Abstract
Background
Programmed death ligand 1 (PD-L1) expression cannot currently be predicted through radiological findings. This study aimed to develop a prediction model capable of differentiating between positive and negative PD-L1 expression through a radiomics-based investigation of computed tomography (CT) images in patients with urothelial carcinoma.
Methods
Sixty-four patients with urothelial carcinoma who underwent immunohistochemical testing for PD-L1 were retrospectively reviewed. The number of patients in the positive and negative PD-L1 groups (PD-L1 expression >5%) was 14 and 50, respectively. CT images obtained 90 seconds after contrast medium administration were selected for radiomic extraction. For all tumors, 1,691 radiomic features were extracted from CT using a manually segmented three-dimensional volume of interest. Univariate and multivariate logistic regression analyses were performed to identify radiomic features that were significant predictors of PD-L1 expression. For the radiomics-based model, a receiver operating characteristic (ROC) analysis was performed.
Results
Among 64 patients, 14 were included in the PD-L1 positive group. Logistic regression analysis found that the following radiomic features significantly predicted PD-L1 expression: wavelet-low-pass, low-pass, and high-pass filters (LLH)_gray-level size-zone matrix (GLSZM)_SmallAreaEmphasis, wavelet-LLH_firstorder_Energy, log-sigma-0-5-mm-3D_GLSZM_SmallAreaHighGrayLevelEmphasis, original_shape_Maximum2DDiameterColumn, wavelet-low-pass, low-pass, and low-pass filters (LLL)_gray-level run-length matrix (GLRLM)_ShortRunEmphasis, and exponential_firstorder_Kurtosis. The radiomics signature was –4.0934+21.6224 (wavelet-LLH_GLSZM_SmallAreaEmphasis)+0.0044 (wavelet-LLH_firstorder_Energy)–4.7389 (log-sigma-0-5-mm-3D_GLSZM_SmallAreaHighGrayLevelEmphasis)+0.0573 (original_shape_Maximum2DDiameterColumn)–29.5892 (wavelet-LLL_GLRLM_ShortRunEmphasis)–0.4324 (exponential_firstorder_Kurtosis). The area under the ROC curve model representing the radiomics signature for differentiating cases that were deemed PD-L1 positive based on immunohistochemistry was 0.96.
Urothelial carcinoma is the ninth leading cause of cancer-related death and is considered one of the most aggressive neoplasms [1,2]. The median survival for metastatic urothelial carcinoma is approximately 12 to 15 months [3]. The first chemotherapy for metastatic urothelial carcinoma was a combination of cisplatin-based drugs [4]. However, over half of these patients cannot receive treatment because of renal dysfunction, poor performance status, or other comorbidities. Other alternative first-line therapies are considered standards but have not been satisfactory, with inferior results compared to those of cisplatin-based therapy. However, immune checkpoint blockade has shown superior results, opening a new field of urothelial cancer treatment with the potential to improve outcomes [5-8].
Immunotherapy targeting programmed cell death protein-1, which is expressed in urothelial carcinoma cells, and programmed death ligand 1 (PD-L1), which binds thereto, is emerging as a new treatment method. In particular, atezolizumab was approved as a first-line therapy in PD-L1-positive patients who were unsuitable for cisplatin-based therapy. In the case of atezolizumab, the most representative monoclonal antibody, an objective response rate (ORR) of approximately 16% was obtained in previous studies; in particular, in the group with positive (≥5%) PD-L1 expression, the ORR was 28% [9-11].
Tissues are essential to confirm the expression rate of PD-L1; if the PD-L1 expression rate can be predicted by analyzing computed tomography (CT) images of patients with urothelial carcinoma, it can provide additional information to help diagnose and treat these patients. However, no means of predicting PD-L1 expression using radiological findings has been identified; therefore, another method is needed.
Radiomics is a quantitative analytical method that extracts and analyzes a large amount of imaging data from radiological images. The radiomic features that can be obtained through image analysis are related to the tumor phenotype or the microenvironment [12-14]. Studies predicting treatment response or cancer prognosis using radiomics are being actively conducted [15,16].
Therefore, this study aimed to develop a prediction model that could differentiate positive PD-L1 expression from negative PD-L1 expression through a radiomics-based investigation of CT images of patients with urothelial carcinoma.
This study was approved by the Ethics Committee of the Pusan National University Hospital (IRB No. 2202-021-112). Owing to the retrospective study design, the requirement for informed consent was waived.
