Abstract
Large language models (LLMs) are rapidly transforming medical writing and publishing. This review article focuses on experimental evidence to provide a comprehensive overview of the current applications, challenges, and future implications of LLMs in various stages of academic research and publishing process. Global surveys reveal a high prevalence of LLM usage in scientific writing, with both potential benefits and challenges associated with its adoption. LLMs have been successfully applied in literature search, research design, writing assistance, quality assessment, citation generation, and data analysis. LLMs have also been used in peer review and publication processes, including manuscript screening, generating review comments, and identifying potential biases. To ensure the integrity and quality of scholarly work in the era of LLM-assisted research, responsible artificial intelligence (AI) use is crucial. Researchers should prioritize verifying the accuracy and reliability of AI-generated content, maintain transparency in the use of LLMs, and develop collaborative human-AI workflows. Reviewers should focus on higher-order reviewing skills and be aware of the potential use of LLMs in manuscripts. Editorial offices should develop clear policies and guidelines on AI use and foster open dialogue within the academic community. Future directions include addressing the limitations and biases of current LLMs, exploring innovative applications, and continuously updating policies and practices in response to technological advancements. Collaborative efforts among stakeholders are necessary to harness the transformative potential of LLMs while maintaining the integrity of medical writing and publishing.
The rapid advancement of generative artificial intelligence (AI) is transforming the landscape of scientific research and academic writing [1]. Large language models (LLMs), such as ChatGPT, Claude, Copilot and Gemini, have demonstrated remarkable capabilities in understanding and generating human-like text. These models are trained on vast amounts of data, allowing them to assist researchers with various tasks, from literature analysis and content generation to language translation and also peer review and publication processes [2,3]. The rapid improvements in model algorithms and the increasing computational power dedicated to running these models are outpacing Moore's Law [4]. As these LLMs are becoming more sophisticated and prevalent in academic publishing, its implications for research integrity and establishing appropriate policies and guidelines has become increasingly important.
As LLMs become increasingly integrated into the research and writing process (Fig. 1), concerns have arisen regarding the quality, accuracy, and transparency of AI-generated content [5]. The scientific community has engaged in debates about the appropriate use of these tools, particularly in light of incidents such as the listing of ChatGPT as an author [6]. Despite the rapid adoption of LLMs, a recent study found that only 18% of the top 100 Korean medical journals had explicit policies addressing their use as of March 2024 [7]. This lack of clear guidelines highlights the need for the scientific community to develop well-defined, realistic, and coherent policies that promote the responsible and productive integration of AI in academic endeavors [8].
The aim of this review article is to provide a comprehensive overview of the current state of LLMs in medical writing and publishing, focusing on experimental evidence rather than perspective papers. By examining the actual capabilities and limitations of these tools, as well as the ethical considerations surrounding their use, this review seeks to inform policy decisions and guide the responsible integration of LLMs in research. The article will explore the applications of LLMs in various stages of the research process, including literature analysis, content generation, and peer review. Additionally, recommendations for researchers, reviewers, and editorial offices will be provided to ensure the integrity and quality of AI-assisted academic work.
The use of LLMs has become increasingly prevalent in academia, particularly in biomedical and clinical sciences [9]. A global survey conducted by Nature in July 2023 found that about one-third (31%) of postdoc respondents reported using AI chatbots for tasks such as refining text, generating or editing code, and managing literature in their fields [10]. Similarly, a global survey of 456 urologists in May 2023 revealed that 47.7% use LLMs [11]. There has been a significant increase in the suspected use of LLMs for writing articles submitted to an orthopedic journal, with 41.0% of articles having suspected AI use over 10% [12]. The median probability of AI-generated abstracts increased from 3.8% to 5.7% in 2022 and 2023 across Q1 journals in medical imaging [13]. Moreover, evidence of AI use in reviews was found in a study of AI conference peer reviews that took place after the release of ChatGPT, suggesting that between 6.5% and 16.9% of reviews have been substantially modified by LLMs [14].
The use of LLM tools in academic writing has been associated with perceived benefits and efficiency gains in the research and writing process [10]. A quantitative study found that incorporating ChatGPT into the workflow for professional writing tasks reduced the average time taken by 40% and increased output quality by 18% [15]. This potential for increased productivity and output quality has been a driving factor in the adoption of LLMs, especially given the growing pressure on researchers to increase their research productivity and output [16].
However, the ease with which LLMs can generate convincing academic content has raised concerns about the potential for misuse and fraud. One study demonstrated that GPT-3 can create a highly convincing fraudulent article resembling a genuine scientific paper in terms of word usage, sentence structure, and overall composition, all within just 1 h and without any special training of the user [17]. Similarly, another study in early 2023 used ChatGPT-4 to generate 2 fake orthopedic surgery papers, with one passing review and being accepted, and the other being rejected but referred to another journal for consideration [18].
The challenges in detecting AI-generated content further complicate the issue. In a study where ChatGPT-3.5 generated 50 fake research abstracts from titles, only 8% met specific formatting criteria, yet achieved a 100% originality score in plagiarism detectors [19]. While AI detectors identified them as AI-created, human reviewers correctly spotted only 68% as AI-crafted and mistakenly tagged 14% of original abstracts as such. This highlights the nuanced challenges and considerations in integrating AI into academic writing while upholding scientific rigor.
