Abstract
Purpose
Recent data have shown that the expression levels of long noncoding RNAs (lncRNAs) are associated with tamoxifen sensitivity in estrogen receptor (ER)-positive breast cancer. Herein, we constructed an lncRNA-based model to predict disease outcomes of ER-positive breast cancer patients treated with tamoxifen.
Methods
LncRNA expression information was acquired from Gene Expression Omnibus by re-mapping pre-existing microarrays of patients with ER-positive breast cancer treated with tamoxifen. The distant metastasis-free survival (DMFS) predictive signature was subsequently built based on a Cox proportional hazard regression model in discover cohort patients, which was further evaluated in another independent validation dataset.
Results
Six lncRNAs were found to be associated with DMFS in the discover cohort, which were used to construct a tamoxifen efficacy-related lncRNA signature (TLS). There were 133 and 362 patients with TLS high- and low-risk signatures in the discover cohort. Both univariate and multivariate analysis demonstrated that TLS was associated with DMFS. TLS high-risk patients had worse outcomes than low-risk patients, with a hazard ratio of 4.04 (95% confidence interval, 2.83–5.77; p<0.001). Both subgroup analysis and receiver operating characteristic analysis indicated that TLS performed better in lymph node-negative, luminal B, 21-gene recurrence score high-risk, and 70-gene prognosis signature high-risk patients. Moreover, in a comparison of the 21-gene recurrence score and 70-gene prognosis signature, TLS showed a similar area under receiver operating characteristic curve in all patients. Gene Set Enrichment Analysis indicated that TLS high-risk patients showed different gene expression patterns related to the cell cycle and nucleotide metabolism from those of low-risk patients.
Breast cancer is one of the most common fatal malignant tumors [1]. Estrogen receptor (ER)-positive breast cancer accounts for more than 60% of all breast cancer cases [2], for which endocrine therapy is among the most effective treatments. Tamoxifen, a selective estrogen-receptor modulator, is widely used in the adjuvant treatment of patients with ER-positive breast cancer [3]. However, de novo or acquired resistance still occurs. Approximately 30% of ER-positive patients do not respond to adjuvant tamoxifen treatment [4]. To determine whether ER-positive patients require further adjuvant treatment beyond tamoxifen, clinical and pathological parameters must be identified to predict the disease outcome following tamoxifen therapy. For patients with a high risk of relapse, additional treatment, such as chemotherapy, may be needed to decrease disease recurrence. Breast cancer tumor are heterogeneous and routine clinical and pathological factors, including age, menopausal status, ER positivity, progesterone receptor (PR) positivity, human epidermal growth factor receptor 2 (HER2) status, and Ki-67 expression level, cannot accurately predict disease outcomes following tamoxifen treatment [5]. In recent decades, several multi-gene assays, such as 21-gene recurrence score, 70-gene prognosis signature, and intrinsic subtype signature, were developed and approved by the U.S. Food and Drug Administration for predicting disease outcomes of ER-positive patients, leading to more individualized administration of chemotherapy and endocrine therapy for these patients [6].
Long noncoding RNAs (lncRNAs) are transcripts longer than 200 nucleotides without protein translational potential. More than 90% of the human genome is transcribed into nonprotein coding RNAs, indicating the potentially important roles of these sequences in cancer progression in addition to messenger RNAs (mRNAs) [7]. In recent years, numerous lncRNAs such as UCA1, DSCAM-AS1, and HOTAIR were found to be associated with tamoxifen sensitivity in ER-positive breast cancer, suggesting that lncRNAs can be applied as prognosis biomarkers in tamoxifen-treated patients with breast cancer [8910]. A predictive model integrating multiple lncRNAs may more accurately predict outcomes than a single lncRNA. Additionally, an lncRNA-based predictive model may provide prognosis information based on pre-existing mRNA signatures, which may cooperate with mRNA prediction models and improve outcome prediction in tamoxifen therapy.
In the present study, we used Gene Expression Omnibus (GEO) data to select lncRNAs related to tamoxifen sensitivity and construct an lncRNA-based signature to predict disease outcomes of ER-positive breast cancer patients treated with tamoxifen, which was then evaluated in an independent validation cohort. Additionally, this lncRNA-based signature was compared with the 21-gene recurrence score and 70-gene prognosis signature in these patients to investigate the potential clinical implications of this approach.
Datasets in the GEO meeting the following criteria were included in our study and formed the discover and validation datasets. First, gene expression data of patients was acquired using the Affymetrix HG-U133 A (GPL96) or HG-U133 Plus 2.0 (GPL570) microarray platform (Affymetrix, Santa Clara, USA). Next, breast cancer patients were with a full record of their ER status and treated with tamoxifen adjuvant treatment. Finally, distant metastasis-free survival (DMFS) records were available for each patient in these datasets. Since all the patients' data were obtained from public available GEO, ethic approvals of study and informed consent were already handled when they were submitted to GEO.
