Abstract
Diagnosing the current health status and disease burden in a population is crucial for public health interventions. The ability to compare the burden of different diseases through a single measure, such as disability-adjusted life years has become feasible and continues to be produced and updated through the Global Burden of Diseases (GBD) study. However, the disease burden values of the GBD study do not accurately reflect the unique situation in a specific country with various circumstances. In response, the Korean National Burden of Disease (KNBD) study was conducted to estimate the disease burden in Koreans by considering Korea’s cultural context and utilizing the available data sources at the national level. Both studies identified non-communicable diseases, such as diabetes mellitus (DM), as the primary cause of disease burden among Koreans. However, the extent of public health interventions currently being conducted by the central and local governments does not align with the severity of the disease burden. This review suggests that despite the high burden of DM in South Korea, the current policies may not fully address its impact, underscoring the need for expanded chronic disease management programs and a shift towards prevention-focused healthcare paradigms.
• DM is one of the leading causes of the global disease burden.
• Global and Korean National burden of disease studies share common findings.
• Given the burden of DM in Korea, national interventions seem insufficient.
• Considering its burden, increased societal interest and a budget for DM are needed.
Owing to resource constraints, measuring the scale and impact of various diseases within a population is essential for efficient public health management [1]. Metrics capturing the burden of each disease serve as a crucial data source, informing prioritization of health interventions. Moreover, this endeavor is a fundamental element for global health promotion within the international community [2].
Until the early 1990s, traditional epidemiological indicators, such as mortality, incidence, and prevalence, were utilized to estimate the impact of diseases [3]. However, comparing the burden of different diseases solely using these indicators proved challenging owing to their differing effects. Some diseases have low mortality but high prevalence rates, whereas others may exhibit high mortality but low prevalence, depending on the degree of each feature across diseases. This makes it extremely challenging to conduct a comprehensive comparison of the burden induced by each disease. Furthermore previous studies often focused on single cause rather than multiple, raising concerns about over or underestimation [4]. These challenges and limitations highlight the need for a more comprehensive approach to measuring burden of disease.
The Global Burden of Diseases, Injuries, and Risk Factors(GBD) study, initiated by Chris Murray from Harvard University and Alan Lopez from the World Health Organization (WHO), enabled a comprehensive comparison of disease burdens, aiding evidence-based policymaking [5]. The first GBD study was conducted in 1991, with its findings appearing in the World Development Report of 1993 [6]. Subsequently, the GBD study has undergone some advancements and was published in The Lancet in 1997 [7-10].
The disability-adjusted life year (DALY) concept introduced in the GBD study combines the number of remaining years of life expectancy at the time of death (years of life lost [YLL]) and the years lived in a state of less than full-health, weighted by illness severity (years lived with disability [YLD]) [11]. Initially, the DALY concept encountered challenges and criticisms, particularly regarding methodology, discount rates, and age weighting [12-15], leading to methodological changes over time. Additionally, several research groups have attempted to measure their own burden of disease in individual countries, such as the Korean National Burden of Disease (KNBD) [16-18].
The feasibility of the KNBD study is deeply rooted in unique Korean circumstances. South Korea, with its universal health coverage and fee-for-service payment system, possesses extensive big data that captures the overall patterns of healthcare utilization among Koreans. Unlike GBD study which mainly depend on given estimation by other studies, these foundations allow KNBD study to measure the distribution and characteristics of specific diseases based on total Korean population big data, enabling the initiation of a tailored to Korea’s context.
This review examines and compares the developmental processes and methodologies of GBD and KNBD study using various literature sources. Recent GBD and KNBD research has identified diabetes mellitus (DM) as one of the leading causes of disease burden for Koreans. Since DM constitutes a major portion of burden of disease in South Korea, this study aimed to understand the burden of DM in the Korean population and explore its current policy situation in South Korea by comparing various research findings.
The DALY, defined as the sum of YLL and YLD, represents the total number of years lost due to premature mortality and the number of years lived with a disability or health impairment. Thus, one DALY equates to one lost year of a healthy life (Fig. 1) [19]. For instance, if an individual’s expected lifespan is 80 years, but passes away at 75 years, the YLL due to the premature death is 5. Additionally, if this individual had lost 10 years of healthy life due to various diseases, such as DM and retinopathy, prior to death, the YLD would be 10, making the total DALY 15. A higher DALY indicates a larger deviation from the ideal state of health and signifies a greater disease burden.
Notably, construction of DALY as a single measure enables comparisons between different diseases. As mentioned previously, diseases possess distinct characteristics, making it challenging to directly compare and determine the diseases that impose a greater burden. However, by converting various disease burden into a unified measure through the DALY, a comprehensive comparison between diseases becomes feasible.
The YLL was calculated as the years lost due to premature mortality by subtracting the age at death from the expected lifespan in each age group and then multiplying it by the number of deaths. As mentioned earlier, the DALY has been created to compare diseases; thus, it is essential to determine the DALY for each disease. Similarly, the YLL must be calculated for each disease. As part of this process, to accurately compute the YLL, it is necessary to simultaneously consider the cause of death. Using the data on cause-specific mortality, the disease burden represented by the YLL for a particular condition can be calculated in a more precise manner.
However, some causes listed in the available mortality data cannot be defined as the underlying causes of death [20]. For example, senility cannot be considered as a cause of death [21], and lower back pain is a sign and symptom, not an underlying cause of death. In other words, the more deaths that cannot be attributed to a direct cause of death, the more difficult it becomes to accurately calculate the YLL. To derive a more precise YLL, such deaths are grouped into ‘garbage codes,’ and deaths attributed to these codes are redistributed to other underlying causes of death at a certain ratio [22-24].
The method for calculating YLD is more complex than that for YLL. There are two methods for measuring the YLD: one is based on incidence and the other on prevalence [25]. The choice between these methods affects the calculation of YLD. The incidence-based approach multiplies the number of new cases of a specific disease by the disability weight and duration, similar to the method described earlier for YLL. Through this process, the incidence-based YLD adopts a forward-looking approach that considers the future disease burden. Conversely, the prevalence-based YLD calculation multiplies the prevalence of the sequelae of a specific disease by its corresponding disability weight (Table 1).
Age weighting and discounting were considered in the calculation of incidence-based DALY [11]. These two elements were established to reflect some characteristics of the future. Discounting was added to account for the uncertainty of the future and time preferences. Age weighting was set up considering the varying values that individuals place on life years at different ages and the productivity of certain age groups [26]. The discount rate initially set in early GBD studies was 3%. This set discount rate serves to reduce the impact of premature death on YLL, as premature death occurs earlier and the discounting effect becomes more significant. It also plays a role in reducing the YLD to a certain extent [26].
