Abstract
Background
Papillary thyroid carcinoma (PTC) can be classified into two distinct molecular subtypes, BRAF-like (BL) and RAS-like (RL). However, the molecular characteristics of each subtype according to clinicopathological factors have not yet been determined. We aimed to investigate the gene signatures and tumor microenvironment according to clinicopathological factors, and to identify the mechanism of progression in BL-PTCs and RL-PTCs.
Methods
We analyzed RNA sequencing data and corresponding clinicopathological information of 503 patients with PTC from The Cancer Genome Atlas database. We performed differentially expressed gene (DEG), Gene Ontology, and molecular pathway enrichment analyses according to clinicopathological factors in each molecular subtype. EcoTyper and CIBERSORTx were used to deconvolve the tumor cell types and their surrounding microenvironment.
Results
Even for the same clinicopathological factors, overlapping DEGs between the two molecular subtypes were uncommon, indicating that BL-PTCs and RL-PTCs have different progression mechanisms. Genes related to the extracellular matrix were commonly upregulated in BL-PTCs with aggressive clinicopathological factors, such as old age (≥55 years), presence of extrathyroidal extension, lymph node metastasis, advanced tumor-node-metastasis (TNM) stage, and high metastasis-age-completeness of resection-invasion-size (MACIS) scores (≥6). Furthermore, in the deconvolution analysis of tumor microenvironment, cancer-associated fibroblasts were significantly enriched. In contrast, in RL-PTCs, downregulation of immune response and immunoglobulin-related genes was significantly associated with aggressive characteristics, even after adjusting for thyroiditis status.
Conclusion
The molecular phenotypes of cancer progression differed between BL-PTC and RL-PTC. In particular, extracellular matrix and cancer-associated fibroblasts, which constitute the tumor microenvironment, would play an important role in the progression of BL-PTC that accounts for the majority of advanced PTCs.
Papillary thyroid carcinoma (PTC) is the most common thyroid malignancy, accounting for approximately 90% of all thyroid malignancies [1]. Patients with PTC generally have a favorable prognosis, with a 10-year survival rate exceeding 90% [2]. Despite the low mortality risk, some patients present aggressive tumor behaviors and a poor prognosis, with recurrence and cancer-specific death rates of up to 30% [3]. Clinicopathological characteristics such as old age at diagnosis, large tumor size, presence of extrathyroidal extension lymph node metastasis, and advanced tumor, node, metastasis (TNM) stage or scoring systems have been known to be associated with poor prognosis for differentiated thyroid cancer [4-6].
The Cancer Genome Atlas (TCGA) proposed that PTC could be classified into two molecular subtypes based on transcriptomic characteristics, BRAF-like (BL) and RAS-like (RL), in which the gene expression profile of the tumor resembles either the BRAFV600E or RAS mutant profiles [7]. They reported that each molecular subtype of PTCs represents differential regulation of intracellular signaling pathways, such as mitogen-activated protein kinase (MAPK) and phosphoinositide 3-kinase (PI3K), resulting in profound behavioral differences. RL-PTCs are highly differentiated tumors that are more frequent in follicular histology, whereas BL-PTCs are less differentiated tumors that are more frequent in classical, tall cell histology and exhibit more aggressive behaviors [8-10]. Although distinct characteristics of BL-PTC and RL-PTC have been demonstrated, the molecular characteristics of the tumors according to the clinicopathological factors in each molecular subtype have not been elucidated.
The tumor microenvironment (TME) surrounding tumor cells consists of immune cells, endothelial cells, fibroblasts, and extracellular matrix (ECM) components [11]. It induces immune tolerance and tumor angiogenesis, contributing to tumor growth and progression. Recently, cancer-associated fibroblasts (CAFs) were reported to promote the progression of PTC distinctively in BRAF-driven tumors [12,13]. Nonetheless, the TME and progression mechanisms of each molecular subtype of PTC have not been fully clarified.
This study investigated gene signatures and the TME according to clinicopathological factors in BL-PTCs and RL-PTCs through comprehensive transcriptomic analyses to identify the progression mechanism in each molecular subtype of PTCs.
The RNA sequencing data of 503 PTC patients were obtained from TCGA (http://portal.gdc.cancer.gov/), and corresponding clinicopathological information was retrieved from cBioportal (https://www.cbioportal.org/) [14]. This research was approved by the Institutional Review Board of CHA Bundang Medical Center (No. 2020-03-029) and the informed consent was waived by the board. The major clinicopathological factors according to the molecular subtype are presented in Supplemental Table S1. The median age of the patients was 46 years (interquartile range [IQR], 35 to 58) and the median tumor size was 2.5 cm (IQR, 1.6 to 4.0). There were no differences in age, tumor size, metastasis-age-completeness of resection-invasion-size (MACIS) prognostic score, or thyroiditis between the BL-PTC and RL-PTC groups. Extrathyroidal extension, lymph node metastasis, and advanced TNM stage were observed more frequently in BL-PTC than in RL-PTC.
BRAF-RAS score (BRS) was determined to assign molecular subtypes of samples which were not specified in the TCGA study. As described in the original study, the centroids for tumors with BRAFV600E mutations, c(B) and RAS activating mutations, c(R) were computed with expression of 71-gene signature via stats R package. BRS score of each tumor was calculated as the difference between the distance from c(B) and c(R) [7]. Then, PTCs with negative BRS were defined as BL, while those with positive BRS were assigned to RL. The read counts were normalized using the variance stabilizing transformation in the DESeq2 package [15], and principal component analysis (PCA) was performed on the normalized gene expression of the most variable 500 genes.