The lncRNA expression data from gene expression chips was obtained and analyzed as previously reported [111213]. Briefly, the Robust Multichip Average package and Combat function in the Surrogate Variable Analysis package were utilized to normalize raw data among different datasets [1415]. Moreover, the Guided Principal Components Analysis package was utilized to assess the batch effect before and after normalization [16]. The statistical variance and the first two principal components from each batch were compared before and after normalization for the discover and validation datasets, respectively. The p-value of batch variance was analyzed accordingly.
To determine lncRNA expression, probes from the arrays were aligned to the human genome (GRCh38/hg38) using SeqMap [17] such that probes matching the lncRNA chromosomal positions from GENCODE (http://www.gencodegenes.org; GRCh38, release 25) were identified [18].
To identify lncRNAs correlated with DMFS, univariate Cox proportional hazards regression analysis was firstly performed to evaluate the relationship between each lncRNA and DMFS in the discover cohort. Only lncRNAs related to DMFS with p<0.002 were considered statistically significant. Each lncRNA was evaluated by stratifying patients in the discover cohort with Cutoff Finder [19]. Multivariate Cox proportional hazards regression analysis was conducted by inputting the significant lncRNAs. By integrating these prognosis-related lncRNAs, a Cox proportional hazards regression prediction model was established. The predictive risk score of each patient was then calculated from the linear combination of lncRNA expression with its regression coefficients generated in multivariate Cox proportional hazards regression analysis. Separated by the optimized cutoff score value, patients were classified into a low-risk group with relatively good survival and a high-risk group with poor survival. Survival curves were derived using the Kaplan-Meier method with log-rank tests to evaluate differences in DMFS between the low- and high-risk groups with R package “survminer”. Receiver operating characteristic (ROC) analysis was performed to assess the predictive capability of the model with the R package “survivalROC” [20].
To evaluate the biological processes driving tamoxifen resistance in the tamoxifen efficacy-related lncRNA signature (TLS) high-risk patients, Gene Set Enrichment Analysis (GSEA; http://www.broadinstitute.org/gsea) was performed to detect the biological differences between TLS high-risk and low-risk patients using MSigDB c2: curated gene sets: all canonical pathways [2122]. Gene sets associated with TLS high-risk patients and identified with a false discovery rate (FDR)<0.01 and p<0.005 were considered as statistically significant. Furthermore, we attempted to annotate the potential function of each lncRNA in the TLS. Because GSEA failed to show significant results for individual lncRNAs in our analysis, a previously described method was adopted [111323]. Briefly, mRNAs highly correlated with each lncRNA were identified in the discover dataset by Pearson correlation analysis (top 1.0%). Next, the positively or negatively correlated mRNAs were input into the Database for Annotation, Visualization, and Integrated Discovery (DAVID; version 6.8; https://david.ncifcrf.gov/) [2425]. Finally, DAVID functional annotations with FDR<0.01 and p<0.005 were visualized with the Enrichment Map plugin in Cytoscape (version 3.2.0; http://www.cytoscape.org) for each lncRNA [26].
All data in this study was analyzed with R software (version 3.3.1; http://www.r-project.org/) and Bioconductor (http://www.bioconductor.org/). PAM50 intrinsic subtypes, 21-gene recurrence score (Oncotype DX®; Genomic Health, Redwood City, USA) and 70-gene prognosis signature (MammaPrint®; Agendia, Amsterdam, the Netherlands) were obtained by using the “genefu” package in R [27]. A p-value less than 0.05 was considered significant.
A total of 1,056 tamoxifen-treated patients with ER-positive breast cancer from GSE6532, GSE9195, GSE17705, GSE19615, GSE26971, and GSE45255 datasets were enrolled in this study. In detail, 197 ER-positive patients from GSE6532 (GPL96 platform part) and 298 ER-positive patients from GSE17705 (GPL96) treated only with 5 years of tamoxifen were combined as the discovery dataset for lncRNA-based model construction. Additionally, 88 patients from GSE6532 (GPL570 platform part), 77 patients from GSE9195 (GPL570), 62 patients from GSE19615 (GPL96), 258 patients from GSE26971 (GPL96), and 74 patients from GSE45255 (GPL96) who were also treated with 5 years of tamoxifen were combined as the validation dataset. Principal component analysis revealed no significant variance among batches for both the discover and validation datasets after normalization (Supplementary Figures 1 and 2, available online).
In the discover cohort, there were 315 and 164 patients with negative and positive lymph nodes. A total of 147 patients were classified as luminal A subtype, while 348 patients had other intrinsic subtypes of tumors. For the 21-gene recurrence score, 253 tumors were classified as recurrence score low and medium, while 242 patients had high recurrence score tumors. In terms of the 70-gene prognosis signature, there were 48 and 447 patients with low-risk and high-risk signatures (Table 1). The medium follow-up period was 7.4 (0.0–16.3) years and 126 patients (25.5%) experienced distant metastasis in the discover cohort.
A total of 558 patients were included in the validation cohort. Two hundred thirty-nine patients had tumors no larger than 2.0 cm, while 308 patients had tumors larger than 2.0 cm. A total of 285 patients were lymph node-negative and 231 patients were lymph node-positive. Additionally, 180 patients were classified as luminal A subtype and 378 as other subtypes. In terms of 21-gene recurrence classification, there were 244 and 314 patients classified as having low to medium and high recurrence scores, respectively. Meanwhile, 94 and 464 patients had low and high 70-gene prognosis scores, respectively (Table 2). After a medium follow-up of 6.3 (0.0–17.6) years, 117 patients (21.0%) had distant metastasis events.
