Abstract
Objective
Decompressive craniectomy (DC) with duroplasty is one of the common surgical treatments for life-threatening increased intracranial pressure (ICP). Once ICP is controlled, cranioplasty (CP) with reinsertion of the cryopreserved autologous bone flap or a synthetic implant is considered for protection and esthetics. Although with the risk of autologous bone flap resorption (BFR), cryopreserved autologous bone flap for CP is one of the important material due to its cost effectiveness. In this article, we performed conventional statistical analysis and the machine learning technique understand the risk factors for BFR.
Methods
Patients aged >18 years who underwent autologous bone CP between January 2015 and December 2021 were reviewed. Demographic data, medical records, and volumetric measurements of the autologous bone flap volume from 94 patients were collected. BFR was defined with absolute quantitative method (BFR-A) and relative quantitative method (BFR%). Conventional statistical analysis and random forest with hyper-ensemble approach (RF with HEA) was performed. And overlapped partial dependence plots (PDP) were generated.
Results
Conventional statistical analysis showed that only the initial autologous bone flap volume was statistically significant on BFR-A. RF with HEA showed that the initial autologous bone flap volume, interval between DC and CP, and bone quality were the factors with most contribution to BFR-A, while, trauma, bone quality, and initial autologous bone flap volume were the factors with most contribution to BFR%. Overlapped PDPs of the initial autologous bone flap volume on the BRF-A crossed at approximately 60 mL, and a relatively clear separation was found between the non-BFR and BFR groups. Therefore, the initial autologous bone flap of over 60 mL could be a possible risk factor for BFR.
Conclusion
From the present study, BFR in patients who underwent CP with autologous bone flap might be inevitable. However, the degree of BFR may differ from one to another. Therefore, considering artificial bone flaps as implants for patients with large DC could be reasonable. Still, the risk factors for BFR are not clearly understood. Therefore, chronological analysis and pathophysiologic studies are needed.
Decompressive craniectomy (DC) with duroplasty is one of the common surgical treatments for life-threatening increased intracranial pressure (ICP) in patients with traumatic brain injury and stroke [2,42,47,48,57]. Once ICP is controlled, cranioplasty (CP) with reinsertion of the cryopreserved autologous bone flap or a synthetic implant is considered for protection and esthetics. The use of the elaborately crafted patient-specific synthetic implants for CP with various materials such as polyetheretherketone (PEEK) or titanium alloy is becoming popular recently, and the results are promising [14,23,28,31,41,49,53,59-61]. However, the reinsertion of the cryopreserved autologous bone flap is still a commonly used method for its cost-effectiveness. Although most CPs with cryopreserved autologous bone flap is performed without incidence, complications including surgical site infections and bone flap resorption (BFR) remains [10,12,19,29,35,45]. In this article, we performed conventional statistical analysis and the machine learning technique understand the risk factors for BFR.
This retrospective study was approved by the Institutional Review Board before data collection (2021-07-024), and the need for patient consent was waived by the relevant ethics board because of the retrospective study design.
In this study, data of patients aged >18 years who underwent autologous bone CP between January 2015 and December 2021 were reviewed. Medical records and images of 202 patients were reviewed. Patients who had multiple follow up brain computed tomography (CT) images, which are adequate for three-dimensional (3D) reconstruction, and were followed for at least 6 months after autologous bone CP were included. Therefore, demographic data, medical records, and volumetric measurements of the autologous bone flap volume from 94 patients were collected. CT scans with abnormally storage Digital Imaging and Communications in Medicine (DICOM) data were excluded from this study.
Once bone flaps were obtained during DC, soft tissues were meticulously removed, packed with two sterile layers, sealed in a sterile Ziploc bag, and then stored at -80°C without the application of local antibiotics or autoclaving. Only large fragments were preserved without fixation. Fragments or fractures were fixed during CP.
Our institute has used 1-mm slice bone setting images in default brain CT protocol since 2015. We used the first postoperative CT scan as the control point and the following CT scans for the BFR analysis. DICOM data of the brain CT was exported to generate a 3D model of the autologous bone flap of the CP using open-source 3D Slicer version 4.10.2 (http://www.slicer.org; Isomics, Inc, Cambridge, MA, USA). The same threshold level was used for each patient to render the whole skull 3D model and the autologous bone flap was precisely segmented using editing tools. Extracted bone flaps from each CT scans were exported in stereolithography file format and then imported to open-source Meshlab version 2016 (Visual Computing Lab; ISTI - CNR, Pisa, Italy) for volume measurement. If the 3D model of the autologous bone flap cannot be trimmed clearly from the 3D slicer, Meshmixer version 3.5 (http://www.meshmixer.com; Autodesk, Inc., San Rafael, CA, USA) was used for additional modification of the autologous bone flap 3D models.
As no standard method of defining BFR has been established for quantitative analysis, BFR was defined in two criteria : absolute quantitative method (BFR-A) and relative quantitative method (BFR%). BFR-A and BFR% are calculated using the following equation :
BFR-A = volume at first postoperative CT − volume at last CT
BFR% = (volume at first postoperative CT − volume at last CT)/volume at first postoperative CT [4].
For the statistical analysis and machine learning analysis, a positive BFR was defined as BFR-A is >20 mL or the BFR% is >30% which were approximately 20% of the dataset.
Hounsfield unit (HU) values were measured from the frontal bone of the non-CP site to estimate the current status of bone quality [36].
The Pearson test, Spearman rho test, and univariate and multivariate logistic regression analyses were employed in the risk factor analysis using R version 4.1.2 (R Project for Statistical Computing, Vienna, Austria). A p-value of <0.05 and 95% confidence intervals were considered statistically significant. The chi-squared test, Spearman rho test, and univariate and multivariate regression analyses were used to determine the correlation between risk factors and BFR.
Machine-learning analysis using RF with HEA was employed to analyze the contribution of each variable to BFR [11,39,46].
Step 1 : generating multiple balanced datasets. Multiple balanced datasets were generated from the original dataset. A hybrid random sampling technique using the ROSE package was utilized to generate multiple balanced datasets from the original [33]. A total of 500 balanced datasets were generated for RF training using 500 randomly generated seed numbers.
Step 2 : RF training (ensemble methods). The RF algorithm was performed with R software (R version 4.1.2; R Project for Statistical Computing) using the randomForest package [32,56]. Each balanced dataset was subjected to RF training by building 50000 decision trees. Approximately 70% of observations were selected randomly with replacement (bootstrap aggregation; bagging) for RF training, and the remaining observations (out of bag [OOB]) were used as test datasets for the evaluation of the RF models [8]. Four randomly selected variables were used to split each node [8]. In handling, absent data were not filled with the na.roughfix function from the randomForest package because menopause should remain absent in male patients. The mean decrease accuracy (MDA) and mean decrease Gini (MDG) were obtained to assess the importance of variables. The mean and standard deviation of the OOB error rate, area under the receiver operating characteristic curve (AUC), accuracy, sensitivity, and specificity were also calculated to validate the 500 RF models.