Differentially expressed genes (DEGs) were identified using DESeq2 according to clinicopathological factors in BL-PTCs and RL-PTCs [15]. The P values were corrected to q values for multiple testing using the Benjamini-Hochberg method. Genes with |log2(fold change)| ≥1 and q value <0.05 were considered as differentially expressed and were visualized by volcano plots. Venn diagrams were used to identify overlapping and non-overlapping DEGs between the molecular subtypes of PTCs.
To identify biological themes and molecular pathways that were significantly enriched in DEGs, the Gene Ontology (GO) and Kyoto Encyclopedia of Genes and Genomes (KEGG) pathway analyses were performed by the DAVID 6.8 database (https://david-d.ncifcrf.gov/home.jsp) [16]. For the ECM score reflecting the abundance of ECM proteins, the enrichment score was determined by single-sample gene set enrichment analysis [17] using a gene set related to collagen-containing ECM (GO:0062023). The gene set was downloaded from the Molecular Signatures Database (MSigDB) version 7.5.1 [18] and the score was calculated using the gene set variation analysis (GSVA) package.
To explore the cell types of the tumors and their surrounding microenvironment, CIBERSORTx (https://cibersortx.stanford.edu/) and EcoTyper (https://ecotyper.stanford.edu/) were applied using Fragments Per Kilobase of transcript per Million mapped reads (FPKM)-normalized expression as the input data file. CIBERSORTx is a digital cytometer used to infer cell type-specific gene expression profiles [19]. CIBERSORTx was run with 100 permutations in relative mode for TR4 and LM22 signature matrices, which have been used to calculate the abundance of fibroblasts and immune cells, and to deconvolve bulk immune cell populations [19,20]. EcoTyper is a machine learning framework for characterizing cell type-specific states and multicellular communities from gene expression data, including bulk transcriptomes [21]. The cell state abundance of fibroblasts was estimated in each sample; in particular, those of CAFs (state 3) and normal-enriched fibroblasts (state 2) were further analyzed. For the validation analyses, we employed additional computational deconvolution tools. xCell and ESTIMATE were used to estimate the overall abundance of fibroblasts and immune cells [22,23]. MCP-counter and EPIC were used to validate the abundance of CAFs [24,25].
The Kolmogorov-Smirnov test was performed on continuous variables to determine normality. Non-normally distributed data were presented as median with IQR, and the two-tailed Wilcoxon rank-sum test was conducted for statistical comparisons. Categorical data were reported as counts with percentages and analyzed using the Pearson’s chi-square test. Statistical significance was set at P<0.05. All data analyses were performed using R studio version 4.1.2 (R Foundation for Statistical Computing, Vienna, Austria).
A total of 503 patients with PTC were classified into two molecular subtypes, BL and RL, by BRS; 349 patients with negative BRS were classified as having BL-PTC, and 154 patients with positive BRS were assigned to RL-PTC. These molecular subtypes showed good separation in the PCA plot (Supplemental Fig. S1).
Next, in each of BL-PTCs and RL-PTCs, gene expression profiles were examined according to the clinicopathological factors, such as age (≥55 years vs. <55 years: n=107 vs. 242 and 59 vs. 95 for BL-PTCs and RL-PTCs, respectively), tumor size (>4 cm vs. ≤4 cm: n=71 vs. 278 and 36 vs. 118 for BL-PTCs and RL-PTCs, respectively), extrathyroidal extension (presence vs. absence: n=138 vs. 211 and 20 vs. 134 for BL-PTCs and RL-PTCs, respectively), lymph node metastasis (N1 vs. N0: n=202 vs. 123 and 23 vs. 105 for BL-PTCs and RL-PTCs, respectively), TNM stage (III–IV vs. I–II: n=133 vs. 216 and 33 vs. 121 for BL-PTCs and RL-PTCs, respectively), and MACIS score (≥6 vs. <6: n=108 vs. 241 and 43 vs. 111 for BL-PTCs and RL-PTCs, respectively). Several DEGs were identified between aggressive and indolent clinicopathological features in each molecular subtype (Fig. 1A). However, even for the same clinicopathological variables, overlapping DEGs between the two molecular subtypes were uncommon, indicating that BL-PTCs and RL-PTCs have different progression mechanisms (Fig. 1B).
Functional enrichment analyses were performed using the upregulated or downregulated DEGs between the aggressive and indolent clinicopathological characteristics of BL-PTCs and RL-PTCs. Notably, in BL-PTCs with aggressive clinicopathological factors, such as old age, presence of extrathyroidal extension, lymph node metastasis, advanced TNM stage, and high MACIS, genes related to ECM and collagen metabolism were commonly upregulated (Fig. 2A, Supplemental Table S2). Immune-related pathways, including the classical complement and Fc receptor signaling pathways, were downregulated in BL-PTCs with aggressive clinicopathological factors, except for ymph node metastasis (Fig. 2B, Supplemental Table S3), but this became insignificant when only patients without thyroiditis (n=228) were analyzed (Fig. 2B, Supplemental Table S4).
Next, in RL-PTCs with aggressive clinicopathological features, there were no molecular pathways or GO enrichment for upregulated DEGs compared with RL-PTCs with indolent features. Instead, downregulated DEGs were mainly immunoglobulin-related genes involved in classical complement and Fc receptor signaling pathways (Fig. 2C, Supplemental Table S5). In contrast to BL-PTCs, this association remained significant after adjusting for thyroiditis status (n=108) (Fig. 2C, Supplemental Table S6).
