This article has been
cited by other articles in ScienceCentral.
Abstract
Background
Hyperkalemia is a potentially fatal condition that mandates rapid identification in emergency departments (EDs). Although a 12-lead electrocardiogram (ECG) can indicate hyperkalemia, subtle changes in the ECG often pose detection challenges. An artificial intelligence application that accurately assesses hyperkalemia risk from ECGs could revolutionize patient screening and treatment. We aimed to evaluate the efficacy and reliability of a smartphone application, which utilizes camera-captured ECG images, in quantifying hyperkalemia risk compared to human experts.
Methods
We performed a retrospective analysis of ED hyperkalemic patients (serum potassium ≥ 6 mmol/L) and their age- and sex-matched non-hyperkalemic controls. The application was tested by five users and its performance was compared to five board-certified emergency physicians (EPs).
Results
Our study included 125 patients. The area under the curve (AUC)-receiver operating characteristic of the application’s output was nearly identical among the users, ranging from 0.898 to 0.904 (median: 0.902), indicating almost perfect interrater agreement (Fleiss’ kappa 0.948). The application demonstrated high sensitivity (0.797), specificity (0.934), negative predictive value (NPV) (0.815), and positive predictive value (PPV) (0.927). In contrast, the EPs showed moderate interrater agreement (Fleiss’ kappa 0.551), and their consensus score had a significantly lower AUC of 0.662. The physicians’ consensus demonstrated a sensitivity of 0.203, specificity of 0.934, NPV of 0.527, and PPV of 0.765. Notably, this performance difference remained significant regardless of patients’ sex and age (P < 0.001 for both).
Conclusion
Our findings suggest that a smartphone application can accurately and reliably quantify hyperkalemia risk using initial ECGs in the ED.
Keywords: Hyperkalemia, Emergency Departments, Artificial Intelligence, Smartphone Application, Electrocardiography
INTRODUCTION
Hyperkalemia is a common electrolyte disturbance that occurs when serum potassium levels exceed the normal range of 3.5–5.0 mmol/L. Severe hyperkalemia, defined as serum potassium levels greater than 6.5 mmol/L, can lead to life-threatening cardiac arrhythmias and cardiac arrest
1 Early detection and prompt management of hyperkalemia are crucial to prevent adverse outcomes.
The 12-lead electrocardiogram (ECG) is a widely available and non-invasive tool that can aid in the diagnosis of hyperkalemia.
23 The classic ECG changes associated with hyperkalemia include tall, peaked T waves, widened QRS complex, and ultimately, loss of P waves.
45 However, these ECG changes can be subtle and difficult to quantify, particularly in the early stages of hyperkalemia.
67
Artificial intelligence (AI) algorithms have been proposed as a potential solution to improve the accuracy and clinical usability of ECG interpretation for various conditions including hyperkalemia.
891011 By quantifying the risk of hyperkalemia using AI, clinicians may be able to make more timely and informed decisions regarding patient management, especially in emergency department (ED).
However, the incorporation of existing AI algorithms for ECG analysis into clinicians’ routines poses significant challenges. Primarily, most of the AI algorithms typically depend on raw waveform data for their analyses. Given most physicians have only access to printed (on-screen or paper) ECGs, they cannot utilize these algorithms unless such services are systemically integrated into their ECG devices or electronical health records (EHRs) system. However, such systemic integration would cost a lot because of the diversity of manufacturers and EHR systems.
Some of these challenges may be mitigated through the development of AI capable of analyzing printed ECG image. Therefore, we previously developed a smartphone application “ECG Buddy
TM”.
1213 The application automatically detect, capture and analyze ECG waveforms using smartphone’s camera and is capable of extracting various digital biomarkers from printed 12-lead ECGs.
In this study, we evaluated its performances and compared it to human experts. As it used photo images as input, which can be subjected to various source of noise and variability, we also tested its reliability as a digital biomarker.