The lack of unified guidelines and unclear policies regarding the extent of AI tool usage considered acceptable has left researchers in a state of uncertainty [8]. The term "use of AI" encompasses a wide spectrum of applications, ranging from providing a keyword to generate an entire manuscript, listing items to be mentioned and converting them into paragraphs, or strictly using AI for typo and punctuation correction only. The difficulty in detecting AI-generated content and the high risk of false-positives, especially for non-native English writing, further compound the issue [20]. The varying results of LLM usage rates in studies from the previous section underscore the challenges in detection and the need for more robust and standardized methods.
AI tools have demonstrated potential in assisting researchers with literature searches and systematic reviews (Table 1). For instance, ChatGPT-3.5 and ChatGPT-4 were used to generate PICO-based search queries in the field of orthodontics, showcasing their ability to aid the systematic review process [21]. In another study, ChatGPT-3.5 was employed to generate 50 topics in medical research and create a research protocol for each topic, with an 84% accuracy rate of references [22]. Additionally, ChatGPT-4 was used to analyze 2,491 abstracts published in European Resuscitation Council conferences, highlighting its capabilities in bibliometric analysis of academic abstracts and its potential impact on academic writing and publishing [23].
LLMs have been extensively applied in various aspects of writing assistance, particularly in abstract generation (Table 1). ChatGPT-3.5 demonstrated the ability to generate high-quality abstracts from clinical trial keywords and data tables, showcasing impressive accuracy with minor errors [24]. However, its performance varied significantly when tasked with writing abstracts on broad, well-documented topics compared to more specific, recently published subjects [25]. The low plagiarism scores and difficult detection of AI-generated abstracts and the ethical boundaries of using such technology in academic writing have also been discussed [19]. Although ChatGPT-3.5 could generate abstracts that were challenging to distinguish from human-written ones in the arthroplasty field, the quality was notably better in those written by humans [26]. Using both ChatGPT-3.5 and ChatGPT-4 to write abstracts for randomized controlled trials revealed that, despite their potential, the quality was not satisfactory, highlighting the need for further development and refinement in generative AI tools [27].
In addition to abstract generation, LLMs have been used to assist in various other writing tasks. For example, GPT-4 was used to generate introduction sections for randomized controlled trials, with non-inferiority confirmed and higher readability scores compared to human-written introductions [28]. ChatGPT was also used to write medical case reports [29] and to write a clinical summary containing patient situation, case evaluation and appropriate interventions [30]. In a study regarding human reproduction, ChatGPT could produce high-quality text and efficiently summarize information, but its ability to interpret data and answer scientific questions was limited [31].
LLMs have been employed to generate cover letters for abstracts, with non-inferiority confirmed by randomized trials and higher readability scores [32]. These tools have also been used to facilitate language learning and improve technical writing skills for non-native English speakers, which is particularly meaningful for scholars using English as a non-primary language [33]. However, it is important to note that the effectiveness of these tools may vary, as one study found that the free version of ChatGPT-3.5 was not an effective writing coach [34]. Interestingly, fine-tuning a language model to an author's previous works can also enhance academic writing, especially for generating text and ideas related to the scholar's prior work, offering a personalized approach to writing assistance [35].
Citation and reference generation is another area where LLMs have been applied, albeit with varying levels of success (Table 1). In a study conducted in early 2023, researchers generated 50 references for 10 common topic keywords relevant to head and neck surgery, finding that only 10% of the generated references were accurate [36]. However, in a study comparing the performance between multiple LLM-based tools, ChatGPT-3.5 outperformed Bing Chat (old version of Microsoft Copilot) and Google Bard (old version of Google Gemini) with a 38% accuracy rate in nephrology reference generation [37]. ChatGPT-4 showed substantial improvements, achieving a 74.3% correct reference rate for otolaryngology topics [38] and a high accuracy rate ranging from 73% to 87% for generating full citations of the most cited otolaryngology papers [39].
Despite these advancements, the lack of a fact-checking step in the text generation algorithms of LLMs leads to inherent inaccuracies in reference generation, suggesting that incorporating techniques such as retrieval-augmented generation is crucial to enhance reliability [40]. Specific tools tailored for article search, such as Perplexity, Elicit and Consensus can be used instead of LLM chatbots for general purpose. These tools analyze the researcher's input using LLMs and retrieve related articles from a scholarly database, thereby reducing the likelihood of generating non-existent references. A tutorial on how to utilize LLM-based tools for each stage of article writing is provided in Supplementary Data 1.
LLMs have shown promise in code generation and data analysis, potentially impacting life sciences education and research by allowing researchers to collaborate with such models to produce functional code [41]. For example, ChatGPT-4 was tested to build two cancer economic models, demonstrating that AI can automate health economic model construction, potentially accelerating development timelines and reducing costs [42]. Furthermore, the Code Interpreter feature in ChatGPT allows users to upload data files and ask the chatbot to perform data analysis using natural language interactions. The chatbot can read the data, plan steps for data analysis, write python code to perform the analysis, and visualize the results, effectively democratizing bioinformatics by breaking down the barrier of code writing [43,44]. These advancements suggest that when integrated with tools, LLMs have the potential to revolutionize the way researchers approach code generation and data analysis in science, making these processes more accessible, efficient, and cost-effective (Table 1).
Recent advancements in LLMs have demonstrated their potential to automate and accelerate scientific discovery across various domains. An approach for automatically generating and testing social scientific hypotheses using LLMs and structural causal models has been introduced [45]. This method enables the proposal and testing of causal relationships in simulated social interactions, providing insights that are not directly available through LLM elicitation alone. In the field of mathematics, an evolutionary procedure called FunSearch has been developed, which pairs a pretrained LLM with a systematic evaluator to surpass best-known results in complex problems [46]. Applying FunSearch to the cap set problem in extremal combinatorics led to the discovery of new constructions of large cap sets, pushing the boundaries of existing LLM-based approaches.