Using an institutional electronic database, we retrospectively identified patients with urothelial carcinoma who had undergone PD-L1 immunohistochemical staining between January 2013 and September 2019. The following patients were included in this study: (1) patients with detailed pathological reports for PD-L1 immunohistochemical staining, and (2) patients who underwent CT within 1 month before the pathological examination. Among the 67 eligible patients, we excluded patients according to the following criteria: (1) patients who underwent chemotherapy between the CT scan and pathological examination and (2) patients with lesions that were too small to be detected on CT. Ultimately, 64 patients were included. The inclusion and exclusion criteria and the patient enrollment flowchart are shown in Fig. 1.
PD-L1 tumor-infiltrating immune cell scoring was performed using immunohistochemical staining for PD-L1 protein, which was considered positive if PD-L1 expression was >5% [17]. Consequently, 14 of the 64 patients were PD-L1-positive (PD-L1-positive group), and the remaining 50 were classified as negative (PD-L1-negative group).
We obtained CT images from the enrolled patients with urothelial carcinoma prior to obtaining tissue that was subjected to PD-L1 immunohistochemistry. Using a 64-channel multidetector row CT scanner (Discovery CT 750 HD, GE Medical System), CT images were acquired 90 seconds after the administration of 150 mL iodixanol (Visipaque, GE Healthcare) at a rate of 3 mL/s. As the single-phase contrast-enhanced CT of our hospital uses a fixed time-delay technique that acquires images 90 seconds after contrast media injection, 90-second images were selected to unify the acquisition time of all CT images. The CT parameters were as follows: tube voltage, 120 kVp; tube current adjusted by automatic exposure control system; voxel spacing, 0.6875 mm×0.6875 mm×3.75 mm; slice thickness, 3.75 mm; field of view, 369–450 mm; matrix, 512×512.
The acquired CT images were analyzed using radiomics software (Radiomics 1.3.0, Siemens Healthineers on a research platform (Syngo via VB40B, Research Frontier, Siemens Healthineers,). For enhancing tumors that appeared to be cancerous on CT, two experienced radiologists (CML and SBH; 5 years and 10 years of experience in urologic imaging, respectively), blinded to the results of PD-L1 immunohistochemical staining, were involved in segmentation. For the whole tumor volume, two experienced radiologists (CML and SBH), blinded to the results of PD-L1 immunohistochemical staining, manually drew the region of interest (ROI) along the border of the tumor on each consecutive slice. Therefore, a three-dimensional volume of interest was acquired (Fig. 2). Any inter-reader disagreements were resolved by consensus.
Radiomic features were extracted from the CT imaging data using manually segmented ROIs. Radiomics analysis was performed using radiomics software on a research platform. The computation of radiomic features was based on the PyRadiomics library, which is compliant with the image biomarker standardization initiative. A variety of options, including Laplacian of Gaussian filtering, wavelet filtering, and nonlinear intensity transforms, including square, square root, logarithms, and exponentials, were provided by the software to customize image pre-processing before feature extraction. To address unbalanced and small data (14 patients, PD-L1-positive group; 50 patients, PD-L1-negative group), random oversampling methods were used (50 patients, PD-L1-positive group; 50 patients, PD-L1-negative group).
The clinical characteristics of the PD-L1-positive and -negative groups were compared using the chi-square test or Fisher exact test. Univariate and multivariate logistic regression analyses were performed using radiomic features extracted from CT images to identify significant radiomic features for predicting the PD-L1 expression rate. A comprehensive model with significant radiomic features was obtained using logistic regression analysis. Receiver operating characteristic (ROC) analysis was performed for the aforementioned model. Accordingly, the sensitivity, specificity, positive likelihood ratio, and negative likelihood ratio for predicting the PD-L1 immunohistochemically positive group were calculated. We assessed the reproducibility of the texture analysis using the intraclass correlation coefficient (ICC) test. The agreement was defined as poor (ICC, 0–0.4), fair to good (ICC, 0.40–0.75), or excellent (ICC, >0.75). Statistical significance was set at p<0.05. All statistical analyses were performed using SPSS version 22 (IBM Corp.).
We enrolled 64 patients (52 men and 12 women; mean age, 74.0 years; standard deviation, 8.4 years). Among the 64 patients, 14 were included in the PD-L1-positive group. The remaining 50 were included in the PD-L1-negative group. The two groups did not significantly differ in terms of age, sex, site of mass, or histological grade. The characteristics of the two groups are summarized in Table 1.