Step 3 : HEA. The results from 500 RF models were combined with the HEA (ensemble of ensembles) [11,39,46]. The mean and standard deviation of the OOB error rate, AUC, accuracy, sensitivity, and specificity were calculated from 500 RF models [11]. Variable importance was visualized with box plots of MDAs and MDGs to present various ranges of results exported from multiple RF models [11].
Among 94 patients, approximately 20% were classified as having positive BFR in both definitions BFR-A and BFR%. Despite the definition of BFR, there was no difference between the non-BFR group and the BFR group in demographics and medical histories. In BFR-A, the initial autologous bone flap volume was statistically significant and correlated with BFR. However, no other risk factors showed statistical significance on both BFR definitions (Table 1). Univariate and multivariate regression analyses also failed to show any statistical significance. The Cohen’s kappa between BFR-A and BFR % was 0.86.
The relative volume of the autologous bone flap was visualized with a spaghetti plot (Fig. 1). The volume of the autologous bone flap decreased over time.
The OBB error rate of each RF model on both BFR-A and BFR% was mostly stabilized within the first 20000 decision trees. The hyper-ensembled OOB error rates on BFR-A and BFR% were 13.44%±5.64% and 10.19%±3.99%, and the AUCs were 0.86±0.06 and 0.89±0.04, respectively. The mean accuracy, sensitivity, and specificity of the BFR-A were 0.88±0.11, 0.91±0.16, and 0.84±0.20 and those of BFR% were 0.90±0.09, 0.94±0.11, and 0.86±0.15, respectively.
The box plot shows that the individual RF models show various ranges in both MDA and MDG. The initial autologous bone flap volume, interval between DC and CP, and bone quality were the factors with most contribution to BFRA prediction according to both MDA and MDG. According to the MDA, trauma, bone quality, and initial autologous bone flap volume were the factors with most contribution to BFR% prediction. However, the ranking of importance of BFR% according to MDG differed with MDA, and trauma dropped to third place (Fig. 2).
Overlapped PDPs of the initial autologous bone flap volume on the BRF-A crossed at approximately 60 mL, and a relatively clear separation was found between the non-BFR and BFR groups. Therefore, the initial autologous bone flap of over 60 mL could be a possible risk factor for BFR. Most PDPs of the interval between DC and CP on BFR-A showed crossing of the predicted probability in approximately 60 days; however, results of PDPs compared with that of the initial autologous bone flap volume were debated. PDPs of bone quality, age, and Glasgow coma scale (GCS) also showed debated results on predicting BFR. Regarding BFR%, none of the variables showed a clear separation between the non-BFR and BFR groups.
CP aims to restore cerebral protection and cosmesis. Benefits reported included the improvement of cerebral perfusion, cerebrospinal fluid dynamics, and neurological function and the relief of the syndrome of the trephined [3,24,34,37,50,58]. Autologous bone flaps are widely used for CP for various reasons such as price competitiveness, biocompatibility, strength, and ideal contour [15,28]. Despite its advantages, the use of autologous bone flaps is at risk of BFR, which is reported in 1.96–22.8% of adult patients and 50% of pediatric patients [16]. Autologous bone flap requires revascularization, osteoconduction, osteoinduction, and osteogenesis for reintegration [20-22,29]. However, autologous bone flaps are devascularized during the harvesting process in DC; therefore, it has limited revascularization ability after CP. The fatty bone marrow also acts as a barrier for osteoblasts; therefore, increased signs of osteoclastic resorption became prominent [21]. In the present study, a large portion of the autologous bone flap had gradual volume loss (Fig. 1). A previous study also showed similar results, that is, the decrease in the autologous bone flap volume is a very common phenomenon, which is observed in up to 90% of patients who underwent CP [4,29].
The pathophysiology indicates that BFR is inevitable with only differences in the extent, and no study has provided a generalized definition of BFR [4,13,16,25,29,38,43] because defining BFR as an autologous bone flap that needs reinforcement or replacement is subjective and the indication of CP re-operation differed among clinicians. Therefore, this study used two criteria for BFR using an absolute quantitative method (BFR-A) and a relative quantitative method (BFR%). BFR-A shows that the amount of BFR corresponded to the autologous bone flap.
Suggested risk factors for BFR include sex, age, interval time between DC and CP, presence of systemic factors, autologous bone flap size, number of bone fragments, and shunt operation [5,7,9,22,38,40,44,45,51]. As much clinical data were collected from the cohort; however, in the conventional statistical analysis of risk factors for BFR, only the initial autologous bone flap volume from BFR-A was found to be significant. For a better understanding of BFR, RF with HEA was applied [7,11].
A decision tree is a classification algorithm that was used to generate and ideally classify the target in the present study, BFR. Generally, only a single decision tree can be derived from a single dataset. RF is an algorithm that assembles the results from multiple decision trees [8]. To generate multiple datasets, it uses bootstrap aggregation (bagging), which is randomly selecting observations (clinical cases) with replacements. In building a decision tree, randomly selected variables are applied to each node, that is, each decision tree gets unique features. This randomness gives RF flexibility and robustness in dealing with high-dimensional data and reduces overfitting [8]. Being a simple method, it is fast compared with other machine-learning methods. RF with HEA takes one step forward by assembling the results of multiple RFs (ensemble of ensembles). This method was first developed to analyze rare genetic variants, and recently, it is used in various clinical studies [1,11,46,52]. RF with HEA minimizes the human error from a single RF, and the range of results from multiple RFs can reveal the tendency of the effect of variables on the target [11]. For easier understanding, multiple studies with the same study design can show various possibilities by generating multiple dataset combinations. As all analyses are based on randomness, results are closer to general expectations presented in multiple studies.
The box plot of the MDA and MDG visualizes the effect of variables on BFR prediction from the RF with HEA. The initial autologous bone flap volume was the variable with the most influence on BFR-A prediction, followed by the interval between DC and CP, bone quality, age, and GCS. In BFR%, trauma as the reason for DC had the most influence, followed by bone quality, initial autologous bone flap volume, interval between DC and CP, and age. In the comparison of box plots of BFR-A and BFR%, the influence between variables in BFR-A was prominent compared with that in BFR%. In BFR%, the top-ranking variable from the MDA was discordant with that of MDG, whereas the ranks in both MDA and MDG from BFR-A were constant. This implies that the results of RF with HEA according to the definition of BFR-A in more reliable.