Moreover, an AI system driven by GPT-4, named Coscientist, has been showcased to autonomously design, plan, and perform complex experiments in chemistry [47]. Coscientist successfully optimized palladium-catalyzed cross-couplings, demonstrating the versatility and efficacy of AI systems in advancing research. These examples highlight the transformative potential of LLMs in automating and accelerating scientific discovery across various disciplines, from social sciences and mathematics to chemistry. As LLMs continue to evolve and become more sophisticated, their impact on research and scientific discovery is expected to grow, potentially revolutionizing the way researchers approach complex problems and accelerating the pace of innovation across multiple fields.
LLMs have shown potential in assisting with manuscript screening and quality assessment (Table 2). Studies have demonstrated their effectiveness in proofreading and error detection [48], as well as predicting peer review outcomes [49]. LLMs can also be used to assess the quality and risk of bias in systematic reviews [50] and develop grading systems for evaluating methodology sections [51]. These applications could be particularly beneficial for researchers from underprivileged regions who may lack access to timely and quality feedback mechanisms [52].
LLMs can assist reviewers in generating opinions and comments on manuscripts, potentially reducing reviewer fatigue and streamlining the peer review process [53]. A large-scale retrospective study comparing GPT-4 generated comments with human reviews found that AI-generated comments had a 31%–39% overlap with human reviewers, while inter-human overlap was 29%–35% [54]. Additionally, a prospective study revealed that 70% of scholars found AI comments to have at least partial alignment with human reviews, and 20% found AI feedback more helpful than human comments [54].
However, a relatively small study using 21 research papers and having 2 human reviewers and AI to give review comments showed that while ChatGPT-3.5 and ChatGPT-4.0 demonstrated good concordance with accepted papers, they provided overly positive reviews for rejected papers [55]. While these limitations should be acknowledged, the overall evidence suggests that LLMs hold great promise in revolutionizing the peer review process by generating valuable insights and reducing the workload of human reviewers, leading to a more efficient and comprehensive evaluation of manuscripts in the era of review shortage (Table 2) [56].
Despite the promising applications of LLMs in peer review, it is crucial to be aware of potential biases and limitations (Table 2). Studies have identified gender bias in LLM-generated recommendation letters [57], as well as biases related to nationality, culture, and demographics [58]. The overreliance on LLMs in peer review may lead to linguistic compression and reduced epistemic diversity, an essential element for the advancement of science [54]. Furthermore, LLMs may lack deep domain knowledge, especially in medical fields and may fail to detect minute errors in specific details [59,60]. To mitigate these issues, human oversight and final decision-making remain essential in the peer review process.
LLMs can be employed in various editorial office applications to manage submissions, detect plagiarism, and disseminate research findings (Table 2). AI-assisted tools can be used to prescreen manuscripts for quality and suitability, provide initial screening results to reviewers, and develop automated reviewer recommendation systems based on expertise. High-level plagiarism checks can be performed using LLMs, and can also help identify and address ethical issues.
To engage readers and promote broader dissemination of research, generative AI tools can generate plain language summaries, graphical abstracts, and personalized content recommendations. These tools can help break down complex scientific concepts into easily understandable language, making research findings more accessible to a wider audience with varying levels of scientific knowledge. Moreover, LLM-powered translation tools can help overcome language barriers by providing accurate translations of research articles, abstracts, and summaries, enabling the dissemination of scientific knowledge across different languages and cultures. This increased accessibility and reach can foster greater public engagement with science and facilitate interdisciplinary collaborations. As a demonstration of this application, the Chatbot Claude 3 Opus was provided with the abstracts of the recent issue of The Korean Journal of Physiology & Pharmacology (Volume 28 Number 3), and has been prompted to write both an editorial review article (Supplementary Data 2) and a plain language summary article in English and Korean (Supplementary Data 3).
However, it is important to consider data privacy concerns, such as the potential for manuscripts to unintentionally become training data for language models if proper precautions are not taken [8]. As LLMs continue to advance, its integration into the peer review and publication process is expected to grow. However, it is essential for the academic community to establish clear guidelines and best practices to ensure the responsible and ethical use of these tools, while maintaining the integrity and quality of scholarly publishing.
To ensure the responsible use of LLMs in medical writing, researchers should prioritize verifying the accuracy and reliability of LLM-generated content. A recent study on GPT-4V, a state-of-the-art LLM, highlights the challenges in this domain [61]. While GPT-4V outperformed human physicians in multi-choice accuracy on the New England Journal of Medicine (NEJM) Image Challenges, it frequently presented flawed rationales even when the answer was correct. This underscores the need for thorough fact-checking and cross-referencing with reliable sources, as well as being cognizant of subtle errors or inconsistencies that can be challenging to detect, especially in the medical context.
In terms of enhancing the research capabilities of individual researchers, it is recommended to utilize AI to generate advice or thought-provoking questions rather than to generate answers [62]. For instance, instead of asking the LLM chatbot to generate a manuscript from an outline or list of ideas, it is more beneficial to request guidance and explanations on how to improve a manually crafted draft. Considering that a scientific article holds value as an author's writing, the choice of words or expressions may be an integral part of its identity and possess unique value.