For each patient, 1,691 radiomic features were extracted, including 324 first-order, 17 shape, and 1,350 texture features (432 gray-level co-occurrence matrix [GLCM] features, 288 gray-level size-zone matrix [GLSZM] features, 288 gray-level run-length matrix [GLRLM] features, 252 gray-level dependence matrix features, and 90 neighboring gray tone difference matrix features). The expression patterns of all 1,691 radiomic features extracted using the radiomics software are shown in Fig. 3. This heat map represents a color-coded array of feature values (y-axis) for all cases (x-axis). For visualization, each radiomic feature was normalized based on all 100 cases.
For the extracted radiomics features, the inter-reader agreement was excellent (ICC, 0.85–0.99).
Univariate logistic regression analysis of the extracted CT radiomic features revealed 782 features with a significant difference between the PD-L1 immunohistochemical staining positive and negative groups, with a p<0.05. The results are presented in Supplementary Table 1.
In the multivariate logistic regression analysis, using the results of the univariate analysis, wavelet-LLH_GLSZM_SmallAreaEmphasis (SAE) (odds ratio: 21.6224, p<0.001), wavelet-LLH_firstorder_Energy (odds ratio: 0.0044, p<0.001), log-sigma-0-5-mm-3D_GLSZM_SmallAreaHighGrayLevelEmphasis (SAHGLE) (odds ratio: –4.7389, p=0.004), original_shape_Maximum2DDiameterColumn (M2DC) (odds ratio: 0.0573, p=0.004), wavelet-LLL_GLRLM_ShortRunEmphasis (SRE) (odds ratio: –29.5892, p=0.002), and exponential_firstorder_Kurtosis (odds ratio: 0.4324, p=0.03) were selected. When other parameters were superimposed, the p-values were >0.05 (Table 2).
Therefore, the radiomics signature was –4.0934+21.6224 (wavelet-LLH_GLSZM_SAE)+0.0044 (wavelet-LLH_firstorder_Energy)–4.7389 (log-sigma-0-5-mm-3D_GLSZM_SAHGLE)+0.0573 (original_shape_M2DC)–29.5892 (wavelet-LLL_GLRLM_SRE)–0.4324 (exponential_firstorder_Kurtosis).
ROC analysis was performed using a model that represented the radiomics signature. The cutoff value obtained through ROC analysis was 0.67. The area under the ROC curve (AUC) was 0.96 (Fig. 4). If cases represented the radiomics signature ≥0.67 in pathologically PD-L1-positive and negative cases, they were regarded as true positive and false positive, respectively. The sensitivity, specificity, positive likelihood ratio, and negative likelihood ratio of our prediction model for differentiating the positive PD-L1 immunohistochemical staining group from the other group were 94.00%, 94.00%, 15.67, and 0.06, respectively (Table 3).
Several recent studies have used CT radiomics to determine PD-1/PD-L1 expression or immunotherapy for solid organ cancer [18-21]. However, they performed radiomics analyses for patients with no urothelial tumor [18-20]. Park et al. [21] showed the predictability of treatment response and survival outcome through CT image radiomics analysis in a patient group who underwent PD-1 and PD-L1 targeting immunotherapy for metastatic urothelial carcinoma. To our knowledge, this is the first study to predict PD-L1 expression in urothelial carcinoma through CT image radiomics analysis, and has significance in this respect. In this study, a final signature equation –4.0934+21.6224 (wavelet-LLH_GLSZM_SAE)+0.0044 (wavelet-LLH_firstorder_Energy)–4.7389 (log-sigma-0-5-mm-3D_GLSZM_SAHGLE)+0.0573 (original_shape_M2DC)–29.5892 (wavelet-LLL_GLRLM_SRE)–0.4324 (exponential_firstorder_Kurtosis) was obtained. The cutoff value (0.67) was obtained through ROC analysis of the aforementioned signature equation. The AUC was 0.96. The sensitivity, specificity, positive likelihood ratio, and negative likelihood ratio of PD-L1-positive prediction using this model were 94.00%, 94.00%, 15.67, and 0.06, respectively. Although our positive PD-L1 group was small (14 out of 64 patients), it can be said that preliminary results were obtained through high AUC values and significant statistical results.
The radiomic features constituting the signature equation in this study included wavelet-LLH_GLSZM_SAE and wavelet-LLH_firstorder_Energy. The significant radiomic features in this study are those from the wavelet filter, which separates the information of high and low spatial frequencies. LLH can be interpreted as a high-pass sub-band with a directional filter (e.g., a high-pass filter along the z-direction and a low-pass filter along the x- and y-directions). GLSZM is a gray-level zone matrix, a texture-based feature that demonstrates the sizes of contiguous regions sharing the same signal intensity after discretization. Small-area emphasis measures the distribution of small-sized areas, and the value increases as there are many small areas, or the overall texture of the area is similarly distributed. The energy feature is made of the value of the voxel magnitude in an image. The total energy is a value that adjusts these energy features according to the volume of the voxel [22-24].