However, the prevalence-based YLD usually does not apply age weighting or discounting [27]. This method calculates the disease burden at a specific point in time, which differs from the forward-looking incidence-based approach. As it calculates the disease burden for each condition at a specific point in time, there is no need to consider the future disease burden; thus, age weighting and discounting are not applied. This led to the conclusion that the prevalence-based approach was slightly more descriptive than the incidence-based approach [26].
The burden of disease was calculated using the methodologies mentioned earlier according to the disease, sex, region, and age group. In the GBD study, the diseases were divided into three groups: communicable, maternal, perinatal, and nutritional conditions; non-communicable diseases (NCDs); and injuries. These three groups represent level 1, the broadest classification, which is further subdivided into more specific categories down to level 4. For example, under level 1 NCDs, there is a level 2 neoplasm, and under that, breast cancer is at level 3. The diseases classified in this manner are mutually exclusive and non-overlapping, allowing the measurement of disease burden according to the disease classification system [28].
Among the factors mentioned earlier, a pivotal aspect of the burden of disease research is the disability weight. It harmonizes morbidity and mortality by uniformly scaling YLL and YLD. The disability weight is valued between 0 and 1, where 0 represents a state of perfect health and 1 represents a state of death [29].
In the initial stages of the GBD study, disability weights were determined by 10 public health experts using the person tradeoff (PTO) method and visual analog scales. In the 2010 GBD study, disability weights were calculated using an improved version of the PTO, known as the population health equivalence and paired comparison methods [30]. The data were collected through online surveys and household questionnaires from approximately 30,000 individuals across five countries, including the United States, Tanzania, and Indonesia. Research from the Netherlands, Sweden, Hungary, and Italy was incorporated to determine disability weights in the 2013 GBD study [31].
However, some argue that the perceived burden of disease varies across societies [32,33], prompting research to calculate unique disability weights for individual countries beyond those established by the GBD study. Since 2000, research has been conducted in South Korea to calculate the disease burden tailored to their own specific context [29,34-36].
In the first GBD study, conducted in the 1990s, measurements were made for eight regions and 107 diseases and injuries, along with 10 risk factors and 483 sequelae. Initially, the approach for measuring the DALY utilized an incidence-based approach, applying both age weighting and discounting. As a result, the disease burden due to premature mortality accounted for 66% of the total, whereas YLDs owing to morbidity accounted for 34% [6]. Following the publication of these findings, various debates have emerged surrounding the DALY, primarily due to the issues of discounting and age weighting [14,15]. These two components of DALY sparked various philosophical and economic discussions. Additionally, debates continue regarding the age standard for calculating premature mortality in the YLL and the uncertainty in the data [12].
Subsequently, Chris Murray continued the GBD study in collaboration with the WHO [37-39], and the GBD study for 2000–2004 achieved various advancements in the methodology and data compared to the initial 1990 GBD study. During this period, the number of measured diseases and injuries increased to 136, and the number of risk factors increased to 25. The geographical units for measuring the disease burden specified from 8 to 211. In terms of the software, the DisMod program used to estimate the duration of disease incidence was revised to develop DisMod-II [40], enabling more refined epidemiological indicator outcomes.
Over time, Chris Murray left the WHO and, with the support of the Bill and Melinda Gates Foundation, established the Institute of Health Metrics and Evaluation (IHME) in 2007 [41]. During this period, the GBD study underwent significant changes, with the number of measured diseases and injuries increasing to 291, risk factors increasing to 67, and sequelae to 1,160 [4]. A new program for calculating the epidemiological indicators, DisMod-MR, was developed [27] which operates based on Python. From this period onwards, the burden of disease was calculated based on the available data through statistical models, and the method for calculating the DALY transitioned from an incidence-based to a prevalence-based approach. Age weighting and discounting were excluded from the GBD calculations. Through these steps, the GBD study has become more refined in calculating the burden of disease across a range of diseases, geographical units, and age groups, and its methodology has seen considerable advancements.
For over two decades, the GBD study have advanced, covering over 200 countries and describing more than 80 risk factors [24,28]. Moreover, the GBD study has notably enhanced data transparency, facilitating priority setting within population health issues [4]. However, when analyzing figures from the GBD study or the IHME, some discrepancies emerge in national level or certain DALY values, prompting questions.
In the GBD study, which covered population scenarios for various countries, the total fertility rate in South Korea was reported to be 1.24 in 2017 and 1.22 in 2019 [42]. However, according to Statistics Korea, the total fertility rate for South Korea was 1.05 in 2017 and 0.92 in 2019 [43]. These values are also used by the World Bank and Organization for Economic Cooperation and Development [44,45]. This discrepancy indicates that some population data used to calculate health-adjusted life expectancy (HALE) [46] do not align with other statistical figures. Such discrepancies raise doubts regarding certain figures.
Furthermore, in 2017, out of 2,107,749 DALYs due to neoplasms in the GBD study, YLLs accounted for 2,012,472 and YLDs accounted for 95,277. In 2019, out of 2,267,782 DALYs, YLLs were 2,164,284, and YLDs were 103,498 [47]. This proportion shows that in 2017, YLLs constituted 95.48% of DALYs, and in 2019, 95.44%. However, the cancer survival rate in South Korea has been continuously increasing [48,49]. From 2013 to 2017, the 5-year relative survival rate of patients with cancer was 70.4% (male 63.5%, female 77.5%), and from 2015 to 2019, the survival rate was 70.7% (male 64.5%, female 77.3%) [48,49]. Given the increasing trend in cancer survival rates, the finding that YLLs constitute 95% of the burden of neoplasms is difficult to accept.
Finally, there is a need to consider the unique circumstances specific to South Korea when measuring the burden of disease. Although the GBD study currently covers South Korea, it is not calculated based on socioeconomic strata, which makes hard to consider the aspects of health equity. South Korea has experienced rapid economic development centered around specific cities [50,51], leading to significant regional disparities [52,53]. However, it is not easy to discern how the patterns of disease burden differ by region and income class based solely on the results of the GBD study. In addition to the limitations mentioned in the GBD study, if the purpose is not international comparison, applying disability weights that reflect the cultural context of Koreans is deemed more suitable for studying the national burden of disease in Korea, given that the disability weight can vary according to the cultural context of each country. Therefore, the KNBD was initiated.
Various studies addressing the overall disease burden among Koreans began with studies that calculated the disease burden in Koreans in early 2000 [18,54,55]. This study used data from Statistics Korea, the National Health Insurance Service (NHIS), and disability weights of South Korea to analyze 121 causes [56]. This study employed an incidence-based approach to calculate the YLL and YLD, applied a 3% discounting rate, and implemented age weighting. Although adopting the main framework of the GBD study, such as disease classification, the significance of the study lies in its execution of a Korea-specific burden of disease study by substituting key indicators, such as data sources and disability weights, with national data [57]. Subsequent research was published in 2013, measuring the burden of disease in Korea at 2007; this study also used the WHO’s definitions of diseases and utilized the NHIS claims data and data from Statistics Korea to measure the burden of 11 chronic diseases [58].