After establishing the discover and validation datasets, we re-annotated the probes corresponding to lncRNAs for the HG-U133A and HG-U133 Plus 2.0 Affymetrix platform. A total of 598 probes corresponding to 452 lncRNAs were obtained for the HG-U133A microarray, while 5,654 probes were matching with 3,793 lncRNAs in the HG-U133 Plus 2.0 microarray. There were 365 lncRNAs overlapping between the two platforms. Figure 1 shows a diagram of the data analysis and model construction.
In the discover group of 495 ER-positive patients treated with tamoxifen, six of these 365 overlapping lncRNAs were significantly associated with DMFS in univariate Cox proportional hazard regression analysis (Table 3). Each of these six lncRNAs was capable of classifying patients into high- and low-risk groups, which could predict disease outcomes in this cohort of patients (Figure 2).
These six lncRNAs were then integrated in a multivariate Cox proportional hazard regression model to construct TLS, which assessed each tamoxifen-treated patient with an individual risk score. TLS scores were calculated as follows: TLS score=0.3753×expression value of RP11-189B4.7+0.1399× expression value of RP11-59H7.3+0.3604×expression value of CTD-2090|13.1+0.1562×expression value of LINC01399+ 0.4007×expression value of RP11-119F7.5+0.0561×expression value of RP11-193F5.1. The ROC curve of TLS was subsequently obtained with an area under the ROC curve (AUC) of 0.764 for 5 years of DMFS in the discover cohort (Figure 3A). In the ROC curve, by selecting the point nearest to the perfect prediction point, the cutoff value was set to 0.362 for TLS. Patients in the discover dataset were then separated into 133 high-risk cases and 362 low-risk cases (Table 1). The heat map revealed distinct lncRNA expression and DMFS in the TLS high- and low-risk groups (Figure 3B). Moreover, patients in the high-risk group had worse DMFS than those in the low-risk group by Kaplan-Meier analysis (Figure 3C). Univariate Cox proportional hazard regression demonstrated that lymph node status (hazard ratio [HR], 1.99; 95% confidence interval [CI], 0.62–6.37; p=0.001), TLS (HR, 4.04; 95% CI, 2.83–5.77; p<0.001), intrinsic subtype (HR, 1.68; 95% CI, 1.10–2.58; p=0.016), 21-gene recurrence score (HR, 2.27; 95% CI, 1.56–3.29; p<0.001) and 70-gene prognosis signature (HR, 2.63; 95% CI, 1.08–6.44; p=0.028) were related to DMFS in the discover cohort. Multivariate Cox proportional hazard regression analysis demonstrated that only TLS (HR, 3.40; 95% CI, 2.34–4.96; p<0.001) and 21-gene recurrence score (HR, 1.72; 95% CI, 1.15–2.58; p=0.008) were independent factors associated with DMFS in the discover cohort.
In the validation cohort, TLS separated patients into 178 high-risk cases and 380 low-risk cases (Table 2). This six lncRNA-based signature was also found to be associated with DMFS (Figure 4). In univariate Cox proportional hazard regression analysis, we found that the TLS (HR, 1.66; 95% CI, 1.15–2.40; p=0.006), 21-gene recurrence score (HR, 1.49; 95% CI, 1.02–2.17; p=0.038), and 70-gene prognosis signature (HR, 1.90; 95% CI, 1.05–3.45; p=0.032) were related to DMFS. Multivariate analysis showed that only TLS (HR, 1.54; 95% CI, 1.06–2.24; p=0.023) was independently correlated with DMFS.
Overall, a total of 1,053 patients were in the discover and validation cohorts. There were 600, 395, and 58 patients with lymph node-negative, -positive, and unknown disease. Patients with known lymph node status were included in the following analysis. According to the PAM50 classification criteria, 327 cases were identified as luminal A subtype, 496 cases as luminal B subtype, 61 cases as HER2-enriched subtype, 44 cases as basal-like subtype and 125 cases as normal-like subtype. Patients with luminal A and B disease were included in further subgroup analysis. In terms of the 21-gene recurrence score, there were 232, 265, and 556 patients classified as having low-, medium-, and high-risk recurrence scores, respectively. For the 70-gene prognosis signature, 142 and 911 patients were classified as having low-risk and high-risk signatures. Subgroups analysis showed that TLS performed better in the lymph node-negative subgroup (HR, 3.28; 95% CI, 2.26–4.75; p<0.001) than in the positive subgroup (HR, 2.02; 95% CI, 1.39–2.93; p<0.001), better in the luminal B subgroup (HR, 2.41; 95% CI, 1.73–3.37; p<0.001) than in the luminal A subgroup (HR, 1.96; 95% CI, 1.07–3.58; p=0.026), better in the 21-gene recurrence score high-risk subgroup (HR, 2.60; 95% CI, 1.89–3.56; p<0.001) than in the low-risk subgroup (HR, 1.91; 95% CI, 0.96–3.83; p=0.062) and medium-risk subgroup (HR, 1.85; 95% CI, 1.00–3.44; p=0.047) and better in the 70-gene prognosis signature high-risk subgroup (HR, 2.35; 95% CI, 1.81–3.06; p<0.001) than in the low-risk subgroup (HR, 2.55; 95% CI, 0.73–8.92; p=0.129) (Figure 5).
The predictive accuracy of TLS was then compared with the 21-gene recurrence score and 70-gene prognosis signature in all patients with AUC values of 0.656, 0.635, and 0.631, respectively (Figure 6). In all lymph node-negative patients, the AUC values were 0.693, 0.676, and 0.623 for the TLS, 21-gene recurrence score and 70-gene prognosis signature in terms of DMFS outcome prediction. Similar results were observed in the lymph node-positive subgroup (AUC values of 0.627, 0.573, and 0.611, respectively). For different intrinsic subtypes of all patients, TLS was not superior to the 21-gene recurrence score and 70-gene prognosis signature in the luminal A subtype (AUC values of 0.548, 0.688, and 0.534, respectively). However, TLS performed much better than the 21-gene recurrence score and 70-gene prognosis signature in the luminal B subtype with AUC values of 0.659, 0.580, and 0.580, respectively. Furthermore, integration of TLS into the current 21-gene recurrence score and 70-gene prognosis signature improved the disease outcome prediction power (Figure 6).