In a CT radiomics study of PD-L1 expression in advanced lung adenocarcinoma, the predictability of PD-L1 expression was investigated using radiomics analysis in a group of patients with advanced-stage lung adenocarcinoma. As a result of the analysis, four radiomics features were selected, and the signature equation was −(1.59423)+Texture_GLCM_ASM×−8.49568+Texture_GLRLM_Run variance×3.58597+Texture_ GLRLM_ Run entropy×(−5.01416)+Texture_GLRLM_ Short Run high gray-level emphasis×0.05253 [20]. The significant radiomic features in this study differed from those in our study. The aforementioned study was performed in patients with advanced lung adenocarcinoma but not urothelial carcinoma. To the best of our knowledge, no study has demonstrated a radiomics prediction model for urothelial cancer.
In bladder cancer, when PD-L1 is expressed at more than 5%, it is generally associated with resistance to Bacillus Calmette-Guérin therapy, and it is known that recurrence after surgery is frequent, and the prognosis is poor [25,26]. Therefore, if we can predict PD-L1 expression with radiomics in advance, the prognosis can be improved using other therapeutic methods, such as immune checkpoint inhibitors.
Atezolizumab was the first PD-L1 inhibitor to show clinical activity in metastatic bladder cancer [27]. In the cohort with locally advanced or metastatic urothelial cancer after platinum chemotherapy, if surgery was not possible, the ORR of atezolizumab in the PD-L1-positive group significantly improved. In addition, the 12-month overall survival rate of the entire patient group was 37%, whereas that of the PD-L1-positive patient group was 50% [10,11]. Therefore, if PD-L1 expression can be predicted by analyzing the CT images of patients with urothelial cancer, immunotherapy can be considered without unnecessary invasive biopsy, which is expected to be useful in clinical practice.
In this study, the acquired CT images were analyzed using radiomics software on a research platform as known as Syngo via. This software has already been widely used (not in house software). Syngo via, using Python programing language, support for DICOM images. It provides the robust ROI definition with Segmentation Tools, including various tools for precise segmentation of CT images. In addition, it can display the segmentation with original CT images, providing the comprehensive visualization for analyzer. Model building for segmentation data is also provided in Syngo via. For image processing, a variety of options, including Laplacian of Gaussian filtering, wavelet filtering, and nonlinear intensity transforms, were provided by the software to customize image pre-processing before feature extraction. For discretization, Syngo via has the FBS (Fixed Bin Size) option [28].
This study had several limitations. First, this retrospective study included patients with urothelial carcinoma who underwent PD-L1 immunohistochemical staining between January 2013 and September 2019. Therefore, there was a selection bias in the patient group. In addition, only radiomics analysis was performed for the images scanned 90 seconds after contrast media injection. For the 64 patients with detailed pathological reports of PD-L1 immunohistochemical staining, CT examination was performed using various protocols. However, all CT examinations included images of the portal venous phase (at 90 seconds). Therefore, we only analyzed images scanned 90 seconds after the contrast injection. Second, the study population included patients with urothelial carcinoma; however, the sites of each mass were diverse, including the renal pelvis, ureter, and bladder. Future investigations should concentrate on more uniform patient populations. Third, there is a limitation in that validation through the control for the signature equation was not performed because of the small number of included patients. In addition, to tackle unbalanced and small data, random over sampling methods, which may result in overation of AUC, was used in this study. Therefore, further studies with larger sample sizes, which can be externally validated, are required.
In conclusion, the radiomics prediction model derived from contrast-enhanced CT can effectively differentiate immunohistochemically PD-L1-positive individuals from other patient groups. The CT radiomics model may be a potential tool for diagnosing PD-L1-positivity in patients with urothelial cancer.
Supplementary material
Supplementary materials are available at https://doi.org/10.7180/kmj.24.103
Supplementary Table 1. Univariate logistic regression analysis of the computed tomography radiomics-based features
Notes
Author contributions
Conceptualization: SK, JYK. Data curation: CML, SBH, JYK. Formal analysis: CML, SBH, JYK. Funding acquisition: SK. Investigation: CML, JYK. Methodology: SBH, JYK. Supervision: SK, JYK. Validation: SK, JYK. Visualization: CML, SBH. Writing - original draft: CML, SBH. Writing - review & editing: SK, HKH, KHK, BJK, NKL. All authors read and approved the final manuscript.
References
1. Antoni S, Ferlay J, Soerjomataram I, Znaor A, Jemal A, Bray F. Bladder cancer incidence and mortality: a global overview and recent trends. Eur Urol. 2017; 71:96–108.