Maintaining transparency in the use of LLMs is crucial, and researchers should disclose the use of these tools in the research and writing process, providing details on the extent and nature of LLM assistance. Developing a collaborative human-AI workflow that leverages LLM's strengths while recognizing their limitations can help optimize the quality of the output. Researchers should iteratively work with LLMs and ensure proper human intervention and oversight in each step [7].
As LLMs become increasingly integrated into both the writing and review processes, and as AI tools can effectively screen for trivial errors such as grammar and formatting, reviewers should shift their focus to higher-order reviewing skills. This includes critically analyzing the overall significance, novelty, and impact of the work, providing nuanced feedback and domain-specific insights, and focusing on the "human" aspects of review [54]. It is important to note that while poor writing quality was previously associated with poor scientific quality, in the era of LLMs, the quality of writing may not necessarily reflect the scientific rigor of the work. Reviewers may also inevitably incorporate LLM-based tools in the peer review workflow, but need to keep in mind that proper vigilance is needed. There is evidence that in cases of overreliance, high-performance AI tools result in worse outcomes than low-performance AI tools with proper human stewardship [63]. Reviewers should be aware of the potential use of LLMs in manuscripts and ensure that conclusions are well-supported by data and analysis, rather than "hallucinated" claims. In cases of suspected unethical AI use, such as plagiarism or undisclosed LLM assistance, reviewers should act according to established reporting procedures and guidelines.
Editorial offices play a crucial role in promoting responsible LLM use in academic writing. Rather than banning AI based on fear, editorial offices should experience the capabilities of LLMs firsthand and develop evidence-based policies and guidelines that align with international standards (e.g., ICMJE, COPE, WAME). These policies should address key components such as AI authorship, disclosure of AI use, and human author responsibility [64]. Implementing robust screening and detection tools while embracing new technology and maintaining rigorous peer review standards is also important [65]. Editorial offices should acknowledge the prevalence of LLM use and focus on content quality and integrity. Providing training and resources for editorial staff and reviewers can help them navigate the challenges and opportunities presented by LLM technology.
Fostering open dialogue and collaboration within the academic community is another key responsibility of editorial offices. This can be achieved by promoting the exchange of ideas and experiences related to LLM use across different fields and disciplines, organizing workshops, seminars, or conferences to discuss challenges and opportunities, and engaging with AI researchers and developers to better understand LLM capabilities and limitations.
The rapid adoption and integration of LLMs in various stages of research and publishing have signaled a growing impact on academic writing and publishing. While LLMs offer potential benefits, they also present challenges for researchers, reviewers, and editorial offices. To harness the transformative potential of AI while maintaining the integrity of scholarly work, it is crucial to establish clear policies and guidelines that promote responsible and transparent use, fostering a culture of transparency and accountability, and encouraging open dialogue within the academic community. Future directions should focus on addressing the limitations and biases of current generative AI technologies, exploring innovative applications of LLMs, and continuously updating policies and practices. Collaborative efforts among researchers, reviewers, editorial offices, and AI developers will be essential in navigating the challenges and opportunities presented by LLMs. Ultimately, while embracing the potential of LLMs, it is important to prioritize the integrity of academic writing and publishing, emphasizing the importance of human judgment and expertise in the era of AI-assisted research and publishing.
Three supplementary data can be found with this article online at https://doi.org/10.4196/kjpp.2024.28.5.393
ACKNOWLEDGEMENTS
The generative AI chatbot Claude 3 Opus was used in the process of writing and revising the outline of the manuscript, as well as in the process of revising the wording and grammar of the manuscript.
Notes
REFERENCES
1. Wong F, Zheng EJ, Valeri JA, Donghia NM, Anahtar MN, Omori S, Li A, Cubillos-Ruiz A, Krishnan A, Jin W, Manson AL, Friedrichs J, Helbig R, Hajian B, Fiejtek DK, Wagner FF, Soutter HH, Earl AM, Stokes JM, Renner LD, et al. 2024; Discovery of a structural class of antibiotics with explainable deep learning. Nature. 626:177–185. DOI: 10.1038/s41586-023-06887-8. PMID: 38123686. PMCID: PMC10866013.
2. Cotton DRE, Cotton PA, Shipway JR. 2024; Chatting and cheating: ensuring academic integrity in the era of ChatGPT. Innov Educ Teach Int. 61:228–239. DOI: 10.1080/14703297.2023.2190148.
3. Carobene A, Padoan A, Cabitza F, Banfi G, Plebani M. 2023; Rising adoption of artificial intelligence in scientific publishing: evaluating the role, risks, and ethical implications in paper drafting and review process. Clin Chem Lab Med. 62:835–843. DOI: 10.1515/cclm-2023-1136. PMID: 38019961.
4. Ho A, Besiroglu T, Erdil E, Owen D, Rahman R, Guo ZC, Atkinson D, Thompson N, Sevilla J. 2024. Algorithmic progress in language models. arXiv:2403.05812 [Preprint]. Available from: https://doi.org/10.48550/arXiv.2403.05812. cited 2024 Mar 18.
5. Perkins M, Roe J. 2024; Academic publisher guidelines on AI usage: a ChatGPT supported thematic analysis. F1000Res. 12:1398. DOI: 10.12688/f1000research.142411.2. PMID: 38322309. PMCID: PMC10844801.
6. Thorp HH. 2023; ChatGPT is fun, but not an author. Science. 379:313. DOI: 10.1126/science.adg7879. PMID: 36701446.
7. Ahn S. 2024. Generative AI guidelines in Korean medical journals: a survey using human-AI collaboration. medRxiv [Preprint]. Available from: https://doi.org/10.1101/2024.03.08.24303960. cited 2024 Mar 15. DOI: 10.1101/2024.03.08.24303960.