Overlapped PDPs from the top five variables for RF with HEA of BFR-A and BFR% were obtained to reveal how each variable affects BFR. Each pair of PDP plots represents the influence of the variables on BFR in a single RF, and the overlapped 500 pairs of PDPs provide an overview of the overall RF results. Moreover, each pair of PDPs is separated, and the variable is more capable of classifying BFR. Among overlapped PDPs, the initial autologous bone flap in BFR-A shows the most significant separation. A bone flap over 60 mL is a break-even point in predicting BFR. Other than the initial autologous bone flap volume from the BFR-A, the interval between DC and CP in the BFR-A is relatively less controversial than the rest of the overlapped PDPs. According to the results of RF with HEA, the initial autologous bone flap volume and interval between DC and CP are possible risk factors according to the definition of BFR-A.
As mentioned above, the initial autologous bone flap volume was statistically significant and pointed out as the most potent risk factor in predicting BFR-A (Table 1). Overlapped PDPs in the initial autologous bone flap volume in the BFR-A showed that the larger bone flap is a potential risk factor of BFR (Fig. 3). The influence of the initial autologous bone flap volume is dampened in the definition of BFR% as it is leveled. Therefore, the initial autologous bone flap volume failed to show statistical significance in BFR%; however, it still ranked third in the BFR% from the results of RF with HEA (Fig. 3). This result may represent that the autologous bone flap size is a strong risk factor. Previous studies also showed that larger bone flap is a risk factor of BFR [22,27,35]. Unfortunately, the main goal of DC is to obtain as much space as possible by removing the bone flap and performing duroplasty. Therefore, reducing the size of the autologous bone flap to prevent BFR is rarely feasible [25].
Some previous studies have proposed that cryopreservation of the autologous bone flap impairs viability and graft revascularization, which leads to BFR [6,27,40,54,55]. The present study also shows similar results from BFR-A. According to the overlapped PDP of the interval between DC and CP in BFR-A, CP after 3 months is expected to be a risk factor for BFR, whereas CP within 2 months shows protective effects on BFR (Fig. 3). Still, some aspects of the RF show debated results, and the results of the overlapped PDPs should be carefully interpreted. However, some studies have shown that prolonged cryopreservation does not affect BFR [26].
BFR in pediatric patients is a well-known risk factor and is reported in up to 50% of cases [7,16,30,35]. According to the idea that BFR is caused by an imbalance between bone formation and bone resorption, we assumed that younger age and osteoporosis could be risk factors for BFR. With further assumption, as menopause is one of the risk factors of osteoporosis, it was also included as a potential risk factor in the present study. During the medical record review, age and menopause data were available; however, owing to the retrospective design, the majority of the patients have not undergone osteoporosis study. Instead gathering osteoporosis data, the skull bone was measured in HUs, as it is a potentially opportunistic osteoporosis screening test [36]. However, unlike our expectations, age, menopause, and bone quality were not statistically significant. In RF with HEA, bone quality and age were among the top five variables in according to MDA and MDG in both definitions (Fig. 2). Overlapped PDPs could not reveal how the variables affect BFR because mixed results are shown (Fig. 3).
In previous studies, trauma and the number of bone fragments were pointed out to be risk factors for BFR [7,17,51]. In the present study, whether trauma is a risk factor of BFR is quite unclear, particularly on the results of the RF with HEA. Trauma only appears as a risk factor with strong effects on BFR% (Fig. 2). However, the ranking of trauma reverses on MDA and MDG. The boxplot also shows that the RF with HEA had a wide range of results compared with other top-ranked risk factors. Owing to the binary nature of variables, overlapped PDPs should be more clearly separated, if the results of the RF with HEA are consistent. However, the overlapped PDPs of trauma show mixed results, and the explanation of the effect of trauma on BFR is unclear (Fig. 3).
Although quantitative methods were applied to determine BFR objectively, few limitations were noted. First, owing to the retrospective design of the study, follow-up intervals and period were not unified. Therefore, chronologic analyses of BFR were unavailable. The annual decrease rate to define BFR was also considered, and the annual decrease rate was not constant throughout time; thus, the annual decrease rate calculated from the large gap between CT scans may not be reliable. To understand the chronologic characteristics of BFR, a well-designed follow-up CT protocol is necessary. Second, the quality of CT could be different. Our institute used the same CT protocol with the same CT machines since 2015. However, two of the volumetric data showed alias data, which are sudden increments of the autologous bone flap volume >200% and dropped to a similar volume compared with the initial autologous volume. We could not find the reason for these phenomena; however, the only plausible explanation is the error during exporting of data to the DICOM server. As the volumetric data might not represent 100% exact volume of the autologous bone flap, we gave a sufficient margin of difference in defining BFR. Third, in the present study, the definitions of BFR in the clinical field are not the same. In the clinical field, determining BFR is necessary to screen patients who underwent CP and need reinforcement or replacement of the autologous bone flap. However, bone volume loss does not mean that the strength of the autologous bone f lap decreased. Therefore, the opinion of the clinicians could intervene on proceeding with additional surgical procedures. Although the clinical significance was unsatisfactory, the volumetric method was chosen to subjectively analyze the risk factors for BFR. Finally, the use of RF with HEA could be questionable, that is, whether it is the most suitable machine-learning technique. A previous study using RF with HEA showed a good explanation of the risk factors in a small cohort [11]. As RF with HEA implies randomness from multistage during the analysis, human alternation was nearly absent, which could affect the results [11]. RF with HEA also has an advantage over support vector machine and neural network on interpretation of its results [11]. The results of the RF with HEA do not represent the causality of the variable to the target, however, overlapped PDPs visualize the tendency of the effects of variables on the target. The results of RF with HEA could be straightforwardly interpreted if the overlapped PDPs are separated clearly. In current study, most of the results of the RF with HEA showed imperfect separation of the overlapped PDPs; therefore, we can only determine which variable is more potent in causing BFR but cannot determine how it influences BFR.
From the present study, BFR in patients who underwent CP with autologous bone flap might be inevitable. However, the degree of BFR may differ from one to another. Currently, personalized artificial bone flaps with PEEK or titanium alloy are widely available. Considering artificial bone flaps as implants for patients with large DC could be reasonable. Still, the risk factors for BFR are not clearly understood. Therefore, chronological analysis and pathophysiologic studies are needed.
Notes
ACKNOWLEDGMENTS
The present research was supported by the research fund of Dankook University in 2021.
References
1. Aguilaniu B, Hess D, Kelkel E, Briault A, Destors M, Boutros J, et al. A machine learning approach to predict extreme inactivity in COPD patients using non-activity-related clinical data. PLoS One. 16:e0255977. 2021.