GSEA was performed to determine the biological difference between the TLS high-risk and low-risk group patients and biological processes driving tamoxifen resistance in TLS high-risk patients. In GSEA, gene sets significantly associated with TLS high-risk patients were identified in the discover cohort (FDR<0.01, p<0.005) (Supplementary Table 1, available online). Our result indicated that most gene sets associated with TLS high-risk patients were mainly correlated with cell cycle (most gene sets were correlated with G0/G1 phase, some were related to S phase and G2/M phase) and nucleotide metabolism (such as transcription, DNA and RNA synthesis). We also attempted to annotate the function of each lncRNA in the TLS by DAVID annotation analysis whose results are shown in Supplementary Figure 3 (available online).
In this study, a 6-lncRNA signature TLS was generated to predict tamoxifen sensitivity and survival outcomes of ER-positive breast cancer patients treated with tamoxifen. Multivariate Cox proportional hazard regression analysis demonstrated that TLS was independently correlated with the DMFS of ER-positive patients in both the discover and validation cohorts. We also found that this TLS predicted disease outcome in different subgroups and the integration of TLS with other gene signatures improved outcome prediction.
Adjuvant tamoxifen treatment can dramatically reduce the recurrence risk of ER-positive breast cancer [3]. However, various factors lead to primary or secondary tamoxifen resistance in ER-positive patients, including difficulties in ER binding, reactivation of ER-mediated downstream biological processes, stimulation from the tumor microenvironment and mutation of the ESR1 gene. Recent studies demonstrated that lncRNAs are related to reactivation of ER downstream pathways, providing insight into the mechanism of tamoxifen resistance [28]. In our study, the lncRNA-based model included six lncRNAs, which have not been thoroughly studied. Our GSEA analysis demonstrated that GSEA high-risk patients had different cell cycle (most gene sets were correlated with G0/G1 phase, some were related to S phase or G2/M phase) and nucleotide metabolism genes from those in the low-risk group (Supplementary Table 1, available online). Estrogen can accelerate the G1 to S phase transition to promote cell cycle progression. Tamoxifen inhibits breast cancer cell growth by arresting cells at G0/G1 phase, during which time nucleotides are prepared for the next step DNA synthesis [2930]. Therefore, we predicted that patients with high TLS scores were resistant to tamoxifen therapy because these lncRNAs interfered with G0/G1 arrest induced by tamoxifen.
The PAM50 subtype, 21-gene recurrence score and 70-gene prognosis signature are the most widely used mRNA signatures to predict the prognosis of ER-positive breast cancer patients. These mRNA signatures are superior to traditional clinicopathological factors and can predict the efficacy of adjuvant chemotherapy and endocrine therapy in these patients. Patients with the luminal B subtype, high recurrence score, or poor 70-gene prognosis signature tumor have a higher recurrence risk, leading to adjuvant chemotherapy recommendation. In contrast, for patients with luminal A, low recurrence score, or good prognosis signature tumor who have a low risk of relapse, adjuvant chemotherapy cannot provide further benefits in addition to endocrine therapy [6]. In the present study, we found TLS provided additional prognosis information compared with the PAM50 subtype, 21-gene recurrence score and 70-gene prognosis signature. Subgroup analysis and ROC analysis demonstrated that the TLS model could further classify patients into different relapse risk groups, particularly in lymph node-negative, luminal B, 21-gene recurrence score high-risk and 70-gene prognosis signature high-risk patients. Additionally, integration of TLS with other mRNA signatures also improved the prediction accuracy for ER-positive breast cancer patients treated with tamoxifen. As a result, chemotherapy can be administrated to patients in need more accurately.
Our study also had several limitations. First, the microarray platform and repurposing method limited the number of lncRNAs available for analysis, which may not have included potentially important lncRNAs. Second, clinicopathological factors including menopausal status, tumor grade, and pathological type of enrolled patients were not available. Thus, it remains uncertain whether TLS can predict the DMFS in certain subgroups. Moreover, it was unknown whether these clinicopathological factors can be integrated with TLS, which may further improve the prediction accuracy for ER-positive patients treated with tamoxifen.
In conclusion, we identified six lncRNAs useful for predicting DMFS in ER-positive breast cancer patients treated with tamoxifen. Our six lncRNA-based model further classified these patients into high TLS score and low TLS score groups, which was independently associated with DMFS and requires further clinical evaluation.
Notes
This work was supported by grants from National Natural Science Foundation of China (grant number: 81472462), Medical Guidance Foundation of Shanghai Municipal Science and Technology Commission (grant number: 15411966400), Technology Innovation Act Plan of Shanghai Municipal Science and Technology Commission (grant number: 15411952500, 15411952501).
References
2. Montemurro F, Aglietta M. Hormone receptor-positive early breast cancer: controversies in the use of adjuvant chemotherapy. Endocr Relat Cancer. 2009; 16:1091–1102. PMID: 19726539.