8. Lin Z. 2024; Towards an AI policy framework in scholarly publishing. Trends Cogn Sci. 28:85–88. DOI: 10.1016/j.tics.2023.12.002. PMID: 38195365.
9. Raman R. 2023; Transparency in research: an analysis of ChatGPT usage acknowledgment by authors across disciplines and geographies. Account Res. 1–22. DOI: 10.1080/08989621.2023.2273377. PMID: 37877216.
10. Nordling L. 2023; How ChatGPT is transforming the postdoc experience. Nature. 622:655–657. DOI: 10.1038/d41586-023-03235-8. PMID: 37845528.
11. Eppler M, Ganjavi C, Ramacciotti LS, Piazza P, Rodler S, Checcucci E, Gomez Rivas J, Kowalewski KF, Belenchón IR, Puliatti S, Taratkin M, Veccia A, Baekelandt L, Teoh JY, Somani BK, Wroclawski M, Abreu A, Porpiglia F, Gill IS, Murphy DG, et al. 2024; Awareness and use of ChatGPT and large language models: a prospective cross-sectional global survey in urology. Eur Urol. 85:146–153. DOI: 10.1016/j.eururo.2023.10.014. PMID: 37926642.
12. Maroteau G, An JS, Murgier J, Hulet C, Ollivier M, Ferreira A. 2023; Evaluation of the impact of large language learning models on articles submitted to Orthopaedics & Traumatology: Surgery & Research (OTSR): a significant increase in the use of artificial intelligence in 2023. Orthop Traumatol Surg Res. 109:103720. DOI: 10.1016/j.otsr.2023.103720. PMID: 37866509.
13. Mese I. 2024; Tracing the footprints of AI in radiology literature: a detailed analysis of journal abstracts. Rofo. doi: 10.1055/a-2224-9230. [Epub ahead of print]. DOI: 10.1055/a-2224-9230. PMID: 38228155.
14. Liang W, Izzo Z, Zhang Y, Lepp H, Cao H, Zhao X, Chen L, Ye H, Liu S, Huang Z, McFarland DA, Zou JY. 2024. Monitoring AI-modified content at scale: a case study on the impact of ChatGPT on AI conference peer reviews. arXiv:2403.07183 [Preprint]. Available from: https://doi.org/10.48550/arXiv.2403.07183. cited 2024 Mar 18.
15. Noy S, Zhang W. 2023; Experimental evidence on the productivity effects of generative artificial intelligence. Science. 381:187–192. DOI: 10.1126/science.adh2586. PMID: 37440646.
16. Haven TL, Bouter LM, Smulders YM, Tijdink JK. 2019; Perceived publication pressure in Amsterdam: survey of all disciplinary fields and academic ranks. PLoS One. 14:e0217931. DOI: 10.1371/journal.pone.0217931. PMID: 31216293. PMCID: PMC6583945.
17. Májovský M, Černý M, Kasal M, Komarc M, Netuka D. 2023; Artificial intelligence can generate fraudulent but authentic-looking scientific medical articles: Pandora's box has been opened. J Med Internet Res. 25:e46924. DOI: 10.2196/46924. PMID: 37256685. PMCID: PMC10267787.
18. Brameier DT, Alnasser AA, Carnino JM, Bhashyam AR, von Keudell AG, Weaver MJ. 2023; Artificial intelligence in orthopaedic surgery: can a large language model "Write" a believable orthopaedic journal article? J Bone Joint Surg Am. 105:1388–1392. DOI: 10.2106/JBJS.23.00473. PMID: 37437021.
19. Gao CA, Howard FM, Markov NS, Dyer EC, Ramesh S, Luo Y, Pearson AT. 2023; Comparing scientific abstracts generated by ChatGPT to real abstracts with detectors and blinded human reviewers. NPJ Digit Med. 6:75. DOI: 10.1038/s41746-023-00819-6. PMID: 37100871. PMCID: PMC10133283.
20. Liang W, Yuksekgonul M, Mao Y, Wu E, Zou J. 2023; GPT detectors are biased against non-native English writers. Patterns (N Y). 4:100779. DOI: 10.1016/j.patter.2023.100779. PMID: 37521038. PMCID: PMC10382961.
21. Demir GB, Süküt Y, Duran GS, Topsakal KG, Görgülü S. 2024; Enhancing systematic reviews in orthodontics: a comparative examination of GPT-3.5 and GPT-4 for generating PICO-based queries with tailored prompts and configurations. Eur J Orthod. 46:cjae011. DOI: 10.1093/ejo/cjae011. PMID: 38452222.
22. Athaluri SA, Manthena SV, Kesapragada VSRKM, Yarlagadda V, Dave T, Duddumpudi RTS. 2023; Exploring the boundaries of reality: investigating the phenomenon of artificial intelligence hallucination in scientific writing through ChatGPT references. Cureus. 15:e37432. DOI: 10.7759/cureus.37432. PMID: 37182055. PMCID: PMC10173677.
23. Fijačko N, Creber RM, Abella BS, Kocbek P, Metličar Š, Greif R, Štiglic G. 2024; Using generative artificial intelligence in bibliometric analysis: 10 years of research trends from the European Resuscitation Congresses. Resusc Plus. 18:100584. DOI: 10.1016/j.resplu.2024.100584. PMID: 38420596. PMCID: PMC10899017.
