Abstract
BACKGROUND/OBJECTIVES
In Argentina, obesity prevalence rose from 14.6% in 2005 to 20.8% in 2013. Although the number of studies on noncommunicable diseases and dietary patterns as a unique dietary exposure measure has increased, information on this topic remains scarce in developing countries. This is the first population-based study investigating the association between diet and obesity using a dietary pattern approach in Argentina. We aimed (a) to identify current dietary patterns of the population of Córdoba city, (b) to investigate its association with obesity prevalence, and (c) to identify and describe dietary patterns from the subgroup of people with obesity.
SUBJECTS/METHODS
The Córdoba Obesity and Diet Study (CODIES) was conducted in Córdoba city by using a random sample of n = 4,327 subjects between 2005 and 2012. Empirically derived dietary patterns were identified through principal component factor analysis. A multiple logistic regression analysis was used to investigate the association of dietary patterns with obesity.
RESULTS
Four dietary patterns were identified, called “Starchy-Sugar”, “Prudent”, “Western”, and “Sugary drinks”. High scores for the “Western” pattern (with strongest factor loading on meats/eggs, processed meats, and alcohol) showed a positive association with obesity (OR: 1.33, 95% CI: 1.06-1.67, for third versus first tertile of factor score). “Meats/Cheeses” and “Snacks/Alcohol” patterns emerged in people with obesity.
Obesity is a complex and multifactorial disease that constitutes one of the major metabolic risk factors for noncommunicable diseases (NCDs) [1]. In Argentina, cardiovascular diseases and cancer were responsible for more than 45% of all deaths in 2013 [2]. According to the National Risk Factor Survey, prevalence of obesity in the adult population rose from 14.6% in 2005 to 20.8% in 2013 in Argentina.
The emergence of urbanized environments and changing lifestyles over past decades has contributed to increased prevalence of obesity both in developed [34] and developing countries [5]. It has been proposed that obesity is the result of complex interactions between genetic and environmental factors, with the latter being a main contributing factor to the increasing prevalence of obesity. Human diet and lifestyle have outstripped the ability of the genome to adjust to a changing environment [6].
Nowadays, there is a consistent body of research on the roles of dietary factors in obesity [7], and most have examined the effects of single nutrients or food intake. Recently, there has been increasing interest in nutritional epidemiology research examining the association between diet and NCDs from a more integrative perspective, such as a dietary patterns approach. However, studies relating dietary patterns to obesity are still scarce in populations living in developing countries.
The dietary patterns approach offers a more reliable view of dietary intake, which is a multidimensional phenomenon. Dietary patterns, as combinations of dietary components (e.g. food items or groups), summarize key aspects of the total diet for the population being studied [8]. An advantage of this approach is that it the issue of multicollinearity can be addressed due to interdependencies between foods items and nutrients. In addition, it provides easily comprehensible dietary information that aids the formulation of public health policies to tackle obesity. This is relevant since global strategies for prevention and control of NCDs have proven inadequate [9], and many nutritional interventions implemented to relieve the global burden of obesity have not been successful.
Argentina, like several low- and middle-income countries, does not have exhaustive information about the dietary intake of its adult population. To our knowledge, there have been no studies on obesity performed from a dietary patterns perspective in this population. Accordingly, the Córdoba Obesity and Diet Study (CODIES) [1011] is a valuable source of data to investigate this issue. This population-based prevalence study was conducted in the second largest city of Argentina (Córdoba, capital city: 1,329,604 people) performing a random sample with n = 4,327 subjects in the 2005-2012 period [11].
For this obesity prevalence study, we hypothesized that there are specific dietary patterns that characterize the eating habits of people living in this urban context. These patterns have their own effects on obesity, which can differ from those previously observed in traditional perspectives based on single-nutrient intakes. Thus, the aims of the present work were (a) to identify current dietary patterns of the population of Córdoba city (Argentina), (b) to investigate the association of dietary patterns with obesity prevalence, and (c) to identify and describe the dietary patterns from the subgroup of people with obesity.
