Abstract
Evaluating cell metabolism is crucial during pluripotent stem cell (PSC) differentiation and somatic cell reprogramming as it affects cell fate. As cultured stem cells are heterogeneous, a comparative analysis of relative metabolism using existing metabolic analysis methods is difficult, resulting in inaccuracies. In this study, we measured human PSC basal metabolic levels using a Seahorse analyzer. We used fibroblasts, human induced PSCs, and human embryonic stem cells to monitor changes in basal metabolic levels according to cell number and determine the number of cells suitable for analysis. We evaluated normalization methods using glucose and selected the most suitable for the metabolic analysis of heterogeneous PSCs during the reprogramming stage. The response of fibroblasts to glucose increased with starvation time, with oxygen consumption rate and extracellular acidification rate responding most effectively to glucose 4 hours after starvation and declining after 5 hours of starvation. Fibroblasts and PSCs achieved appropriate responses to glucose without damaging their metabolism 2∼4 and 2∼3 hours after starvation, respectively. We developed a novel method for comparing basal metabolic rates of fibroblasts and PSCs, focusing on quantitative analysis of glycolysis and oxidative phosphorylation using glucose without enzyme inhibitors. This protocol enables efficient comparison of energy metabolism among cell types, including undifferentiated PSCs, differentiated cells, and cells undergoing cellular reprogramming, and addresses critical issues, such as differences in basal metabolic levels and sensitivity to normalization, providing valuable insights into cellular energetics.
Studying cellular energy metabolism can help identify the biochemical pathways that produce and consume adenosine triphosphate (ATP), and intermediate metabolites and elucidate the interconnected processes and changes in cellular signal control mechanisms. Metabolic flux changes distinctly and dynamically during early human embryonic development, human pluripotent stem cell (hPSC) differentiation, and fibroblast reprogramming, with metabolic levels varying depending on the tissue (1-6). The metabolic dynamics of these stem cells indicate that cell metabolism is important in supporting energy demands and is closely related to cell identity, function, gene expression, phenotype, and epigenetic factors (7-14). Particularly, controlling the glucose metabolic pathway during stem cell differentiation or reprogramming regulates or increases the efficiency of these mechanisms (15, 16).
Following cellular uptake, glucose enters the glycolysis pathway to produce ATP and pyruvate, which is converted into two acetyl-coenzyme A (acetyl-CoA) molecules that enter the mitochondria or lactate and excreted from the cell via the monocarboxylate transporter in the plasma membrane (17). The two acetyl-CoAs produced by pyruvate go through the tricarboxylic acid (TCA) cycle to provide energy products to the electron transport chain for cellular respiration within the mitochondrial matrix, with the mitochondria using oxygen to produce ATP (18). Intermediate metabolites generated during pyruvate production are used to synthesize nucleotide precursors via the pentose phosphate pathway (PPP) (19-21). Therefore, glucose metabolism is important in producing both cellular energy and the various precursors required in cellular homeostasis, thus delicately regulating the maintenance of cell properties and fate (13, 22, 23). Additionally, quantitative measurement of metabolic levels is important for evaluating cellular functions, characteristics, and cell fate.
Pluripotent stem cells (PSCs), which can differentiate into various cells and proliferate indefinitely via self-renewal, include human embryonic stem cells (hESCs) derived from fertilized eggs and human induced pluripotent stem cells (hiPSCs) generated by introducing four genes into fibroblasts (24, 25). Cell differentiation into various target cells by an appropriate induction factor in vitro and maintenance of the newly acquired cell characteristics require a shift in energy metabolism (26-28). PSCs and differentiated fibroblasts exhibit different energy metabolism characteristics (7). PSCs, which have less-mature mitochondria than differentiated cells, more actively produce ATP via glycolysis, which is less efficient for energy production than oxidative phosphorylation (OXPHOS, i.e., mitochondrial function) (5, 7, 13, 29, 30). This is because PSCs, which proliferate faster than fibroblasts, require active nucleotide and lipid biosynthesis, resulting in an increased flux via the PPP (7, 31).
To measure cellular metabolic characteristics and activity, the oxygen consumption rate (OCR) and extracellular acidification rate (ECAR) are measured using the Seahorse XF96 Extracellular Flux Analyzer (Seahorse Bioscience), which quantifies glycolysis and OXPHOS by inhibiting the functions of glycolytic and mitochondrial enzymes (32, 33). Oligomycin (complex V inhibitor), FCCP (trifluoromethoxy carbonylcyanide phenylhydrazone, carbonyl cyanide 4-(trifluoromethoxy)phenylhydrazone, ATPase inhibitor), antimycin A (complex III inhibitor), and rotenone (complex I inhibitor) measure mitochondrial respiration (32, 33). Metabolic level differences between somatic cells or within cell lines can be efficiently evaluated in this way. However, as PSCs and reprogrammed somatic cells are highly heterogeneous compared with other animal cells and form colonies, it is difficult to precisely describe their metabolic characteristics (33). Metabolic analysis using the Seahorse XF96 analyzer fails to adequately represent the characteristics of various cell types, including stem cells, and can sometimes disrupt mitochondrial respiratory function or, particularly in the case of sensitive cells, such as PSCs, induce metabolic stress and cell death when conventional mitochondrial inhibitors are used (33-35). Furthermore, optimizing inhibitor concentrations and processing times can be challenging, especially when assessing cellular metabolic rates in heterogeneous cell populations, such as those undergoing reprogramming or differentiation (33-35). Therefore, a novel analysis method is required. To address this, we developed a novel method for measuring stem cell energy metabolism using metabolic substrates, tested the method under starvation conditions, and developed novel normalization methods.
To compare the metabolic differences between PSCs and somatic cells, hESCs (H1 and H9; WiCell Research Institute), hiPSCs (CMC-hiPSC-003 and CMC-hiPSC-009; (National Center for Stem Cell and Regenerative Medicine) and fibroblast cell lines (BJ1 and MRC5) were used. This study was approved by the Institutional Review Board (IRB No: P01-202309-03-001). These PSCs were maintained in complete TeSR-E8 Medium (STEMCELL Technologies) as the glucose-free culture. Cells were cultured on Vitronectin XF-coated plates (STEMCELL Technologies) and dissociated by incubation with TrypLE Express (Gibco) at 37℃ for 3 minutes. The fibroblast cell lines, BJ1 and MRC5, were maintained in Dulbecco’s modified Eagle medium (high-glucose DMEM; Corning Life Sciences) supplemented with 10% fetal bovine serum (FBS; Corning Life Sciences) and 100× penicillin-streptomycin (Corning Life Sciences). hESCs and hiPSCs were routinely passaged every 3 days using TrypLE Express, whereas fibroblasts were passaged every 3∼5 days using 0.05% trypsin-ethylenediaminetetraacetic acid. All cells were maintained in an incubator at 37℃ and 5% CO2.
