Dear Editor,
Vancomycin is used to treat methicillin-resistant Staphylococcus aureus (MRSA) infections [1]. Administering vancomycin at concentrations below the minimum effective concentration leads to treatment failure, whereas administering concentrations above the minimum toxic concentration can result in acute kidney injury (AKI). Therefore, therapeutic drug monitoring (TDM) of vancomycin is crucial in personalized medicine [2].
In TDM of vancomycin, the area under the curve (AUC)/minimum inhibitory concentration (MIC) is recommended as the best pharmacokinetic and pharmacodynamic (PK/PD) parameter [3-5]. In serious MRSA infections, an AUC/MIC of 400–600 (assuming a vancomycin MIC of 1 mg/L) is considered the PK/PD efficacy target [5]. As it is difficult to calculate the AUC in clinical practice, a trough concentration of 15–20 mg/L has been used as a surrogate marker for vancomycin TDM [4]. However, the trough does not accurately reflect the AUC and even the therapeutic trough may cause AKI [5]. TDM software to calculate the AUC has been developed. Bayesian dose-optimizing TDM software allows reliable AUC and optimal vancomycin dose estimation [5]. However, it requires a large amount of manual data input, which is repetitive, time-consuming, and labor-intensive, increasing the risk of clerical error and limiting its clinical utility [6]. Reducing manual data entry may increase the utility of AUC-guided dosing in clinical practice.
MwPharm++ (Mediware, Prague, Czech Republic) is a Bayesian TDM software that ranked first in benchmarking studies because of its user-friendly interface and excellent PK calculation function [7, 8]. Between October 2020 to April 2021, we developed an automated data transfer system (ADTS) for automated data entry into MwPharm++ and evaluated improvements in work efficiency. The ADTS consists of an interface that extracts information for AUC calculation from the electronic health record (EHR) and a macro software that automatically inputs the extracted information into MwPharm++.
Before the ADTS was introduced, we reviewed the EHRs of patients who required vancomycin TDM and summarized the data into MwPharm++. The appropriateness of the patients’ PK parameters calculated by MwPharm++ was reviewed. Subsequently, doses and administration intervals were adjusted and simulated to match the target AUC/MIC. EHR review and data entry were manual and accounted for more than 95% of the entire process time. After data entry into MwPharm++, calculation of the AUC/MIC takes 10–30 seconds. The entire process took at least 15 minutes per patient, with most time spent on data entry.
As the revised consensus guidelines for TDM of vancomycin recommend using AUC/MIC monitoring instead of trough-only monitoring [5], we expected the demand for AUC-based TDM reports to increase. Therefore, we developed an ADTS for automated data entry into MwPharm++ and evaluated improvements in work efficiency. The ADTS was introduced in April 2021. The interface software of the ADTS extracts information required for AUC calculation, including patient identifier, name, race, sex, age, height, weight, serum creatinine concentration, vancomycin trough concentration, and vancomycin dosing history, from the EHR (Fig. 1A) and saves it in a.txt file (Fig. 1B). We loaded the patient and laboratory information closest in time to the last sampling date for vancomycin trough concentration determination. We developed a C++ language-based keyboard/mouse macro software (macro, Fig. 1C), which automatically enters the extracted information into MwPharm++, using Microsoft Visual Studio (Microsoft, Redmond, WA, USA). Upon executing the macro, information in the.txt file is sequentially entered into MwPharm++, which then automatically calculates individual PK parameters of patients. After execution of the macro, we reviewed patient clinical conditions in the EHRs and confirmed the expected AUC. This process required only 2–3 minutes per patient. The cost of developing the ADTS was 10,000,000 KRW.
To automate data input into the Bayesian software, it is ideal to directly interface EHRs with the TDM software. TUCUXI and AutoKinetics realize this by establishing a direct EHR interface [9, 10]. However, hospitals have different means of storing and managing medical information; therefore, it is difficult to directly interface EHRs with TDM software in a similar manner in every institution, and this process requires extensive support from TDM software manufacturers. Moreover, as most TDM software programs are developed outside Korea, technical support is limited for domestic users. Therefore, there are practical difficulties in automatic data entry via direct interfacing of TDM software and EHRs. Hence, we chose to develop a system that automates the repetitive process of data input into MwPharm++ using a third-party program developer.
In conclusion, we developed an ADTS for prompt calculation of the AUC for vancomycin TDM. This system reduces the report preparation time per patient by at least one-third. Sharing our experience will help other hospitals with similar challenges find solutions for AUC-guided dosing.
ACKNOWLEDGEMENTS
The authors thank the staff at the Department of Laboratory Medicine of Asan Medical Center for their hard work in sample handling and data management.
Notes
AUTHOR CONTRIBUTIONS
Conceptualization: Min WK; Methodology: Lee W and Chun S; Data curation: Lee S and Yoon S; Writing—original draft preparation: Lee S; Writing—review and editing: Kim S and Kim HK; Supervision: Chun S and Min WK.
All authors have accepted responsibility for the entire content of this manuscript and approved its submission.
REFERENCES
1. Koh YR, Kim KH, Chang CL, Yi J. 2016; Prevalence and clinical impact of heterogeneous vancomycin-intermediate Staphylococcus aureus isolated from hospitalized patients. Ann Lab Med. 36:235–43. DOI: 10.3343/alm.2016.36.3.235. PMID: 26915612. PMCID: PMC4773264.