The present work analyzed a new topic in the Córdoba Obesity and Diet Study (CODIES). This random sample population-based study was conducted from January 2005 to December 2012. The general design and previous results of the study have been published elsewhere [1011]. All participants were 18-years-old or older, and data collection was conducted by a household survey. Sample size was calculated for a hypothetical overweight proportion of 0.35 (as suggested for Latin American countries) [12], a desired probabilistic Type I error of 0.05 and a power of 0.90. Thus, the sample size was 4,327 subjects, including those who agreed to participate in the study and those without inconsistent or incomplete data [11].
Information included demographic and lifestyle characteristics, body mass index (BMI), and dietary intake data obtained from a validated food frequency questionnaire (FFQ) [13] comprising 127 food items administered to 2,495 women and 1,832 men of Córdoba city by trained interviewers. The FFQ inquired about intake frequency (by day and week or month) of each particular food item over the previous 12 months and serving size (three categories: small, medium, and large), using a validated photographical atlas as a complement based on standard portion sizes in Argentina. Nutrio software was used to transform frequency of intake and portion size into food grams (or mg/day) of daily intake. In addition, the validated short form of the International Physical Activity Questionnaire (IPAQ) [14] was applied to explore physical activity habits, expressed as number of metabolic equivalent (MET) min/week. Systematically, the consistency of the data collected was checked once a month.
This study was performed in accordance with the ethical standards laid down in the 1964 Declaration of Helsinki and its later amendments and was approved by the Ethical Committee of the School of Medical Sciences, University of Córdoba [Res. 2913/2008]. All persons gave their informed consent prior to their inclusion in this study.
Firstly, empirically derived dietary patterns were identified for the total population (n = 4,327 subjects). Thus, factor analysis was applied to grams of food groups consumed per day, using principal component factor analysis (PCFA) as the extraction method. Before runing PCFA on the correlation matrix of the database, it was necessary to collapse the 127 food items of the FFQ into 15 major food groups, which were chosen to provide comprehensive representation of the Argentinean diet. These groups were milk and yogurt, cheeses, meats and eggs, processed meats, non-starchy vegetables, fruits, refined grains and starchy vegetables, whole grains and pulses, bakery products, added sugar and sweets (sugar, jam, honey, candies), fats (butter, milk cream, vegetable oils and mayonnaise), alcoholic beverages, tea, coffee, and “mate” (a popular herb infusion), non-alcoholic caloric beverages, and snacks (a small portion of food eaten between regular meals, often with high energetic density and sodium such as chips). Then, the PCFA allows us to describe the variance-covariance structure among food groups in terms of a few underlying unobservable and randomly varying factors, which are named dietary patterns [15].
The Kaiser-Meyer-Olkin (KMO) index and Bartlett's sphericity test were used to evaluate factorability of the correlation matrix [16]. KMO measures of sampling adequacy with values > 0.60 indicate a satisfactory size. An orthogonal varimax rotation was then applied to the factor loading matrix. This rotation leads to uncorrelated factors that are considered simpler and easier to interpret [17]. We chose four factors to retain based on criteria of ensuring a factor eigenvalue greater than 1 and factor interpretability. To account for parsimony and plausibility of the factors, Bayesian Information (BIC) and Akaike information (AIC) criteria were employed [17]. Each factor was labeled according to those items with absolute rotated factor loading > 0.52, which were considered as dominant food groups.
Factor scoring coefficients were then calculated for each study participant by applying the regression method. These scores indicate the degree to which each subject's food consumption conforms to each one of the emerged patterns [1516], allowing us to assign these scores to three categories or “level of adherence” to each dietary pattern (low, medium, and high) according to tertiles of factor scores.
Multiple logistic regression (MLR) analysis was used to investigate the association of dietary patterns with obesity (BMI ≥ 30 kg/m2) prevalence. Separate models for each dietary factor were fitted. AIC was used to select the appropriate adjusted models. Thus, each dietary factor model included obesity (binary response) as an outcome and dietary pattern (tertiles of scored pattern) as a covariate; sex (male/female), age (in years), physical activity (insufficient: < 600 MET min/week), educational level (complete high school or more, yes/no), energy intake (kcal/daily), smoking (yes/no), and marital status (married or living common-law, yes/no) were included as adjustment variables. ORs and the corresponding 95% confidence intervals (95% CI) were estimated for each tertile category of factor scores. Tests for linear trends were computed for comparison of means across tertiles of scores.