2. Bray F, Ferlay J, Soerjomataram I, Siegel RL, Torre LA, Jemal A. Global cancer statistics 2018: GLOBOCAN estimates of incidence and mortality worldwide for 36 cancers in 185 countries. CA Cancer J Clin. 2018; 68:394–424.
3. Seront E, Machiels JP. Molecular biology and targeted therapies for urothelial carcinoma. Cancer Treat Rev. 2015; 41:341–53.


4. Oing C, Rink M, Oechsle K, Seidel C, von Amsberg G, Bokemeyer C. Second line chemotherapy for advanced and metastatic urothelial carcinoma: vinflunine and beyond: a comprehensive review of the current literature. J Urol. 2016; 195:254–63.
5. Rouanne M, Loriot Y, Lebret T, Soria JC. Novel therapeutic targets in advanced urothelial carcinoma. Crit Rev Oncol Hematol. 2016; 98:106–15.


6. Balar AV, Castellano D, O’Donnell PH, Grivas P, Vuky J, Powles T, et al. First-line pembrolizumab in cisplatin-ineligible patients with locally advanced and unresectable or metastatic urothelial cancer (KEYNOTE-052): a multicentre, single-arm, phase 2 study. Lancet Oncol. 2017; 18:1483–92.


7. Davarpanah NN, Yuno A, Trepel JB, Apolo AB. Immunotherapy: a new treatment paradigm in bladder cancer. Curr Opin Oncol. 2017; 29:184–95.
8. Bidnur S, Savdie R, Black PC. Inhibiting immune checkpoints for the treatment of bladder cancer. Bladder Cancer. 2016; 2:15–25.


9. Bellmunt J, de Wit R, Vaughn DJ, Fradet Y, Lee JL, Fong L, et al. Pembrolizumab as second-line therapy for advanced urothelial carcinoma. N Engl J Med. 2017; 376:1015–26.


10. Rosenberg JE, Hoffman-Censits J, Powles T, van der Heijden MS, Balar AV, Necchi A, et al. Atezolizumab in patients with locally advanced and metastatic urothelial carcinoma who have progressed following treatment with platinum-based chemotherapy: a single-arm, multicentre, phase 2 trial. Lancet. 2016; 387:1909–20.


11. Loriot Y, Rosenberg JE, Powles TB, Necchi A, Hussain S, Morales R, et al. Atezolizumab (atezo) in platinum (plat)-treated locally advanced/metastatic urothelial carcinoma (mUC): updated OS, safety and biomarkers from the Ph II IMvigor210 study. Ann Oncol. 2016; 27:vi270.


12. Gillies RJ, Anderson AR, Gatenby RA, Morse DL. The biology underlying molecular imaging in oncology: from genome to anatome and back again. Clin Radiol. 2010; 65:517–21.
13. Aerts HJ, Velazquez ER, Leijenaar RT, Parmar C, Grossmann P, Carvalho S, et al. Decoding tumour phenotype by noninvasive imaging using a quantitative radiomics approach. Nat Commun. 2014; 5:4006.


14. Bak SH, Park H, Lee HY, Kim Y, Kim HL, Jung SH, et al. Imaging genotyping of functional signaling pathways in lung squamous cell carcinoma using a radiomics approach. Sci Rep. 2018; 8:3284.


15. Huang YQ, Liang CH, He L, Tian J, Liang CS, Chen X, et al. Development and validation of a radiomics nomogram for preoperative prediction of lymph node metastasis in colorectal cancer. J Clin Oncol. 2016; 34:2157–64.


16. Xu X, Zhang HL, Liu QP, Sun SW, Zhang J, Zhu FP, et al. Radiomic analysis of contrast-enhanced CT predicts microvascular invasion and outcome in hepatocellular carcinoma. J Hepatol. 2019; 70:1133–44.


17. Bellmunt J, Mullane SA, Werner L, Fay AP, Callea M, Leow JJ, et al. Association of PD-L1 expression on tumor-infiltrating mononuclear cells and overall survival in patients with urothelial carcinoma. Ann Oncol. 2015; 26:812–7.
18. Sun R, Limkin EJ, Vakalopoulou M, Dercle L, Champiat S, Han SR, et al. A radiomics approach to assess tumour-infiltrating CD8 cells and response to anti-PD-1 or anti-PD-L1 immunotherapy: an imaging biomarker, retrospective multicohort study. Lancet Oncol. 2018; 19:1180–91.


19. Nardone V, Tini P, Pastina P, Botta C, Reginelli A, Carbone SF, et al. Radiomics predicts survival of patients with advanced non-small cell lung cancer undergoing PD-1 blockade using Nivolumab. Oncol Lett. 2020; 19:1559–66.