2. Monteiro JF, Hahn SR, Gonçalves J, Fresco P. 2018; Vancomycin therapeutic drug monitoring and population pharmacokinetic models in special patient subpopulations. Pharmacol Res Perspect. 6:e00420. DOI: 10.1002/prp2.420. PMID: 30156005. PMCID: PMC6113434.


3. Álvarez R, López Cortés LE, Molina J, Cisneros JM, Pachón J. 2016; Optimizing the clinical use of vancomycin. Antimicrob Agents Chemother. 60:2601–9. DOI: 10.1128/AAC.03147-14. PMID: 26856841. PMCID: PMC4862470.


4. Rybak M, Lomaestro B, Rotschafer JC, Moellering R Jr, Craig W, Billeter M, et al. 2009; Therapeutic monitoring of vancomycin in adult patients: a consensus review of the American Society of Health-System Pharmacists, the Infectious Diseases Society of America, and the Society of Infectious Diseases Pharmacists. Am J Health Syst Pharm. 66:82–98. DOI: 10.2146/ajhp080434. PMID: 19106348.


5. Rybak MJ, Le J, Lodise TP, Levine DP, Bradley JS, Liu C, et al. 2020; Therapeutic monitoring of vancomycin for serious methicillin-resistant Staphylococcus aureus infections: a revised consensus guideline and review by the American Society of Health-System Pharmacists, the Infectious Diseases Society of America, the Pediatric Infectious Diseases Society, and the Society of Infectious Diseases Pharmacists. Am J Health Syst Pharm. 77:835–64. DOI: 10.1093/ajhp/zxaa036. PMID: 32191793.
6. Van Der Heggen T, Buyle FM, Claus B, Somers A, Schelstraete P, De Paepe P, et al. 2021; Vancomycin dosing and therapeutic drug monitoring practices: guidelines versus real-life. Int J Clin Pharm. 43:1394–403. DOI: 10.1007/s11096-021-01266-7. PMID: 33913087.


7. Fuchs A, Csajka C, Thoma Y, Buclin T, Widmer N. 2013; Benchmarking therapeutic drug monitoring software: a review of available computer tools. Clin Pharmacokinet. 52:9–22. DOI: 10.1007/s40262-012-0020-y. PMID: 23196713.


8. Kantasiripitak W, Van Daele R, Gijsen M, Ferrante M, Spriet I, Dreesen E. 2020; Software tools for model-informed precision dosing: how well do they satisfy the needs? Front Pharmacol. 11:620. DOI: 10.3389/fphar.2020.00620. PMID: 32457619. PMCID: PMC7224248.


9. Dubovitskaya A, Buclin T, Schumacher M, Aberer K, Thoma Y. 2017. TUCUXI: an intelligent system for personalized medicine from individualization of treatments to research databases and back. Proceedings of the 8th ACM International Conference on Bioinformatics, Computational Biology, and Health Informatics. p. 223–32.
10. Roggeveen LF, Guo T, Driessen RH, Fleuren LM, Thoral P, van der Voort PHJ, et al. 2020; Right dose, right now: development of autokinetics for real time model informed precision antibiotic dosing decision support at the bedside of critically ill patients. Front Pharmacol. 11:646. DOI: 10.3389/fphar.2020.00646. PMID: 32499697. PMCID: PMC7243359.