METHODS
Study design and patient selection
This was a retrospective study that included patients who visited the ED of an academic hospital between July to September 2021. We included patients who had a serum potassium level of 6 mmol/L or more (hyperkalemic group) with a 12-lead ECG done at the visit and their age- and sex-matched controls with a serum potassium level less than 6 mmol/L (non-hyperkalemic group) and an ECG from the same period.
Data collection
We collected demographic information including age and sex of the patient, chief complaints, initial serum potassium level and captured images of the waveform area of the ECGs from the electronic medical record system. Patients suspected of having pseudohyperkalemia were excluded.
ECG analysis
An AI smartphone application, named “ECG Buddy,” was used to analyze ECG images (
Fig. 1). It can analyze 12-lead ECGs and provide risk scores (Quantitatve ECG [QCG®] scores, ranging from 0 to 100) for various cardiac, hemodynamic and electrolyte problems using deep learning algorithms.
1213 Briefly, users take a picture of a 12-lead ECG printed either on paper or computer display to analyze the image and get AI risk scores for 10 conditions including critical events (respiratory or circulatory failure), acute coronary syndrome, ST-elevation myocardial infarction, myocardial injury, pulmonary edema, large pericardial effusion, left ventricular dysfunction, right ventricular dysfunction, pulmonary hypertension and hyperkalemia.
Fig. 1
The operating screen of the evaluated artificial intelligence software. (A) ECG image input. (B) ECG image analysis result. It reports rhythm classification and the risk scores of 10 cardiac function abnormalities and emergencies including hyperkalemia.
ECG = electrocardiogram.
To establish a benchmark for the AI score for hyperkalemia, a consensus score was obtained from a group of five board-certified emergency physicians who were blinded to the patients’ clinical information and potassium levels. The physicians were emergency medicine (EM) professors with at least 6-years of clinical work experience in tertiary care centers. Each physician was presented with ECG images and asked to determine whether the patient had hyperkalemia, defined as a potassium level of 6 mmol/L or higher. The percentage of experts who voted “yes” was calculated as the consensus score, and the consensus decision was based on the majority vote among the experts. This consensus score and decision were used to compare the accuracy of the AI model.
Statistical analysis
The application’s performance was evaluated in comparison to the consensus score obtained from the panel of physicians, using the area under the receiver operating characteristic curve (AUC-ROC). The sensitivity, specificity, positive predictive value (PPV), and negative predictive value (NPV) of the application were calculated by binning the AI score based on the Youden index. To assess the inter-rater agreement among the application users and among the expert physicians, Fleiss' Kappa was calculated. The data analysis was performed using R software, version 4.1.0.
141516
Ethics statement
The study was approved by the Institutional Review Board (IRB) of the Seoul National University Bundang Hospital (IRB No.: B-2201-735-104), and informed consent was waived due to its retrospective nature.
RESULTS
A total of 125 patients (64 hyperkalemic and 61 non-hyperkalemic) were included in this study (
Table 1). The mean age of the hyperkalemic group was 73.4 ± 10.6 years and that of the non-hyperkalemic group was 71.6 ± 11.3 years. There were no significant differences in age and sex, and the most common cause of ED visit was due to dyspnea in both of the groups. There were significant differences in QCG score between the groups with 75.9 (28.2–92.2) in hyperkalemia group and 2.8 (1.5–7.0) in normokalemia group.