hiF-T fibroblasts (Davide Cacchiarelli; Broad Institute) for reprogramming were cultured in an optimized DMEM/F12 culture medium supplemented with 10% FBS. Reprogramming was performed using the KnockOut Serum Replacement media formulation (Invitrogen, Gibco) and doxycycline hyclate (D9891; Sigma-Aldrich), according to the manufacturer’s instructions. hiF-T-derived hiPSCs were cultured in TeSR-E8 Medium containing Vitronectin XF.
OCR and ECAR were measured using a Seahorse XF96 Extracellular Flux Analyzer, according to the manufacturer’s instructions. Cells were plated into the wells of an XF96 cell culture microplate and incubated at 37℃ in a CO2 incubator for 24 hours to ensure attachment. The assay was initiated after cells were equilibrated for 1 hour in XF assay medium supplemented with 10 mM glucose, 5 mM sodium pyruvate, and 2 mM glutamine in a non-CO2 incubator. Substrate-based metabolic assays were performed by injecting 10 mM glucose after starvation in XF DMEM assay media (pH 7.4; Seahorse Bioscience). For starvation, the culture medium was removed at hourly intervals for a total of 5 hours, washed once with phosphate-buffered saline, and starvation was induced by adding XF DMEM assay media (without glucose, pyruvate, and glutamine). During the starvation process, cells were maintained at 37℃ in a CO2 incubator. The ATP rate assay involved sequential injection of 2 μM oligomycin and 0.5 μM rotenone/antimycin A.
Seahorse XF96-derived OCR and ECAR measurement accuracy depends on probe resolution and measurement range. These measurements require an appropriate measurement range (36, 37). However, the differences between cell types in metabolic levels make it difficult to obtain accurate results, leading to a wide range of measurements. During PSC metabolic analysis, in particular, it is often necessary to compare differentiated and undifferentiated cells or to compare reprogrammed cells with each other. To address this, we used six cell lines (fibroblasts [MRC5 and BJ1], hiPSCs [iPSC-003 and iPSC-009], and hESCs [H1 and H9]) to obtain the appropriate number of PSCs and somatic fibroblast cells for precisely analyzing stem cell energy metabolism. We evaluated changes in OCR (pmol/min), ECAR (mpH/min), and the OCR/ECAR ratio according to the number of cells.
We seeded hiPSCs and hESCs at 2.5×103, 5.0×103, 1.0×104, 2.0×104, 3.0×104, 4.0×104, 5.0×104, 6.0×104, 7.0×104, and 8.0×104 cells/well in 96-well microplates. The XF DMEM assay media (pH 7.4) was replaced after 24 hours, following washing with phosphate-buffered saline. We measured OCR and ECAR to perform linear regression analysis and analyzed the changes in the OCR/ECAR ratio (Fig. 1). In all cell types, OCR and ECAR increased with cell number (R2>0.9) (Fig. 1A) at a constant rate, whereas the OCR/ECAR ratio remained constant only in a specific cell-number range (Fig. 1B). Fibroblasts exhibited a constant OCR/ECAR ratio at 1.0×104 to 8.0×104 cells/well, whereas hiPSCs, H1, and H9 cells showed a consistent ratio in the ranges 2.0×104 to 8.0×104, 2.0×104 to 8.0×104, and 3.0×104 to 8.0×104, respectively. Fibroblasts exhibited measurements at lower cell numbers than PSCs; however, PSC measurements were normal, with a consistent OCR/ECAR ratio, only when at least 2.0×104 cells were seeded. Regardless of cell type, we detected measurements only in the cell number range that exhibited marginal changes in ECAR and OCR. Overall, compared with fibroblasts, PSCs exhibited lower metabolic levels, and higher PSC cell numbers were required to detect changes in OCR or ECAR. These results indicate that when energy metabolism analysis is performed using different cell types, it is necessary to first consider the appropriate numbers of seeded cells and review the basal metabolic rates of the cell types. Specifically, before metabolic analysis and after monitoring changes in OCR and ECAR based on cell number, it is recommended to determine the appropriate cell number that achieves a linear increase (R2=0.9) with OCR or ECAR and confirm a constant OCR/ECAR ratio. Based on these findings, for these six cell lines, we propose using 3.0×104 to 8.0×104 cells/well to enable the acquisition of consistent cellular energy metabolism values.
Various stem cell types, including ESCs, are maintained in a heterogeneous state (38); hence, their susceptibility to mitochondrial enzyme inhibitors varies. To analyze their diverse metabolic characteristics, it is more effective to use glucose as a metabolic substrate to measure cellular metabolism rather than using mitochondrial enzyme inhibitors. Furthermore, as cells cultured in heterogeneous states are at different cell cycle stages, their energy metabolism levels differ. Therefore, glucose starvation is required to align their metabolic states before metabolic analysis. Depending on their metabolic characteristics, cells consume different amounts of glucose, varying in their glucose uptake and metabolism mechanisms and ability (39, 40). This suggests that induced starvation, followed by glucose supplementation, could provide a more natural method for evaluating the inherent metabolic ability of heterogeneous stem cell populations. Therefore, we compared the metabolic characteristics of stem and somatic cells by applying glucose starvation and supplementation.
Before this experiment, to determine the optimal starvation conditions under which each cell line responds to glucose, we took measurements every hour for 5 hours using glucose-free assay media to induce starvation, after which we added glucose to evaluate changes in metabolism. The response of fibroblasts (BJ1 cells) to glucose increased markedly with starvation time, with OCR and ECAR responding most effectively to glucose 4 hours after starvation and declining after 5 hours of starvation (Fig. 2A, 2B, Supplementary Fig. S1A, S1B). However, for PSCs (hiPSCs and H1 cells), ECAR responded the most to glucose starvation at 2∼3 hours of starvation, declining from 4 hours, with greater SDs between measurements from 4 hours onward (Fig. 2B, Supplementary Fig. S1B). Consequently, glucose starvation for 2∼4 hours for fibroblasts and 2∼3 hours for PSCs achieved appropriate responses to glucose without damaging their metabolism (Fig. 2A, 2B, Supplementary Fig. S1A, S1B).
Cells with different cell characteristics have different structural systems for glucose uptake and maintenance of cellular homeostasis. Thus, it is preferable to measure metabolism after the complete consumption of the glucose present in cells. Accordingly, we applied glucose starvation before measuring cell metabolism. By adding glucose after various periods of cell starvation and analyzing cell metabolism, glycolysis and OXPHOS can be measured by observing glucose consumption. This protocol can be applied to PSCs at both extremes of differentiation from somatic cells and to cells during differentiation or somatic cell reprogramming. Although this method does not exclude nonmitochondrial oxygen respiration and nonglycolytic acidification (i.e., due to CO2 produced by the TCA cycle), it enables comparison of relative metabolic capacity via analysis and normalization of basal metabolic rates in different cells.