2. Anderson J. Decompressive craniectomy in diffuse traumatic brain Injury : Cooper DJ, Rosenfeld JV, Murray L, et al. N Engl J Med 2011; 364: 1493-502. J Emerg Med. 41:450. 2011.
3. Ashayeri K, Jackson EM, Huang J, Brem H, Gordon CR. Syndrome of the trephined: a systematic review. Neurosurgery. 79:525–534. 2016.
4. Barzaghi LR, Parisi V, Gigliotti CR, Giudice L, Snider S, Dell’Acqua A, et al. Bone resorption in autologous cryopreserved cranioplasty: quantitative evaluation, semiquantitative score and clinical significance. Acta Neurochir (Wien). 161:483–491. 2019.


5. Beez T, Munoz-Bendix C, Ahmadi SA, Steiger HJ, Beseoglu K. From decompressive craniectomy to cranioplasty and beyond-a pediatric neurosurgery perspective. Childs Nerv Syst. 35:1517–1524. 2019.


6. Bhaskar IP, Yusheng L, Zheng M, Lee GY. Autogenous skull flaps stored frozen for more than 6 months: do they remain viable? J Clin Neurosci. 18:1690–1693. 2011.


7. Bowers CA, Riva-Cambrin J, Hertzler DA 2nd, Walker ML. Risk factors and rates of bone flap resorption in pediatric patients after decompressive craniectomy for traumatic brain injury. J Neurosurg Pediatr. 11:526–532. 2013.


8. Breiman L. Random forests. Mach Learn. 45:5–32. 2001.
9. Brommeland T, Rydning PN, Pripp AH, Helseth E. Cranioplasty complications and risk factors associated with bone flap resorption. Scand J Trauma Resusc Emerg Med. 23:75. 2015.


10. Broughton E, Pobereskin L, Whitfield PC. Seven years of cranioplasty in a regional neurosurgical centre. Br J Neurosurg. 28:34–39. 2014.