Table 1
Study population
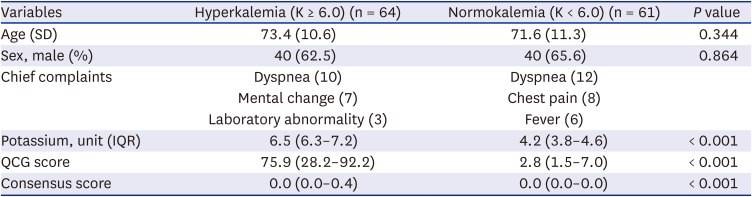
Variables |
Hyperkalemia (K ≥ 6.0) (n = 64) |
Normokalemia (K < 6.0) (n = 61) |
P value |
Age (SD) |
73.4 (10.6) |
71.6 (11.3) |
0.344 |
Sex, male (%) |
40 (62.5) |
40 (65.6) |
0.864 |
Chief complaints |
Dyspnea (10) |
Dyspnea (12) |
|
Mental change (7) |
Chest pain (8) |
Laboratory abnormality (3) |
Fever (6) |
Potassium, unit (IQR) |
6.5 (6.3–7.2) |
4.2 (3.8–4.6) |
< 0.001 |
QCG score |
75.9 (28.2–92.2) |
2.8 (1.5–7.0) |
< 0.001 |
Consensus score |
0.0 (0.0–0.4) |
0.0 (0.0–0.0) |
< 0.001 |
The application was evaluated by five evaluators including two physicians, two nurses and a paramedic (
Table 2). The AUC-ROC of the application for detecting hyperkalemia ranged from 0.898 (95% confidence interval, 0.842–0.954) to 0.904 (0.849–0.959) with almost perfect inter-rater agreement (Fleiss’ Kappa 0.948). Since all five evaluators show almost identical evaluation results (
Fig. 2), following analyses will be based on the assessment results of physician #1 whose AUC was 0.900.
Table 2
Accuracy and inter-rater agreement of the smartphone application

Evaluator |
AUC-ROC (95% CI) |
Fleiss’ Kappa |
App-PH1 |
0.900 (0.844–0.955) |
0.948 (Almost perfect agreement, using threshold of 22.1) |
App-PH2 |
0.898 (0.842–0.954) |
App-RN1 |
0.903 (0.849–0.956) |
App-RN2 |
0.902 (0.847–0.956) |
App-PM |
0.904 (0.849–0.959) |
Fig. 2
Scatter plots of artificial intelligence scores by the five application users.
PH1 = physician #1, PH2 = physician #2, RN1= registered nurse #1, RN2 = registered nurse #2, PM = paramedic.
The AUC of EM physicians ranged 0.522 (0.472–0.572) to 0.638 (0.568–0.709) with moderate inter-rater agreement (Fleiss’ Kappa 0.551;
Table 3). Even the consensus score of the EM physicians achieved AUC of only 0.662 (0.585–0.739) which was significantly lower than all of the application evaluations (
P < 0.001 for all).
Table 3
Accuracy and inter-rater agreement of the emergency physicians
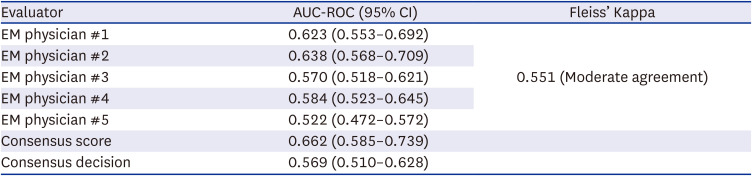
Evaluator |
AUC-ROC (95% CI) |
Fleiss’ Kappa |
EM physician #1 |
0.623 (0.553–0.692) |
0.551 (Moderate agreement) |
EM physician #2 |
0.638 (0.568–0.709) |
EM physician #3 |
0.570 (0.518–0.621) |
EM physician #4 |
0.584 (0.523–0.645) |
EM physician #5 |
0.522 (0.472–0.572) |
Consensus score |
0.662 (0.585–0.739) |
|
Consensus decision |
0.569 (0.510–0.628) |
|
Binned at the threshold of 22.1, the application (physician #1) showed a sensitivity of 0.797 (0.703–0.891), specificity of 0.934 (0.869–0.984), NPV of 0.815 (0.746–0.891), and PPV of 0.927 (0.862–0.982;
Table 4). The consensus decision of the EM physicians showed a sensitivity of 0.203 (0.109–0.297), specificity of 0.934 (0.869–0.984), PPV of 0.527 (0.491–0.563) and NPV of 0.765 (0.545–0.944).