3. Early Breast Cancer Trialists' Collaborative Group (EBCTCG). Effects of chemotherapy and hormonal therapy for early breast cancer on recurrence and 15-year survival: an overview of the randomised trials. Lancet. 2005; 365:1687–1717. PMID: 15894097.
4. Vendrell JA, Ghayad S, Ben-Larbi S, Dumontet C, Mechti N, Cohen PA. A20/TNFAIP3, a new estrogen-regulated gene that confers tamoxifen resistance in breast cancer cells. Oncogene. 2007; 26:4656–4667. PMID: 17297453.


5. Jirström K, Rydén L, Anagnostaki L, Nordenskjöld B, Stål O, Thorstenson S, et al. Pathology parameters and adjuvant tamoxifen response in a randomised premenopausal breast cancer trial. J Clin Pathol. 2005; 58:1135–1142. PMID: 16254100.
6. Kittaneh M, Montero AJ, Glück S. Molecular profiling for breast cancer: a comprehensive review. Biomark Cancer. 2013; 5:61–70. PMID: 24250234.


7. Mattick JS, Makunin IV. Non-coding RNA. Hum Mol Genet. 2006; 15 Spec No 1:R17–R29. PMID: 16651366.


8. Wang H, Guan Z, He K, Qian J, Cao J, Teng L. LncRNA UCA1 in anti-cancer drug resistance. Oncotarget. 2017; 8:64638–64650. PMID: 28969100.


9. Niknafs YS, Han S, Ma T, Speers C, Zhang C, Wilder-Romans K, et al. The lncRNA landscape of breast cancer reveals a role for DSCAM-AS1 in breast cancer progression. Nat Commun. 2016; 7:12791. PMID: 27666543.


10. Xue X, Yang YA, Zhang A, Fong KW, Kim J, Song B, et al. LncRNA HOTAIR enhances ER signaling and confers tamoxifen resistance in breast cancer. Oncogene. 2016; 35:2746–2755. PMID: 26364613.


11. Wang G, Chen X, Liang Y, Wang W, Shen K. A long noncoding RNA signature that predicts pathological complete remission rate sensitively in neoadjuvant treatment of breast cancer. Transl Oncol. 2017; 10:988–997. PMID: 29096247.


12. Du Z, Fei T, Verhaak RG, Su Z, Zhang Y, Brown M, et al. Integrative genomic analyses reveal clinically relevant long noncoding RNAs in human cancer. Nat Struct Mol Biol. 2013; 20:908–913. PMID: 23728290.


13. Zhou M, Zhong L, Xu W, Sun Y, Zhang Z, Zhao H, et al. Discovery of potential prognostic long non-coding RNA biomarkers for predicting the risk of tumor recurrence of breast cancer patients. Sci Rep. 2016; 6:31038. PMID: 27503456.


14. Gautier L, Cope L, Bolstad BM, Irizarry RA. Affy: analysis of Affymetrix GeneChip data at the probe level. Bioinformatics. 2004; 20:307–315. PMID: 14960456.
15. Johnson WE, Li C, Rabinovic A. Adjusting batch effects in microarray expression data using empirical Bayes methods. Biostatistics. 2007; 8:118–127. PMID: 16632515.


16. Reese SE, Archer KJ, Therneau TM, Atkinson EJ, Vachon CM, de Andrade M, et al. A new statistic for identifying batch effects in highthroughput genomic data that uses guided principal component analysis. Bioinformatics. 2013; 29:2877–2883. PMID: 23958724.


17. Jiang H, Wong WH. SeqMap: mapping massive amount of oligonucleotides to the genome. Bioinformatics. 2008; 24:2395–2396. PMID: 18697769.


18. Harrow J, Frankish A, Gonzalez JM, Tapanari E, Diekhans M, Kokocinski F, et al. GENCODE: the reference human genome annotation for The ENCODE Project. Genome Res. 2012; 22:1760–1774. PMID: 22955987.


19. Budczies J, Klauschen F, Sinn BV, Győrffy B, Schmitt WD, Darb-Esfahani S, et al. Cutoff Finder: a comprehensive and straightforward Web application enabling rapid biomarker cutoff optimization. PLoS One. 2012; 7:e51862. PMID: 23251644.


20. Heagerty PJ, Lumley T, Pepe MS. Time-dependent ROC curves for censored survival data and a diagnostic marker. Biometrics. 2000; 56:337–344. PMID: 10877287.


21. Subramanian A, Tamayo P, Mootha VK, Mukherjee S, Ebert BL, Gillette MA, et al. Gene set enrichment analysis: a knowledge-based approach for interpreting genome-wide expression profiles. Proc Natl Acad Sci U S A. 2005; 102:15545–15550. PMID: 16199517.


