Data are a valuable resource in industrial societies and are becoming increasingly important in the healthcare field. The relevance of big data analysis in the healthcare industry is growing because of paradigm shifts in healthcare services, increasing social needs, and technological advancements. Unlike typical big data characterized by the “5Vs” (volume, variety, velocity, value, and veracity), healthcare data are characterized by heterogeneity, incompleteness, timeliness, longevity, privacy, and ownership [
1]. In particular, healthcare data comprise heterogeneous (multimodal) data types, including structured data as well as unstructured data, such as computed tomography scans, magnetic resonance images, and X-ray images [
1]. Additionally, numerous challenges exist in terms of data collection in the healthcare domain, including issues related to privacy protection and ownership, and in terms of healthcare data management, including data storage, sharing, and security [
1]. Given the inherent complexity of healthcare data, which are not collected for research or industrial purposes, the lack of systematic data collection methods makes it difficult to ensure data quality.
The definition of “data quality” varies, but the most widely accepted definition is “fitness for use” [
2], which refers to data that are current, accurate, interconnected, and provide tangible value to users. Low-quality data can lead to operational losses, delays, and increased costs associated with data cleansing, potentially resulting in higher prices for products or services in data-related industries [
3]. Additionally, the quality of data can significantly impact the performance of artificial intelligence (AI) models [
4]. Particularly in healthcare, the use of low-quality data in areas such as treatment, surgery, research, and policy decisions can result in significant losses. In the United States, the use of big data in healthcare may save approximately $300 billion in economic costs annually [
5]. In this context, low-quality healthcare data pose a threat to public life and health and can lead to inefficiencies in the healthcare system. Therefore, high-quality healthcare data are essential for ongoing healthcare data research.
Various data management quality methods are being researched and reported. In 2003, the WHO published guidelines to enhance the quality of healthcare data [
6]. These guidelines provide directives for healthcare professionals and information managers, enhancing understanding of all aspects of data collection and creation. In Korea, organizations such as the National Information Society Agency (NIA) [
7] have established guidelines and directives for data quality management, and the Korea Data Industry Promotion Agency (KData) (
http://dataqual.co.kr/bbs/content.php?co_id=quality_concept) has been operating a data quality certification system since 2006. This highlights the national and social recognition of the need for systematic management to consistently maintain and improve data quality from the user’s perspective.
The NIA is building and releasing AI learning data. After quality assessment by the Telecommunications Technology Association (TTA)—an external organization that does not build the data—according to the guidelines and directives for data quality management, the data are released via AI-Hub (
https://aihub.or.kr). In big data research using laboratory data, external quality assessment results are used to evaluate data quality or accuracy [
8,
9]. However, current national and international research on healthcare data quality lacks clearly defined standards or criteria. Specifically, standards and quality management criteria reflecting the unique characteristics of healthcare data, such as imaging information and biometric signals, remain insufficient. This gap highlights the need to develop quality management measures and ensure the production and utilization of high-quality healthcare data.
Research on quality indicators and systems that align with the specific nature of healthcare data are limited [
10,
11]. Furthermore, healthcare data are not only voluminous but also varied in type, form, and attributes [
12]. Therefore, a quality management system suited to the characteristics of healthcare data is necessary for effective management and utilization. We propose three stages of quality management direction (
Fig. 1), as follows.
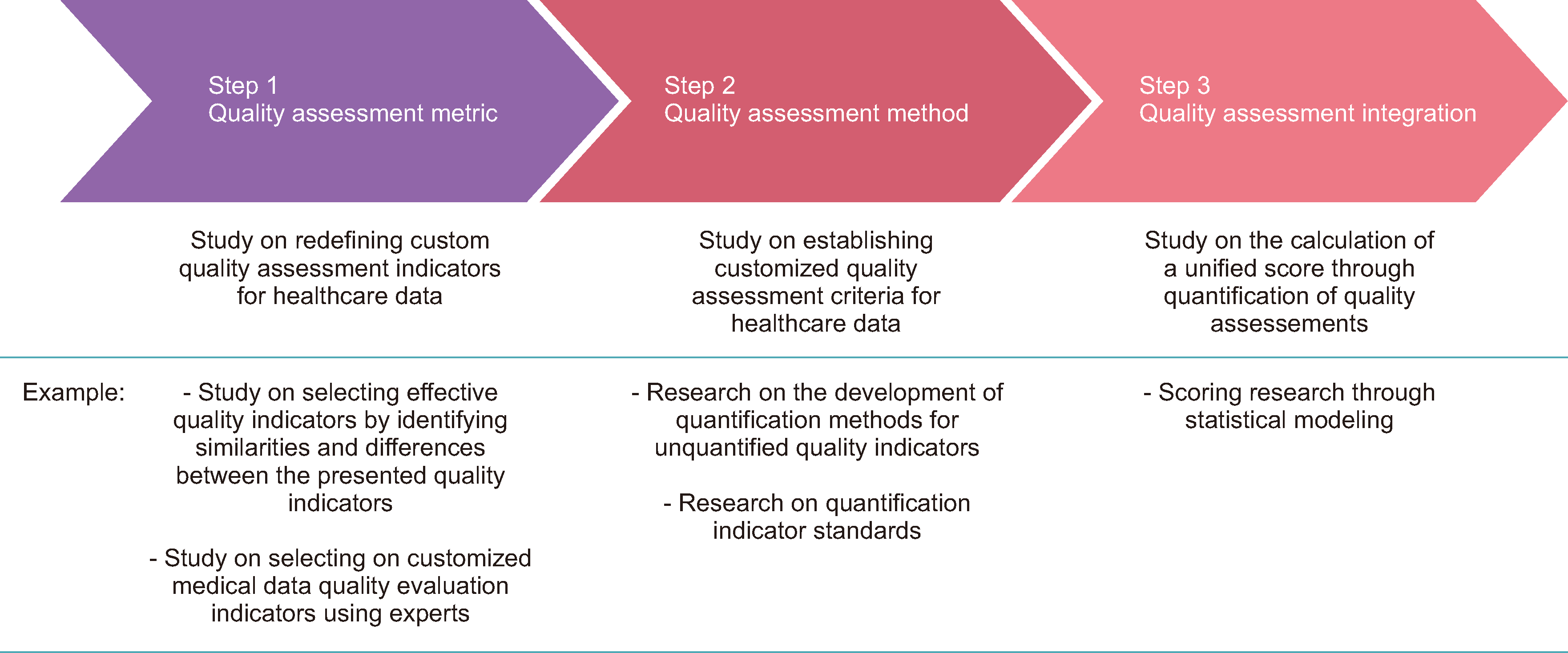 | Fig. 1 Three stages of quality management direction for customized healthcare data.
|
First, customized quality assessment indicators for healthcare data must be established. Numerous studies on quality indicators for measuring data quality have been conducted [
11]. However, the terminology and definitions of quality assessment indicators lack consistency, leading to confusion and trial-and-error because of the adoption of different quality elements and measurement items. Hwang,
et al. [
