Abstract
Background
Methods
Results
ACKNOWLEDGEMENTS
Notes
SUPPLEMENTARY MATERIALS
Supplementary materials can be found via https://doi.org/10.3343/alm.2023.0345
AUTHOR CONTRIBUTIONS
Zheng L and Guo C conceived the study. Chen W and Yu P curated the data. Yu P and Cai S analyzed the data. Chen W, Zheng C, and Zheng L developed the methods used in the study. Zhen L and Guo C were responsible for project administration. Chen W, Cai S, and Guo C acquired resources for the project. Zheng C developed software. Chen Chao and Chen Chaojin supervised the project. Chen Chao and Chen J validated the findings. Chen W, Chen Chao, and Chen J drafted the original manuscript. Yu P and Chen Chaojin reviewed and edited the manuscript. All the authors have read and approved the final manuscript.
References

































Fig. 1
Study flow chart showing the inclusion and exclusion criteria.
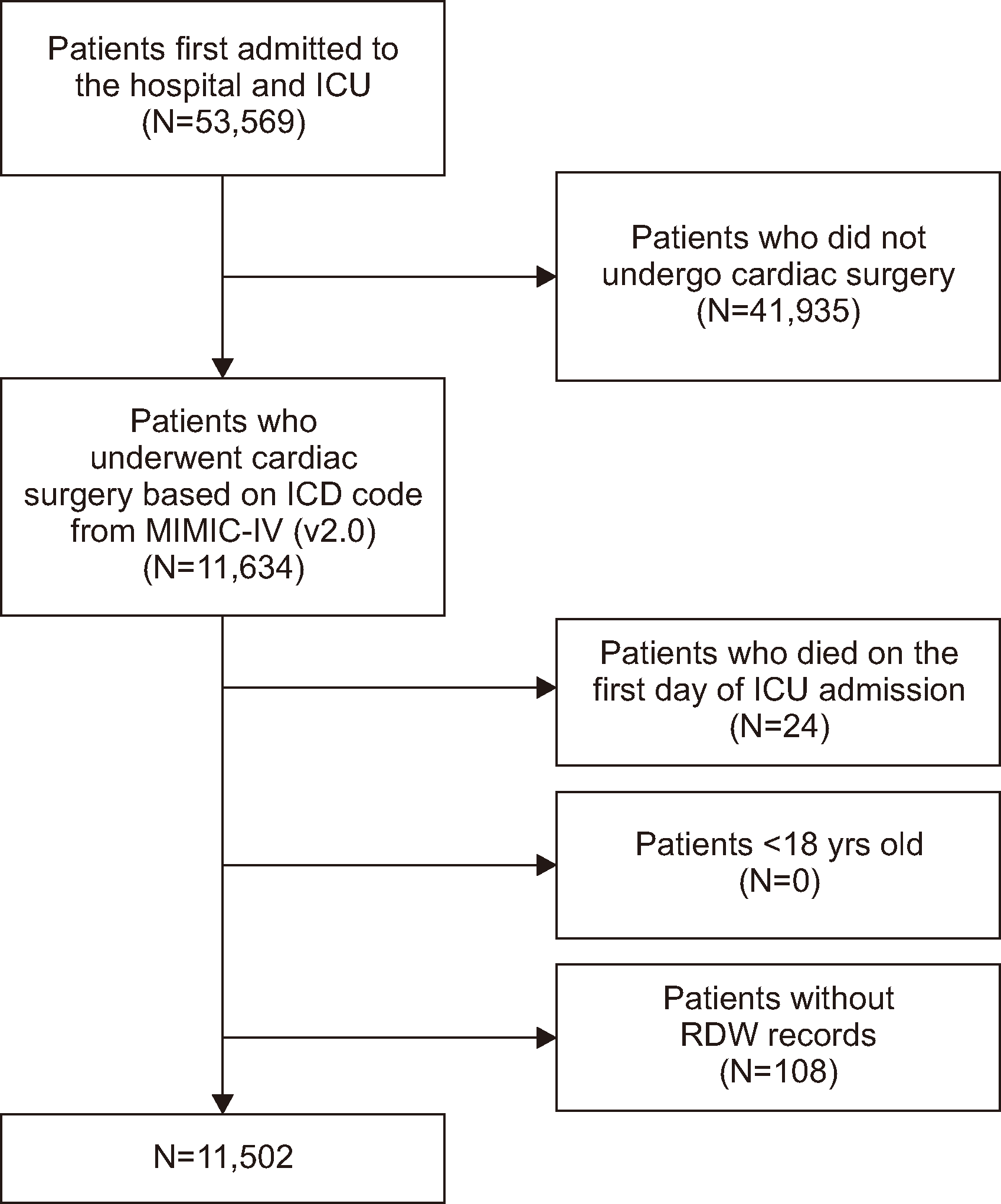
Fig. 2
Kaplan–Meier curves showing the association between the red blood cell distribution width (RDW) and the 30-day mortality of patients after undergoing cardiac surgery.