To verify the validity of this method, we examined whether we could quantitatively compare the metabolic capacity of cells during reprogramming by providing glucose following 3 hours of glucose starvation. The human secondary reprogrammed hiF-T cell line (used in the dox-inducible reprogramming system) (40) was subjected to doxycycline treatment for 20 days to induce reprogramming, generating TRA-1-60 + cells, which are pluripotency markers. MRC5 and H1 cells were used as positive controls for fibroblasts and hiPSCs, respectively. The hiF-T and TRA-1-60 + cells were seeded at 5.0×104 cells/well and treated with glucose after starvation for 3 hours (Fig. 2C, Supplementary Fig. S1C). Following glucose starvation and treatment, OCR and ECAR were significantly elevated in fibroblasts (MRC5 and hiF-T cells), whereas only ECAR was significantly elevated in PSCs (hiPSCs and H1 cells) (Fig. 2C, Supplementary Fig. S1C). These results indicated that undifferentiated cells not only have a low metabolic level but also depend on glycolysis. Moreover, we revealed that this method can effectively compare metabolic capacity and OXPHOS and glycolysis usage during cellular differentiation or reprogramming.
For quantitatively comparing metabolic capacity across cell types, performing normalization via protein quantification or using the same cell number is common. However, neither approach achieves accurate normalization because, regardless of how accurately cell number is measured, errors caused by differences in cell viability and cell-counting accuracy might affect the interpretation of results. Furthermore, normalization using total protein concentration does not reflect cell viability nor protein amount per cell, which varies depending on cell type (Fig. 3A, 3B). For instance, besides cell size differences, the nucleus-to-cytoplasmic size ratio is high in PSCs but low in somatic cells (Fig. 3A). During reprogramming, cell protein content varies with cell size, with cell size and total protein content decreases (Fig. 3B). Thus, when comparing the metabolism of different cell types, normalization using total protein content is neither suitable nor accurate. To overcome these problems and to accurately analyze the metabolic levels of undifferentiated PSCs and fibroblasts, information on the basal metabolic rate is required, which depends on the respiratory rate and varies between cells.
We conducted an interval-based assay for each cell type to identify their unique basal metabolic rates and determine the cell numbers required for analysis. This assay is based on the premise that when cell number increases at a constant rate, each cell type will exhibit a unique and constant increase in metabolic level. Fibroblastic hiF-T cells, reprogrammed hiF-T cells (TRA-1-60 +), fibroblasts (MRC5), and PSCs (H1) were diluted two-fold starting from 1.0×108 cells and were seeded in a 96-well plate. The increase in OCR and ECAR was then analyzed using the Seahorse XF96 analyzer. We analyzed the linear correlation between cell number and respiration rate to quantify the increase in metabolism with cell number.
OCR and ECAR were more highly correlated in fibroblasts (including fibroblastic hiF-T cells) than in PSCs (Fig. 3C). The cell types exhibited unique OCR and ECAR intervals according to their metabolic characteristics. Similar to previous findings (16), cellular metabolic levels decreased during reprogramming, whereas glucose metabolism increased. After calculating the differences in the numbers of respiring cells by substituting the OCR and ECAR values into the standard curve for each cell type, the relative differences in cell numbers were used to normalize the obtained values. As this normalization method provides a standard curve based on the change in cell number and metabolic level, it appears to be more accurate than the protein content-based method. Comparison of the basal metabolic rate via interval-based assays, followed by cell number normalization using the standard curve, might be useful for comparing the basal metabolic characteristics of differentiated or reprogrammed cells.
To confirm the validity of our normalization method, we conducted a real-time ATP rate assay and analyzed the results according to the calibration method used. The real-time ATP rate assay is the only assay that quantifies the live-cell rate of ATP production from glycolysis and mitochondria using label-free technology. We seeded the same number of MRC5, hiF-T, TRA-1-60 +, and hESCs (8.0×104 cells/well each) and performed a real-time ATP rate assay. OCR (Fig. 4A) and ECAR (Fig. 4B) changed more dynamically in fibroblasts than in hPSCs, indicating significantly higher ATP production in fibroblasts. After correcting these values using the total amount of protein or respiratory rate, we analyzed the differences in ATP production rates according to the normalization method used (Fig. 4C). Normalization using total protein produced a lower ATP production rate than normalization using the respiratory rate. Total protein-based normalization produced a significantly lower ATP production rate in reprogrammed cells and PSCs than in somatic cells, whereas this difference was not detected using respiratory rate-based normalization (Fig. 4C). This indicates that total protein-based normalization might over-correct the metabolic values for PSCs.
Our study presents a novel method for comparing the basal metabolic rates of fibroblasts and PSCs, focusing on quantitative analysis of glycolysis and OXPHOS using glucose without enzyme inhibitors. We successfully determined the optimal cell number for the metabolic analysis of undifferentiated stem cells, compared metabolic capacities following glucose supplementation after starvation, and verified the applicability of this approach by determining basal metabolic levels. This protocol enables efficient comparison of energy metabolism among different cell types, including undifferentiated PSCs, differentiated cells, and cells undergoing cellular reprogramming. Moreover, it addresses critical issues, such as differences in basal metabolic levels and sensitivity to normalization, providing valuable insights into cellular energetics.
Supplementary data including one figure can be found with this article online at https://doi.org/10.15283/ijsc23167
Acknowledgments
We express our sincere gratitude to Davide Cacciarelli from Broad Institute for generously providing us with the hiF-T cell line used in this study for human reprogramming research. We would also like to thank the Soonchunhyang Biomedical Research Core Facility of the Korea Basic Science Institute for their help with microscopy. Special thanks go to STEMOPIA.
Notes
Authors’ Contribution
Conceptualization: HKK, JSO, SHM, JSM, MRL. Data curation: HKK, YS, SBP, JHK, MK, BY, HC, MRL. Formal analysis: HKK, YS, MK, BY, MRL. Funding acquisition: MRL. Methodology: HKK, JSO, JSM, SHM, MRL. Project administration: JSO, SHM, MRL. Supervision: MRL. Validation: HKK, JSO, JSM, MRL. Visualization: HKK, YS, MK, BY, MRL. Writing – original draft: HKK. Writing – review and editing: HKK, MRL. All authors have read and agreed to the published version of the manuscript.
References
1. Zhang J, Nuebel E, Daley GQ, Koehler CM, Teitell MA. 2012; Metabolic regulation in pluripotent stem cells during reprogramming and self-renewal. Cell Stem Cell. 11:589–595. DOI: 10.1016/j.stem.2012.10.005. PMID: 23122286. PMCID: PMC3492890.