11. Chung J, Cheong JH, Kim JM, Lee DH, Yi HJ, Choi KS, et al. Is fetal-type posterior cerebral artery a risk factor for recurrence in coiled internal carotid artery-incorporating posterior communicating artery aneurysms? Analysis of conventional statistics, computational fluid dynamics, and random forest with hyper-ensemble approach. Neurosurgery. 93:611–621. 2023.


12. Coulter IC, Pesic-Smith JD, Cato-Addison WB, Khan SA, Thompson D, Jenkins AJ, et al. Routine but risky: a multi-centre analysis of the outcomes of cranioplasty in the Northeast of England. Acta Neurochir (Wien). 156:1361–1368. 2014.


13. da Costa Benalia VH, Pedrozo CAG, Kormanski MK, Veiga JCE, de Aguiar GB. Spontaneous bone flap resorption following cranioplasty using autologous bone. J Craniofac Surg. 32:293–296. 2021.


14. Dabadi S, Dhungel RR, Sharma U, Shrestha D, Gurung P, Shrestha R, et al. Customized cost-effective polymethyl-methacrylate cranioplasty implant using three-dimensional printer. Asian J Neurosurg. 16:150–154. 2021.


15. Dowlati E, Pasko KBD, Molina EA, Felbaum DR, Mason RB, Mai JC, et al. Decompressive hemicraniectomy and cranioplasty using subcutaneously preserved autologous bone flaps versus synthetic implants: perioperative outcomes and cost analysis. J Neurosurg. 137:1831–1838. 2022.


16. Ernst G, Qeadan F, Carlson AP. Subcutaneous bone flap storage after emergency craniectomy: cost-effectiveness and rate of resorption. J Neurosurg. 129:1604–1610. 2018.


17. Frassanito P, Massimi L, Caldarelli M, Tamburrini G, Di Rocco C. Complications of delayed cranial repair after decompressive craniectomy in children less than 1 year old. Acta Neurochir (Wien). 154:927–933. 2012.


18. Friedman JH. Greedy function approximation: a gradient boosting machine. Ann Stat. 29:1189–1232. 2001.


19. Gooch MR, Gin GE, Kenning TJ, German JW. Complications of cranioplasty following decompressive craniectomy: analysis of 62 cases. Neurosurg Focus. 26:E9. 2009.


20. Gosain AK, Gosain SA, Sweeney WM, Song LS, Amarante MTJ. Regulation of osteogenesis and survival within bone grafts to the calvaria: the effect of the dura versus the pericranium. Plast Reconstr Surg. 128:85–94. 2011.


21. Göttsche J, Mende KC, Schram A, Westphal M, Amling M, Regelsberger J, et al. Cranial bone flap resorption-pathological features and their implications for clinical treatment. Neurosurg Rev. 44:2253–2260. 2021.


22. Grant GA, Jolley M, Ellenbogen RG, Roberts TS, Gruss JR, Loeser JD. Failure of autologous bone-assisted cranioplasty following decompressive craniectomy in children and adolescents. J Neurosurg. 100(2 Suppl Pediatrics):163–168. 2004.


23. Gupta D. Novel solutions to cranioplasty: from exchange cranioplasty to synthetic patient-specific implants. Neurol India. 69:618–619. 2021.


24. Halani SH, Chu JK, Malcolm JG, Rindler RS, Allen JW, Grossberg JA, et al. Effects of cranioplasty on cerebral blood flow following decompressive craniectomy: a systematic review of the literature. Neurosurgery. 81:204–216. 2017.


25. Hersh DS, Anderson HJ, Woodworth GF, Martin JE, Khan YM. Bone flap resorption in pediatric patients following autologous cranioplasty. Oper Neurosurg (Hagerstown). 20:436–443. 2021.


26. Iwama T, Yamada J, Imai S, Shinoda J, Funakoshi T, Sakai N. The use of frozen autogenous bone flaps in delayed cranioplasty revisited. Neurosurgery. 52:591–596. discussion 595-596. 2003.


27. Kim JH, Kim JH, Kwon TH, Chong K, Hwang SY, Yoon WK. Aseptic bone flap resorption after cranioplasty with autologous bone: incidence, risk factors, and clinical implications. World Neurosurg. 115:e111–e118. 2018.


28. Kim JK, Lee SB, Yang SY. Cranioplasty using autologous bone versus porous polyethylene versus custom-made titanium mesh : a retrospective review of 108 patients. J Korean Neurosurg Soc. 61:737–746. 2018.


29. Korhonen TK, Salokorpi N, Niinimäki J, Serlo W, Lehenkari P, Tetri S. Quantitative and qualitative analysis of bone flap resorption in patients undergoing cranioplasty after decompressive craniectomy. J Neurosurg. 130:312–321. 2018.


30. Korhonen TK, Tetri S, Huttunen J, Lindgren A, Piitulainen JM, Serlo W, et al. Predictors of primary autograft cranioplasty survival and resorption after craniectomy. J Neurosurg. 130:1672–1679. 2018.


31. Li J, von Campe G, Pepe A, Gsaxner C, Wang E, Chen X, et al. Automatic skull defect restoration and cranial implant generation for cranioplasty. Med Image Anal. 73:102171. 2021.


32. Liaw A, Wiener M. Classification and regression by randomForest. R News. 2:18–22. 2002.
33. Lunardon N, Menardi G, Torelli N. ROSE: a package for binary imbalanced learning. R J. 6:79–89. 2014.