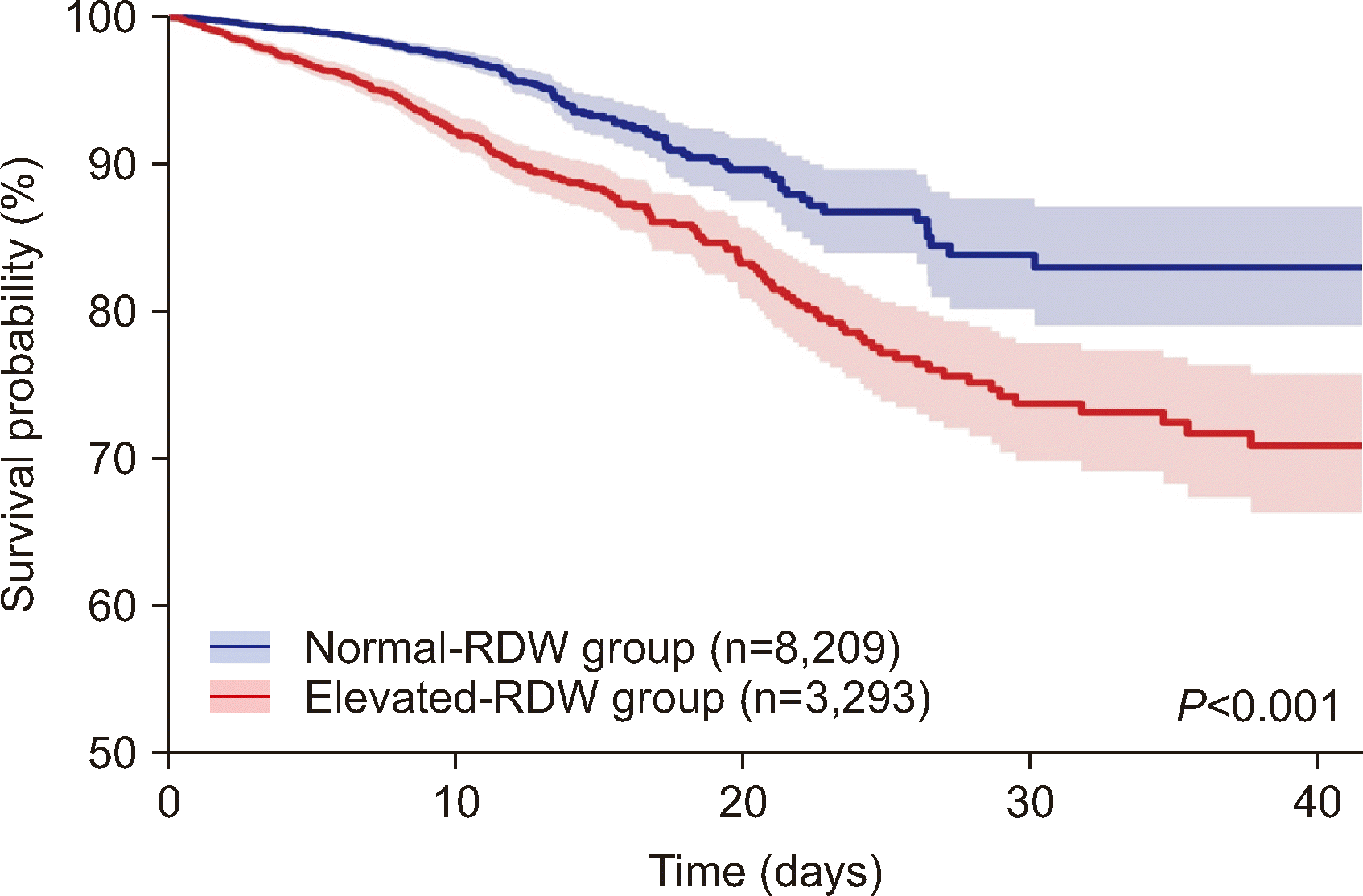
Fig. 3
Forest plot showing subgroup analysis of the association between red blood cell distribution width (RDW) and 30-day mortality.