Subsequent studies addressing the disease burden among Koreans were conducted by individual researchers [59]. In 2013, with the support of the Ministry of Health and Welfare of South Korea, a research and development project was conducted over approximately 5 years [60]. Through this research, a unique garbage code algorithm and disability weights for Korea were developed, the regional HALE was calculated, and the DALY for 313 diseases was measured. The results of this study were published as a supplement to the Journal of Korean Medical Science [29,56,61-71]. The significance of measuring the disease burden in South Korea in 2012 lies in the development of methodologies and use of data suited to the Korean context. These studies did not primarily aim for international comparison, but to measure a disease burden more appropriate for Korea, considering the cultural context unique to Korea and using the data representative of Koreans.
Subsequent research was conducted to measure the disease burden and HALE more precisely among Koreans. The primary goal of this study was to measure the level of health considering sociodemographic factors and specific geographical unit. In other words, it achieved the expansion and concretization of the range of indicators related to disease burden and advancements in the methodology of measuring the disease burden [72]. Through this research, it was possible to understand the burden of disease according to various sociodemographic characteristics of South Korea, identify disparities of the disease burden by region and income level. And discuss health equity from the perspective of burden of disease. As a result, this research has significant policy implications, as it has been used as an indicator for national health promotion development projects, and the HALE and DALY calculated by region can serve as the basic data for community health promotion projects.
Even after the aforementioned studies, the KNBD study kept evolving. Using DALY and HALE, the disease burden among Koreans was calculated by each region and social stratum, and the disability weights for Koreans were continually updated alongside methodological advancements [34].
Similar to the GBD study, the KNBD study has also evolved over time. The data sources used in the most recent KNBD study were as follows: for all diseases except injuries, the NHIS claims data were used to identify both the prevalence and incidence. Each disease has different criteria for inpatient and outpatient services and exceeding these criteria qualifies as a prevalent case. Subsequently, incident cases among the prevalent cases were defined by applying different washout periods (1 to 5 years) for each disease. For injuries, data from the Korea National Hospital Discharge In-Depth Injury Survey of the Korea Disease Control and Prevention Agency were used. Also the cause-of-death statistics from Statistics Korea were used to determine the number of deaths by the disease [73,74].
The methodology for calculating the DALY and HALE among Koreans are an incidence-based approach [73-75]. The number of incident cases for calculating the YLD for specific diseases was determined using the NHIS claims data, as mentioned earlier, and the disability weights were determined using figures developed in South Korea. Discounting and age weighting were also applied at a discounting rate of 3%. Adopting an incidence-based approach requires the average age of onset and duration of illness for each disease, which are calculated by using the DisMod-II developed by the WHO. However, for communicable diseases, the duration of illness is directly calculated using the NHIS claims data. These generated figures were used to calculate the YLD for each disease. The YLL was calculated using cause-of-death statistics and by applying discounting and age weighting. The Korea-specific garbage code developed in the KNBD study was used in this process [63].
The calculated YLL and YLD were produced at the incomeand regional-level units [73]. NHIS imposes insurance premiums based on income and asset levels, hence the premium variable observable in claim data can serve as a proxy indicator for income. For the YLD, to ascertain the income level, the insurance premiums recorded in the NHIS are utilized to equalize the household income, thereby categorizing individual income levels. Medical Aid beneficiaries who did not pay insurance premiums were assumed to earn no income [76]. The population, combining the NHIS and Medical Aid beneficiaries, was divided into quintiles to measure the YLD. However, in case of Korea’s healthcare system, the government covers most medical expenses for low-income individuals under Medical Aid, which may allow them unrestricted access to medical services. This could potentially lead to an overestimation of disease burden if claims data are used to calculate burden of diseases. Nevertheless, the KNBD study mitigates this concern by calculating disease prevalence-based on claims data and establishing different washout periods for each disease to determine incident cases, thereby minimizing the risk of overestimation due to unrestricted medical access. Due to the data limitations, the income levels cannot be directly determined for YLL; therefore, the distribution of deaths by income level for the total population was identified through the NHIS claims data. Subsequently, YLL is redistributed according to the income level to calculate the YLL by income level.
The HALE was derived based on YLD, utilizing Sullivan’s method for its calculations [74]. Sullivan’s method comprises three major steps: the calculation of life expectancy, YLD, and HALE [77]. The NHIS claims data were used to ensure data consistency in calculating the life expectancy. These data were used to determine the probability of death, which formed the basis for calculating the life expectancy. Once the life expectancy is calculated, the YLD using the previously mentioned incidence-based approach is needed subsequently. Finally, with both the life expectancy and YLD prepared, these components were used to calculate the HALE.
Due to the changes in methodology and data sources, it is difficult to directly compare the values from past KNBD studies with those from currently conducted KNBD studies. However, reviewing the burden of disease among Koreans based on the results of recent research, the disease that accounted for the largest portion of the disease burden in Koreans in 2020 was DM (Fig. 2) [78]. Among the top 20 diseases, NCDs constituted the majority, with injuries comprising the remainder. Although the composition of the top 20 diseases did not significantly change over time, there were some changes within the NCDs, such as rank shifts [73].
A closer examination of the burden of DM reveals the following: as of 2018, per 100,000 population, the burden of DM for men was 2,451 DALYs, and for women, it was 1,644 DALYs. Even in 2020, when overall DALYs decreased due to coronavirus disease 2019 (COVID-19), DM recorded 3,054 DALYs for men and 2,064 DALYs for women, per 100,000 population. In other words, despite an overall decrease in disease burden, the burden of DM has increased. As the disease burden increased, so did the proportion of DM in the total disease burden by sex. In 2018, DM accounted for 9.3% and 6.8% of the disease burden for men and women, respectively, but in 2020, these proportions increased to 11.8% for men and 8.3% for women. These recent results from the KNBD study indicate that the proportion of DM in the disease burden among Koreans is continuously increasing.
DM has consistently caused a high disease burden from the past to the present, and compared to the past, the disease burden of low back pain has significantly increased. The increase in the burden of these chronic diseases followed a pattern similar to that observed in other developed countries. The increase in the disease burden of DM and low back pain has been attributed to aging, westernized dietary habits, and sedentary lifestyles [79,80]. Furthermore, the disease burden of Alzheimer’s disease has increased compared to the past, which is understood as a change in the pattern of disease burden due to rapid aging [81]. The increase in the disease burden of depressive disorders aligns with the analysis results of the Health Insurance Review and Assessment Service, showing a continuous upward trend in the number of patients with depression, especially a shift in the age structure where, if individuals in their 50s and 60s were predominantly affected in 2017, the trend has now shifted predominantly towards those in their 20s [82].