Finally, for comparative purposes, dietary patterns identification by PCFA was repeated using only participants with obesity (735 subjects).
Stata 12.0 software (Statacorp LP. CollegeStation, TX,USA) was used for all analyses.
Of the total 4,327 subjects studied (mean age 42 years old), 58% were men, 31% had a low instruction level, 32% were non-smoking, and 50% were married. Physical activity level was insufficient in 68% of the participants. Prevalence of obesity was similar between men and women (16.7% and 17.2%, respectively).
Table 1 shows the results from the PCFA based on dietary intakes of the whole population of Córdoba city. Four factors were retained, which explained around 42% of the total variance in the original dataset of food groups. Overall estimate of KMO value (0.728) indicated that this factor analysis was adequate for the dataset. Results of Bartlett's test of sphericity (P < 0.001) led us to reject the null hypothesis that the correlation matrix is an identity matrix.
The first dietary pattern identified was called as “Starchy-Sugar” (Table 1), which presented strong positive factor loadings on refined grains and starchy vegetables, added sugar and sweets, and bakery products. The second factor, defined as “Prudent”, was based on non-starchy vegetables, fruits, and milk and yogurt. The third one, called “Western” pattern, had the strongest factor loadings on meats and eggs, processed meats, and alcoholic beverages. The last one, labeled “Sugary drinks”, was characterized by the highest loadings on non-alcoholic caloric beverages (and negative loadings for tea, coffee, and “mate” intakes). In a simplified manner, negative factor scores can be interpreted as dominant food groups whose intakes are detrimental to intakes of those food items with positive factor scores, in the same factor. The mentioned patterns explained 12%, 11%, 11%, and 8% of the total variance in the dataset, respectively (Table 1).
As shown in Table 3, four MLR models were applied. For each model, the ORs and corresponding 95% CI were calculated for the second and thirth tertiles of the dietary pattern scores, compared to the first as a reference to estimate dietary pattern effects on obesity, and adjusted by potential confounders.
There was a positive and significant association between the “Western” pattern and obesity occurrence (P = 0.01), with a 33% higher chance of obesity in people with high versus low levels of adherence to this pattern (OR 1.33, 95% CI 1.06-1.67) in Córdoba city. There was a significant linear trend for this pattern (P = 0.015) (Table 3).
Subsequently, we identified four characteristic dietary patterns in the subgroup of the obese population (17% of total sample population). KMO measure was 0.725, indicating middling sampling adequacy. Bartlett's test of sphericity showed that the correlation matrix was not an identity matrix (P < 0.001). The retained factors accounted for 43% of the cumulative proportion of total variance in analysis of foods (Table 4).
The first and third dietary patterns in people with obesity were similar to those derived in the first and second orders from the overall population (“Starchy-Sugar” and “Prudent”). Similar to all subjects, a pattern characterized by high positive loadings of meats and eggs and processed meats, including cheeses as the dominant food group, was identified in the subgroup with obesity (named “Meats and Cheeses” pattern). In addition, one specific pattern emerged, which was characterized by strong factor loadings of snacks and alcoholic beverages (“Snacks and Alcohol” pattern), as shown in Table 4.
This is the first population-based study investigating the association between diet and obesity prevalence using a dietary pattern approach, applied to an urban population of Argentina. The relationship between social characteristics, single-nutrient/food group intakes, and overweight status has been reported previously in the same population by Aballay et al. [1011]. The present study added evidence on four dietary patterns that characterize the eating habits of people living in Córdoba city. Our results indicate that high scores for the “Western” pattern showed a positive association with obesity. In addition, some specific dietary patterns emerged in the subgroup of the population with obesity, including “Meats and Cheeses” and “Snacks and Alcohol” patterns.
Changes in dietary intake and energy expenditure related to the global “nutrition transition” [18] are driving the obesity epidemic in Latin America [119]. However, the diet-obesity relationship has been inconclusively studied in the region, especially from an epidemiological perspective of dietary patterns.
In Argentina, obesity is already a recognized public health issue [20]. Similar to other Latin America cities [21], Córdoba presents relatively high obesity prevalence (17% according to CODIES) [11]. Published results of the Argentina National Risk Factor Survey for NCDs showed a heterogeneous distribution of obesity across geographical areas, socio-economic conditions, and sex [2022].