20. Yoon J, Suh YJ, Han K, Cho H, Lee HJ, Hur J, et al. Utility of CT radiomics for prediction of PD-L1 expression in advanced lung adenocarcinomas. Thorac Cancer. 2020; 11:993–1004.


21. Park KJ, Lee JL, Yoon SK, Heo C, Park BW, Kim JK. Radiomics-based prediction model for outcomes of PD-1/PD-L1 immunotherapy in metastatic urothelial carcinoma. Eur Radiol. 2020; 30:5392–403.


22. Pyradiomics Community. Open-source python package for the extraction of Radiomics features from medical imaging [Internet]. Pyradiomics Community;c2016. [cited 2022 Nov 30]. https://pyradiomics.readthedocs.io/en/latest/index.html.
23. Shur JD, Doran SJ, Kumar S, Ap Dafydd D, Downey K, O’Connor JP, et al. Radiomics in oncology: a practical guide. Radiographics. 2021; 41:1717–32.
24. Hou Z, Yang Y, Li S, Yan J, Ren W, Liu J, et al. Radiomic analysis using contrast-enhanced CT: predict treatment response to pulsed low dose rate radiotherapy in gastric carcinoma with abdominal cavity metastasis. Quant Imaging Med Surg. 2018; 8:410–20.


25. Nakanishi J, Wada Y, Matsumoto K, Azuma M, Kikuchi K, Ueda S. Overexpression of B7-H1 (PD-L1) significantly associates with tumor grade and postoperative prognosis in human urothelial cancers. Cancer Immunol Immunother. 2007; 56:1173–82.


26. Inman BA, Sebo TJ, Frigola X, Dong H, Bergstralh EJ, Frank I, et al. PD-L1 (B7-H1) expression by urothelial carcinoma of the bladder and BCG-induced granulomata: associations with localized stage progression. Cancer. 2007; 109:1499–505.


27. Powles T, Eder JP, Fine GD, Braiteh FS, Loriot Y, Cruz C, et al. MPDL3280A (anti-PD-L1) treatment leads to clinical activity in metastatic bladder cancer. Nature. 2014; 515:558–62.