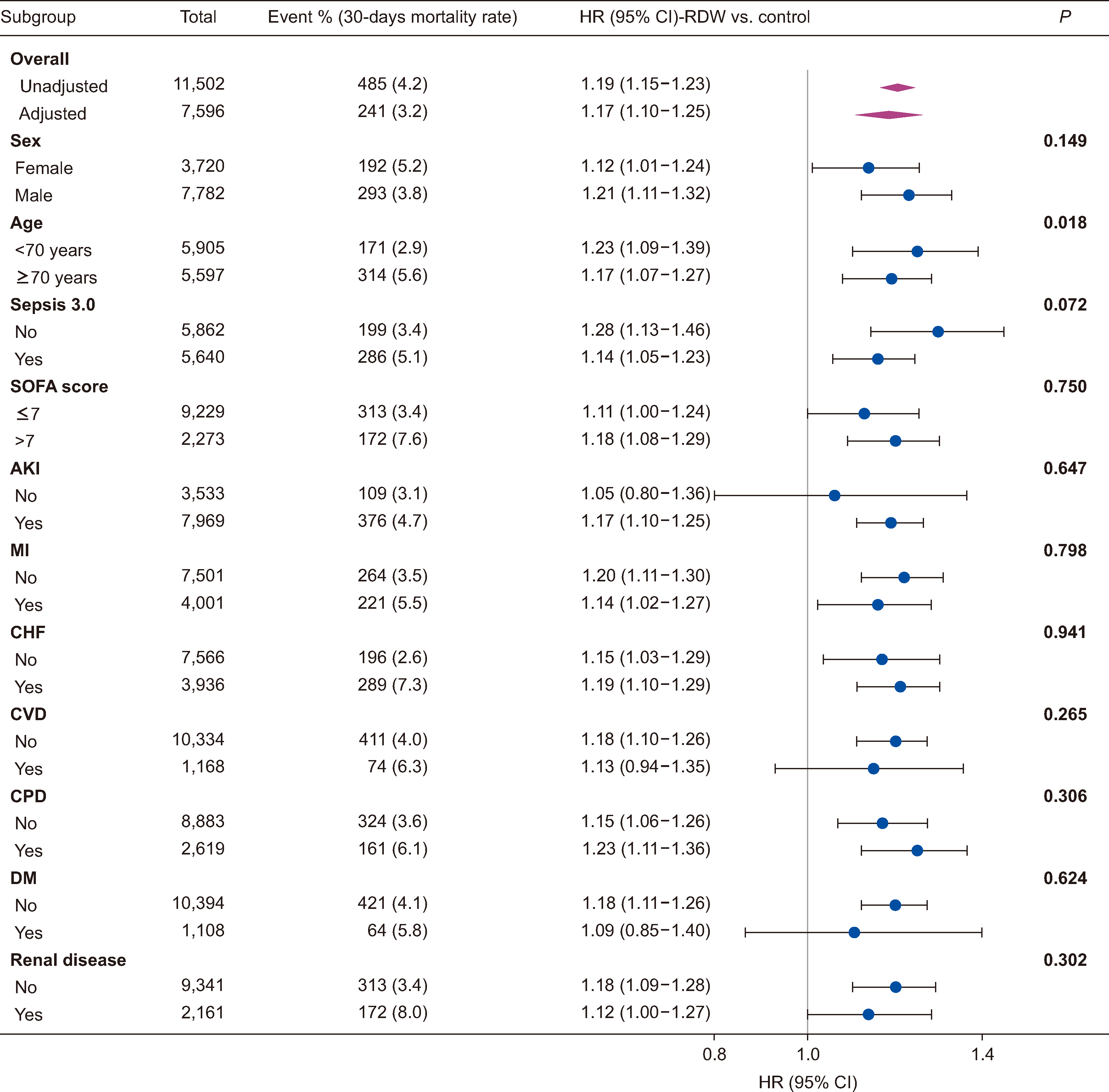
Table 1
Baseline characteristics of the study participants and their outcome parameters
Data were presented as mean±SD or median (first quartile, third quartile) based on their distribution.