Traditionally, the Argentinian population has a particular diet characterized by unusually high consumption of barbecued red meat, regular drinking of red wine, and low fiber intake [232425]. However, to our knowledge, no observational studies on the relationship between dietary patterns and obesity (or BMI categories) in adults have been published in Argentina; only few works about this topic have been performed in Latin American countries [2627]. Nevertheless, several case-control studies on cancer have applied PCFA to identify dietary patterns in the Southern Cone region of Latin America [16242829303132] and were performed by pooling controls from these research studies [16242829]. So far, no population-based study design with comprehensive characterization of dietary patterns has been reported. Dietary patterns similar to our “Prudent”, “Western”, and “Sugary drinks” patterns have been identified in case-control studies [173132]. Particularly, in our study, refined grains-starchy vegetables, added sugar and sweets, and bakery products emerged as dominant food groups in the first dietary pattern, which characterizes the diet of the Córdoba city population. This finding is consistent with worldwide trends of a shift from traditional toward "modern" diets based on refined grains and ultra processed, high-fat, and sugary foods [33], especially in urban societies.
Regarding the “Sugary drinks” pattern, it was previously reported that Argentina (as well as Chile, United States, and Mexico) experienced the highest consumption of soft drinks in 2010. However, whether or not consumption of this type of drink is significantly linked to overweight status and obesity, even in low- and middle-income countries [34], could not be confirmed in our study. This result can be attributed to homogeneous consumption both in people with or without obesity in our population.
It is notable that many food items that characterized the dietary patterns of the Cordoba city population have already been reported worldwide as risk factors for obesity [7]. In addition, intake of these food groups showed association with obesity (versus normal/overweight) in our population, from the traditional single-nutrient/food perspective [11]. Even so, we detected lack of association between most dietary patterns and obesity. We argue that this reinforces the idea that dietary patterns have their own effects on health, which are independent from the individual effects of food groups.
In our study, high scores for the “Western” pattern were positively related to obesity risk in the overall population. Other studies also detected similar dietary patterns associated with being overweight or obese [3536]. The above-mentioned common habit among the population of Argentina (and Córdoba city) [23] of eating barbecued red meat and drinking red wine explains the emergence of this pattern. In turn, its effect may be related to the fact that the “Western” pattern includes food groups rich in proteins and with high saturated fat content, intake of which is associated with certain NCDs such as obesity [37]. Moreover, these types of foods have high palatability, hedonic effects, and low impact on satiety, which could lead to overconsumption and increased fat body storage and obesity [3839]. On the other hand, this pattern contained alcohol, a dietary component with high energy density associated with obesity. The energy derived from alcohol cannot be stored, unlike other macronutrients, and has absolute priority in metabolism, with very high potential to affect different metabolic pathways such as reduction of fat oxidation and promotion of fat body storage [40]. Interestingly, among the obese population, “Meats and Cheese” and “Snacks and Alcohol” patterns, which includes foods with high fat content and alcoholic drinks as dominant groups, were identified.
The “Prudent” pattern did not show a protective effect on obesity in our work, which is in contrast to other studies on dietary patterns and BMI in adult populations [4142]. In line with our results, it has been suggested that significant weight loss is unlikely if there increased fruit and vegetable consumption is not accompanied by compensatory reduction in total energy intake [43]. In addition, Kant reported that the protective effects of healthy diet patterns in most published studies were relatively modest, suggesting that these dietary patterns are reported along with other desirable health behaviors, thereby confounding pattern and health association [41]. Although we considered potential confounders in the risk analysis, other factors not considered (such as likely dieting and preparation methods) may be causing confusion.