22. Mootha VK, Lindgren CM, Eriksson KF, Subramanian A, Sihag S, Lehar J, et al. PGC-1alpha-responsive genes involved in oxidative phosphorylation are coordinately downregulated in human diabetes. Nat Genet. 2003; 34:267–273. PMID: 12808457.
23. Liao Q, Liu C, Yuan X, Kang S, Miao R, Xiao H, et al. Large-scale prediction of long non-coding RNA functions in a coding-non-coding gene co-expression network. Nucleic Acids Res. 2011; 39:3864–3878. PMID: 21247874.


24. Huang da W, Sherman BT, Lempicki RA. Systematic and integrative analysis of large gene lists using DAVID bioinformatics resources. Nat Protoc. 2009; 4:44–57. PMID: 19131956.


25. Huang da W, Sherman BT, Lempicki RA. Bioinformatics enrichment tools: paths toward the comprehensive functional analysis of large gene lists. Nucleic Acids Res. 2009; 37:1–13. PMID: 19033363.
26. Merico D, Isserlin R, Stueker O, Emili A, Bader GD. Enrichment map: a network-based method for gene-set enrichment visualization and interpretation. PLoS One. 2010; 5:e13984. PMID: 21085593.


27. Gendoo DM, Ratanasirigulchai N, Schröder MS, Paré L, Parker JS, Prat A, et al. Genefu: an R/Bioconductor package for computation of gene expression-based signatures in breast cancer. Bioinformatics. 2016; 32:1097–1099. PMID: 26607490.
28. Hayes EL, Lewis-Wambi JS. Mechanisms of endocrine resistance in breast cancer: an overview of the proposed roles of noncoding RNA. Breast Cancer Res. 2015; 17:40. PMID: 25849966.


29. Osborne CK, Boldt DH, Clark GM, Trent JM. Effects of tamoxifen on human breast cancer cell cycle kinetics: accumulation of cells in early G1 phase. Cancer Res. 1983; 43:3583–3585. PMID: 6861130.
30. Lane AN, Fan TW. Regulation of mammalian nucleotide metabolism and biosynthesis. Nucleic Acids Res. 2015; 43:2466–2485. PMID: 25628363.