24. Babl FE, Babl MP. 2023; Generative artificial intelligence: can ChatGPT write a quality abstract? Emerg Med Australas. 35:809–811. DOI: 10.1111/1742-6723.14233. PMID: 37142327. PMCID: PMC10946929.
25. Williams DO, Fadda E. 2023; Can ChatGPT pass Glycobiology? Glycobiology. 33:606–614. DOI: 10.1093/glycob/cwad064. PMID: 37531256.
26. Lawrence KW, Habibi AA, Ward SA, Lajam CM, Schwarzkopf R, Rozell JC. 2024; Human versus artificial intelligence-generated arthroplasty literature: A single-blinded analysis of perceived communication, quality, and authorship source. Int J Med Robot. 20:e2621. DOI: 10.1002/rcs.2621. PMID: 38348740.
27. Hwang T, Aggarwal N, Khan PZ, Roberts T, Mahmood A, Griffiths MM, Parsons N, Khan S. 2024; Can ChatGPT assist authors with abstract writing in medical journals? Evaluating the quality of scientific abstracts generated by ChatGPT and original abstracts. PLoS One. 19:e0297701. DOI: 10.1371/journal.pone.0297701. PMID: 38354135. PMCID: PMC10866463.
28. Sikander B, Baker JJ, Deveci CD, Lund L, Rosenberg J. 2023; ChatGPT-4 and human researchers are equal in writing scientific introduction sections: a blinded, randomized, non-inferiority controlled study. Cureus. 15:e49019. DOI: 10.7759/cureus.49019.
29. Buholayka M, Zouabi R, Tadinada A. 2023; The readiness of ChatGPT to write scientific case reports independently: a comparative evaluation between human and artificial intelligence. Cureus. 15:e39386. DOI: 10.7759/cureus.39386. PMID: 37378091. PMCID: PMC10292135.
30. Zhou Z. 2023; Evaluation of ChatGPT's capabilities in medical report generation. Cureus. 15:e37589. DOI: 10.7759/cureus.37589.
31. Semrl N, Feigl S, Taumberger N, Bracic T, Fluhr H, Blockeel C, Kollmann M. 2023; AI language models in human reproduction research: exploring ChatGPT's potential to assist academic writing. Hum Reprod. 38:2281–2288. DOI: 10.1093/humrep/dead207. PMID: 37833847.
32. Deveci CD, Baker JJ, Sikander B, Rosenberg J. 2023; A comparison of cover letters written by ChatGPT-4 or humans. Dan Med J. 70:A06230412.
33. Song C, Song Y. 2023; Enhancing academic writing skills and motivation: assessing the efficacy of ChatGPT in AI-assisted language learning for EFL students. Front Psychol. 14:1260843. DOI: 10.3389/fpsyg.2023.1260843. PMID: 38162975. PMCID: PMC10754989.
34. Lingard L, Chandritilake M, de Heer M, Klasen J, Maulina F, Olmos-Vega F, St-Onge C. 2023; Will ChatGPT's free language editing service level the playing field in science communication?: insights from a collaborative project with non-native English scholars. Perspect Med Educ. 12:565–574. DOI: 10.5334/pme.1246.
35. Porsdam Mann S, Earp BD, Møller N, Vynn S, Savulescu J. 2023; AUTOGEN: a personalized large language model for academic enhancement-ethics and proof of principle. Am J Bioeth. 23:28–41. DOI: 10.1080/15265161.2023.2233356. PMID: 37487183.
36. Wu RT, Dang RR. 2023; ChatGPT in head and neck scientific writing: a precautionary anecdote. Am J Otolaryngol. 44:103980. DOI: 10.1016/j.amjoto.2023.103980. PMID: 37459740.
37. Aiumtrakul N, Thongprayoon C, Suppadungsuk S, Krisanapan P, Miao J, Qureshi F, Cheungpasitporn W. 2023; Navigating the landscape of personalized medicine: the relevance of ChatGPT, BingChat, and Bard AI in nephrology literature searches. J Pers Med. 13:1457. DOI: 10.3390/jpm13101457. PMID: 37888068. PMCID: PMC10608326.
38. Frosolini A, Franz L, Benedetti S, Vaira LA, de Filippis C, Gennaro P, Marioni G, Gabriele G. 2023; Assessing the accuracy of ChatGPT references in head and neck and ENT disciplines. Eur Arch Otorhinolaryngol. 280:5129–5133. DOI: 10.1007/s00405-023-08205-4. PMID: 37679532.
39. Lechien JR, Briganti G, Vaira LA. 2024; Accuracy of ChatGPT-3.5 and -4 in providing scientific references in otolaryngology-head and neck surgery. Eur Arch Otorhinolaryngol. 281:2159–2165. DOI: 10.1007/s00405-023-08441-8. PMID: 38206389.
40. Wu K, Wu E, Cassasola A, Zhang A, Wei K, Nguyen T, Riantawan S, Riantawan PS, Ho DE, Zou J. 2024. How well do LLMs cite relevant medical references? An evaluation framework and analyses. arXiv:2402.02008 [Preprint]. Available from: https://doi.org/10.48550/arXiv.2402.02008. cited 2024 Mar 15.
41. Piccolo SR, Denny P, Luxton-Reilly A, Payne SH, Ridge PG. 2023; Evaluating a large language model's ability to solve programming exercises from an introductory bioinformatics course. PLoS Comput Biol. 19:e1011511. DOI: 10.1371/journal.pcbi.1011511. PMID: 37769024. PMCID: PMC10564134.