It is interesting to note that “cheesses” emerged as the dominant food group in the second indentified pattern for people with obesity, considering that the role of dairy is still debated in diet-obesity research studies [4445]. Several mechanisms underlie the effects of dairy products on body weight, mediated primarily by dietary calcium-induced modulation of adipocyte lipid metabolism, lipogenesis and lipolysis, fat oxidation, and fat absorption [45]. While current evidence suggests that dairy fat or high-fat dairy foods (as cheeses commonly eaten in Argentina) do not contribute to obesity [46], the presence of cheeses as a main food group, frequently associated with prepared foods such as pizza in our study, characterized the dietary habits of the subgroup with obesity [46]. Moreover, some scientists claimed that dairy products (with high concentrations of saturated fat and dietary antigens in cow milk) are a major cause of chronic inflammatory diseases such as obesity [47].
We also demonstrated “snacks” as a dominant food group in the last dietary pattern of the obese population. "Snacking" is an eating behavior promoted by the food industry, both in developing and developed countries, comprising a wide array of snack foods (some healthy and others unhealthy, with added sugar, salt, or saturated fat) [33]. This issue has been rarely studied in our region and should be addressed in future obesity research.
It should be underlined that our work presents some methodological strengths, such as its population-based epidemiological design, a sampling size of 4,327 subjects, and its BMI assessment method (measured, not self-reported). However, some limitations need to be considered. Concerning obesity research, a common bias is the underestimation of dietary exposure due to selective underreporting of certain food types by obese people [48]. In order to reduce this source of bias and remove interviewer bias, several training and monitoring procedures were applied along with standardization of data collection procedures. In addition, selection bias was minimized through rigorous design of the multistage population random sample [1011].
Regarding the index-based dietary patterns approach, several weaknesses have been described, such as subjectivity in naming of patterns, differences in dietary variables, and analytic decisions that limit its replication and comparability across studies [41]. However, dietary habits differ across populations. Therefore, in order to respond to the rising problem of obesity, it is imperative to define more effective and targeted interventions. Despite its limitations, we believe that this approach provides valuable information that complements data obtained by other methodological strategies.
In conclusion, the present study provides new informative dimensions for analysis of the diet-obesity relationship in Córdoba city, Argentina. We propose that this population can represent a specific subgroup that has been scarcely studied from the diet-obesity perspective in adults living in an urban context of a developing country. Our findings suggest that, in this population, there is increased obesity risk due to dietary patterns characterized by high intakes of meats and eggs, processed meats, and alcoholic beverages (“Western” pattern). Additionally, we found that subjects with obesity showed characteristic dietary patterns that differ from those patterns observed in the overall population. This information should be considered in future research studies about diet patterns in people with established obesity in order to prevent obesity incidence and design treatments. Finally, regarding the complex etiology of obesity worldwide, it is necessary to go beyond promotion of dietary changes at the individual level by recognizing the socially determined environment, which can condition individual decisions of food selection.
Figures and Tables
Table 1
Rotated factor loading matrix and explained variances for major dietary patterns identified by factor analysis in Córdoba city population (n = 4,327), Argentina. CODIES 2005-2012.
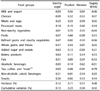
Table 2
Mean intakes of dominant food groups (g/day or cc/day) by tertiles of scored dietary patterns in Córdoba city population (n = 4,327), Argentina. CODIES 2005-2012.
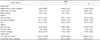
Table 3
Obesity risk estimates obtained from MLR modeling on tertiles of scored dietary patterns in Córdoba city population (n = 4,327), Argentina. CODIES 2005-2012.
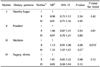
OR, odds ratio. CI, confidence interval.
1) Tertile I, II, or III of pattern score distribution.
2) Adjusted for age (continuous), sex (dichotomic), physical activity level (dichotomic), total energy intake (continuous), educational level (dichotomic), smoking (dichotomic), and marital status (dichotomic).
* P < 0.05 as levels of significance.
ACKNOWLEDGMENTS
We would like to thank the Science and Technical Secretariat of the University of Córdoba (SECyT-UNC) and National University Council (CIN) for supporting the AGDLQ and CAF's fellowships. We thank Gabriela Díaz Cortez for her language assistance. We also would like to acknowledge the cooperation of all participants in the sample.
References
1. Aballay LR, Eynard AR, Díaz MP, Navarro A, Muñoz SE. Overweight and obesity: a review of their relationship to metabolic syndrome, cardiovascular disease, and cancer in South America. Nutr Rev. 2013; 71:168–179.