When examining the disparities in the burden of disease by income level and region, the group with the lowest income level experienced a higher burden of disease than the other groups. A regional analysis of the disease burden revealed that Seoul, new towns, and some districts within other metropolitan cities have a significantly lower disease burden than other areas [73]. This quantitatively indicates that the current health disparities among Koreans vary by income level and region, highlighting the necessity of efforts to reduce these differences to improve the health equity.
The KNBD study has been consistently compiling and reporting the disease burden of Koreans from the past to the present into a single, comparable figure. This not only provides a comprehensive view of changes in the population structure, lifestyle habits, and disease patterns among Koreans, but also serves as the basis and criterion for setting the priorities for public health interventions [83]. In particular, this indicator allows the identification of diseases whose importance is underrecognized relative to their disease burden, with mental disorders being a prime example [84]. A high DALY indicates a high disease burden on the population, underscoring the need for public health interventions. However, health policy priorities are sometimes based on historical and political issues [85]. Therefore, based on the results of the KNBD study, it is possible to assess the necessity of public health interventions for diseases that have been excluded from the priorities, and the appropriateness of the current health policies.
As previously mentioned, DM accounts for the largest proportion of disease burden among Koreans. Especially in 2020, due to the impact of COVID-19, the overall DALYs in South Korea decreased compared to the previous year. When comparing the changes in DALYs per 100,000 people for the causes with the highest disease burden, it was observed that the rate of increase in disease burden for some conditions like asthma and chronic lower respiratory disease slowed down or decreased from 2019 to 2020. However, DM, which ranks highest in disease burden, showed a consistent increase without any decrease [78]. Considering the accelerated aging process, the increasing incidence and prevalence of DM at younger ages [86-88], and the rising prevalence of obesity in the total population [89,90], the disease burden of DM is expected to increase. Specifically, as of 2020, it is estimated that 16.7% of Koreans aged 30 and older have DM, and 44.0% have prediabetes [91]. This indicates that there is already a significant population burdened with DM, in addition to a substantial at-risk group likely to develop DM in the future. Consequently, it can be anticipated that DM will continue to constitute a major portion of the disease burden among Koreans. Moreover, DM incurs a significant economic burden, with studies reporting that it causes greater economic strain than overall cancers [92]. Considering these factors, it is reasonable to predict that the future burden of DM could be catastrophic.
However, it is crucial to not overlook the fact that DM leads to various complications [93]. DM causes microvascular complications, including retinopathy [94], neuropathy [95], and macrovascular complications, such as cardiovascular diseases [96]. Particularly, a closer examination of the KNBD study results reveals that ischemic stroke and ischemic heart disease, which are the most prominent complications of DM, rank as the third and fourth leading causes of disease burden among Koreans. This indicates that DM not only represents the largest single contributor to the disease burden in Korea but also significantly contributes as a risk factor for complications that lead to substantial additional disease burden. Beyond these traditional complications, continuous research has been conducted on the emerging complications of DM, such as depression [97] and cognitive impairment [98]. In other words, DM contributes significantly to disease burden among Koreans through various diseases.
To understand the overall status of the disease burden caused by DM and quantitatively represent the benefits obtained through DM management as perceiving DM as a risk factor for other complications, it is necessary to calculate the population-attributable fraction (PAF) of complications caused by DM. The KNBD study calculates the burden of various diseases, including DM, individually, which presents a limitation in accurately determining the extent of the disease burden caused by complications arising from DM. If the PAF for the occurrence of complications due to DM could be known, it would enable the calculation of the disease burden for each diabetic complications, thereby quantitatively representing the overall disease burden on Koreans due to DM. Moreover, by determining the PAF for various diseases caused by DM, it is possible to ascertain how much DM contributes to these complications and comprehensively evaluate the potential reduction in disease burden that can be achieved by addressing DM [99]. Therefore, further research on the PAF of DM and its complications is needed.
The policy situation in South Korea regarding DM is underrecognized compared to the disease burden it imposes. Some regions of South Korea have implemented management projects, such as the “Hypertension and Diabetes Registry Program” [100] and the “Primary Medical Chronic Disease Man agement Pilot Project” [101] to manage DM and hypertension. However, the attention and budget allocated to DM are insufficient, given the disease burden it poses to Koreans. According to the 2024 budget report by the Ministry of Health and Welfare, the major investment projects include emergency medical, pediatric medical, and mental healthcare services [102]. As previously mentioned, priorities for public health interventions are determined by various factors. Nevertheless, proactive interventions at the national and local levels are necessary for DM, which imposes the highest disease burden on Koreans. Previous studies reported the positive effects of ongoing chronic disease management pilot projects [103]. Considering the burden of DM imposed on Koreans, expanding these projects and programs as well as shifting towards a preventive care paradigm through primary care strengthening seems imperative [104]. To achieve this, it is necessary to evaluate the cost-effectiveness of projects and programs to demonstrate the benefits of prevention to policymakers. Additionally, providing financial incentives and incorporating a comprehensive range of participants in prevention programs to maximize their effects are crucial [105].
Attempts to measure the disease burden of a population with a single measure have made significant progress compared to the past. Various countries are using calculation methodology for this measure to produce disease burden figures that suit their circumstances. South Korea is doing the same, independently calculating the disease burden of Koreans through various data sources generated within Korea. As a result, it was found that various NCDs, including DM, account for the majority of the disease burden among Koreans. However, the level of societal interest and budget willing to be invested in these diseases is insufficient compared to the extent of their burden, leading to a discrepancy between the degree of disease burden and the level of intervention. Considering the disease burden of DM, it is deemed necessary to expand the target of currently implemented projects and enhance policy interventions to increase their sustainability.
REFERENCES
1. Eyal N, Hurst SA, Murray CJL, Schroeder SA, Wikler D. Measuring the global burden of disease: philosophical dimensions. New York: Oxford University Press;2020. Chapter 17, The uses of burden-of-disease data for priority setting. p. 293–306.
2. Murray CJ, Salomon JA, Mathers C. A critical examination of summary measures of population health. Bull World Health Organ. 2000; 78:981–94.
3. Snyder JD, Merson MH. The magnitude of the global problem of acute diarrhoeal disease: a review of active surveillance data. Bull World Health Organ. 1982; 60:605–13.
4. Murray CJ, Lopez AD. Measuring global health: motivation and evolution of the Global Burden of Disease Study. Lancet. 2017; 390:1460–4.