42. Reason T, Rawlinson W, Langham J, Gimblett A, Malcolm B, Klijn S. 2024; Artificial intelligence to automate health economic modelling: a case study to evaluate the potential application of large language models. Pharmacoecon Open. 8:191–203. DOI: 10.1007/s41669-024-00477-8. PMID: 38340276. PMCID: PMC10884386.
43. Wang L, Ge X, Liu L, Hu G. 2024; Code interpreter for bioinformatics: are we there yet? Ann Biomed Eng. 52:754–756. DOI: 10.1007/s10439-023-03324-9. PMID: 37482573.
44. Ahn S. 2024; Data science through natural language with ChatGPT's Code Interpreter. Transl Clin Pharmacol. 32:e8. DOI: 10.12793/tcp.2024.32.e8. PMID: 38974344. PMCID: PMC11224898.
45. Manning BS, Zhu K, Horton JJ. 2024. Automated social science: language models as scientist and subjects. arXiv:2404.11794 [Preprint]. Available from: https://doi.org/10.48550/arXiv.2404.11794. cited 2024 Jun 3.
46. Romera-Paredes B, Barekatain M, Novikov A, Balog M, Kumar MP, Dupont E, Ruiz FJR, Ellenberg JS, Wang P, Fawzi O, Kohli P, Fawzi A. 2024; Mathematical discoveries from program search with large language models. Nature. 625:468–475. DOI: 10.1038/s41586-023-06924-6. PMID: 38096900. PMCID: PMC10794145.
47. Boiko DA, MacKnight R, Kline B, Gomes G. 2023; Autonomous chemical research with large language models. Nature. 624:570–578. DOI: 10.1038/s41586-023-06792-0. PMID: 38123806. PMCID: PMC10733136.
48. Lechien JR, Gorton A, Robertson J, Vaira LA. 2024; Is ChatGPT-4 accurate in proofread a manuscript in otolaryngology-head and neck surgery? Otolaryngol Head Neck Surg. 170:1527–1530. DOI: 10.1002/ohn.526. PMID: 37717252.
49. Checco A, Bracciale L, Loreti P, Pinfield S, Bianchi G. 2021; AI-assisted peer review. Humanit Soc Sci Commun. 8:25. DOI: 10.1057/s41599-020-00703-8.
50. Nashwan AJ, Jaradat JH. 2023; Streamlining systematic reviews: harnessing large language models for quality assessment and risk-of-bias evaluation. Cureus. 15:e43023. DOI: 10.7759/cureus.43023.
51. Dang R, Hanba C. 2024; A large language model's assessment of methodology reporting in head and neck surgery. Am J Otolaryngol. 45:104145. DOI: 10.1016/j.amjoto.2023.104145. PMID: 38103488.
52. Merton RK. 1968; The Matthew effect in science. The reward and communication systems of science are considered. Science. 159:56–63. DOI: 10.1126/science.159.3810.56. PMID: 5634379.
53. Diaz Milian R, Moreno Franco P, Freeman WD, Halamka JD. 2023; Revolution or peril? The controversial role of large language models in medical manuscript writing. Mayo Clin Proc. 98:1444–1448. DOI: 10.1016/j.mayocp.2023.07.009. PMID: 37793723.
54. Liang W, Zhang Y, Cao H, Wang B, Ding D, Yang X, Vodrahalli K, He S, Smith D, Yin Y, McFarland D, Zou J. 2024. Can large language models provide useful feedback on research papers? A large-scale empirical analysis. arXiv:2310.01783 [Preprint]. Available from: https://doi.org/10.48550/arXiv.2310.01783. cited 2024 Mar 19.
55. Saad A, Jenko N, Ariyaratne S, Birch N, Iyengar KP, Davies AM, Vaishya R, Botchu R. 2024; Exploring the potential of ChatGPT in the peer review process: an observational study. Diabetes Metab Syndr. 18:102946. DOI: 10.1016/j.dsx.2024.102946. PMID: 38330745.
56. Hosseini M, Horbach SPJM. 2023; Fighting reviewer fatigue or amplifying bias? Considerations and recommendations for use of ChatGPT and other large language models in scholarly peer review. Res Integr Peer Rev. 8:4. Erratum. DOI: 10.1186/s41073-023-00133-5. PMID: 37198671. PMCID: PMC10191680.
57. Kaplan DM, Palitsky R, Arconada Alvarez SJ, Pozzo NS, Greenleaf MN, Atkinson CA, Lam WA. 2024; What's in a name? Experimental evidence of gender bias in recommendation letters generated by ChatGPT. J Med Internet Res. 26:e51837. DOI: 10.2196/51837. PMID: 38441945. PMCID: PMC10951834.
58. Navigli R, Conia S, Ross B. 2023; Biases in large language models: origins, inventory, and discussion. ACM J Data Inf Qual. 15:10. DOI: 10.1145/3597307.
59. Rawashdeh B, Kim J, AlRyalat SA, Prasad R, Cooper M. 2023; ChatGPT and artificial intelligence in transplantation research: is it always correct? Cureus. 15:e42150. DOI: 10.7759/cureus.42150.
60. Lukac S, Dayan D, Fink V, Leinert E, Hartkopf A, Veselinovic K, Janni W, Rack B, Pfister K, Heitmeir B, Ebner F. 2023; Evaluating ChatGPT as an adjunct for the multidisciplinary tumor board decision-making in primary breast cancer cases. Arch Gynecol Obstet. 308:1831–1844. DOI: 10.1007/s00404-023-07130-5. PMID: 37458761. PMCID: PMC10579162.