Abbreviations: RDW, red blood cell distribution width; BMI, body–mass index; MI, myocardial infarction; CHF, congestive heart failure; CVD, cerebrovascular disease; CPD, chronic pulmonary disease; DM, diabetes mellitus (with complications); CCI, Charlson comorbidity index; SOFA, sequential organ failure assessment; SAPS II, Simplified Acute Physiology Score II; HR, heart rate; bpm, beats/min; MAP, mean artery pressure; RR, respiratory rate; SpO2, peripheral oxygen saturation; Hb, hemoglobin; PLT, platelet; WBC, white blood cell; BUN, blood urea nitrogen; CR, creatinine; GLU, glucose; K, potassium; LAC, lactate; P/F, partial pressure of oxygen: fraction of inspired oxygen ratio; BE, base excess; GCS, Glasgow Coma Scale; AKI, acute kidney injury; Los, length of stay; ICU, Intensive care unit.
Table 2
Multivariate Cox regression to assess the association between the RDW and 30-day mortality
Variables | Normal RDW | Elevated RDW | RDW | ||||
---|---|---|---|---|---|---|---|
HR | HR (95% CI) | P | HR (95% CI) | P | |||
Unadjusted | 1 | 2.32 (2.14–2.52) | <0.001 | 1.19 (1.17–1.21) | <0.001 | ||
Model 1* | 1 | 2.20 (2.02–2.39) | <0.001 | 1.17 (1.16–1.19) | <0.001 | ||
Model 2† | 1 | 2.00 (1.83–2.18) | <0.001 | 1.16 (1.14–1.18) | <0.001 | ||
Model 3‡ | 1 | 1.83 (1.68–2.00) | <0.001 | 1.13 (1.11–1.15) | <0.001 | ||
Model 4§ | 1 | 1.84 (1.69–2.01) | <0.001 | 1.13 (1.11–1.15) | <0.001 | ||
Model 5ІІ | 1 | 1.79 (1.64–1.96) | <0.001 | 1.12 (1.10–1.14) | <0.001 |
*Models 1–5 were derived from multivariate Cox regression models. Model 1 covariates were adjusted for sex, age, and body–mass index (BMI).
†Model 2 covariates were adjusted for sex, age, BMI, myocardial infarction, congestive heart failure, cerebrovascular disease, chronic pulmonary disease, diabetes with complications, and renal disease.
‡Model 3 covariates were adjusted for sex, age, BMI, myocardial infarction, congestive heart failure, cerebrovascular disease, chronic pulmonary disease, diabetes with complications, renal disease, Charlson comorbidity index, sequential organ failure assessment (SOFA) score, and Simplified Acute Physiology Score (SAPS) II.
§Model 4 covariates were adjusted for sex, age, BMI, myocardial infarction, congestive heart failure, cerebrovascular disease, chronic pulmonary disease, diabetes with complications, renal disease, Charlson comorbidity index, SOFA score, SAPS II, heart rate, mean arterial pressure (MAP), respiratory rate, and peripheral oxygen saturation (SpO2).
ІІModel 5 covariates were adjusted for sex, age, BMI, myocardial infarction, congestive heart failure, cerebrovascular disease, chronic pulmonary disease, diabetes with complications, renal disease, Charlson comorbidity index, SOFA score, SAPS II, heart rate, MAP, respiratory rate, SpO2, hemoglobin, platelets, white blood cells, blood urea nitrogen, creatinine, glucose, potassium, lactate, partial pressure of oxygen: fraction of inspired oxygen ratio, Glasgow Coma Scale, and base excess.