5. Murray CJL, Lopez AD. The global burden of disease: a comprehensive assessment of mortality and disability from diseases, injuries, and risk factors in 1990 and projected to 2020. Cambridge: Harvard University Press;1996.
6. World Bank. World development report 1993: investing in health. New York: Oxford University Press;1993.
7. Murray CJ, Lopez AD. Alternative projections of mortality and disability by cause 1990-2020: Global Burden of Disease Study. Lancet. 1997; 349:1498–504.
8. Murray CJ, Lopez AD. Global mortality, disability, and the contribution of risk factors: Global Burden of Disease Study. Lancet. 1997; 349:1436–42.


9. Murray CJ, Lopez AD. Mortality by cause for eight regions of the world: Global Burden of Disease Study. Lancet. 1997; 349:1269–76.


10. Murray CJ, Lopez AD. Regional patterns of disability-free life expectancy and disability-adjusted life expectancy: Global Burden of Disease Study. Lancet. 1997; 349:1347–52.


11. Murray CJ. Quantifying the burden of disease: the technical basis for disability-adjusted life years. Bull World Health Organ. 1994; 72:429–45.
12. Cooper RS, Osotimehin B, Kaufman JS, Forrester T. Disease burden in sub-Saharan Africa: what should we conclude in the absence of data? Lancet. 1998; 351:208–10.
13. Nord E. Disability weights in the Global Burden of Disease 2010: unclear meaning and overstatement of international agreement. Health Policy. 2013; 111:99–104.


14. Arnesen T, Nord E. The value of DALY life: problems with ethics and validity of disability adjusted life years. BMJ. 1999; 319:1423–5.


15. Anand S, Hanson K. Disability-adjusted life years: a critical review. J Health Econ. 1997; 16:685–702.


16. Begg SJ, Vos T, Barker B, Stanley L, Lopez AD. Burden of disease and injury in Australia in the new millennium: measuring health loss from diseases, injuries and risk factors. Med J Aust. 2008; 188:36–40.


17. Naghavi M, Abolhassani F, Pourmalek F, Lakeh M, Jafari N, Vaseghi S, et al. The burden of disease and injury in Iran 2003. Popul Health Metr. 2009; 7:9.
18. Yoon SJ, Bae SC, Lee SI, Chang H, Jo HS, Sung JH, et al. Measuring the burden of disease in Korea. J Korean Med Sci. 2007; 22:518–23.


19. Mathers CD, Ezzati M, Lopez AD. Measuring the burden of neglected tropical diseases: the global burden of disease framework. PLoS Negl Trop Dis. 2007; 1:e114.


20. Iburg KM, Mikkelsen L, Adair T, Lopez AD. Are cause of death data fit for purpose?: evidence from 20 countries at different levels of socio-economic development. PLoS One. 2020; 15:e0237539.


21. Ellingsen CL, Alfsen GC, Ebbing M, Pedersen AG, Sulo G, Vollset SE, et al. Garbage codes in the Norwegian Cause of Death Registry 1996-2019. BMC Public Health. 2022; 22:1301.


22. GBD 2017 Causes of Death Collaborators. Global, regional, and national age-sex-specific mortality for 282 causes of death in 195 countries and territories, 1980-2017: a systematic analysis for the Global Burden of Disease Study 2017. Lancet. 2018; 392:1736–88.
23. GBD 2017 Disease and Injury Incidence and Prevalence Collaborators. Global, regional, and national incidence, prevalence, and years lived with disability for 354 diseases and injuries for 195 countries and territories, 1990-2017: a systematic analysis for the Global Burden of Disease Study 2017. Lancet. 2018; 392:1789–858.
24. GBD 2019 Risk Factors Collaborators. Global burden of 87 risk factors in 204 countries and territories, 1990-2019: a systematic analysis for the Global Burden of Disease Study 2019. Lancet. 2020; 396:1223–49.
25. Kim YE, Jung YS, Ock M, Yoon SJ. DALY estimation approaches: understanding and using the incidence-based approach and the prevalence-based approach. J Prev Med Public Health. 2022; 55:10–8.
26. Solberg CT, Sorheim P, Muller KE, Gamlund E, Norheim OF, Barra M. The devils in the DALY: prevailing evaluative assumptions. Public Health Ethics. 2020; 13:259–74.


27. Murray CJ, Ezzati M, Flaxman AD, Lim S, Lozano R, Michaud C, et al. GBD 2010: design, definitions, and metrics. Lancet. 2012; 380:2063–6.


28. GBD 2019 Diseases and Injuries Collaborators. Global burden of 369 diseases and injuries in 204 countries and territories, 1990-2019: a systematic analysis for the Global Burden of Disease Study 2019. Lancet. 2020; 396:1204–22.
29. Ock M, Lee JY, Oh IH, Park H, Yoon SJ, Jo MW. Disability weights measurement for 228 causes of disease in the Korean Burden of Disease Study 2012. J Korean Med Sci. 2016; 31(Suppl 2):S129–38.


30. Salomon JA, Vos T, Hogan DR, Gagnon M, Naghavi M, Mokdad A, et al. Common values in assessing health outcomes from disease and injury: disability weights measurement study for the Global Burden of Disease Study 2010. Lancet. 2012; 380:2129–43.
31. Salomon JA, Haagsma JA, Davis A, de Noordhout CM, Polinder S, Havelaar AH, et al. Disability weights for the Global Burden of Disease 2013 study. Lancet Glob Health. 2015; 3:e712–23.
32. He Q, Zhou M, Yin P, Jiang B, Qi J, Liu Y, et al. Can global or national disability weights represent provincial level? BMC Public Health. 2023; 23:461.


33. Ustun TB, Saxena S, Rehm J, Bickenbach J. Are disability weights universal? WHO/NIH Joint Project CAR Study Group. Lancet. 1999; 354:1306.
34. Im D, Mahmudah NA, Yoon SJ, Kim YE, Lee DH, Kim YH, et al. Updating Korean disability weights for causes of disease: adopting an add-on study method. J Prev Med Public Health. 2023; 56:291–302.


35. Kim YE, Jo MW, Park H, Oh IH, Yoon SJ, Pyo J, et al. Updating disability weights for measurement of healthy life expectancy and disability-adjusted life year in Korea. J Korean Med Sci. 2020; 35:e219.


36. Ock M, Ko S, Lee HJ, Jo MW. Review of issues for disability weight studies. Health Policy Manag. 2016; 26:352–8.
37. Mathers CD. History of global burden of disease assessment at the World Health Organization. Arch Public Health. 2020; 78:77.


38. World Health Organization. World health report. Health systems: improving performance. Geneva: WHO;2000. p. 216.
39. World Health Organization. World health report: reducing risks, promoting healthy life. Geneva: WHO;2002. p. 248.
40. Barendregt JJ, Van Oortmarssen GJ, Vos T, Murray CJ. A generic model for the assessment of disease epidemiology: the computational basis of DisMod II. Popul Health Metr. 2003; 1:4.


41. Murray CJ, Lopez AD, Black R, Mathers CD, Shibuya K, Ezzati M, et al. Global burden of disease 2005: call for collaborators. Lancet. 2007; 370:109–10.


42. Vollset SE, Goren E, Yuan CW, Cao J, Smith AE, Hsiao T, et al. Fertility, mortality, migration, and population scenarios for 195 countries and territories from 2017 to 2100: a forecasting analysis for the Global Burden of Disease Study. Lancet. 2020; 396:1285–306.


