Abstract
Notes
References

























Fig. 1
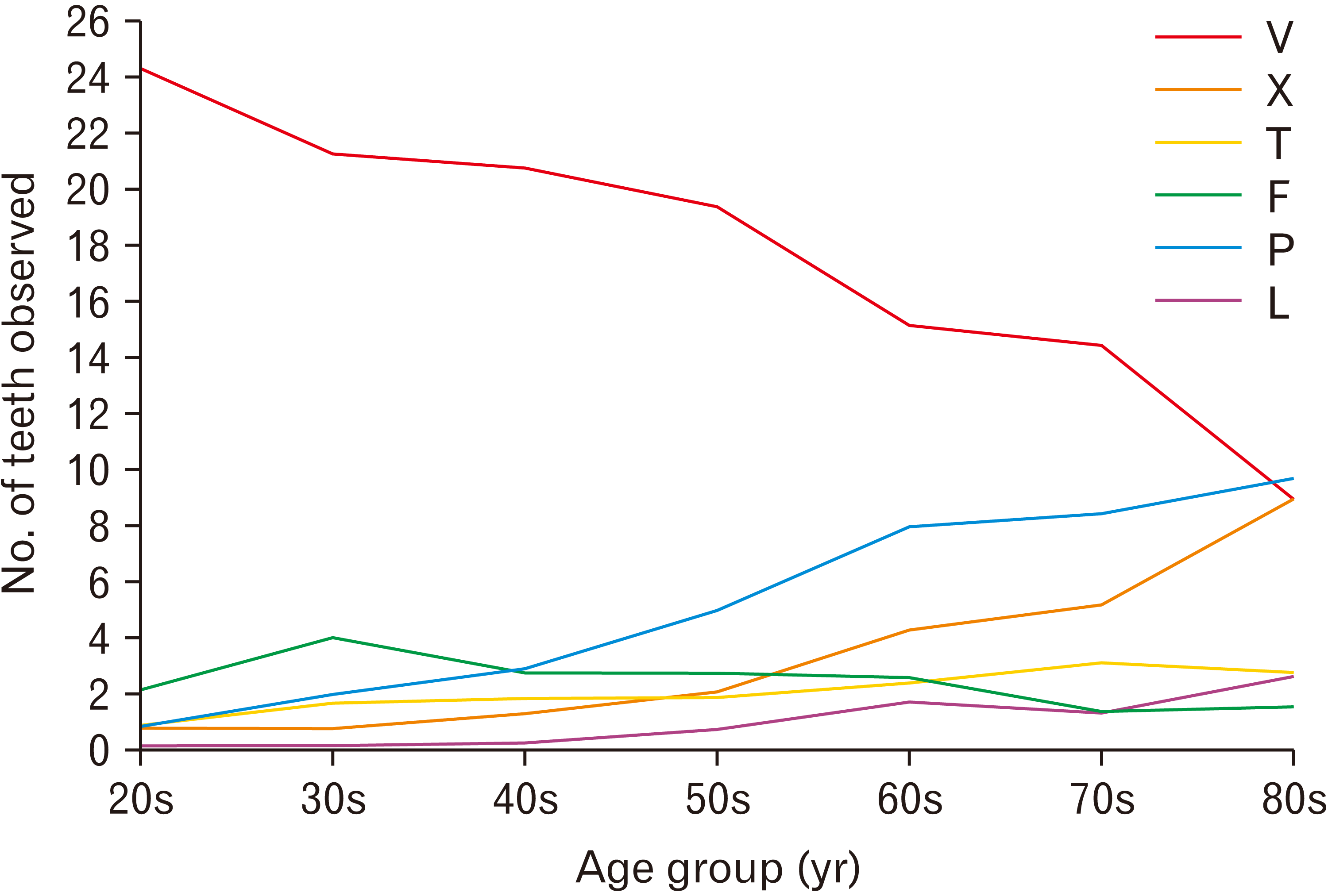
Fig. 2
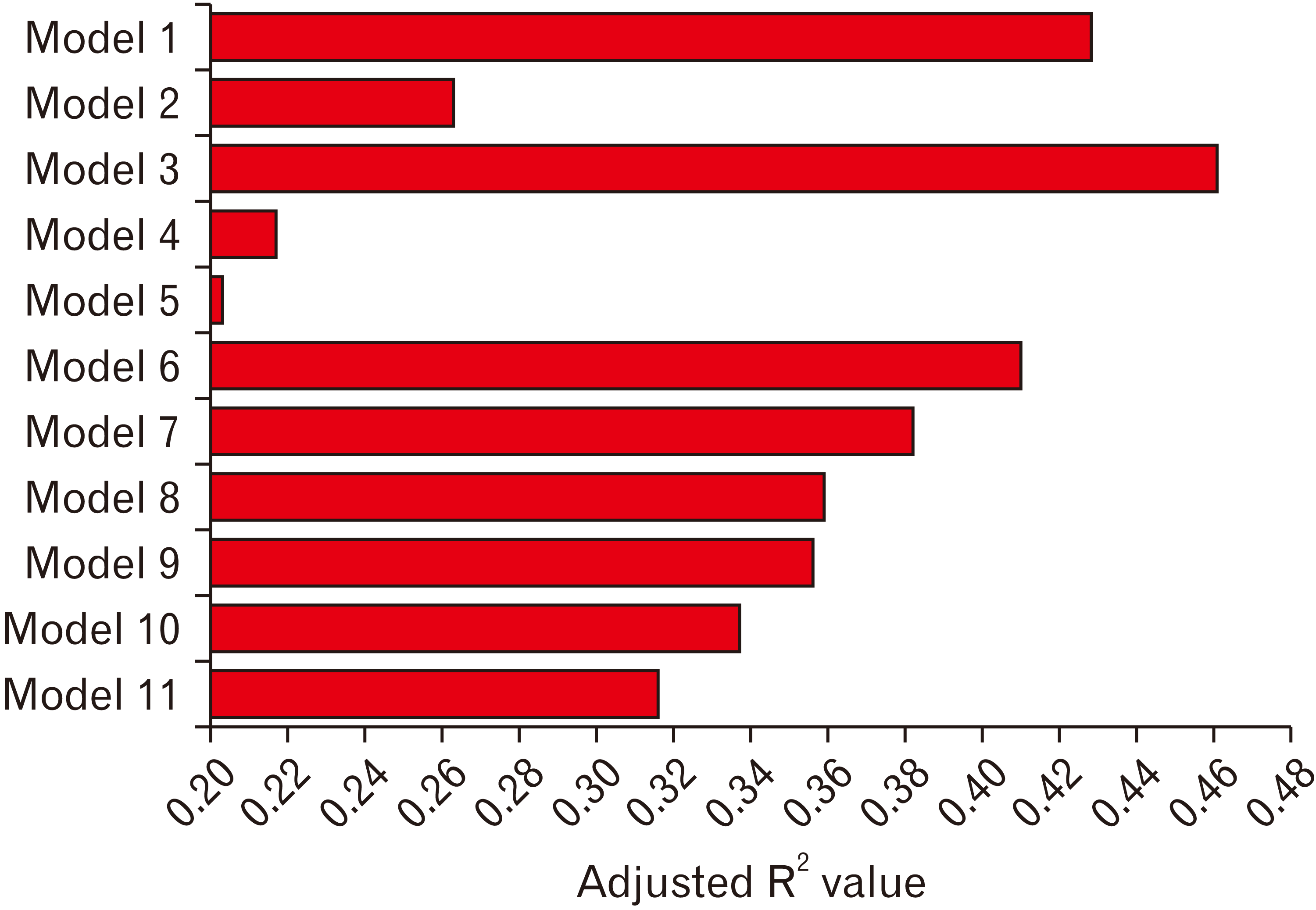
Table 1
Table 2
The contents of this table were modified from the study by Lee et al. [25].
Table 3
Model | Dental sextant combinations (types of teetha)) |
---|---|
1 | All six sextants (all teeth excluding four third molars) |
2 | Maxillary and mandibular anterior sextants (11, 12, 13, 21, 22, 23, 31, 32, 33, 41, 42, 43) |
3 | Maxillary and mandibular, right and left posterior sextants (14, 15, 16, 17, 24, 25, 26, 27, 34, 35, 36, 37, 44, 45, 46, 47) |
4 | Maxillary anterior sextant (11, 12, 13, 21, 22, 23) |
5 | Mandibular anterior sextant (31, 32, 33, 41, 42, 43) |
6 | Maxillary right and left posterior sextants (14, 15, 16, 17, 24, 25, 26, 27) |
7 | Mandibular right and left posterior sextants (34, 35, 36, 37, 44, 45, 46, 47) |
8 | Maxillary right posterior sextant (14, 15, 16, 17) |
9 | Maxillary left posterior sextant (24, 25, 26, 27) |
10 | Mandibular left posterior sextant (34, 35, 36, 37) |
11 | Mandibular right posterior sextant (44, 45, 46, 47) |
a)The types of teeth were noted by FDI two-digit teeth designation system [26].