Then, using the deconvolution methods, we estimated the proportions of fibroblast and immune cells and compared their abundance between BL-PTCs and RL-PTCs (Supplemental Fig. S2). BL-PTCs showed higher abundance of fibroblast and immune cell than RL-PTCs irrespective of thyroiditis status (Supplemental Fig. S2A, B). In both BL-PTCs and RL-PTCs, there were no differences in total immune cell abundance according to clinicopathological factors (Supplemental Fig. S2C, D). Although immunoglobulin-related genes were commonly downregulated in aggressive RL-PTCs regardless thyroiditis state, no common immune cell subtypes showed differences according to clinicopathological factors in both BL-PTCs and RL-PTCs (Supplemental Fig. S3).
Because ECM- and collagen-related genes were mainly activated in the DEG analysis of BL-PTCs, the ECM score was investigated to represent the degree of upregulation of ECM components. The score was significantly increased in BL-PTCs, particularly those with aggressive clinicopathological factors, such as old age (P=0.019), presence of extrathyroidal extension (P<0.001), advanced TNM stage (P<0.001), and high MACIS (P=0.002). However, the ECM score did not show differences in RL-PTCs according to clinicopathological factors, except for a significant decrease in old age (P=0.002) (Fig. 3A).
Next, the abundance of fibroblasts that synthesize ECM and collagen was analyzed. The abundance of total fibroblasts was significantly increased in BL-PTCs with aggressive clinicopathological characteristics, such as old age (P<0.001), extrathyroidal extension (P<0.001), advanced TNM stage (P<0.001), and high MACIS score (P<0.001) (Supplemental Fig. S2C). Furthermore, when fibroblasts were classified based on their state by the EcoTyper, those in cancer-associated and normal-enriched states showed significant differences in BL-PTCs according to clinicopathological factors (Fig. 3B), while other states of fibroblasts, including myofibroblast-like, migratory-like, and promigratory-like states, did not show the difference (Supplemental Fig. S4). CAFs significantly increased in old age (P=0.027), presence of extrathyroidal extension (P<0.001), advanced TNM stage (P<0.001), and high MACIS (P<0.001). In contrast, the abundance of normal fibroblasts was reduced with extrathyroidal extension (P=0.007), lymph node metastasis (P=0.030), advanced TNM stage (P=0.043), and high MACIS (P=0.020).
Unlike BL-PTCs, the total fibroblast abundance did not differ according to each clinicopathological factor in RL-PTCs (Supplemental Fig. S2C). The CAF abundance was even lower in RL-PTCs with old age (P=0.026). The normal fibroblast abundance was decreased in large tumor size (P=0.049). All other comparisons in RL-PTCs did not show significant differences in fibroblast cell state abundance (Fig. 3B).
To validate the findings, we further analyzed using additional deconvolution tools (Supplemental Figs. S5, S6). We can observe the consistent results that the high-risk clinicopathological features were associated with the increased abundance of total fibroblasts and CAFs in BL-PTCs but not in RL-PTCs.
This study showed gene signatures and the TME according to the clinicopathological factors in BL-PTCs and RL-PTCs. The DEGs and changes in the TME observed between tumors with aggressive and indolent phenotypes in the two molecular subtypes exhibited little overlap, suggesting that BL-PTCs and RL-PTCs have distinct mechanisms of tumor progression.
In BL-PTCs with aggressive clinicopathological characteristics, genes encoding ECM were differentially upregulated, suggesting the importance of these molecular components in the progression of BL-PTCs. CAFs have been demonstrated to modulate cancer development by depositing and remodeling ECM [26]. Indeed, the results of the present study showed that CAFs were significantly increased, whereas normal fibroblasts were decreased in BL-PTCs with aggressive features. These findings correspond well with those of previous studies [12,13]. In addition, consistent with our findings, a recent study on single-cell RNA sequencing of PTC demonstrated that a dedifferentiated subtype of BL-PTCs showed a distinct ecotype enriched in CAFs [27]. This indicates that CAFs can play key roles in cancer progression, especially in BL-PTCs.
In RL-PTCs with aggressive clinicopathological characteristics, genes related to immune response and immunoglobulin were differentially downregulated even after adjustment for thyroiditis status. Moreover, RAS mutant PTCs are immune cell-poor and correlate with significantly decreased fractions of B cells and M1 macrophages [28]. B cells and immunoglobulins in the TME play important roles in anti-tumor immunity [29]. B cells can suppress tumors by producing tumor-specific antibodies; however, certain subsets of B cells can promote tumor growth by releasing immunosuppressive cytokines and ineffective antibodies [29,30]. Recently, B-cell-specific immunoglobulin genes have been suggested as biomarkers for better prognosis in triple-negative breast cancer [31]. Thus, we hypothesized that RL-PTC promotes tumor progression by suppressing the anti-tumor immune response. However, when we deconvolved B-cell abundance in RL-PTCs, there was no significant difference between aggressive and indolent tumors, which might be due to the low total abundance of immune cells in RL-PTCs (Supplemental Fig. S2A, C). Further research is required to assess the immunoglobulin repertoires and clonotypes of B-cell populations.