2. Dirección de Estadísticas e Información de Salud (AR). Estadísticas vitales, información básica - año 2013, serie 5 - número 57 [Internet]. Buenos Aires: Ministerio de Salud de la República Argentina;2014. cited 2016 May 16. Available from: http://www.deis.gov.ar/Publicaciones/Archivos/Serie5Nro57.pdf.
3. Gallus S, Lugo A, Murisic B, Bosetti C, Boffetta P, La Vecchia C. Overweight and obesity in 16 European countries. Eur J Nutr. 2015; 54:679–689.


4. Wang Y, Beydoun MA. The obesity epidemic in the United States--gender, age, socioeconomic, racial/ethnic, and geographic characteristics: a systematic review and meta-regression analysis. Epidemiol Rev. 2007; 29:6–28.


5. Popkin BM, Slining MM. New dynamics in global obesity facing low- and middle-income countries. Obes Rev. 2013; 14:Suppl 2. 11–20.


7. Milagro FI, Mansego ML, De Miguel C, Martínez JA. Dietary factors, epigenetic modifications and obesity outcomes: progresses and perspectives. Mol Aspects Med. 2013; 34:782–812.


8. Randi G, Edefonti V, Ferraroni M, La Vecchia C, Decarli A. Dietary patterns and the risk of colorectal cancer and adenomas. Nutr Rev. 2010; 68:389–408.


9. World Health Organization. Global Status Report on Noncommunicable Diseases 2014. Geneva: World Health Organization;2014.
10. Aballay LR, Osella AR, Celi A, Del Pilar Díaz M. Overweight and obesity: prevalence and their association with some social characteristics in a random sample population-based study in Córdoba city, Argentina. Obes Res Clin Pract. 2009; 3:I–II.


11. Aballay LR, Osella AR, De La Quintana AG, Díaz MP. Nutritional profile and obesity: results from a random-sample population-based study in Córdoba, Argentina. Eur J Nutr. 2016; 55:675–685.


12. Filozof C, Gonzalez C, Sereday M, Mazza C, Braguinsky J. Obesity prevalence and trends in Latin-American countries. Obes Rev. 2001; 2:99–106.


13. Navarro A, Osella AR, Guerra V, Muñoz SE, Lantieri MJ, Eynard AR. Reproducibility and validity of a food-frequency questionnaire in assessing dietary intakes and food habits in epidemiological cancer studies in Argentina. J Exp Clin Cancer Res. 2001; 20:365–370.
14. Craig CL, Marshall AL, Sjöström M, Bauman AE, Booth ML, Ainsworth BE, Pratt M, Ekelund U, Yngve A, Sallis JF, Oja P. International physical activity questionnaire: 12-country reliability and validity. Med Sci Sports Exerc. 2003; 35:1381–1395.


