve; BED10, biologically effective dose with α/β=10; CNV, copy number variation; MUO, metastasis of unknown origin; PPV, positive predictive value; RT, radiation therapy; SV, structural variation.
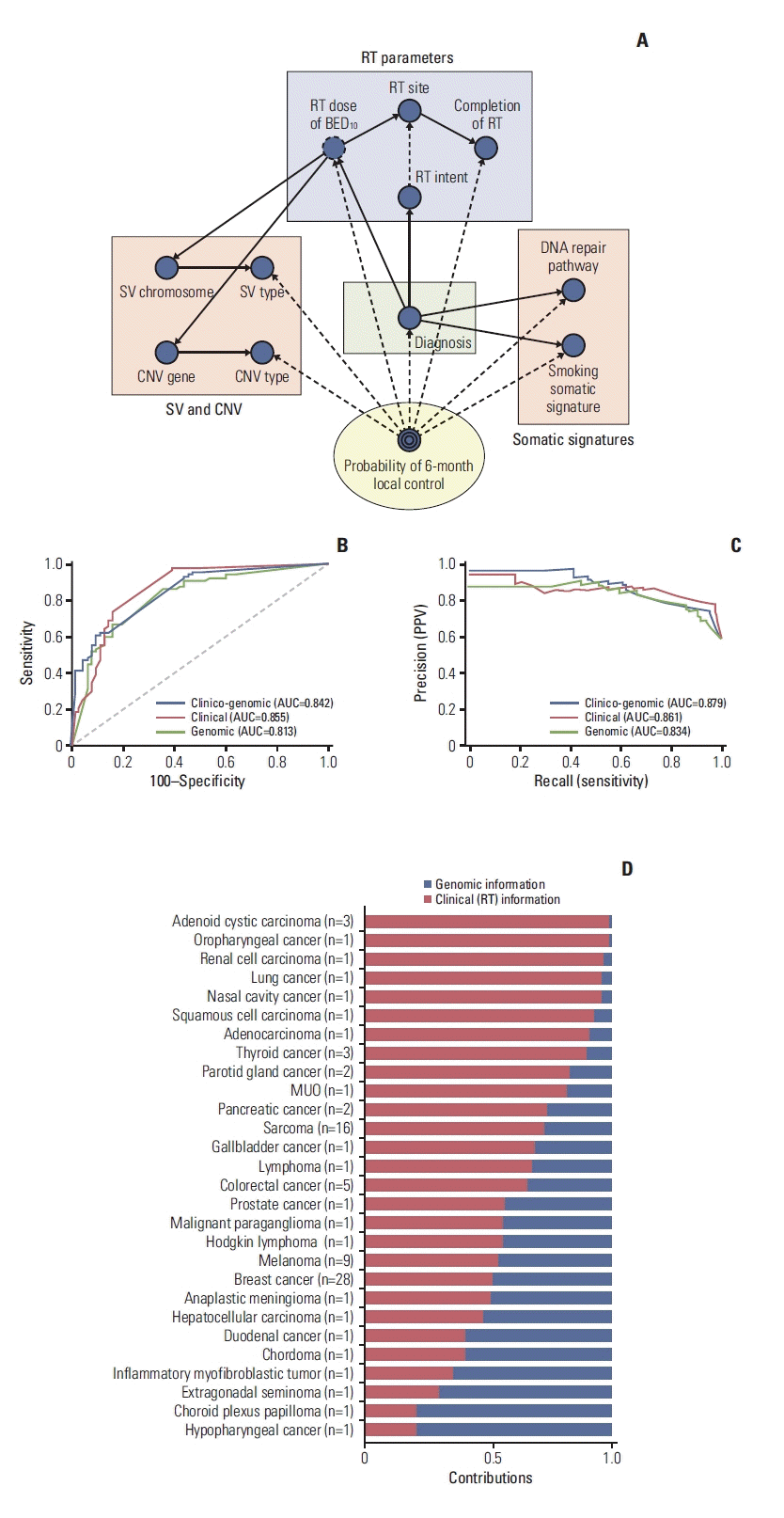
Table 1
Patient characteristics