The main driver events of thyroid cancer, BRAFV600E and RAS mutations, contribute to oncogenic transformation and cell proliferation by activating distinct intracellular signaling pathways. Although both BL-PTCs and RL-PTCs activate the MAPK/extracellular signal-regulated kinase (ERK) pathway, the ERK score, developed by the TCGA study, was found to be strongly correlated with BRS [7]. Consequently, BL-PTCs exhibit higher MAPK activity compared to RL-PTCs. Moreover, RL-PTCs are primarily involved in the activation of PI3K/AKT/mammalian target of rapamycin (mTOR) pathway. A previous study found that PTC can progress to poorly-differentiated thyroid cancer via the MAPK-dependent epithelial-mesenchymal transition process [32]. This finding may partly explain our results of the increased CAF abundance in BL-PTC, especially in those with aggressive clinicopathological features. Likewise, immunological properties can be different depending on the molecular subtype, due to differences in activation of the MAPK/ERK and PI3K/AKT/mTOR pathways, which are involved in various immune responses [33,34]. However, further studies are needed to decipher the biological mechanisms responsible for the different TME changes during cancer progression.
Our study has several strengths. First, we compared gene signatures and the TME according to various clinicopathological prognostic factors. Also, because PTC is divided two distinct molecular subtypes by gene expression profiles, BL and RL, resulting in different tumor phenotypes and behaviors, we analyzed according to clinicopathological characteristics within each molecular subtype to eliminate the confounding effect of divergent gene signatures of BL and RL. In addition, we analyzed large-scale and representative TCGA data with various clinicopathological factors. There were an adequate number of patients to in each group, which allowed for valid comparisons. Regarding the limitations of our study, the TME of each molecular subtype was assessed using a deconvolution method for the bulk gene expression data. It remains unclear whether certain subsets of B cells differ in RL-PTCs with aggressive clinicopathological features. Nevertheless, when compared to a recent single-cell transcriptome study [27], we found similar results for CAF enrichment in BL-PTCs and minimized the selection bias by using a larger number of samples.
In conclusion, the phenotypes of PTC progression differed according to the molecular subtype. ECM and CAFs play crucial roles in the progression of BL-PTCs by modulating the TME. Suppression of the immune response and immunoglobulin levels may be related to the progression of RL-PTCs. Controlling different progression mechanisms, especially alterations in the TME, can be a tailored strategy to prevent cancer progression and treat refractory PTCs.
Supplementary Material
Supplemental Table S1.
Clinicopathological Characteristics of Patients with Papillary Thyroid Carcinoma according to the Molecular Subtype
Supplemental Table S2.
Upregulated Differentially Expressed Genes and Enriched Functional Annotations of BL-PTCs according to the Clinicopathological Prognostic Factors
Supplemental Table S3.
Downregulated Differentially Expressed Genes and Enriched Functional Annotations of BL-PTCs before Adjustment for the Thyroiditis Status according to the Clinicopathological Prognostic Factors
Supplemental Table S4.
Downregulated Differentially Expressed Genes and Enriched Functional Annotations of BL-PTCs after Adjustment for the Thyroiditis Status according to the Clinicopathological Prognostic Factors
Supplemental Table S5.
Downregulated Differentially Expressed Genes and Enriched Functional Annotations of RL-PTCs before Adjustment for the Thyroiditis Status according to the Clinicopathological Prognostic Factors
Supplemental Table S6.
Downregulated Differentially Expressed Genes and Enriched Functional Annotations of RL-PTCs after Adjustment for the Thyroiditis Status according to the Clinicopathological Prognostic Factors
Supplemental Fig. S1.
Transcriptomic profiles of papillary thyroid carcinoma (PTC) according to molecular subtype. Principal component analysis plot of PTC.
Supplemental Fig. S2.
Tumor microenvironment of BRAF-like papillary thyroid carcinoma (BL-PTC) and RAS-like papillary thyroid carcinoma (RL-PTC). Abundance of (A) fibroblasts and (B) immune cells in BL-PTCs and RL-PTCs irrespective of clinicopathological factors. Abundance of fibroblasts and immune cells in BL-PTCs and RL-PTCs (C) in all patients and (D) in patients without thyroiditis according to the clinicopathological characteristics. ETE, extrathyroidal extension; LNM, lymph node metastasis; TNM, tumor-node-metastasis; MACIS, metastasis-age-completeness of resection-invasion-size; NS, not significant (P≥0.05). aP<0.05; bP<0.001; cP<0.0001.
Supplemental Fig. S3.
Abundance of immune cell population in (A) BRAF-like papillary thyroid carcinoma and (B) RAS-like papillary thyroid carcinoma without thyroiditis according to the clinicopathological factors. ETE, extrathyroidal extension; LNM, lymph node metastasis; TNM, tumor-node-metastasis; MACIS, metastasis-age-completeness of resection-invasion-size; NS, not significant (P≥0.05); NK, natural killer. aP<0.05; bP<0.01.
Supplemental Fig. S4.
Heatmap representing the cell state abundances of fibroblasts in each sample. ETE, extrathyroidal extension; LNM, lymph node metastasis; TNM, tumor-node-metastasis; MACIS, metastasis-age-completeness of resection-invasion-size.
Supplemental Fig. S5.
Fibroblast and immune cell infiltration in BRAF-like papillary thyroid carcinomas and RAS-like papillary thyroid carcinomas estimated by (A) xCell and (B) ESTIMATE. ETE, extrathyroidal extension; LNM, lymph node metastasis; TNM, tumor-nodemetastasis; MACIS, metastasis-age-completeness of resection-invasion-size; NS, not significant (P≥0.05). aP<0.05; bP<0.01.
Supplemental Fig. S6.