28. Paquier Z, Chao SL, Acquisto A, Fenton C, Guiot T, Dhont J, et al. Radiomics software comparison using digital phantom and patient data: IBSI-compliance does not guarantee concordance of feature values. Biomed Phys Eng Express. 2022; 8:065008.
Fig. 1.
Flow diagram of the inclusion and exclusion criteria. CT, computed tomography; PD-L1, programmed death ligand 1.
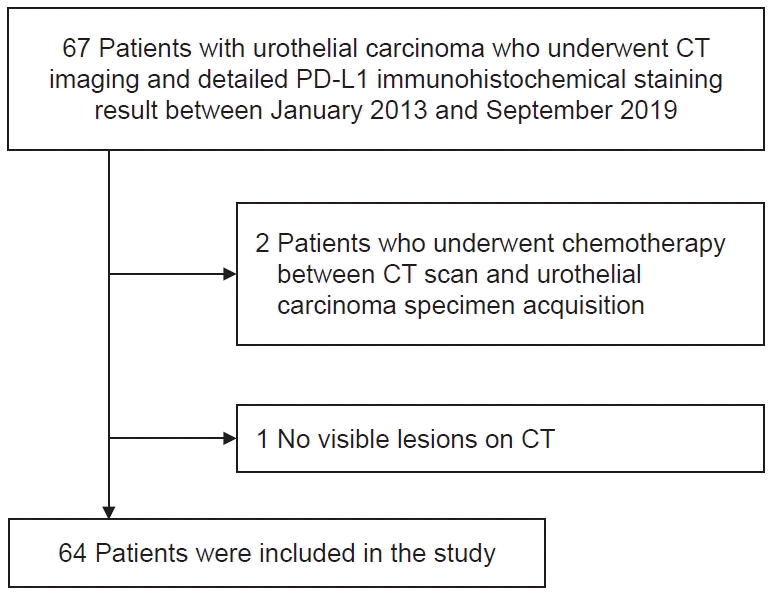
Fig. 2.
Three-dimensional tumor segmentation procedure for urothelial carcinoma of the kidney (A), ureter (B), and bladder (C) from three patients. The segmentation was performed by manually drawing the regions of interest on the entire mass in 90-second delayed computed tomography images.