Fig. 1
Automated data transference system at Asan Medical Center. (A) The interface software. This software loads patient information, laboratory information, and drug dosing history from electronic health records, which are essential to calculate the area under curve in MwPharm++. (B) The data are exported to two text files by clicking the “Generate .txt” button. (C) In-house keyboard-mouse macro software. By clicking the “Start” button, this software automatically inputs the data in .txt files into MwPharm++. (D) A screenshot of MwPharm++. The data described in Figure 1B were automatically entered into MwPharm++ through the macro software.
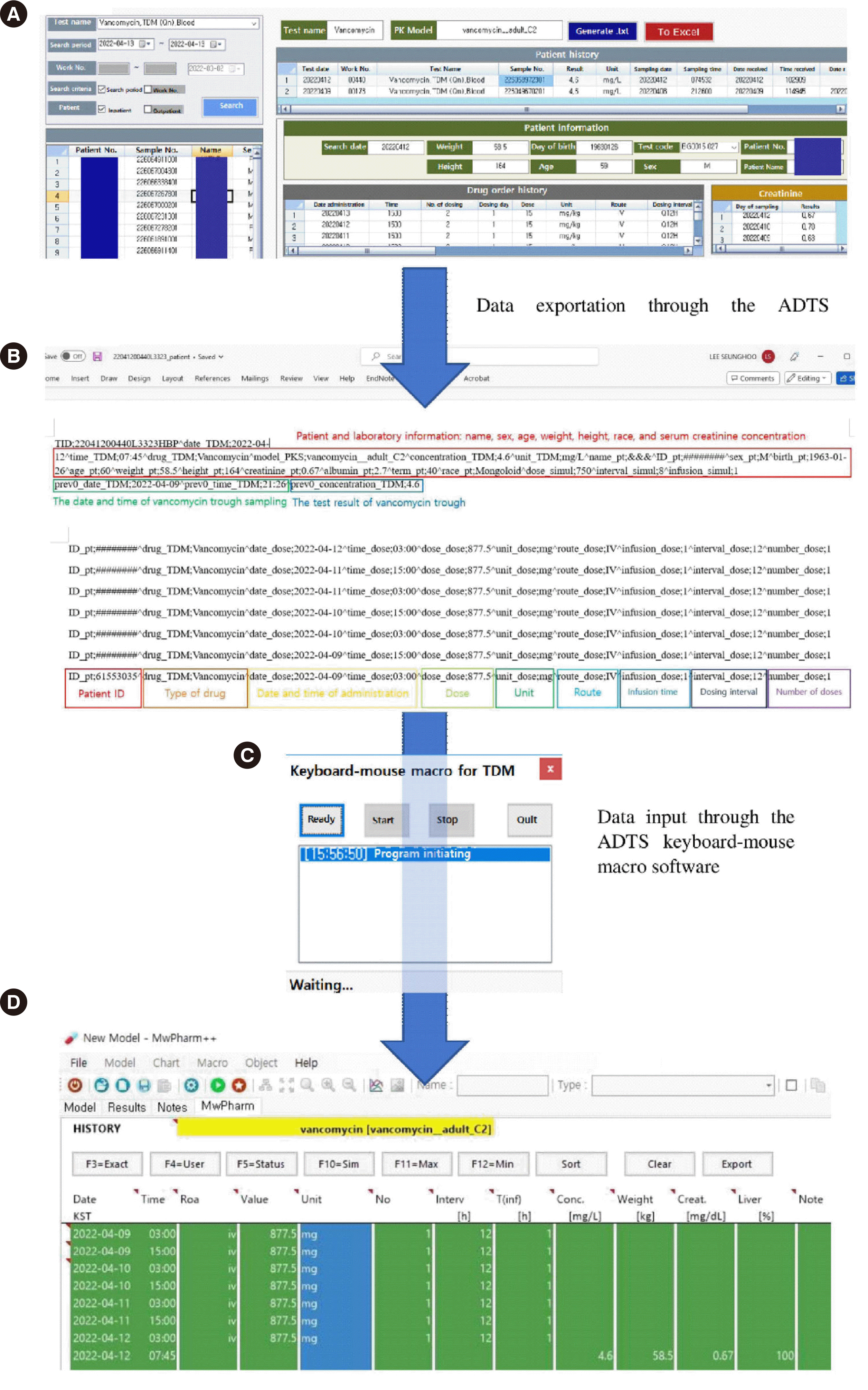