Cancer-associated fibroblast (CAF) infiltration in BRAF-like papillary thyroid carcinomas and RAS-like papillary thyroid carcinomas estimated by (A) MCP-counter and (B) EPIC. ETE, extrathyroidal extension; LNM, lymph node metastasis; TNM, tumor-node-metastasis; MACIS, metastasis-age-completeness of resection-invasion-size; NS, not significant (P≥0.05). aP<0.05; bP<0.01; cP<0.001; dP<0.0001.
ACKNOWLEDGMENTS
This work was supported by the National Research Foundation of Korea (NRF) grant funded by the Korea government (MSIT) (No. NRF-2020R1C1C1003924).
REFERENCES
1. Davies L, Welch HG. Current thyroid cancer trends in the United States. JAMA Otolaryngol Head Neck Surg. 2014; 140:317–22.
2. Amin MB, Edge SB, Greene FL, Bryd DR, Brookland RK, Washington MK, et al. AJCC cancer staging manual. 8th ed. New York: Springer;2017.
3. Grogan RH, Kaplan SP, Cao H, Weiss RE, Degroot LJ, Simon CA, et al. A study of recurrence and death from papillary thyroid cancer with 27 years of median follow-up. Surgery. 2013; 154:1436–47.
4. Nixon IJ, Wang LY, Migliacci JC, Eskander A, Campbell MJ, Aniss A, et al. An international multi-institutional validation of age 55 years as a cutoff for risk stratification in the AJCC/UICC staging system for well-differentiated thyroid cancer. Thyroid. 2016; 26:373–80.
5. Leboulleux S, Rubino C, Baudin E, Caillou B, Hartl DM, Bidart JM, et al. Prognostic factors for persistent or recurrent disease of papillary thyroid carcinoma with neck lymph node metastases and/or tumor extension beyond the thyroid capsule at initial diagnosis. J Clin Endocrinol Metab. 2005; 90:5723–9.
6. Hay ID, Bergstralh EJ, Goellner JR, Ebersold JR, Grant CS. Predicting outcome in papillary thyroid carcinoma: development of a reliable prognostic scoring system in a cohort of 1779 patients surgically treated at one institution during 1940 through 1989. Surgery. 1993; 114:1050–8.
7. Cancer Genome Atlas Research Network. Integrated genomic characterization of papillary thyroid carcinoma. Cell. 2014; 159:676–90.
8. Yoo SK, Lee S, Kim SJ, Jee HG, Kim BA, Cho H, et al. Comprehensive analysis of the transcriptional and mutational landscape of follicular and papillary thyroid cancers. PLoS Genet. 2016; 12:e1006239.
9. Song YS, Won JK, Yoo SK, Jung KC, Kim MJ, Kim SJ, et al. Comprehensive transcriptomic and genomic profiling of subtypes of follicular variant of papillary thyroid carcinoma. Thyroid. 2018; 28:1468–78.
10. Song YS, Park YJ. Genomic characterization of differentiated thyroid carcinoma. Endocrinol Metab (Seoul). 2019; 34:1–10.
11. Whiteside TL. The tumor microenvironment and its role in promoting tumor growth. Oncogene. 2008; 27:5904–12.
12. Jolly LA, Novitskiy S, Owens P, Massoll N, Cheng N, Fang W, et al. Fibroblast-mediated collagen remodeling within the tumor microenvironment facilitates progression of thyroid cancers driven by BrafV600E and Pten loss. Cancer Res. 2016; 76:1804–13.
13. Minna E, Brich S, Todoerti K, Pilotti S, Collini P, Bonaldi E, et al. Cancer associated fibroblasts and senescent thyroid cells in the invasive front of thyroid carcinoma. Cancers (Basel). 2020; 12:112.
14. Cerami E, Gao J, Dogrusoz U, Gross BE, Sumer SO, Aksoy BA, et al. The cBio cancer genomics portal: an open platform for exploring multidimensional cancer genomics data. Cancer Discov. 2012; 2:401–4.
15. Love MI, Huber W, Anders S. Moderated estimation of fold change and dispersion for RNA-seq data with DESeq2. Genome Biol. 2014; 15:550.
16. Huang da W, Sherman BT, Lempicki RA. Systematic and integrative analysis of large gene lists using DAVID bioinformatics resources. Nat Protoc. 2009; 4:44–57.
17. Barbie DA, Tamayo P, Boehm JS, Kim SY, Moody SE, Dunn IF, et al. Systematic RNA interference reveals that oncogenic KRAS-driven cancers require TBK1. Nature. 2009; 462:108–12.
18. Subramanian A, Tamayo P, Mootha VK, Mukherjee S, Ebert BL, Gillette MA, et al. Gene set enrichment analysis: a knowledge-based approach for interpreting genome-wide expression profiles. Proc Natl Acad Sci U S A. 2005; 102:15545–50.
19. Newman AM, Liu CL, Green MR, Gentles AJ, Feng W, Xu Y, et al. Robust enumeration of cell subsets from tissue expression profiles. Nat Methods. 2015; 12:453–7.
20. Newman AM, Steen CB, Liu CL, Gentles AJ, Chaudhuri AA, Scherer F, et al. Determining cell type abundance and expression from bulk tissues with digital cytometry. Nat Biotechnol. 2019; 37:773–82.
21. Luca BA, Steen CB, Matusiak M, Azizi A, Varma S, Zhu C, et al. Atlas of clinically distinct cell states and ecosystems across human solid tumors. Cell. 2021; 184:5482–96.
22. Aran D, Hu Z, Butte AJ. xCell: digitally portraying the tissue cellular heterogeneity landscape. Genome Biol. 2017; 18:220.