2. Nishimura K, Fukuda A, Hisatake K. 2019; Mechanisms of the metabolic shift during somatic cell reprogramming. Int J Mol Sci. 20:2254. DOI: 10.3390/ijms20092254. PMID: 31067778. PMCID: PMC6539623.


3. Agathocleous M, Love NK, Randlett O, et al. 2012; Metabolic differentiation in the embryonic retina. Nat Cell Biol. 14:859–864. DOI: 10.1038/ncb2531. PMID: 22750943. PMCID: PMC3442239.


4. Facucho-Oliveira JM, Alderson J, Spikings EC, Egginton S, St John JC. 2007; Mitochondrial DNA replication during differentiation of murine embryonic stem cells. J Cell Sci. 120(Pt 22):4025–4034. DOI: 10.1242/jcs.016972. PMID: 17971411.


5. Prigione A, Fauler B, Lurz R, Lehrach H, Adjaye J. 2010; The senescence-related mitochondrial/oxidative stress pathway is repressed in human induced pluripotent stem cells. Stem Cells. 28:721–733. DOI: 10.1002/stem.404. PMID: 20201066.


6. Suhr ST, Chang EA, Tjong J, et al. 2010; Mitochondrial rejuvenation after induced pluripotency. PLoS One. 5:e14095. DOI: 10.1371/journal.pone.0014095. PMID: 21124794. PMCID: PMC2991355.


7. Xu X, Duan S, Yi F, Ocampo A, Liu GH, Izpisua Belmonte JC. 2013; Mitochondrial regulation in pluripotent stem cells. Cell Metab. 18:325–332. DOI: 10.1016/j.cmet.2013.06.005. PMID: 23850316.


8. Carey BW, Finley LW, Cross JR, Allis CD, Thompson CB. 2015; Intracellular α-ketoglutarate maintains the pluripotency of embryonic stem cells. Nature. 518:413–416. DOI: 10.1038/nature13981. PMID: 25487152. PMCID: PMC4336218.


9. Moussaieff A, Kogan NM, Aberdam D. 2015; Concise review: energy metabolites: key mediators of the epigenetic state of pluripotency. Stem Cells. 33:2374–2380. DOI: 10.1002/stem.2041. PMID: 25873344.


10. Shiraki N, Shiraki Y, Tsuyama T, et al. 2014; Methionine metabolism regulates maintenance and differentiation of human pluripotent stem cells. Cell Metab. 19:780–794. DOI: 10.1016/j.cmet.2014.03.017. PMID: 24746804.


11. Wellen KE, Hatzivassiliou G, Sachdeva UM, Bui TV, Cross JR, Thompson CB. 2009; ATP-citrate lyase links cellular metabolism to histone acetylation. Science. 324:1076–1080. DOI: 10.1126/science.1164097. PMID: 19461003. PMCID: PMC2746744.


12. Lunt SY, Vander Heiden MG. 2011; Aerobic glycolysis: meeting the metabolic requirements of cell proliferation. Annu Rev Cell Dev Biol. 27:441–464. DOI: 10.1146/annurev-cellbio-092910-154237. PMID: 21985671.


13. Ryall JG, Cliff T, Dalton S, Sartorelli V. 2015; Metabolic reprogramming of stem cell epigenetics. Cell Stem Cell. 17:651–662. DOI: 10.1016/j.stem.2015.11.012. PMID: 26637942. PMCID: PMC4672395.


14. Teslaa T, Teitell MA. 2015; Pluripotent stem cell energy metabolism: an update. EMBO J. 34:138–153. DOI: 10.15252/embj.201490446. PMID: 25476451. PMCID: PMC4337063.


15. Madonna R, Görbe A, Ferdinandy P, De Caterina R. 2013; Glucose metabolism, hyperosmotic stress, and reprogramming of somatic cells. Mol Biotechnol. 55:169–178. DOI: 10.1007/s12033-013-9668-2. PMID: 23657997.


16. Cha Y, Han MJ, Cha HJ, et al. 2017; Metabolic control of primed human pluripotent stem cell fate and function by the miR-200c-SIRT2 axis. Nat Cell Biol. 19:445–456. DOI: 10.1038/ncb3517. PMID: 28436968. PMCID: PMC5545746.