15. Edefonti V, Bravi F, Garavello W, La Vecchia C, Parpinel M, Franceschi S, Dal Maso L, Bosetti C, Boffetta P, Ferraroni M, Decarli A. Nutrient-based dietary patterns and laryngeal cancer: evidence from an exploratory factor analysis. Cancer Epidemiol Biomarkers Prev. 2010; 19:18–27.


16. Pou SA, Díaz MP, Osella AR. Applying multilevel model to the relationship of dietary patterns and colorectal cancer: an ongoing case-control study in Córdoba, Argentina. Eur J Nutr. 2012; 51:755–764.


17. Edefonti V, Decarli A, La Vecchia C, Bosetti C, Randi G, Franceschi S, Dal Maso L, Ferraroni M. Nutrient dietary patterns and the risk of breast and ovarian cancers. Int J Cancer. 2008; 122:609–613.


18. Popkin BM, Adair LS, Ng SW. Global nutrition transition and the pandemic of obesity in developing countries. Nutr Rev. 2012; 70:3–21.


19. Chatenoud L, Bertuccio P, Bosetti C, Malvezzi M, Levi F, Negri E, La Vecchia C. Trends in mortality from major cancers in the Americas: 1980-2010. Ann Oncol. 2014; 25:1843–1853.


20. Linetzky B, De Maio F, Ferrante D, Konfino J, Boissonnet C. Sex-stratified socio-economic gradients in physical inactivity, obesity, and diabetes: evidence of short-term changes in Argentina. Int J Public Health. 2013; 58:277–284.


21. Schargrodsky H, Hernández-Hernández R, Champagne BM, Silva H, Vinueza R, Silva Ayçaguer LC, Touboul PJ, Boissonnet CP, Escobedo J, Pellegrini F, Macchia A, Wilson E. CARMELA Study Investigators. CARMELA: assessment of cardiovascular risk in seven Latin American cities. Am J Med. 2008; 121:58–65.


22. Christine PJ, Diez Roux AV, Wing JJ, Alazraqui M, Spinelli H. Temporal trends in BMI in Argentina by socio-economic position and province-level economic development, 2005-2009. Public Health Nutr. 2015; 18:817–826.


23. Navarro A, Muñoz SE, Lantieri MJ, del Pilar Diaz M, Cristaldo PE, de Fabro SP, Eynard AR. Meat cooking habits and risk of colorectal cancer in Córdoba, Argentina. Nutrition. 2004; 20:873–877.


24. Pou SA, Niclis C, Aballay LR, Tumas N, Román MD, Muñoz SE, Coquet JB, Díaz MP. Cancer and its association with dietary patterns in Córdoba (Argentina). Nutr Hosp. 2014; 29:618–628.
25. Muñoz SE, Navarro A, Lantieri MJ, Fabro ME, Peyrano MG, Ferraroni M, Decarli A, La Vecchia C, Eynard AR. Alcohol, methylxanthine-containing beverages, and colorectal cancer in Córdoba, Argentina. Eur J Cancer Prev. 1998; 7:207–213.


26. Cunha DB, de Almeida RM, Sichieri R, Pereira RA. Association of dietary patterns with BMI and waist circumference in a low-income neighbourhood in Brazil. Br J Nutr. 2010; 104:908–913.


27. Flores M, Macias N, Rivera M, Lozada A, Barquera S, Rivera-Dommarco J, Tucker KL. Dietary patterns in Mexican adults are associated with risk of being overweight or obese. J Nutr. 2010; 140:1869–1873.