Table 4
Diagnostic performance of binary decisions of the smartphone application (user: physician #1) and emergency physicians
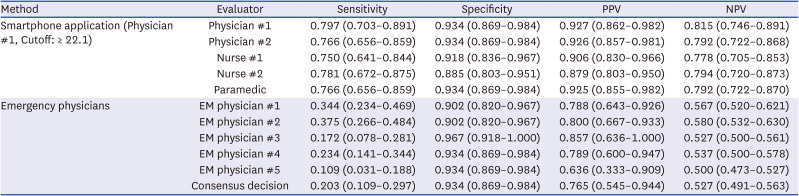
Method |
Evaluator |
Sensitivity |
Specificity |
PPV |
NPV |
Smartphone application (Physician #1, Cutoff: ≥ 22.1) |
Physician #1 |
0.797 (0.703–0.891) |
0.934 (0.869–0.984) |
0.927 (0.862–0.982) |
0.815 (0.746–0.891) |
Physician #2 |
0.766 (0.656–0.859) |
0.934 (0.869–0.984) |
0.926 (0.857–0.981) |
0.792 (0.722–0.868) |
Nurse #1 |
0.750 (0.641–0.844) |
0.918 (0.836–0.967) |
0.906 (0.830–0.966) |
0.778 (0.705–0.853) |
Nurse #2 |
0.781 (0.672–0.875) |
0.885 (0.803–0.951) |
0.879 (0.803–0.950) |
0.794 (0.720–0.873) |
Paramedic |
0.766 (0.656–0.859) |
0.934 (0.869–0.984) |
0.925 (0.855–0.982) |
0.792 (0.722–0.870) |
Emergency physicians |
EM physician #1 |
0.344 (0.234–0.469) |
0.902 (0.820–0.967) |
0.788 (0.643–0.926) |
0.567 (0.520–0.621) |
EM physician #2 |
0.375 (0.266–0.484) |
0.902 (0.820–0.967) |
0.800 (0.667–0.933) |
0.580 (0.532–0.630) |
EM physician #3 |
0.172 (0.078–0.281) |
0.967 (0.918–1.000) |
0.857 (0.636–1.000) |
0.527 (0.500–0.561) |
EM physician #4 |
0.234 (0.141–0.344) |
0.934 (0.869–0.984) |
0.789 (0.600–0.947) |
0.537 (0.500–0.578) |
EM physician #5 |
0.109 (0.031–0.188) |
0.934 (0.869–0.984) |
0.636 (0.333–0.909) |
0.500 (0.473–0.527) |
Consensus decision |
0.203 (0.109–0.297) |
0.934 (0.869–0.984) |
0.765 (0.545–0.944) |
0.527 (0.491–0.563) |
When analyzed by age category (≥ 73, median age cutoff) or sex, the AUC-ROC of the QCG score was 0.905 (0.826–0.984) for the older age group, 0.896 (0.816–0.977) for the younger age group, 0.961 (0.916–1.000) for the female population, and 0.863 (0.778–0.947) for the male population, respectively; these results revealed a significant sex difference (
P = 0.045) and an insignificant age difference (
P = 0.879), as shown in
Table 5. The performance was consistently higher compared to EM physicians’ consensus score in all of the groups (
P < 0.001 for all).
Table 5
Comparison of AUC-ROC between the AI score (user: physician #1) and the emergency physicians’ consensus score in patient subgroups

Subgroup |
Application |
Physicians |
P value |
Older (≥ 73) |
0.905 (0.826–0.984) |
0.673 (0.569–0.777) |
< 0.001 |
Younger (< 73) |
0.896 (0.816–0.977) |
0.659 (0.544–0.774) |
< 0.001 |
Female |
0.961 (0.916–1.000) |
0.733 (0.623–0.843) |
< 0.001 |
Male |
0.863 (0.778–0.947) |
0.623 (0.521–0.726) |
< 0.001 |
DISCUSSION
In this study, we appraised the efficiency of a smartphone-based AI analyzer that utilizes camera input for screening hyperkalemia in ED patients. Our findings demonstrate that the AI ECG reader, relying on image input, surpasses human experts in identifying hyperkalemia from preliminary ECGs, exhibiting near-perfect inter-rater consistency. This constitutes the inaugural study that reports the accuracy of an ECG image analyzer, smartphone-based and camera-operated, in diagnosing hyperkalemia. Furthermore, it is among the rare studies that report the inter-rater reliability of an AI application.