SUPPLEMENTARY MATERIAL
Supplementary Table 1
Detail of the Gene Set Enrichment Analysis for all six lncRNAs
Supplementary Figure 1
The evaluation of batch effect before and after normalization in the discover dataset. The batch effect before and after Combat function normalization was assessed by Guided Principal Components Analysis package for discover dataset. The variance among batches was statistically assessed with p-value before (A) and after (B) Combat normalization for discover dataset. Meanwhile, the first two principle components (PC) of each batch were also compared before (C) and after (D) Combat normalization.
Supplementary Figure 2
The evaluation of batch effect before and after normalization in the validation dataset. The batch effect before and after Combat function normalization was assessed by Guided Principal Components Analysis package for validation dataset. The variance among batches was statistically assessed with p-value before (A) and after (B) Combat normalization for validation dataset. Meanwhile, the first two principle components (PC) of each batch were also compared before (C) and after (D) Combat normalization.
Supplementary Figure 3
Identification of the biological function associated with each individual long noncoding RNA (lncRNA) in the tamoxifen efficacy-related lncRNA signature (TLS). Messenger RNAs highly correlated with each lncRNA in TLS were input into the Database for Annotation, Visualization, and Integrated Discovery (DAVID) and results were visualized by Enrichment Map plugin in Cytoscape. (A) RP11-189B4.7, (B) RP11-59H7.3, (C) CTD-2090|13.1, (D) LINC01399, (E) RP11-119F7.5, and (F) RP11-193F5.1. Nodes represent DAVID annotation terms whose size is positively correlated with the number of genes in terms.
Figure 1
The diagram of the construction and validation of the tamoxifen efficacy-related long noncoding RNA (lncRNA) signature.
ROC=receiver operating characteristic.
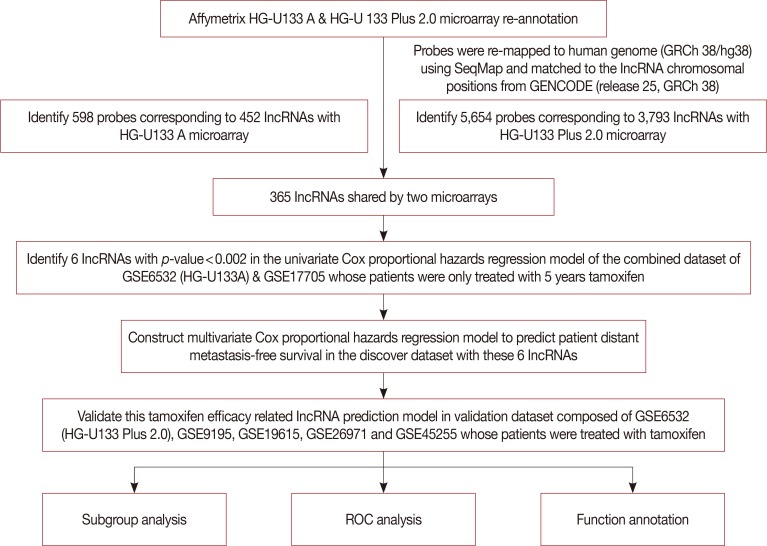
Figure 2
The six lncRNAs selected for the construction of the tamoxifen efficacy-related long noncoding RNA (lncRNA) signature. Six lncRNAs were identified with p-value less than 0.002 in the univariate Cox proportional hazards regression analysis of distant metastasis-free survival (DMFS) for the discover dataset. Each of them successfully divided patients in the discover dataset into high-risk and low-risk groups. (A) RP11-189B4.7, (B) RP11-59H7.3, (C) CTD-2090|13.1, (D) LINC01399, (E) RP11-119F7.5, and (F) RP11-193F5.1.
HR=hazard ratio.
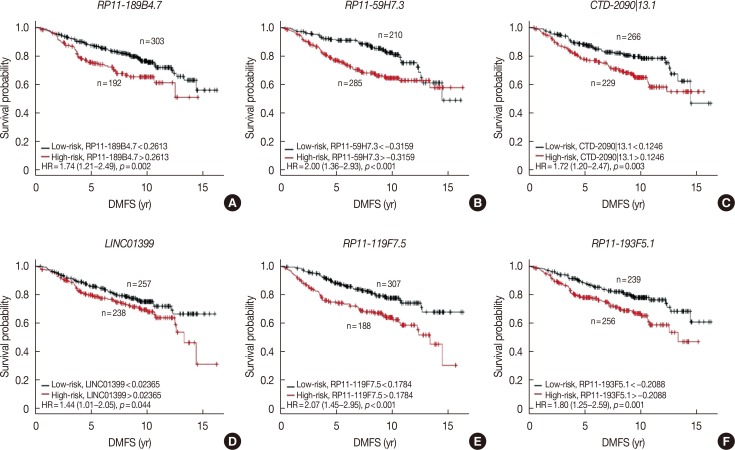
Figure 3