28. Tumas N, Niclis C, Aballay LR, Osella AR, Díaz MP. Traditional dietary pattern of South America is linked to breast cancer: an ongoing case-control study in Argentina. Eur J Nutr. 2014; 53:557–566.


29. Pou SA, Niclis C, Eynard AR, Díaz MP. Dietary patterns and risk of urinary tract tumors: a multilevel analysis of individuals in rural and urban contexts. Eur J Nutr. 2014; 53:1247–1253.


30. De Stefani E, Deneo-Pellegrini H, Boffetta P, Ronco AL, Aune D, Acosta G, Mendilaharsu M, Brennan P, Ferro G. Dietary patterns and risk of cancer: a factor analysis in Uruguay. Int J Cancer. 2009; 124:1391–1397.


31. De Stefani E, Ronco AL, Deneo-Pellegrini H, Boffetta P, Aune D, Acosta G, Brennan P, Ferro G, Mendilaharsu M. Dietary patterns and risk of advanced prostate cancer: a principal component analysis in Uruguay. Cancer Causes Control. 2010; 21:1009–1016.


32. De Stefani E, Boffetta P, Correa P, Deneo-Pellegrini H, Ronco AL, Acosta G, Mendilaharsu M. Dietary patterns and risk of cancers of the upper aerodigestive tract: a factor analysis in Uruguay. Nutr Cancer. 2013; 65:384–389.


33. Popkin BM. Synthesis and implications: China's nutrition transition in the context of changes across other low- and middle-income countries. Obes Rev. 2014; 15:Suppl 1. 60–67.


34. Basu S, McKee M, Galea G, Stuckler D. Relationship of soft drink consumption to global overweight, obesity, and diabetes: a cross-national analysis of 75 countries. Am J Public Health. 2013; 103:2071–2077.


35. Haidari F, Shirbeigi E, Cheraghpour M, Mohammadshahi M. Association of dietary patterns with body mass index, waist circumference, and blood pressure in an adult population in Ahvaz, Iran. Saudi Med J. 2014; 35:967–974.
36. Cho YA, Shin A, Kim J. Dietary patterns are associated with body mass index in a Korean population. J Am Diet Assoc. 2011; 111:1182–1186.


37. Rouhani MH, Salehi-Abargouei A, Surkan PJ, Azadbakht L. Is there a relationship between red or processed meat intake and obesity? A systematic review and meta-analysis of observational studies. Obes Rev. 2014; 15:740–748.


39. Wang Y, Beydoun MA. Meat consumption is associated with obesity and central obesity among US adults. Int J Obes (Lond). 2009; 33:621–628.


40. Suter PM, Tremblay A. Is alcohol consumption a risk factor for weight gain and obesity? Crit Rev Clin Lab Sci. 2005; 42:197–227.


42. Dugee O, Khor GL, Lye MS, Luvsannyam L, Janchiv O, Jamyan B, Esa N. Association of major dietary patterns with obesity risk among Mongolian men and women. Asia Pac J Clin Nutr. 2009; 18:433–440.
43. Kaiser KA, Brown AW, Bohan Brown MM, Shikany JM, Mattes RD, Allison DB. Increased fruit and vegetable intake has no discernible effect on weight loss: a systematic review and meta-analysis. Am J Clin Nutr. 2014; 100:567–576.


44. Zemel MB, Miller SL. Dietary calcium and dairy modulation of adiposity and obesity risk. Nutr Rev. 2004; 62:125–131.


45. Dougkas A, Reynolds CK, Givens ID, Elwood PC, Minihane AM. Associations between dairy consumption and body weight: a review of the evidence and underlying mechanisms. Nutr Res Rev. 2011; 24:72–95.


46. Kratz M, Baars T, Guyenet S. The relationship between high-fat dairy consumption and obesity, cardiovascular, and metabolic disease. Eur J Nutr. 2013; 52:1–24.


47. Melnik BC. Milk--the promoter of chronic Western diseases. Med Hypotheses. 2009; 72:631–639.