17. Kuo TC, Huang KY, Yang SC, et al. 2020; Monocarboxylate transporter 4 is a therapeutic target in non-small cell lung cancer with aerobic glycolysis preference. Mol Ther Oncolytics. 18:189–201. DOI: 10.1016/j.omto.2020.06.012. PMID: 32695876. PMCID: PMC7364124.


18. Alberts B, Johnson A, Lewis J, Raff M, Roberts K, Walter P. 2002. Molecular biology of the cell. 4th ed. Garland Science.
19. Tsogtbaatar E, Landin C, Minter-Dykhouse K, Folmes CDL. 2020; Energy metabolism regulates stem cell pluripotency. Front Cell Dev Biol. 8:87. DOI: 10.3389/fcell.2020.00087. PMID: 32181250. PMCID: PMC7059177.


20. Gu W, Gaeta X, Sahakyan A, et al. 2016; Glycolytic metabolism plays a functional role in regulating human pluripotent stem cell state. Cell Stem Cell. 19:476–490. DOI: 10.1016/j.stem.2016.08.008. PMID: 27618217. PMCID: PMC5055460.


21. Lees JG, Cliff TS, Gammilonghi A, et al. 2019; Oxygen regulates human pluripotent stem cell metabolic flux. Stem Cells Int. 2019:8195614. DOI: 10.1155/2019/8195614. PMID: 31236115. PMCID: PMC6545818.


22. Vander Heiden MG, Cantley LC, Thompson CB. 2009; Understanding the Warburg effect: the metabolic requirements of cell proliferation. Science. 324:1029–1033. DOI: 10.1126/science.1160809. PMID: 19460998. PMCID: PMC2849637.


23. DeBerardinis RJ, Lum JJ, Hatzivassiliou G, Thompson CB. 2008; The biology of cancer: metabolic reprogramming fuels cell growth and proliferation. Cell Metab. 7:11–20. DOI: 10.1016/j.cmet.2007.10.002. PMID: 18177721.


24. Takahashi K, Tanabe K, Ohnuki M, et al. 2007; Induction of pluripotent stem cells from adult human fibroblasts by defined factors. Cell. 131:861–872. DOI: 10.1016/j.cell.2007.11.019. PMID: 18035408.


25. Tachibana M, Amato P, Sparman M, et al. 2013; Human embryonic stem cells derived by somatic cell nuclear transfer. Cell. 153:1228–1238. DOI: 10.1016/j.cell.2013.05.006. PMID: 23683578. PMCID: PMC3772789.


26. Wang Q, Xiong Y, Zhang S, et al. 2021; The dynamics of metabolic characterization in iPSC-derived kidney organoid differentiation via a comparative omics approach. Front Genet. 12:632810. DOI: 10.3389/fgene.2021.632810. PMID: 33643392. PMCID: PMC7902935.


27. Kim YH, Heo JS, Han HJ. 2006; High glucose increase cell cycle regulatory proteins level of mouse embryonic stem cells via PI3-K/Akt and MAPKs signal pathways. J Cell Physiol. 209:94–102. DOI: 10.1002/jcp.20706. PMID: 16775839.


28. Folmes CD, Dzeja PP, Nelson TJ, Terzic A. 2012; Metabolic plasticity in stem cell homeostasis and differentiation. Cell Stem Cell. 11:596–606. DOI: 10.1016/j.stem.2012.10.002. PMID: 23122287. PMCID: PMC3593051.


29. Folmes CD, Nelson TJ, Martinez-Fernandez A, et al. 2011; Somatic oxidative bioenergetics transitions into pluripotency-dependent glycolysis to facilitate nuclear reprogramming. Cell Metab. 14:264–271. DOI: 10.1016/j.cmet.2011.06.011. PMID: 21803296. PMCID: PMC3156138.


30. Kondoh H, Lleonart ME, Nakashima Y, et al. 2007; A high glycolytic flux supports the proliferative potential of murine embryonic stem cells. Antioxid Redox Signal. 9:293–299. DOI: 10.1089/ars.2006.1467. PMID: 17184172.


31. Prieto J, García-Cañaveras JC, León M, et al. 2021; c-MYC triggers lipid remodelling during early somatic cell reprogramming to pluripotency. Stem Cell Rev Rep. 17:2245–2261. DOI: 10.1007/s12015-021-10239-2. PMID: 34476741. PMCID: PMC8599373.


32. Gu X, Ma Y, Liu Y, Wan Q. 2020; Measurement of mitochondrial respiration in adherent cells by Seahorse XF96 Cell Mito Stress Test. STAR Protoc. 2:100245. DOI: 10.1016/j.xpro.2020.100245. PMID: 33458707. PMCID: PMC7797920.


33. Zhang J, Nuebel E, Wisidagama DR, et al. 2012; Measuring energy metabolism in cultured cells, including human pluripotent stem cells and differentiated cells. Nat Protoc. 7:1068–1085. DOI: 10.1038/nprot.2012.048. PMID: 22576106. PMCID: PMC3819135.


34. Ruas JS, Siqueira-Santos ES, Amigo I, Rodrigues-Silva E, Kowaltowski AJ, Castilho RF. 2016; Underestimation of the maximal capacity of the mitochondrial electron transport system in oligomycin-treated cells. PLoS One. 11:e0150967. DOI: 10.1371/journal.pone.0150967. PMID: 26950698. PMCID: PMC4780810.


35. Plitzko B, Loesgen S. 2018; Measurement of oxygen consumption rate (OCR) and extracellular acidification rate (ECAR) in culture cells for assessment of the energy metabolism. Bio Protoc. 8:e2850. DOI: 10.21769/BioProtoc.2850. PMID: 34285967. PMCID: PMC8275291.


36. Schmidt CA, Fisher-Wellman KH, Neufer PD. 2021; From OCR and ECAR to energy: perspectives on the design and interpretation of bioenergetics studies. J Biol Chem. 297:101140. DOI: 10.1016/j.jbc.2021.101140. PMID: 34461088. PMCID: PMC8479256.