43. Kim E, Yun J, Kim CY, Chung SH, Son SH, Choi YS, et al. Estimates of future population, birth rate, and number of total births and children in South Korea: comparison with past statistics. Perinatology. 2021; 32:166–76.
44. Organisation for Economic Co-operation and Development. Fertility rates. Available from: https://data.oecd.org/pop/fertility-rates.htm (cited 2024 Jun 19).
45. World Bank. Fertility rate, total (births per woman). Available from: https://data.worldbank.org/indicator/SP.DYN.TFRT.IN (cited 2024 Jun 19).
46. GBD 2019 Demographics Collaborators. Global age-sex-specific fertility, mortality, healthy life expectancy (HALE), and population estimates in 204 countries and territories, 1950-2019: a comprehensive demographic analysis for the Global Burden of Disease Study 2019. Lancet. 2020; 396:1160–203.
47. Institute of Health Metrics and Evaluation. GBD results. Available from: https://vizhub.healthdata.org/gbd-results (cited 2024 Jun 19).
48. Hong S, Won YJ, Park YR, Jung KW, Kong HJ, Lee ES, et al. Cancer statistics in Korea: incidence, mortality, survival, and prevalence in 2017. Cancer Res Treat. 2020; 52:335–50.


49. Kang MJ, Won YJ, Lee JJ, Jung KW, Kim HJ, Kong HJ, et al. Cancer statistics in Korea: incidence, mortality, survival, and prevalence in 2019. Cancer Res Treat. 2022; 54:330–44.


50. Leipziger D. Korea’s economy volume 30. Washington D.C: Korea Economic Institute of America;2016. Chapter, Lessons from the economic development experience of South Korea. p. 1–5.
51. GBD 2019 South Korea BoD Collaborators. Population health outcomes in South Korea 1990-2019, and projections up to 2040: a systematic analysis for the Global Burden of Disease Study 2019. Lancet Public Health. 2023; 8:e639–50.
52. Go DS, Kim YE, Radnaabaatar M, Jung Y, Jung J, Yoon SJ. Regional differences in years of life lost in Korea from 1997 to 2015. J Korean Med Sci. 2018; 34(Suppl 1):e91.


53. Jo MW, Seo W, Lim SY, Ock M. The trends in health life expectancy in Korea according to age, gender, education level, and subregion: using quality-adjusted life expectancy method. J Korean Med Sci. 2018; 34(Suppl 1):e88.
54. Yoon SJ, Bae SC. Current scope and perspective of burden of disease study based on health related quality of life. J Korean Med Assoc. 2004; 47:600–2.


55. Bae S, Lee S, Yoon S, Yim J, Hong D, Do Y, et al. A study on improvement of health-related quality of life by measurement of disease burden in Korea. Seoul: Ministry of Health and Welfare;2005.
56. Yoon J, Yoon SJ. Quantifying burden of disease to measure population health in Korea. J Korean Med Sci. 2016; 31(Suppl 2):S101–7.


57. Kim YE, Yoon SJ. Measuring the Korean National Burden of Diseases: international comparison and policy implication. Evid Value Healthc. 2017; 3:138–50.
58. Kim EJ, Yoon SJ, Jo MW, Kim HJ. Measuring the burden of chronic diseases in Korea in 2007. Public Health. 2013; 127:806–13.
59. Lee KS, Park JH. Burden of disease in Korea during 2000-10. J Public Health (Oxf). 2014; 36:225–34.


60. Yoon S, Jo M, Park H, Oh I, Kim B, Kim D, et al. A study on measuring and forecasting the burden of disease in Korea. Seoul: Cardiovascular and Metabolic Diseases Etiology Research Center;2015.
61. Park SY, Kwon I, Oh IH. Burden of disease study and priority setting in Korea: an ethical perspective. J Korean Med Sci. 2016; 31(Suppl 2):S108–13.


62. Lee YH, Yoon SJ, Kim A, Seo H, Ko S. Health performance and challenges in Korea: a review of the global burden of disease study 2013. J Korean Med Sci. 2016; 31(Suppl 2):S114–20.


63. Lee YR, Kim YA, Park SY, Oh CM, Kim YE, Oh IH. Application of a modified garbage code algorithm to estimate cause-specific mortality and years of life lost in Korea. J Korean Med Sci. 2016; 31(Suppl 2):S121–8.
64. Lee JY, Ock M, Kim SH, Go DS, Kim HJ, Jo MW. Health-adjusted life expectancy (HALE) in Korea: 2005-2011. J Korean Med Sci. 2016; 31(Suppl 2):S139–45.


65. Yoon J, Oh IH, Seo H, Kim EJ, Gong YH, Ock M, et al. Disability-adjusted life years for 313 diseases and injuries: the 2012 Korean Burden of Disease Study. J Korean Med Sci. 2016; 31(Suppl 2):S146–57.


66. Yoon J, Seo H, Oh IH, Yoon SJ. The non-communicable disease burden in Korea: findings from the 2012 Korean Burden of Disease Study. J Korean Med Sci. 2016; 31(Suppl 2):S158–67.


67. Gong YH, Yoon SJ, Jo MW, Kim A, Kim YA, Yoon J, et al. The burden of cancer in Korea during 2012: findings from a prevalence-based approach. J Korean Med Sci. 2016; 31(Suppl 2):S168–77.


68. Lee YR, Moon K, Kim YA, Park SY, Oh CM, Lee KS, et al. Disability-adjusted life years for communicable disease in the Korean Burden of Disease Study 2012. J Korean Med Sci. 2016; 31(Suppl 2):S178–83.
69. Kim SH, Lee HJ, Ock M, Go DS, Kim HJ, Lee JY, et al. Disability-adjusted life years for maternal, neonatal, and nutritional disorders in Korea. J Korean Med Sci. 2016; 31(Suppl 2):S184–90.


70. Lim D, Lee WK, Park H. Disability-adjusted life years (DALYs) for mental and substance use disorders in the Korean Burden of Disease Study 2012. J Korean Med Sci. 2016; 31(Suppl 2):S191–9.


71. Lee WK, Lim D, Park H. Disability-adjusted life years (DALYs) for injuries using death certificates and hospital discharge survey by the Korean Burden of Disease Study 2012. J Korean Med Sci. 2016; 31(Suppl 2):S200–7.


72. Park B, Ock M, Jo MW, Lee HA, Lee EK, Park B, et al. Health gap for multimorbidity: comparison of models combining uniconditional health gap. Qual Life Res. 2020; 29:2475–83.


73. Jung YS, Kim YE, Park H, Oh IH, Jo MW, Ock M, et al. Measuring the burden of disease in Korea, 2008-2018. J Prev Med Public Health. 2021; 54:293–300.
74. Jung YS, Kim YE, Ock M, Yoon SJ. Trends in healthy life expectancy (HALE) and disparities by income and region in Korea (2008-2020): analysis of a nationwide claims database. J Korean Med Sci. 2024; 39:e46.
75. Kim YE, Park H, Jo MW, Oh IH, Go DS, Jung J, et al. Trends and patterns of burden of disease and injuries in Korea using disability-adjusted life years. J Korean Med Sci. 2019; 34(Suppl 1):e75.