23. Yoshihara K, Shahmoradgoli M, Martinez E, Vegesna R, Kim H, Torres-Garcia W, et al. Inferring tumour purity and stromal and immune cell admixture from expression data. Nat Commun. 2013; 4:2612.
24. Becht E, Giraldo NA, Lacroix L, Buttard B, Elarouci N, Petitprez F, et al. Estimating the population abundance of tissue-infiltrating immune and stromal cell populations using gene expression. Genome Biol. 2016; 17:218.
25. Racle J, Gfeller D. EPIC: a tool to estimate the proportions of different cell types from bulk gene expression data. Methods Mol Biol. 2020; 2120:233–48.
26. Sahai E, Astsaturov I, Cukierman E, DeNardo DG, Egeblad M, Evans RM, et al. A framework for advancing our understanding of cancer-associated fibroblasts. Nat Rev Cancer. 2020; 20:174–86.
27. Pu W, Shi X, Yu P, Zhang M, Liu Z, Tan L, et al. Single-cell transcriptomic analysis of the tumor ecosystems underlying initiation and progression of papillary thyroid carcinoma. Nat Commun. 2021; 12:6058.
28. Bergdorf K, Ferguson DC, Mehrad M, Ely K, Stricker T, Weiss VL. Papillary thyroid carcinoma behavior: clues in the tumor microenvironment. Endocr Relat Cancer. 2019; 26:601–14.
29. Sarvaria A, Madrigal JA, Saudemont A. B cell regulation in cancer and anti-tumor immunity. Cell Mol Immunol. 2017; 14:662–74.
30. Mauri C, Bosma A. Immune regulatory function of B cells. Annu Rev Immunol. 2012; 30:221–41.
31. Hsu HM, Chu CM, Chang YJ, Yu JC, Chen CT, Jian CE, et al. Six novel immunoglobulin genes as biomarkers for better prognosis in triple-negative breast cancer by gene co-expression network analysis. Sci Rep. 2019; 9:4484.
32. Knauf JA, Sartor MA, Medvedovic M, Lundsmith E, Ryder M, Salzano M, et al. Progression of BRAF-induced thyroid cancer is associated with epithelial-mesenchymal transition requiring concomitant MAP kinase and TGFb signaling. Oncogene. 2011; 30:3153–62.
33. Dong C, Davis RJ, Flavell RA. MAP kinases in the immune response. Annu Rev Immunol. 2002; 20:55–72.
34. Fruman DA, Chiu H, Hopkins BD, Bagrodia S, Cantley LC, Abraham RT. The PI3K pathway in human disease. Cell. 2017; 170:605–35.
Fig. 1.
Transcriptomic profiles of BRAF-like papillary thyroid carcinoma (BL-PTC) and RAS-like papillary thyroid carcinoma (RL-PTC) according to the clinicopathological factors. (A) Volcano plots showing differentially expressed genes (DEGs) of BL-PTCs (left) and RL-PTCs (right) between aggressive and indolent clinicopathological characteristics. (B) Venn diagrams displaying the number of overlapping and non-overlapping DEGs of BL-PTCs (red) and RL-PTCs (blue) according to the clinicopathological factors. ETE, extrathyroidal extension; LNM, lymph node metastasis; TNM, tumor-node-metastasis; MACIS, metastasis-age-completeness of resection-invasion-size.
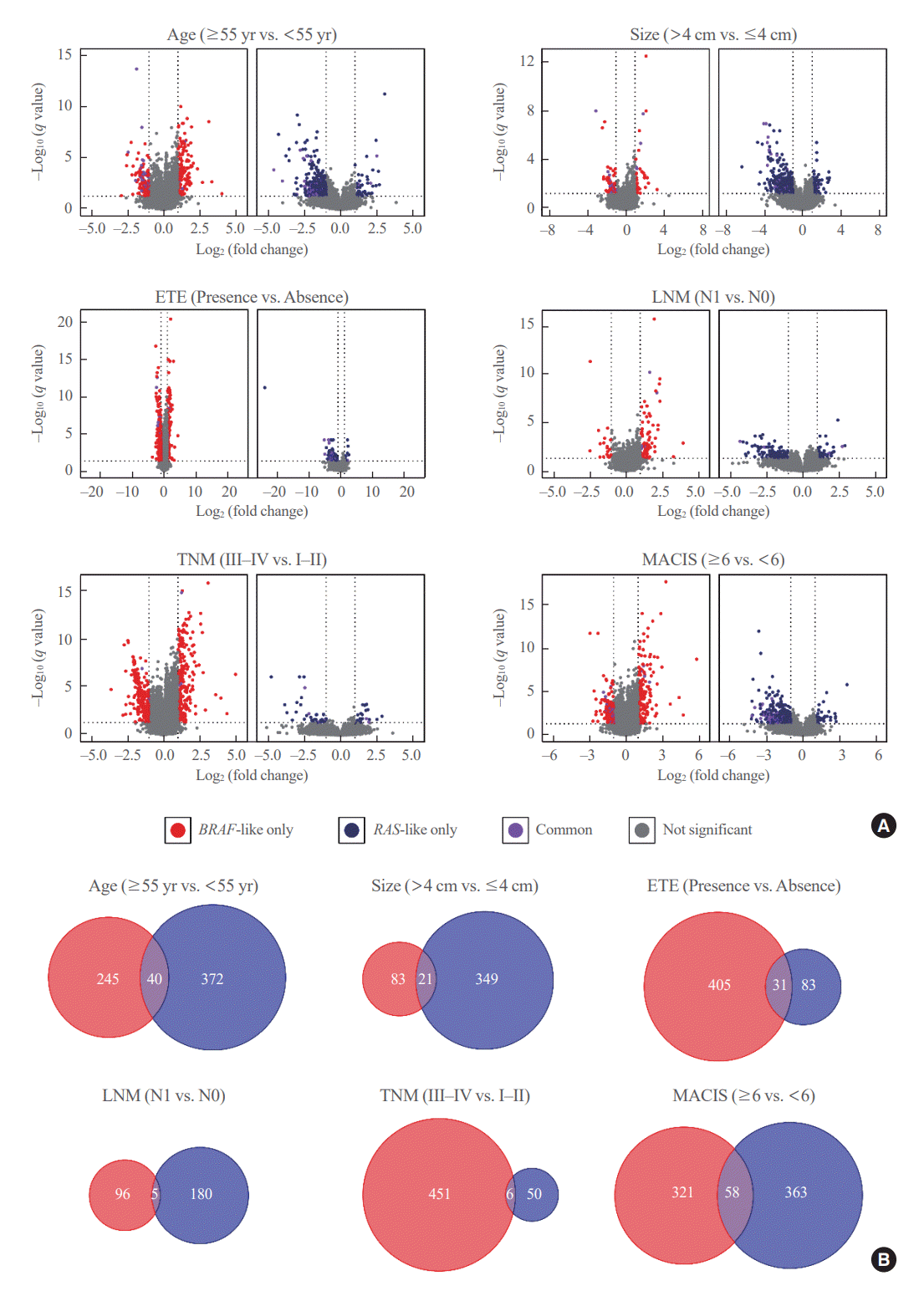
Fig. 2.