34. Malcolm JG, Rindler RS, Chu JK, Chokshi F, Grossberg JA, Pradilla G, et al. Early cranioplasty is associated with greater neurological improvement: a systematic review and meta-analysis. Neurosurgery. 82:278–288. 2018.


35. Martin KD, Franz B, Kirsch M, Polanski W, von der Hagen M, Schackert G, et al. Autologous bone flap cranioplasty following decompressive craniectomy is combined with a high complication rate in pediatric traumatic brain injury patients. Acta Neurochir (Wien). 156:813–824. 2014.


36. Na MK, Won YD, Kim CH, Kim JM, Cheong JH, Ryu JI, et al. Opportunistic osteoporosis screening via the measurement of frontal skull Hounsfield units derived from brain computed tomography images. PLoS One. 13:e0197336. 2018.


37. Parichay PJ, Khanapure K, Joshi KC, Aniruddha TJ, Sandhya M, Hegde AS. Clinical and radiological assessment of cerebral hemodynamics after cranioplasty for decompressive craniectomy - a clinical study. J Clin Neurosci. 42:97–101. 2017.


38. Park SP, Kim JH, Kang HI, Kim DR, Moon BG, Kim JS. Bone flap resorption following cranioplasty with autologous bone: quantitative measurement of bone flap resorption and predictive factors. J Korean Neurosurg Soc. 60:749–754. 2017.


39. Petrini A, Mesiti M, Schubach M, Frasca M, Danis D, Re M, et al. parSMURF, a high-performance computing tool for the genome-wide detection of pathogenic variants. GigaScience. 9:giaa052. 2020.


40. Piedra MP, Thompson EM, Selden NR, Ragel BT, Guillaume DJ. Optimal timing of autologous cranioplasty after decompressive craniectomy in children. J Neurosurg Pediatr. 10:268–272. 2012.


41. Piitulainen JM, Kauko T, Aitasalo KM, Vuorinen V, Vallittu PK, Posti JP. Outcomes of cranioplasty with synthetic materials and autologous bone grafts. World Neurosurg. 83:708–714. 2015.


42. Polin RS, Shaffrey ME, Bogaev CA, Tisdale N, Germanson T, Bocchicchio B, et al. Decompressive bifrontal craniectomy in the treatment of severe refractory posttraumatic cerebral edema. Neurosurgery. 41:84–94. discussion 92-94. 1997.


43. Rashidi A, Sandalcioglu IE, Luchtmann M. Aseptic bone-flap resorption after cranioplasty - incidence and risk factors. PLoS One. 15:e0228009. 2020.


44. Rocque BG, Agee BS, Thompson EM, Piedra M, Baird LC, Selden NR, et al. Complications following pediatric cranioplasty after decompressive craniectomy: a multicenter retrospective study. J Neurosurg Pediatr. 22:225–232. 2018.


45. Schoekler B, Trummer M. Prediction parameters of bone flap resorption following cranioplasty with autologous bone. Clin Neurol Neurosurg. 120:64–67. 2014.


46. Schubach M, Re M, Robinson PN, Valentini G. Imbalance-aware machine learning for predicting rare and common disease-associated noncoding variants. Sci Rep. 7:2959. 2017.


47. Schwab S, Steiner T, Aschoff A, Schwarz S, Steiner HH, Jansen O, et al. Early hemicraniectomy in patients with complete middle cerebral artery infarction. Stroke. 29:1888–1893. 1998.


48. Scotter J, Iorga R, Stefanou D, Wilson MH. Management of malignant middle cerebral artery infarction following a cardiac stab wound--the role of early decompressive craniectomy. Br J Neurosurg. 28:534–535. 2014.


49. Shah AM, Jung H, Skirboll S. Materials used in cranioplasty: a history and analysis. Neurosurg Focus. 36:E19. 2014.


50. Shahid AH, Mohanty M, Singla N, Mittal BR, Gupta SK. The effect of cranioplasty following decompressive craniectomy on cerebral blood perfusion, neurological, and cognitive outcome. J Neurosurg. 128:229–235. 2018.


51. Signorelli F, Giordano M, Caccavella VM, Ioannoni E, Gelormini C, Caricato A, et al. A systematic review and meta-analysis of factors involved in bone flap resorption after decompressive craniectomy. Neurosurg Rev. 45:1915–1922. 2022.


52. Smedley D, Schubach M, Jacobsen JOB, Köhler S, Zemojtel T, Spielmann M, et al. A whole-genome analysis framework for effective identification of pathogenic regulatory variants in Mendelian disease. Am J Hum Genet. 99:595–606. 2016.


53. Sorek S, Miller A, Griepp D, Moawad S, Zanzerkia R, Rahme R. Skull reconstruction using a custom-made, three-dimensional-printed, hydroxyapatite-titanium cranioplasty implant: largest single-center U.S. experience. World Neurosurg. 167:e1387–e1394. 2022.


54. Stevenson S, Li XQ, Davy DT, Klein L, Goldberg VM. Critical biological determinants of incorporation of non-vascularized cortical bone grafts. Quantification of a complex process and structure. J Bone Joint Surg Am. 79:1–16. 1997.


55. Sultan SM, Davidson EH, Butala P, Schachar JS, Witek L, Szpalski C, et al. Interval cranioplasty: comparison of current standards. Plast Reconstr Surg. 127:1855–1864. 2011.