76. Jung YS, Yoon SJ. Trends and patterns of cancer burdens by region and income level in Korea: a national representative big data analysis. Cancer Res Treat. 2023; 55:408–18.


78. Jung YS, Kim YE, Ock M, Yoon SJ. Measuring the burden of disease in Korea using disability-adjusted life years (2008-2020). J Korean Med Sci. 2024; 39:e67.
79. Heneweer H, Picavet HS, Staes F, Kiers H, Vanhees L. Physical fitness, rather than self-reported physical activities, is more strongly associated with low back pain: evidence from a working population. Eur Spine J. 2012; 21:1265–72.


80. LaMonte MJ, Blair SN, Church TS. Physical activity and diabetes prevention. J Appl Physiol (1985). 2005; 99:1205–13.


81. Li X, Feng X, Sun X, Hou N, Han F, Liu Y. Global, regional, and national burden of Alzheimer’s disease and other dementias, 1990-2019. Front Aging Neurosci. 2022; 14:937486.


82. Health Insurance Review and Assessment Service. Analysis of the treatment status for depression and anxiety disorders over the last five years (2017-2021). Available from: https://url.kr/lz4a5t (cited 2024 Jun 19).
83. Morrow RH, Hyder AA, Murray CJ, Lopez AD. Measuring the burden of disease. Lancet. 1998; 352:1859–61.


84. Go DS, Kim YE, Paik JW, Roh S, Yoon SJ. A comparison of disease burden and the government budget for mental health in Korea. J Ment Health. 2022; 31:471–8.
85. Smith N, Mitton C, Davidson A, Williams I. A politics of priority setting: ideas, interests and institutions in healthcare resource allocation. Public Policy Adm. 2014; 29:331–47.


86. Choi HH, Choi G, Yoon H, Ha KH, Kim DJ. Rising incidence of diabetes in young adults in South Korea: a national cohort study. Diabetes Metab J. 2022; 46:803–7.


87. Yang YS, Han K, Sohn TS, Kim NH. Young-onset type 2 diabetes in South Korea: a review of the current status and unmet need. Korean J Intern Med. 2021; 36:1049–58.


88. Hong YH, Chung IH, Han K, Chung S; Taskforce Team of the Obesity Fact Sheet of the Korean Society for the Study of Obesity. Prevalence of type 2 diabetes mellitus among Korean children, adolescents, and adults younger than 30 years: changes from 2002 to 2016. Diabetes Metab J. 2022; 46:297–306.


89. Yang YS, Han BD, Han K, Jung JH, Son JW; Taskforce Team of the Obesity Fact Sheet of the Korean Society for the Study of Obesity. Obesity fact sheet in Korea, 2021: trends in obesity prevalence and obesity-related comorbidity incidence stratified by age from 2009 to 2019. J Obes Metab Syndr. 2022; 31:169–77.
90. Jung YS, Kim YE, Go DS, Yoon SJ. Projecting the prevalence of obesity in South Korea through 2040: a microsimulation modelling approach. BMJ Open. 2020; 10:e037629.


91. Bae JH, Han KD, Ko SH, Yang YS, Choi JH, Choi KM, et al. Diabetes fact sheet in Korea 2021. Diabetes Metab J. 2022; 46:417–26.


92. Oh SH, Ku H, Park KS. Prevalence and socioeconomic burden of diabetes mellitus in South Korean adults: a population-based study using administrative data. BMC Public Health. 2021; 21:548.


93. Tomic D, Shaw JE, Magliano DJ. The burden and risks of emerging complications of diabetes mellitus. Nat Rev Endocrinol. 2022; 18:525–39.


94. Song SJ, Han K, Choi KS, Ko SH, Rhee EJ, Park CY, et al. Trends in diabetic retinopathy and related medical practices among type 2 diabetes patients: results from the National Insurance Service Survey 2006-2013. J Diabetes Investig. 2018; 9:173–8.
95. Moon SS, Kim CH, Kang SM, Kim ES, Oh TJ, Yun JS, et al. Status of diabetic neuropathy in Korea: a National Health Insurance Service-National Sample Cohort Analysis (2006 to 2015). Diabetes Metab J. 2021; 45:115–9.


96. Jung CH, Chung JO, Han K, Ko SH, Ko KS, Park JY, et al. Improved trends in cardiovascular complications among subjects with type 2 diabetes in Korea: a nationwide study (2006-2013). Cardiovasc Diabetol. 2017; 16:1.


97. Nouwen A, Adriaanse MC, van Dam K, Iversen MM, Viechtbauer W, Peyrot M, et al. Longitudinal associations between depression and diabetes complications: a systematic review and meta-analysis. Diabet Med. 2019; 36:1562–72.


98. Xue M, Xu W, Ou YN, Cao XP, Tan MS, Tan L, et al. Diabetes mellitus and risks of cognitive impairment and dementia: a systematic review and meta-analysis of 144 prospective studies. Ageing Res Rev. 2019; 55:100944.


99. Ezzati M, Lopez AD, Rodgers A, Murray CJL. Comparative quantification of health risks: global and regional burden of disease attributable to selected major risk factors. Geneva: World Health Organization;2004. Chapter 27, Potential health gains from reducing multiple risk factors. p. 2167–91.
100. Park MK, Park HK, Kim HJ, Kim OJ, Gong MY. Health management experience of residents through participation in the community based hypertension and diabetes registry program. J Korean Acad Community Health Nurs. 2021; 32:518–28.


101. Yun SK. Current status and problems of national diabetes care. J Korean Diabetes. 2020; 21:173–8.


102. Ministry of Health and Welfare. An overview of Ministry of Health and Welfare budget and fund operation plan in 2024. Available from: https://url.kr/koqp6w (cited 2024 Jun 19).
103. Song E, Kim YE, Ji S. Impact of a primary health care chronic diseases management pilot program. Korean J Med. 2021; 96:7–12.