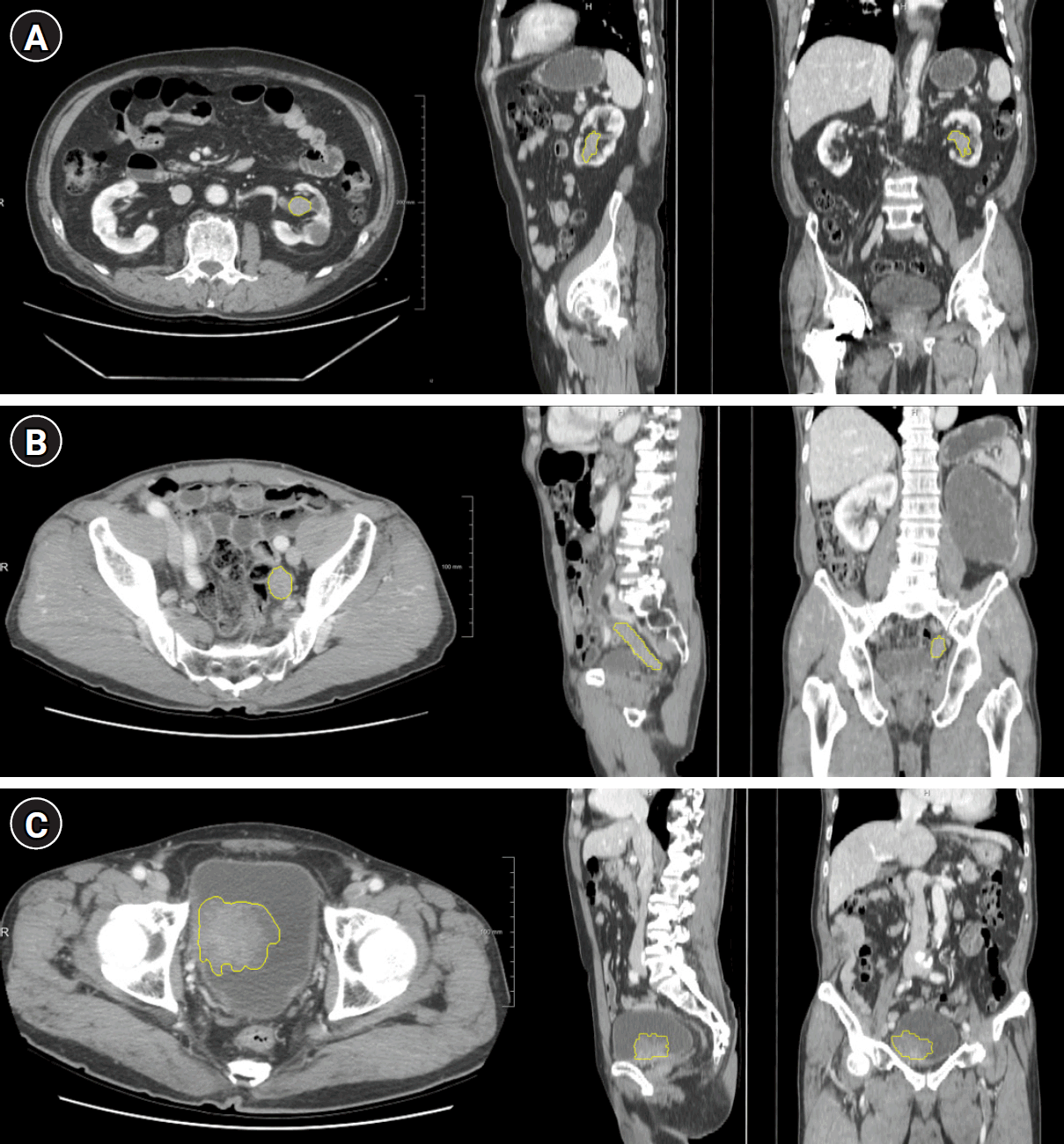
Fig. 3.
Radiomics heat map. Clustering of patients with urothelial cancer on the x-axis and computed tomography radiomics-based features on the y-axis.
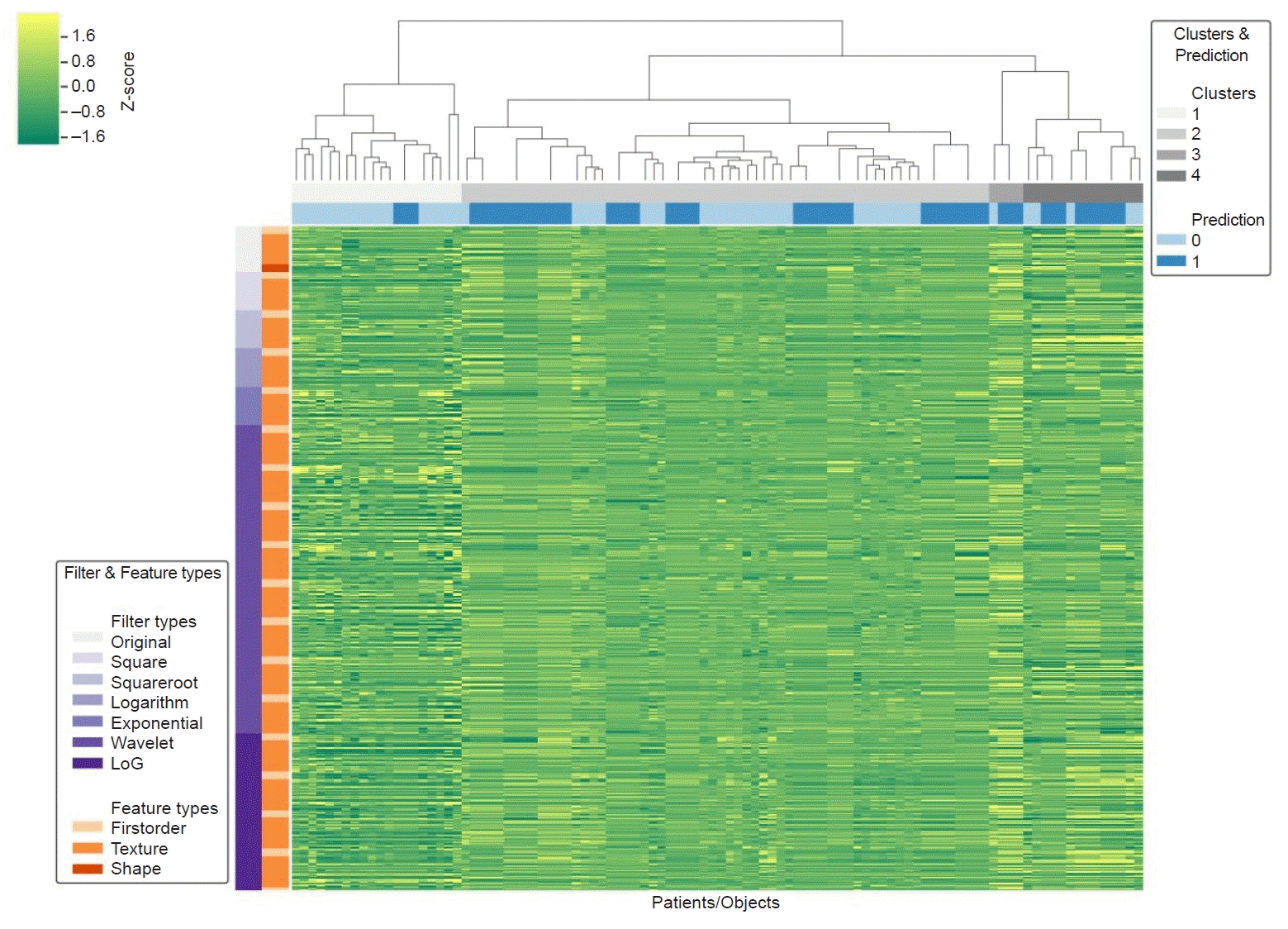
Fig. 4.
Receiver operating characteristic (ROC) curve of the radiomics-based model for predicting immunohistochemical positivity for programmed death ligand 1.
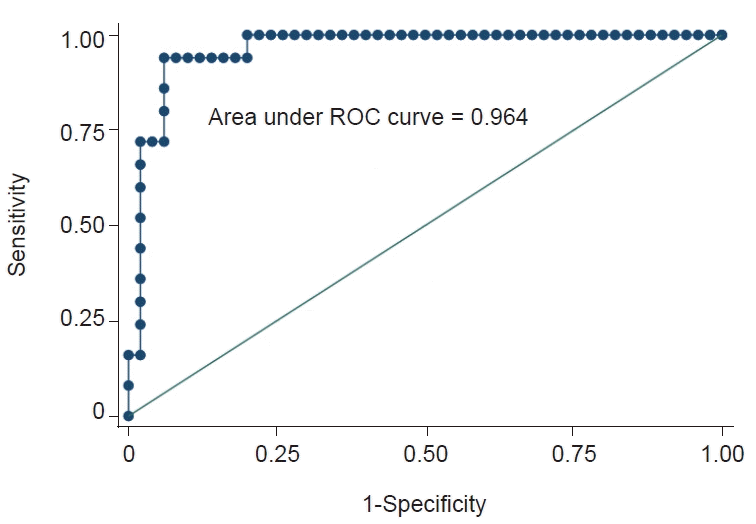
Table 1.
Demographic and clinical characteristics of the included patients (n=64)
Table 2.
Multivariate logistic regression analysis to predict PD-L1 immunohistochemical positivity
Table 3.
Diagnostic performance of the model for PD-L1 expression in urothelial cancer