The prediction performance of the tamoxifen efficacy-related long noncoding RNA (lncRNA) signature (TLS) in the discover dataset after optimization. The TLS was optimized with best cutoff value. After that, the performance of TLS and expression profile of lncRNAs in TLS was analyzed in the discover dataset. (A) In the discover dataset, receiver operating characteristic (ROC) curve for the performance of TLS in distant metastasis-free survival (DMFS) was plotted with the corresponding area under the ROC curve (AUC) and the best cutoff for TLS score was determined. (B) The expression profile of the six lncRNAs in TLS, the risk score of TLS and patients' DMFS were integrated and then evaluated in the discover cohort. (C) Patients classified by TLS with optimized cutoff value were evaluated in Kaplan-Meier analysis in the discover dataset.
HR=hazard ratio.
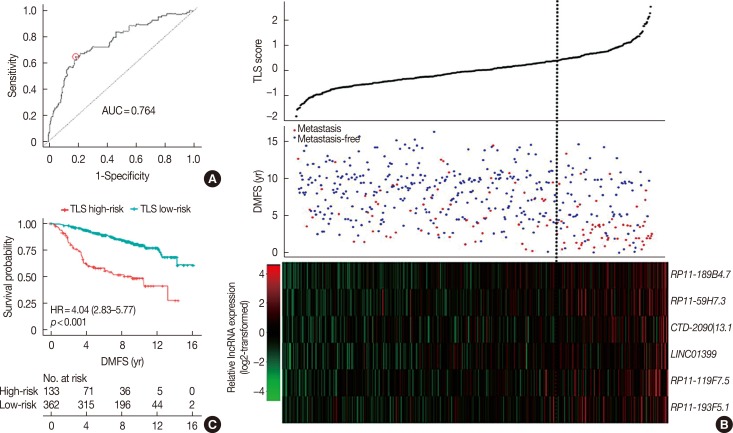
Figure 4
The evaluation of prediction capability of the tamoxifen efficacy-related long noncoding RNA (lncRNA) signature (TLS) in the validation dataset. (A) The expression profile of lncRNAs in TLS, the risk score of TLS and patients' distant metastasis-free survival (DMFS) were integrated and then evaluated in the validation cohort. (B) Patients classified by TLS with optimized cutoff value were evaluated in Kaplan-Meier analysis in the validation dataset.
HR=hazard ratio.
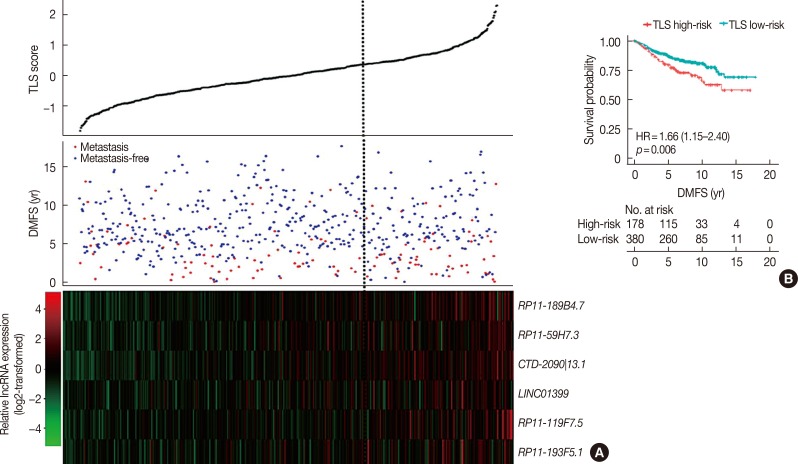
Figure 5
The evaluation of prediction power of the tamoxifen efficacy-related long noncoding RNA signature (TLS) in different subgroups of all tamoxifen treated breast cancer patients. Survival analysis of distant metastasis-free survival (DMFS) was performed to assess the prediction power of TLS in all tamoxifen treated patients, lymph node-negative subgroup, lymph node-positive subgroup, luminal A subgroup, luminal B subgroup, 21-gene recurrence score low-risk subgroup, 21-gene recurrence score medium-risk subgroup, 21-gene recurrence score high-risk subgroup, 70-gene prognosis signature low-risk subgroup and 70-gene prognosis signature high-risk subgroup.
HR=hazard ratio; CI=confidence interval.
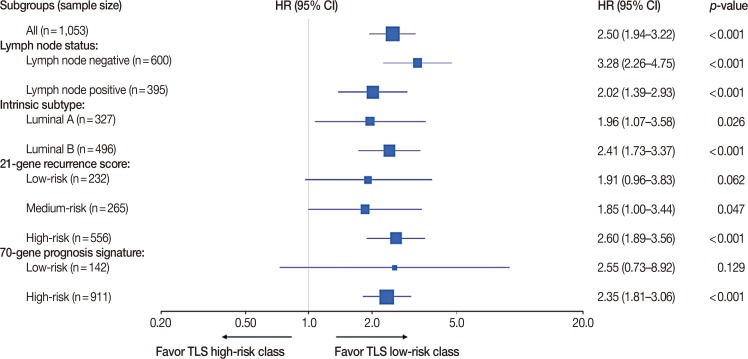
Figure 6
The comparison of the predictive power among 21-gene recurrence score (Gene21), 70-gene prognosis signature (Gene70), the tamoxifen efficacy-related long noncoding RNA signature (TLS), the integrated model of Gene21 with TLS and the integrated model of Gene70 with TLS in different subgroups of all patients. The receiver operating characteristic (ROC) of Gene21, Gene70, TLS, the integrated model of Gene21 with TLS and the integrated model of Gene70 with TLS were plotted and corresponding area under the ROC curve (AUC) was calculated in total tamoxifen treated breast cancer patients (A), all lymph node-negative patients (B), all lymph node-positive patients (C), all luminal A patients (D), and all luminal B patients (E).
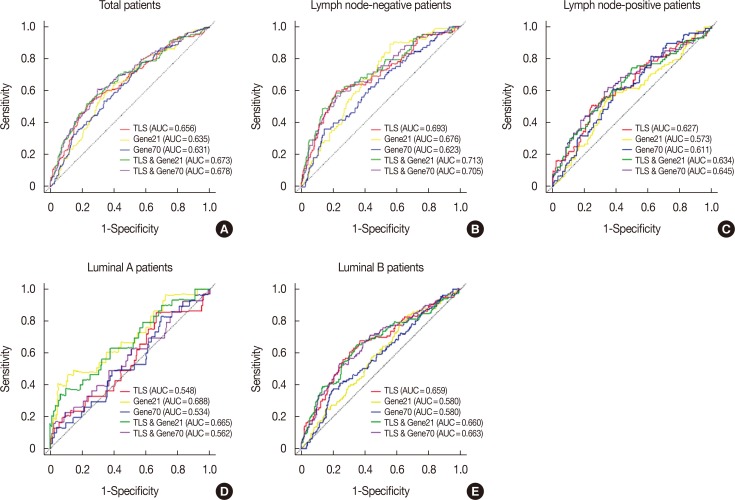
Table 1
Distribution of patients and parameters correlated with DMFS in discover set
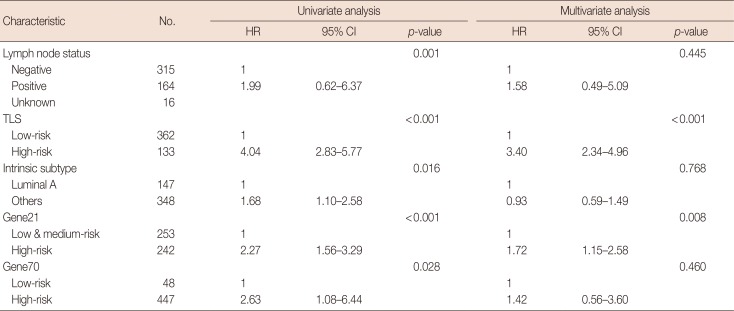
Table 2
Distribution of patients and parameters correlated with DMFS in validation set
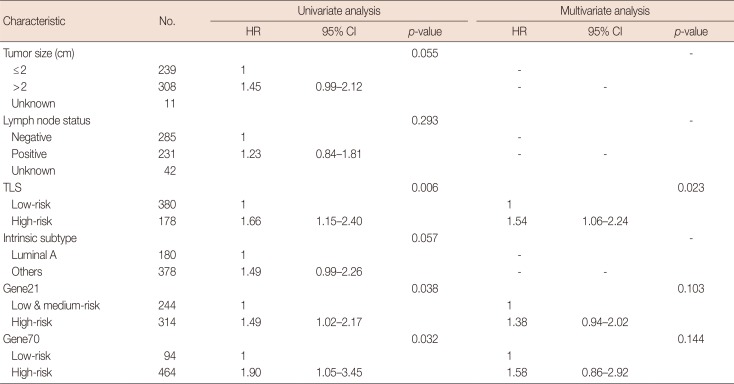
Table 3
LncRNAs identified to be associated with DMFS in discover data set