61. Jin Q, Chen F, Zhou Y, Xu Z, Cheung JM, Chen R, Summers RM, Rousseau JF, Ni P, Landsman MJ, Baxter SL, Al'Aref SJ, Li Y, Chen A, Brejt JA, Chiang MF, Peng Y, Lu Z. 2024. Hidden flaws behind expert-level accuracy of GPT-4 vision in medicine. arXiv:2401.08396 [Preprint]. Available from: https://doi.org/10.48550/arXiv.2401.08396. cited 2024 Mar 19. DOI: 10.1038/s41746-024-01185-7. PMID: 39043988. PMCID: PMC11266508.
62. Kumar H, Rothschild DM, Goldstein DG, Hofman JM. 2023. Math education with large language models: peril or promise? SSRN [Preprint]. Available from: https://ssrn.com/abstract=4641653. cited 2024 Mar 19. DOI: 10.2139/ssrn.4641653.
63. Dell'Acqua F. 2022. Falling asleep at the wheel: human/AI collaboration in a field experiment on HR recruiters. Available from: https://static1.squarespace.com/static/604b23e38c22a96e9c78879e/t/62d5d9448d061f7327e8a7e7/1658181956291/Falling+Asleep+at+the+Wheel+-+Fabrizio+DellAcqua.pdf. cited 2024 Mar 19.
64. Ganjavi C, Eppler MB, Pekcan A, Biedermann B, Abreu A, Collins GS, Gill IS, Cacciamani GE. 2024; Publishers' and journals' instructions to authors on use of generative artificial intelligence in academic and scientific publishing: bibliometric analysis. BMJ. 384:e077192. DOI: 10.1136/bmj-2023-077192. PMID: 38296328. PMCID: PMC10828852.
65. Ballester PL. 2023; Open science and software assistance: commentary on "artificial intelligence can generate fraudulent but authentic-looking scientific medical articles: Pandora's box has been opened". J Med Internet Res. 25:e49323. DOI: 10.2196/49323. PMID: 37256656. PMCID: PMC10267777.
Fig. 1
Large language models (LLMs) can be used in various steps of research and writing.
A detailed tutorial of how to utilize large language models during each process is provided as a supplementary material.
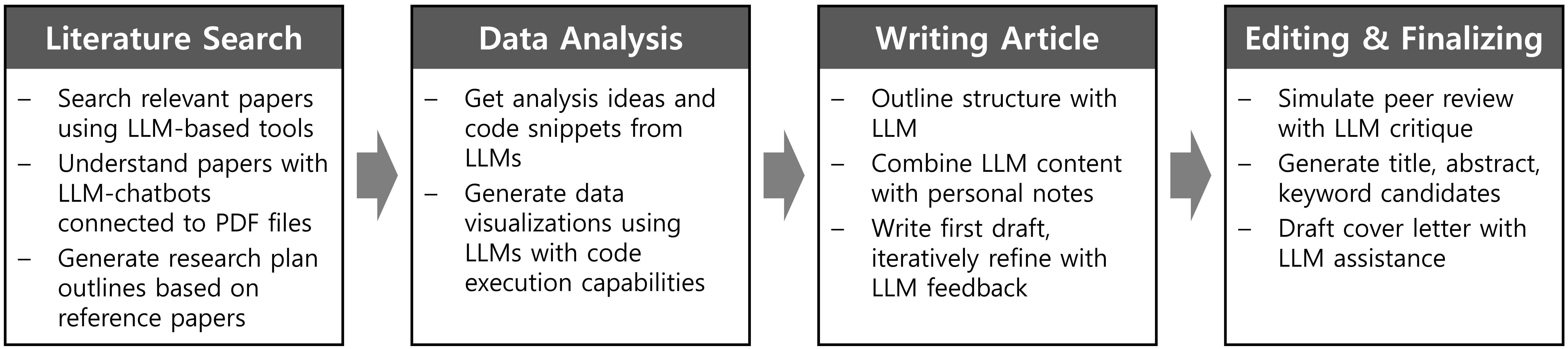
Table 1
Applications of large language models (LLMs) in research and writing
Literature search & research design |
Writing assistance & quality assessment |
Citation & reference generation |
Code generation & data analysis |
---|---|---|---|
- Aid systematic reviews [21] - Create research protocols [22] - Perform bibliometric analysis [23] |
- Generate abstracts with minor errors [24,25] - Artificial intelligence-generated abstracts raise ethical concerns [19,26] - LLM writing quality varies [27-31] - Facilitate non-native English writing [33] - Fine-tuning LLMs for personalized assistance [35] |
- LLM reference accuracy varies (10%–87%) [36-39] - Retrieval-augmented generation crucial for reliability [40] |
- Produce code for data analysis [41] - Health economic modeling [42] - Data analysis using natural language interactions [43,44] |
Table 2
Applications of large language models (LLMs) in peer review and publication
Manuscript screening & quality assessment |
Generating review comments & feedback |
Potential biases & limitations |
Editorial office applications |
---|---|---|---|
- Assist in proofreading and error detection [48,49] - Assess quality and bias in systematic reviews [50] - Develop methodology grading systems [51] - Benefit underprivileged researchers [52] |
- Streamline peer review [53] - LLM comments overlap with human [54] - Tend to provide overly positive reviews [55] - May reduce reviewer overload [56] |
- Demographic biases [57,58] - Overreliance may reduce diversity [54] - Lack deep domain knowledge [59,60] - Human oversight remains essential [54] |
- Prescreen manuscripts - Convert into easily understandable language and multilingual translation - Consider data privacy |