56. Team R Core. R: A language and environment for statistical computing. Available at : https://www.r-project.org/.
57. Vahedi K, Vicaut E, Mateo J, Kurtz A, Orabi M, Guichard JP, et al. Sequential-design, multicenter, randomized, controlled trial of early decompressive craniectomy in malignant middle cerebral artery infarction (DECIMAL trial). Stroke. 38:2506–2517. 2007.


58. Voss HU, Heier LA, Schiff ND. Multimodal imaging of recovery of functional networks associated with reversal of paradoxical herniation after cranioplasty. Clin Imaging. 35:253–258. 2011.


59. Yoon HG, Ko Y, Kim YS, Bak KH, Chun HJ, Na MK, et al. Efficacy of 3D-printed titanium mesh-type patient-specific implant for cranioplasty. Korean J Neurotrauma. 17:91–99. 2021.


60. Zanotti B, Zingaretti N, Verlicchi A, Robiony M, Alfieri A, Parodi PC. Cranioplasty: review of materials. J Craniofac Surg. 27:2061–2072. 2016.
Fig. 1.
Chronological presentation of relative autologous bone flap volume. The spaghetti plot autologous bone flap volume shows that most of the autologous bone flap volume decreases over time.
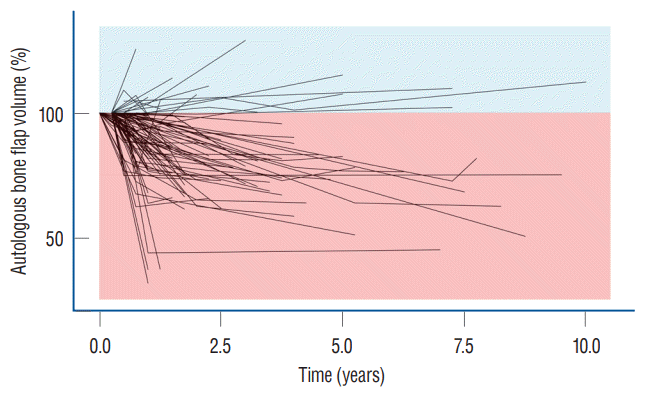
Fig. 2.
Importance of variable on impact of bone flap resorption (BFR). Importance of variable on impact of BFR is calculated with MDA (A and B) and MDG (C and D). Initial bone flap volume and interval between DC and CP was the most impact variable in BFR-A (A and C). Top ranked variables in MDA and MDG were not constant in BFR%. MDA : mean decreased accuracy, BFR-A : definition of BFR in absolute quantitative method, HU : Hounsfield unit, GCS : Glasgow coma scale, KPS : Karnofsky performance scale, HTN : hypertension, DM : diabetes mellitus, Op. : operation, MDG : mean decreased Gini, BFR% : definition of BFR in and relative quantitative method, DC : decompressive craniectomy, CP : cranioplasty.