12] searched the literature from 1990 to 2023 using the search terms “data quality,” “data quality assessment,” and “data quality dimensions” in Google Scholar to examine the diversity of quality indicators. Their search yielded 23 publications with 80 different data quality assessment indicators with various definitions. This implies that the data quality assessment indicators used in research use the same term with different definitions or different terms with the same definition (
Table 1). Therefore, a consensus on the definition of data quality assessment indicators is needed to quantify data quality. In particular, quality assessment indicator research that reflects the characteristics of healthcare data is needed. In other words, to ensure the validity and reliability of healthcare data quality assessment, it is first necessary to clearly conceptualize the definitions of terms used in measuring quality. Therefore, further studies are required to redefine customized quality assessment criteria for healthcare data.
Table 1
Data quality evaluation indicators collected by Hwang, et al. [12] via a literature search
Group*
|
Indicators |
Definitions†
|
1 |
Coherence |
The extent to which data are consistent over time and across providers |
|
Compliance |
The extent to which data adhere to standards or regulations |
|
Conformity |
The extent to which data are presented following a standard format |
|
Consistency |
The extent to which data are presented following the same rule, format, and/or structure |
|
Directionality |
The extent to which data is consistently represented in the graph |
|
Identifiability |
The extent to which data have an identifier, such as a primary key |
|
Integrability |
The extent to which data follow the same definitions so that they can be integrated |
|
Integrity |
The extent to which the data format adheres to criteria |
|
Isomorphism |
The extent to which data are modeled in a compatible way |
|
Joinability |
Whether a table contains a primary key of another table |
|
Punctuality |
Whether the data are available or reported within the promised time frame |
|
Referential integrity |
Whether the data have unique and valid identifiers |
|
Representational adequacy |
The extent to which operationalization is consistent |
|
Structuredness |
The extent to which data are structured in the correct format and structure |
|
Validity |
The extent to which data conform to appropriate standards |
2 |
Ambiguity |
The extent to which data are presented properly to prevent data from being interpreted in more than one way |
|
Clarity |
The extent to which data are clear and easy to understand |
|
Comprehensibility |
The extent to which data concepts are understandable |
|
Definition |
The extent to which data are interpreted |
|
Granularity |
The extent to which data are detailed |
|
Interpretability |
The extent to which data are defined clearly and presented appropriately |
|
Naturalness |
The extent to which data are expressed using conventional, typified terms and forms according to a general-purpose reference source |
|
Presentation, Readability |
The extent to which data are clear and understandable |
|
Understandability |
The extent to which data have attributes that enable them to be read, interpreted, and understood easily |
|
Vagueness |
The extent to which data are unclear or unspecific |
3 |
Accuracy |
The extent to which data are close to the real-world or correct value(by experts) |
|
Believability |
The extent to which data are credible |
|
Correctness |
The extent to which data are true |
|
Credibility |
The extent to which data are true and correct to the content |
|
Plausibility |
The extent to which the data make sense based on external knowledge |
|
Precision |
The extent to which data are exact |
|
Reliability |
Whether the data represent reality accurately |
|
Transformation |
The error rate due to data transformation |
|
Typing |
Whether the data are typed properly |
|
Verifiability |
The extent to which data can be demonstrated to be correct |
4 |
Concise representation |
The extent to which data are represented in a compact manner |
|
Complexity |
The extent of data complexity |
|
Redundancy |
The extent to which data have a minimum content that represents the reality |
5 |
Currency |
The extent to which data are old |
|
Freshness |
The extent to which replica of data are up-to-date |
|
Timeliness |
The extent to which data are up-to-date |
|
Distinctness |
The extent to which duplicate values exist |
|
Duplication |
The extent to which data contain the same entity more than once |
|
Uniqueness |
The extent to which data have duplicates |
6 |
Ease of manipulation |
The extent to which data are applicable according to a task |
|
Rectifiability |
Whether data can be corrected |
|
Versatility |
The extent to which data can be presented using alternative representations |
7 |
Accessibility |
The extent to which data are retrieved easily and quickly |
|
Availability |
The extent to which data can be accessed |
8 |
Authority |
The extent to which the data source is credible |
|
License |
Whether the data source license is clearly defined |
|
Reputation |
The extent to which data are highly regarded in terms of their source or content |
9 |
Cohesiveness |
The extent to which the data content is focused on one topic |