Table 4
Values are presented as mean±SD. The definitions for each code are provided in Table 2.
Table 5
The definitions for each code are provided in Table 2. NSC, number of subjects where the code was observed; TNS, total number of subjects.
Table 6
Tooth type | Pearson’s correlation coefficient | |||||
---|---|---|---|---|---|---|
V | X | T | F | P | L | |
11 | –0.4156* | 0.3126* | 0.0682 | –0.0124 | 0.3015* | 0.0815 |
12 | –0.4031* | 0.3148* | 0.0493 | 0.0298 | 0.2666* | 0.0924 |
13 | –0.4451* | 0.2428* | 0.1310* | 0.0510 | 0.3372* | 0.0932 |
14 | –0.3466* | 0.2158* | 0.1698* | –0.0065 | 0.2932* | 0.0965* |
15 | –0.4176* | 0.3664* | 0.0448 | –0.0279 | 0.2948* | 0.1477* |
16 | –0.3364* | 0.3809* | 0.0491 | –0.2219* | 0.2578* | 0.2121* |
17 | –0.3965* | 0.4551* | 0.0761 | –0.2349* | 0.2509* | 0.2048* |
21 | –0.3961* | 0.3412* | 0.0537 | –0.0472 | 0.2780* | 0.1231* |
22 | –0.4188* | 0.3009* | 0.0888 | 0.0526 | 0.2679* | 0.0819 |
23 | –0.4252* | 0.2446* | 0.1886* | 0.1038* | 0.3019* | 0.1368* |
24 | –0.3085* | 0.1532* | 0.1213* | –0.0434 | 0.3080* | 0.1430* |
25 | –0.4041* | 0.3763* | –0.0027 | –0.1216* | 0.2908* | 0.2228* |
26 | –0.3356* | 0.3940* | 0.0194 | –0.2451* | 0.2458* | 0.2231* |
27 | –0.4486* | 0.4461* | 0.1385* | –0.1858* | 0.2756* | 0.1676* |
31 | –0.4091* | 0.3379* | 0.1436* | 0.0816 | 0.3372* | N/A |
32 | –0.4113* | 0.2100* | 0.2440* | 0.0894 | 0.3393* | 0.0812 |
33 | –0.4388* | 0.1453* | 0.2473* | 0.1072* | 0.3545* | 0.1219* |
34 | –0.2717* | 0.0312 | 0.1922* | –0.0284 | 0.3319* | 0.0686 |
35 | –0.4119* | 0.2591* | 0.1579* | –0.1139* | 0.3661* | 0.2425* |
36 | –0.3201* | 0.3320* | 0.0039 | –0.2641* | 0.3069* | 0.2616* |
37 | –0.3992* | 0.3975* | 0.0111 | –0.2186* | 0.2463* | 0.2385* |
41 | –0.4461* | 0.3339* | 0.1747* | 0.0610 | 0.3504* | 0.0727 |
42 | –0.3928* | 0.2780* | 0.1707* | 0.0459 | 0.3236* | 0.0907 |
43 | –0.3982* | 0.2141* | 0.2453* | 0.0725 | 0.3114* | 0.0988* |
44 | –0.2922* | 0.0135 | 0.1763* | 0.0641 | 0.3143* | 0.1469* |
45 | –0.3769* | 0.2350* | 0.0909 | –0.0789 | 0.3217* | 0.2089* |
46 | –0.2889* | 0.2995* | 0.0492 | –0.2943* | 0.3274* | 0.2738* |
47 | –0.2999* | 0.3585* | 0.0906 | –0.3371* | 0.3543* | 0.2752* |
Table 7
This multiple linear regression was performed with the number of teeth observed for dental codes and chronological age. The estimated age can be calculated as the sum of the products of each number of teeth observed for a dental code and its respective coefficient plus its respective intercept. The definitions for each code are provided in Table 2. RMSE, root mean square error; adjusted R2, coefficient of determination.