37. Kim HK, Ha TW, Lee MR. 2021; Single-cell transcriptome analysis as a promising tool to study pluripotent stem cell reprogramming. Int J Mol Sci. 22:5988. DOI: 10.3390/ijms22115988. PMID: 34206025. PMCID: PMC8198005.


38. Zhang C, Skamagki M, Liu Z, et al. 2017; Biological significance of the suppression of oxidative phosphorylation in induced pluripotent stem cells. Cell Rep. 21:2058–2065. DOI: 10.1016/j.celrep.2017.10.098. PMID: 29166598. PMCID: PMC5841608.


39. Yu L, Ji KY, Zhang J, et al. 2019; Core pluripotency factors promote glycolysis of human embryonic stem cells by activating GLUT1 enhancer. Protein Cell. 10:668–680. DOI: 10.1007/s13238-019-0637-9. PMID: 31152430. PMCID: PMC6711954.


40. Cacchiarelli D, Trapnell C, Ziller MJ, et al. 2015; Integrative analyses of human reprogramming reveal dynamic nature of induced pluripotency. Cell. 162:412–424. DOI: 10.1016/j.cell.2015.06.016. PMID: 26186193. PMCID: PMC4511597.


Fig. 1
Optimization of cell number is essential for the Seahorse cell metabolic assay. (A) Standard curves of oxygen consumption rate (OCR) and extracellular acidification rate (ECAR) according to cell number. (B) The appropriate number of cells is represented by a constant OCR/ECAR ratio. iPSC: induced pluripotent stem cell.
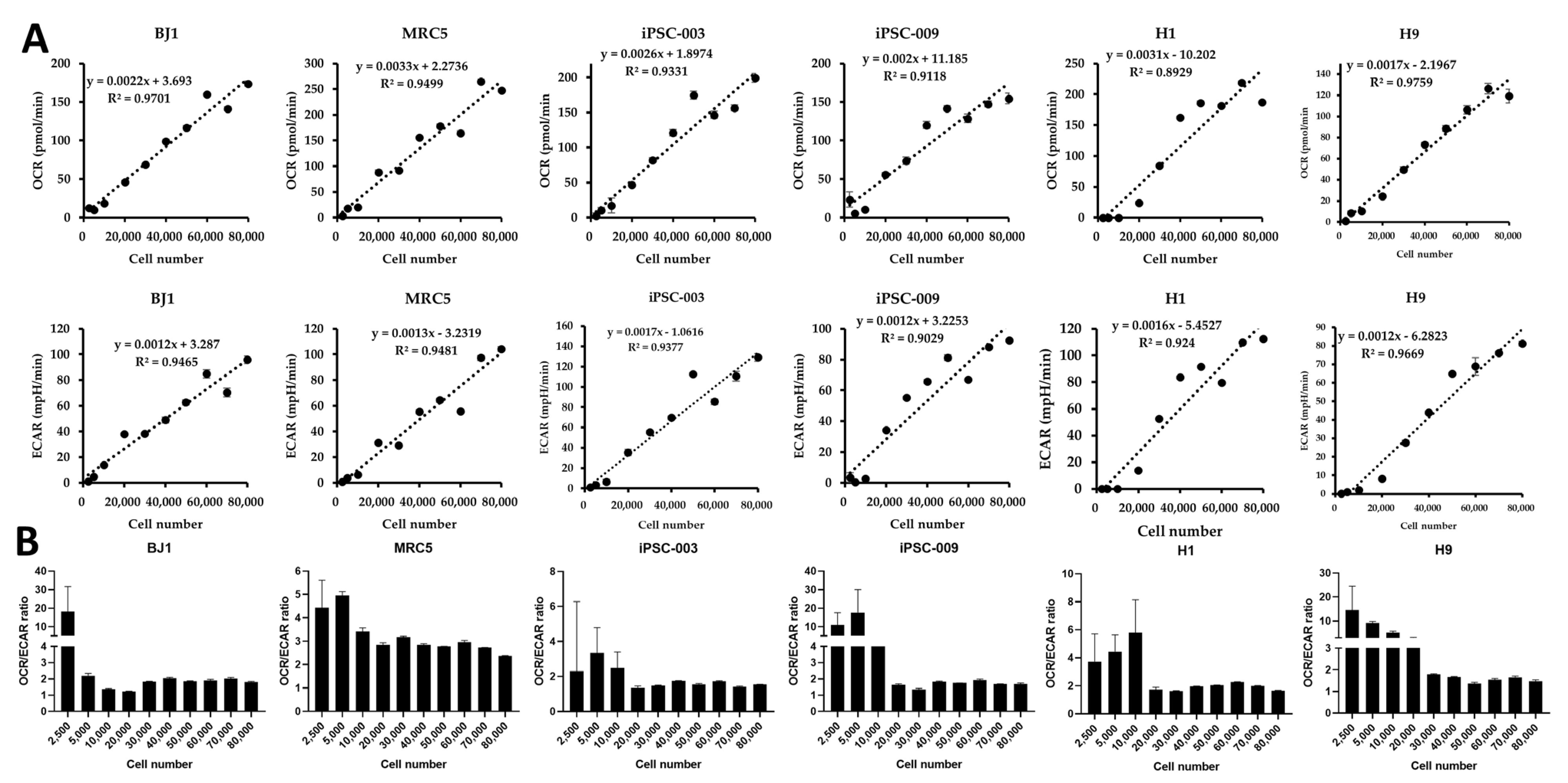
Fig. 2
Determination of the appropriate starvation period. (A) Oxygen consumption rate (OCR) response to glucose after glucose starvation. (B) Extracellular acidification rate (ECAR) response to glucose after glucose starvation. (C) Changes in OCR and ECAR responses to glucose in different cell types after glucose starvation. Student’s t-test: *p<0.05. iPSCs: induced pluripotent stem cells, hESCs: human embryonic stem cells.
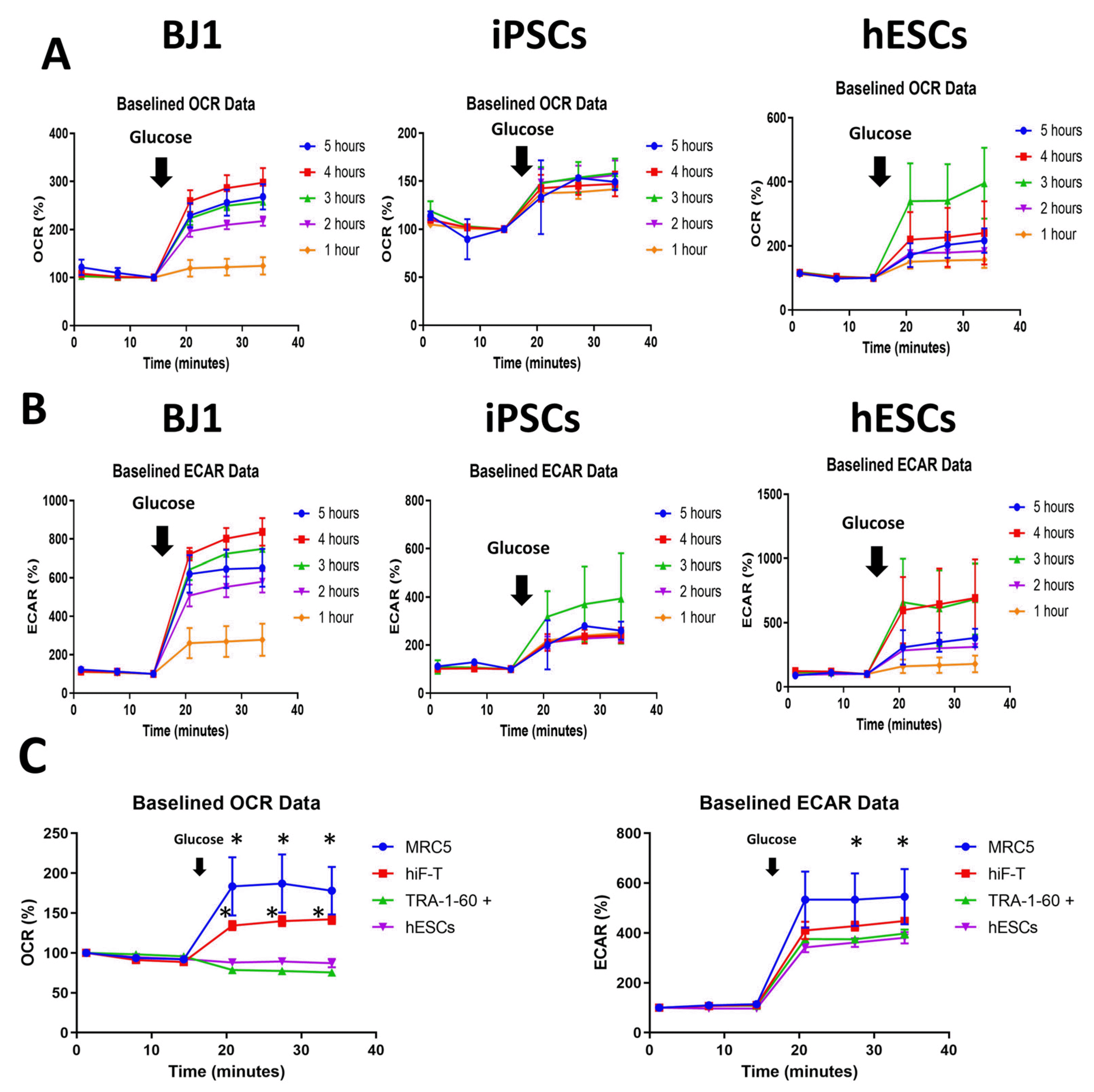
Fig. 3