Enriched functional annotations of BRAF-like papillary thyroid carcinoma (BL-PTC) and RAS-like papillary thyroid carcinoma (RLPTC) according to the clinicopathological factors. Bubble charts showing the top 10 significantly enriched Gene Ontology (GO) terms and Kyoto Encyclopedia of Genes and Genomes (KEGG) pathways in (A) upregulated differentially expressed genes (DEGs) of BL-PTCs, (B) downregulated DEGs of all BL-PTCs (left) and BL-PTCs without thyroiditis (right), and (C) downregulated DEGs of all RL-PTCs (left) and RL-PTCs without thyroiditis (right). FDR, false discovery rate; ECM, extracellular matrix; RNAPII, RNA polymerase II; ETE, extrathyroidal extension; LNM, lymph node metastasis; TNM, tumor, node, metastasis; MACIS, metastasis-age-completeness of resection-invasion-size.
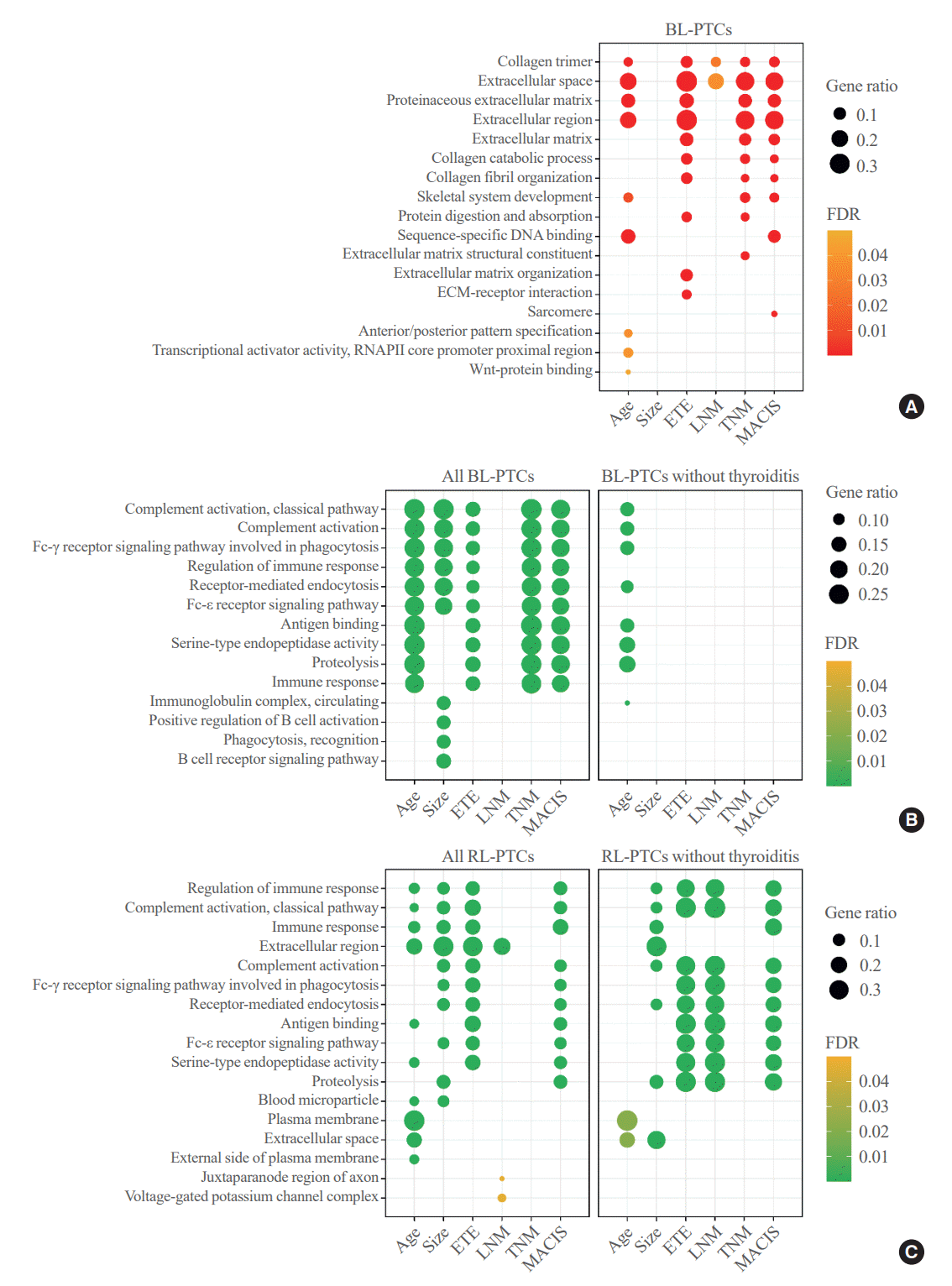
Fig. 3.