17
Prompt detection of hyperkalemia is crucial in ED, given that severe cases can progress to fatal cardiac arrest. Immediate interventions, including the administration of insulin and glucose, bicarbonate, and beta-agonists, can preempt this critical event. Nevertheless, the time required to wait for laboratory results to confirm hyperkalemia may lead to considerable delays, thereby amplifying the risk of adverse outcomes.
In contrast to laboratory tests, an ECG test requires merely a minute. Thus, the primary advantage of utilizing AI for hyperkalemia screening in the ED in comparison to laboratory results is its expedience, which can facilitate the immediate initiation of the appropriate therapeutic strategy. In this study, we have demonstrated the precision and reliability of AI as a diagnostic tool for hyperkalemia screening. Given that hyperkalemia's emergency treatment is straightforward and relatively safe, while severe hyperkalemia can precipitate cardiac arrest, the application of ECG AI for hyperkalemia might significantly enhance ED triage and emergency treatment.
Earlier studies have demonstrated that ECG AIs can accurately detect hyperkalemia. For instance, Galloway et al. predicted hyperkalemia employing a convolution neural network using a variable number of ECG leads.
9 Lin et al.
8 utilized a hierarchical attention network architecture (ECG12Net) and reported superior accuracy in detecting dyskalemia compared to emergency physicians and cardiologists. Moreover, Kwon et al.
18 developed deep learning models to predict abnormalities in electrolyte levels, including potassium, sodium, and calcium. While their methodology demonstrated excellent accuracy, these AI services have not seen widespread use due to the requirement of raw ECG signal data, notwithstanding that healthcare providers are only accessible to printed ECG materials.
Thus, the use of camera input data, as opposed to ECG raw data, for AI analysis as exhibited in our study may considerably increase the accessibility of healthcare workers to AI technology. The ubiquity of smartphones with camera capabilities among healthcare workers allows for ease of access to this technology simply by downloading the application. Moreover, this approach has a financial advantage, given that there is no need to replace or upgrade existing ECG machines or EHR systems.
The AI demonstrated enhanced performance within the female group. While this could potentially be a coincidental observation, it's important to acknowledge the existence of sex differences in cardiac electrophysiology. For example, ECGs from men typically display a higher T-wave amplitude and an increased ST angle, while those from women often show a longer QT duration.
19 Furthermore, reports have indicated sex differences in ECG changes associated with pathological conditions, such as left ventricular hypertrophy (LVH).
20 Despite these known differences, no previous studies have specifically addressed the sex-based performance disparity of AI in hyperkalemia screening. As such, further research is necessary to determine whether this finding can be replicated.
Our study is not without limitations. Firstly, it is a single-center, retrospective study, potentially limiting the generalizability of our findings. Secondly, the sample size is also relatively small, suggesting a need for verification in a larger, multi-center study. Thirdly, the decision threshold for the QCG score, set at 22.1 based on the Youden index, yielded a sensitivity of 79.7% and a specificity of 93.4%. However, in a real clinical application, this threshold could be adjusted to meet predetermined minimum sensitivity or specificity requirements, depending on the ED's policies or available resources. Fourthly, the AI application lacks an explainability feature, which represents a significant limitation. This absence hinders users from learning from the AI algorithms, as understanding the features used to classify signals could provide valuable insights. Lastly, while our results illustrate the superior ability of the image-based AI ECG reader over clinicians in detecting hyperkalemia, the clinical efficacy of this technology needs further exploration. Future investigations should aim to evaluate the impact of this technology on patient outcomes and healthcare costs.
In conclusion, the use of an image-based AI ECG reader with camera input is both an accurate and reliable tool for hyperkalemia screening at ED triage. This potentially enables early treatment of hyperkalemic patients and leads to improved patient outcomes.