Linear regression of total protein concentration and cellular metabolism according to cell number. (A) Differences in size between fibroblasts (MRC5 and hiF-T) and pluripotent stem cells (TRA-1-60 + and human embryonic stem cells [hESCs]) (hiF-T-derived TRA-1-60 + and hESCs. (B) Linear regression of protein concentration according to cell type. (C) Linear regression of oxygen consumption rate (OCR) and extracellular acidification rate (ECAR) according to cell number.
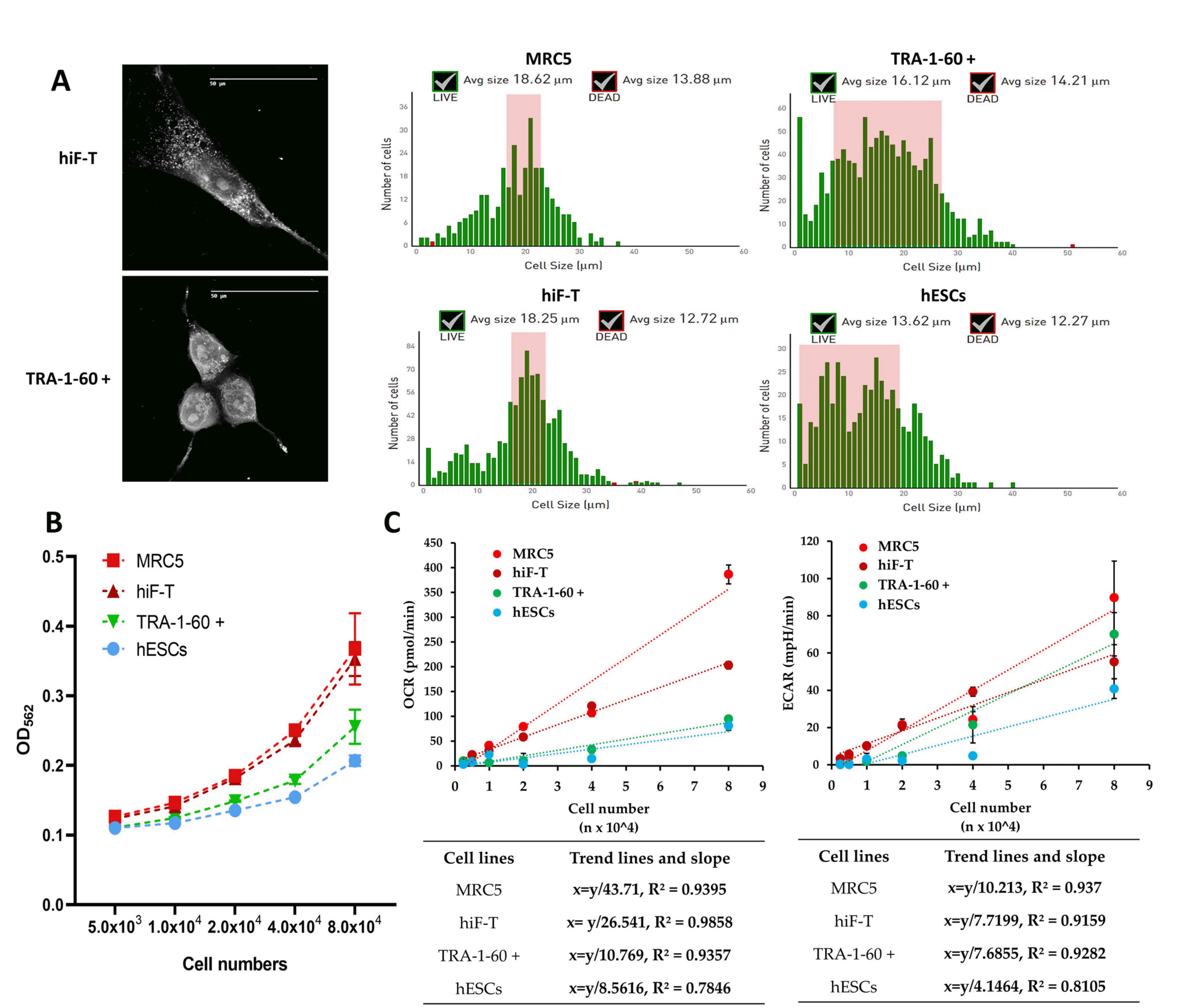
Fig. 4
Differences in the measurement of metabolic levels according to normalization method. (A) Real-time adenosine triphosphate (ATP) rate assay of somatic and pluripotent stem cells. (B) Non-normalized ATP production rate (pmol/min). (C) Normalized ATP production rate (pmol/min) by total protein, oxygen consumption rate (OCR), and extracellular acidification rate (ECAR). Student’s t-test: *p<0.05. R/A: rotenone & antimycin A, hESCs: human embryonic stem cells.
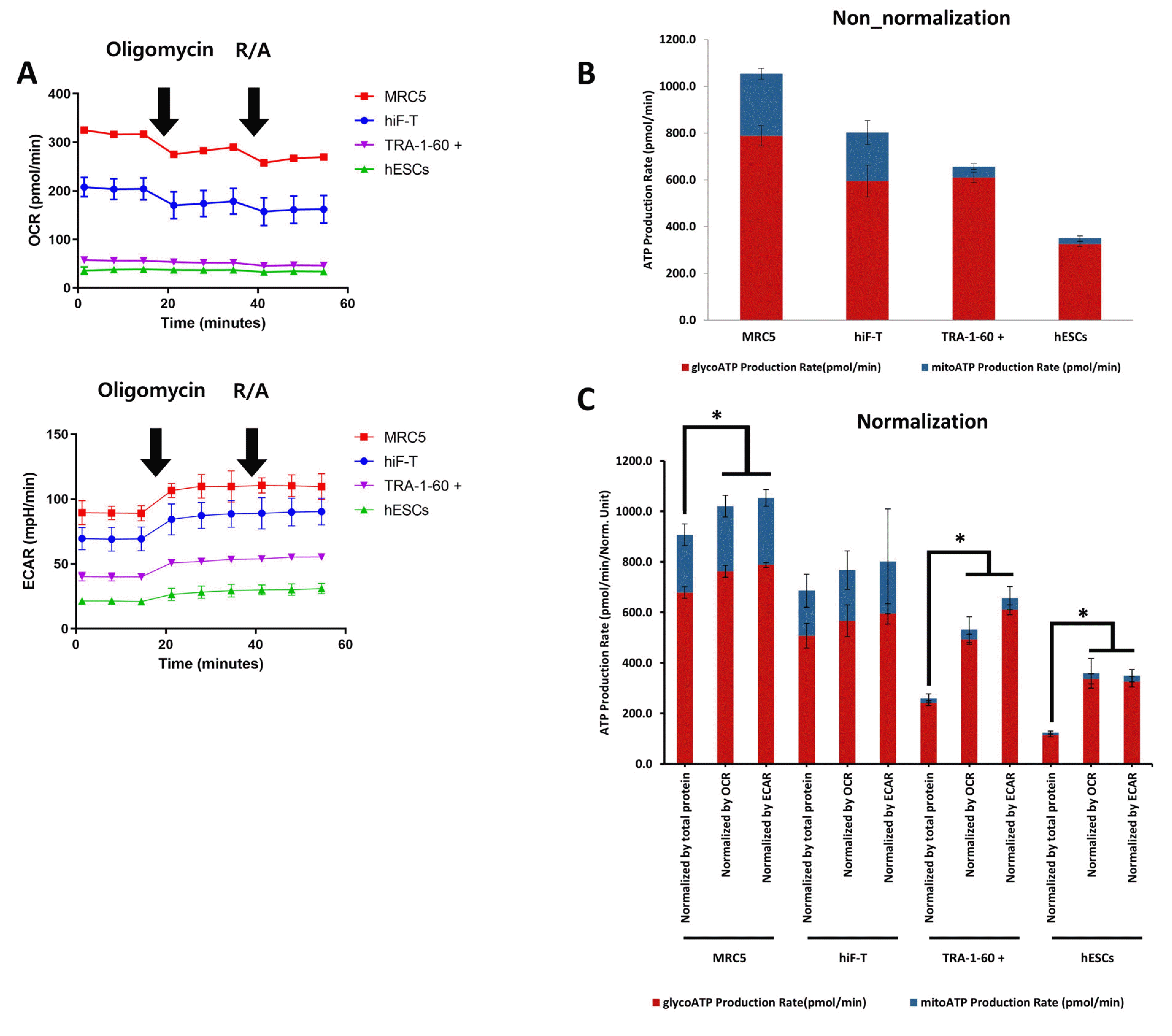