Tumor microenvironment of BRAF-like papillary thyroid carcinoma (BL-PTC) and RAS-like papillary thyroid carcinoma (RL-PTC). (A) Extracellular matrix (ECM) score and (B) abundance of cancer-associated fibroblasts (CAFs) and normal fibroblasts in BL-PTCs (upper) and RL-PTCs (lower) between aggressive and indolent clinicopathological characteristics. ETE, extrathyroidal extension; LNM, lymph node metastasis; TNM, tumor, node, metastasis; MACIS, metastasis-age-completeness of resection-invasion-size; NS, not significant (P≥0.05). aP<0.05; bP<0.01; cP<0.001; dP<0.0001.
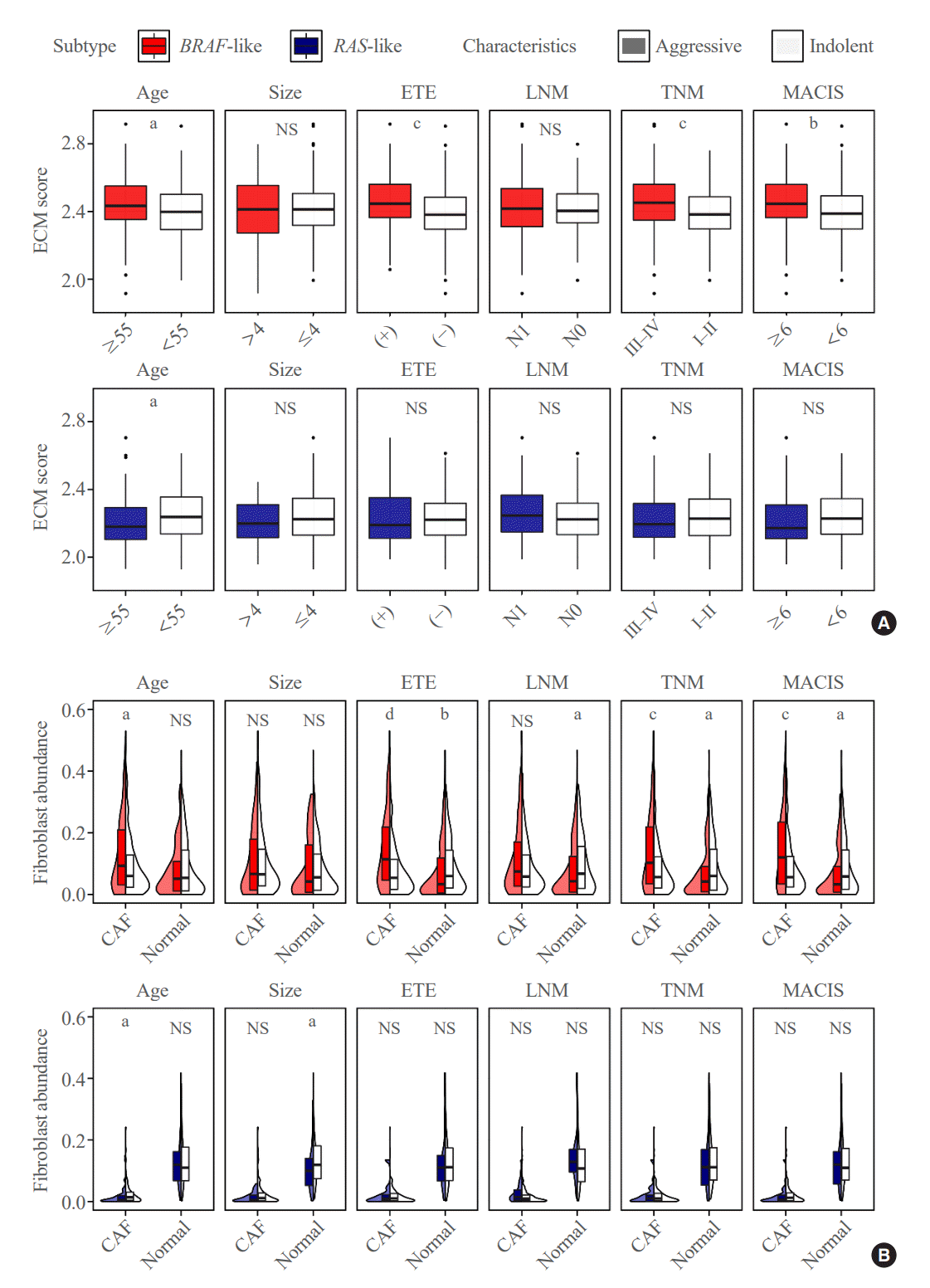