104. Ganz M. Prevention of type 2 diabetes. Chichester: John Wiley & Sons;2005. Chapter 9, A paradigm shift is needed in the primary prevention of type 2 diabetes. p. 153–68.
105. Pryor K, Volpp K. Deployment of preventive interventions: time for a paradigm shift. N Engl J Med. 2018; 378:1761–3.
Fig. 1.
An illustration of the concept of disability-adjusted life year. Disease A, B, C, and death mean disease and death occurrence.
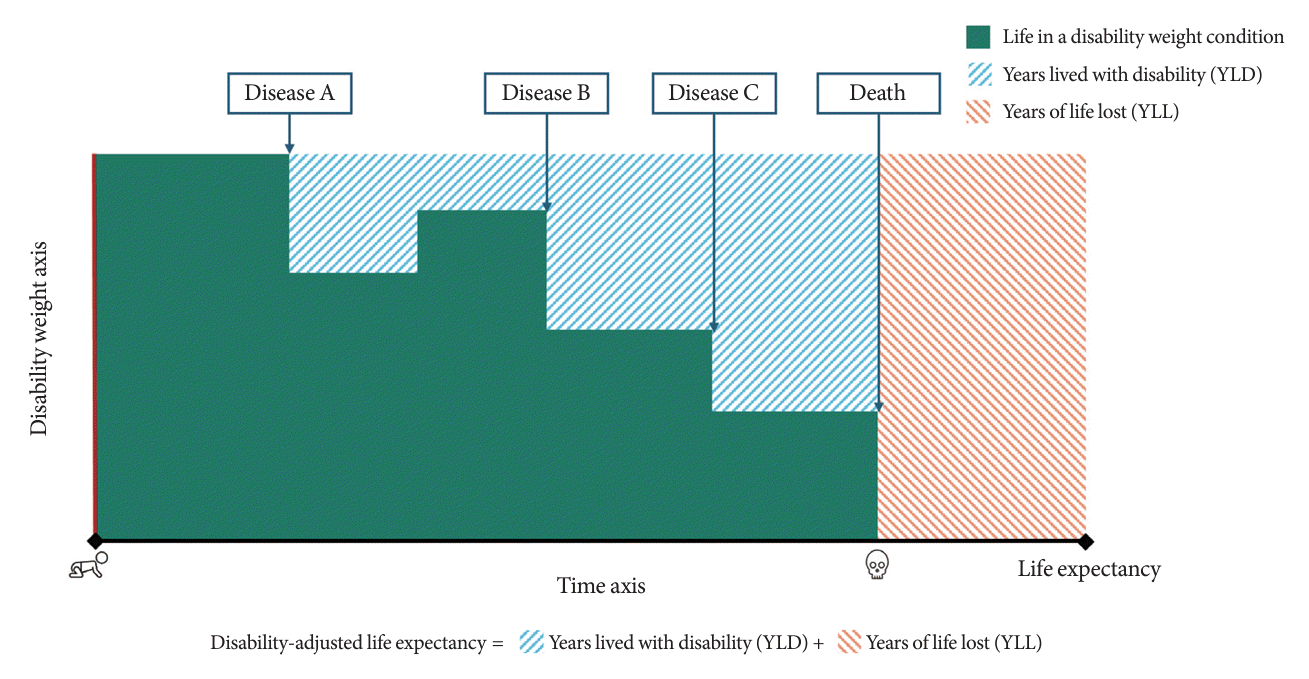
Fig. 2.
(A) Top 20 leading causes of burden of diseases in Korea. This figure represents a restructured version of the authors’ research results. Adapted from Jung et al. [78]. (B) Trends of Korean national burden of diabetes mellitus by sex. DALY, disability-adjusted life year; TNI, tubulointerstitial nephritis; UNI, urinary tract infection.
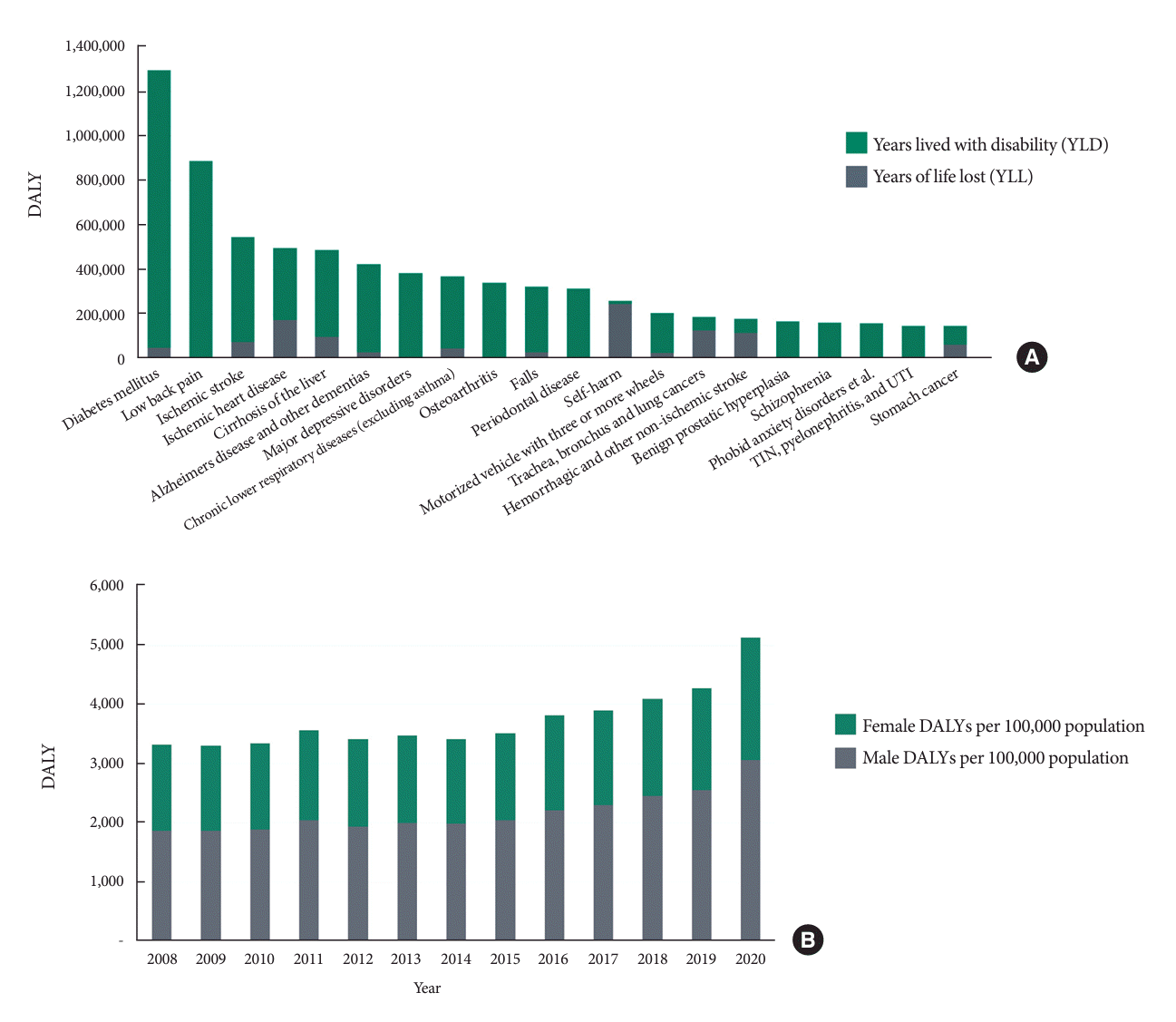
Table 1.
Summary of incidence-based approach and prevalence-based approach