Fig. 3.
Overlapped partial dependence plots (PDP) of result of random forest with hyper-ensemble approach (RF with HEA). Top ranked variables from importance calculation according to mean decreased accuracy from RF with HEA are visualize with overlapped PDPs to reveal the effect of individual factors on the target. If the PDP plot widely apart, the factor is more capable of classifying bone flap resorption (BFR). However, initial bone flap volume seems to be the only potential risk factor for bone flap resorption. BFR-A : definition of BFR in absolute quantitative method, BFR% : definition of BFR in and relative quantitative method, DC : decompressive craniectomy, CP : cranioplasty, HU : Hounsfield unit, GCS : Glasgow coma scale.
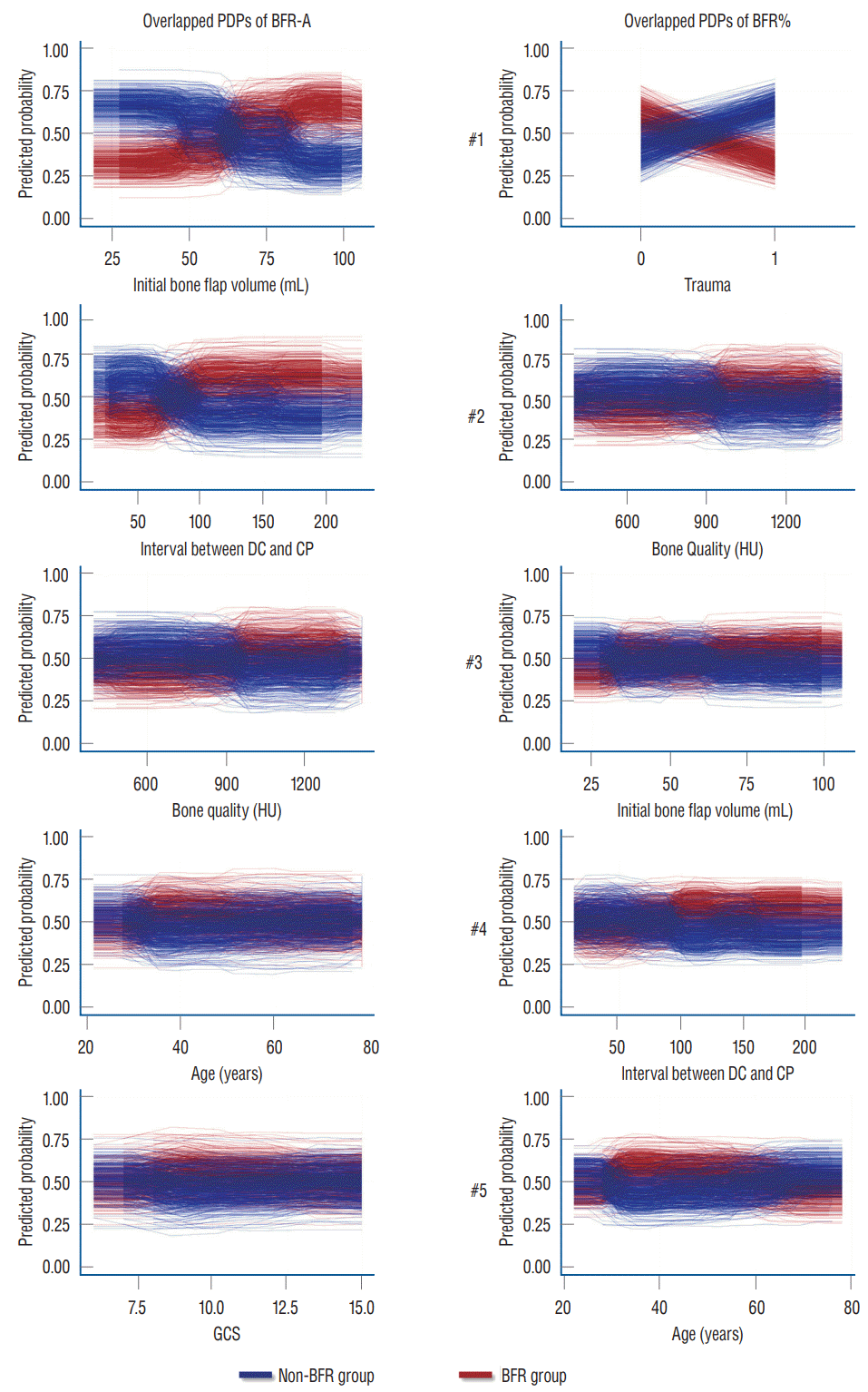
Table 1.
Demographics of the patients and statistical analysis
Factor | Overall (n=94) |
BFR-A |
p-value |
BFR% |
p-value | |||
---|---|---|---|---|---|---|---|---|
Non-BFR (n=72) | BFR (n=22) | Non-BFR (n=75) | BFR (n=19) | |||||
Age (years) | 51.43±14.14 | 52.33±14.37 | 48.45±13.26 | 0.172 | 52.36±14.53 | 47.74±12.14 | 0.160 | |
Sex, female | 58 (61.7) | 28 (38.9) | 8 (36.4) | 1.000 | 27 (36.0) | 9 (47.4) | 0.432 | |
Patient past history | ||||||||
Hypertension | 37 (39.4) | 30 (41.7) | 7 (31.8) | 0.463 | 32 (42.7) | 5 (26.3) | 0.293 | |
DM | 9 (9.6) | 7 (9.7) | 2 (9.1) | 1.000 | 7 (9.3) | 2 (10.5) | 1.000 | |
Cardiovascular disease | 8 (8.5) | 8 (11.1) | 0 (0.0) | 0.191 | 7 (9.3) | 1 (5.3) | 1.000 | |
Cerebral infarction | 1 (1.1) | 1 (1.4) | 0 (0.0) | 1.000 | 0 (0.0) | 1 (5.3) | 0.202 | |
Menopause | 15 (42.9) | 11 | 4 | 0.700 | 11 | 4 | 1.000 | |
Alcohol abuse | 47 (50.0) | 38 (52.8) | 9 (40.9) | 0.337 | 37 (50.7) | 9 (47.4) | 0.802 | |
Smoking history | 30 (31.9) | 22 (30.6) | 8 (36.4) | 0.795 | 22 (29.3) | 8 (42.1) | 0.409 | |
Chronic use of steroids | 1 (1.1) | 0 (0.0) | 1 (4.5) | 0.237 | 1 (1.3) | 0 (0.0) | 1.000 | |
Initial state on trauma | ||||||||
Trauma | 51 (54.3) | 51 (56.9) | 10 (45.5) | 0.464 | 43 (57.3) | 8 (42.1) | 0.304 | |
Open wound | 4 (4.3) | 4 (5.6) | 0 (0.0) | 0.569 | 4 (5.3) | 0 (0.0) | 0.578 | |
GCS | 12.5±2.9 | 12.5±2.9 | 12.3±3.0 | 0.760 | 12.4±3.0 | 12.8±2.4 | 0.785 | |
KPS | 56.0±19.1 | 56.5±19.6 | 54.1±17.6 | 0.786 | 56.1±20.1 | 55.3±14.7 | 0.793 | |
Cranioplasty related factor | ||||||||
Cranioplasty timing (days) | 86.5±49.8 | 84.0±46.9 | 94.6±58.9 | 0.503 | 86.8±48.3 | 85.2±56.8 | 776 | |
Initial bone flap volume (mL) | 62.94±20.36 | 57.98±18.61 | 79.18±17.41 | <0.001* | 62.24±20.44 | 65.68±20.33 | 0.684 | |
Skull fracture | 23 (24.5) | 19 (26.4) | 4 (18.2) | 0.574 | 19 (25.3) | 4 (21.1) | 0.774 | |
Bone quality (HU) | 826.94±293.30 | 825.8±292.05 | 830.64±304.24 | 0.894 | 828.96±289.39 | 818.96±316.33 | 0.885 | |
Operation site infection | 2 (2.1) | 1 (1.4) | 1 (4.5) | 0.419 | 2 (2.7) | 0 (0.0) | 1.000 | |
Shunt operation | 17 (18.0) | 13 (18.1) | 4 (18.2) | 1.000 | 16 (21.3) | 1 (5.3) | 0.344 |