|
Fitness |
The extent to which data match the theme |
10 |
Confidentiality |
The extent to which data are for authorized users only |
|
Security |
The extent to which data are restricted in terms of access |
11 |
Performance |
The latency time and throughput for coping with data with increasing requests |
|
Storage penalty |
The time spent for storage |
12 |
History |
The extent to which the data user can be traced |
|
Traceability |
The extent to which access to and changes made to data can be traced |
13 |
Appropriate amount of data |
The extent to which the data volume is appropriate for the task |
14 |
Completeness |
The extent to which data do not contain missing values |
15 |
Concordance |
The extent to which there is agreement between data elements (E.g., diagnosis of diabetes, but all A1C results are normal) |
16 |
Connectedness |
The extent to which datasets are combined at the correct resource |
17 |
Fragmentation |
The extent to which data are in one place in the record |
18 |
Objectivity |
The extent to which data are not biased |
19 |
Provenance |
Whether data contain sufficient metadata |
20 |
Volatility |
How long the information is valid in the context of a specific activity |
21 |
Volume |
Percentage of values contained in data with respect to the source from which they are extracted |
22 |
Cleanness |
The extent to which data are clean and not polluted with irrelevant information, not duplicated, and formed in a consistent way |
23 |
Normalization |
Whether data are compatible and interpretable |
24 |
Referential correspondence |
Whether the data are described using accurate labels, without duplication |
25 |
Appropriateness |
The extent to which data are appropriate for the task |
26 |
Efficiency |
The extent to which data can be processed and provide the expected level of performance |
27 |
Portability |
The extent to which data can be preserved in existing quality under any circumstance |
28 |
Recoverability |
The extent to which data have attributes that allow the preservation of quality under any circumstance |
29 |
Relevancy |
The extent to which data match the user requirements |
30 |
Usability |
The extent to which data satisfy the user requirements |
31 |
Value-added |
The extent to which data are beneficial |

Second, a method for quantifying customized quality indicators for healthcare data and benchmarks for assigning quality evaluation scores must be established. For example, Lee and Shin [
13] reported that labeling accuracy, one of the quality indicators, can be set at different levels depending on the similarity among the characteristic variables of the data and the level of class imbalance. This implies that the level of quality indicators for healthcare data quality assessment should be determined based on characteristics such as data similarity, sample size, and imbalance. Data quality indicators are evaluated both quantitatively and qualitatively. Quantitative evaluation is based on factors such as data completeness, validity, consistency, and accuracy, and quality levels are defined in four classes (ace, high, middle, low) using the Six Sigma concept [
7]. In qualitative evaluation, evaluators subjectively judge criteria through a checklist for each indicator by answering yes-or-no questions [
7].
Additionally, the measurement of quality indicators varies depending on the data type (structured/unstructured). As healthcare data are multimodal, it is necessary to review the appropriateness of the criteria for quantifying quality indicators considering the data characteristics. Therefore, further studies are required to establish customized quality assessment criteria for healthcare data.
Third, a unified quality score presentation should be established to facilitate an intuitive understanding of data quality by the end user. Moges,
et al. [
14] analyzed the relative importance of quality indicators by surveying private companies in various countries, which revealed that the importance of quality items varies by industry. The relative importance of quality indicators for healthcare data can be analyzed to establish a unified quality score. Hence, studies on calculating a unified score through the quantification of quality assessments are needed.
Data quality assessment requires a multidisciplinary approach to reflect the demand for sustainable, high-quality data across various domains. Therefore, research on healthcare data quality should not be limited to specific domain knowledge areas but can be conducted as a joint study by experts with knowledge in various fields such as medicine, data science, statistics, and information technology. A creative approach to data quality necessitates effective interdisciplinary collaboration among experts from various fields [
15].
In conclusion, we emphasize the necessity of high-quality data and present a direction for establishing quality indicators and systems suitable for the characteristics of healthcare data. Developing a clear and consistent definition of data quality and systematic methods and approaches for data quality assessment require more extensive research.