Abstract
Background
Methods
Results
Notes
AUTHOR CONTRIBUTIONS
Albert C, Albert A, Bellomo R, and Braun-Dullaeus RC contributed to the conception and design of the study. Haase M and Albert C acquired patient data. Albert C and Albert A performed formal analysis, interpretation and illustration of the data, and wrote the first draft of the manuscript. Albert C supervised the study. All authors participated in drafting and/or revising the paper and provided intellectual content of critical importance to the work, read and approved the final manuscript for publication, and agreed to be accountable for all aspects of the work in ensuring that questions related to the accuracy or integrity of any part of the work are appropriately investigated and resolved.
CONFLICTS OF INTEREST
Albert A has received honoraria speaking for Abbott Diagnostics on unrelated work. Haase M has received honoraria speaking for Abbott Diagnostics, Alere, Biosite Inc., and Siemens Healthineers. Albert C has received honoraria speaking for Siemens Healthineers. All other authors declare no potential conflicts of interest with respect to the research, authorship, and/or publication of this article.
REFERENCES



























Fig. 1
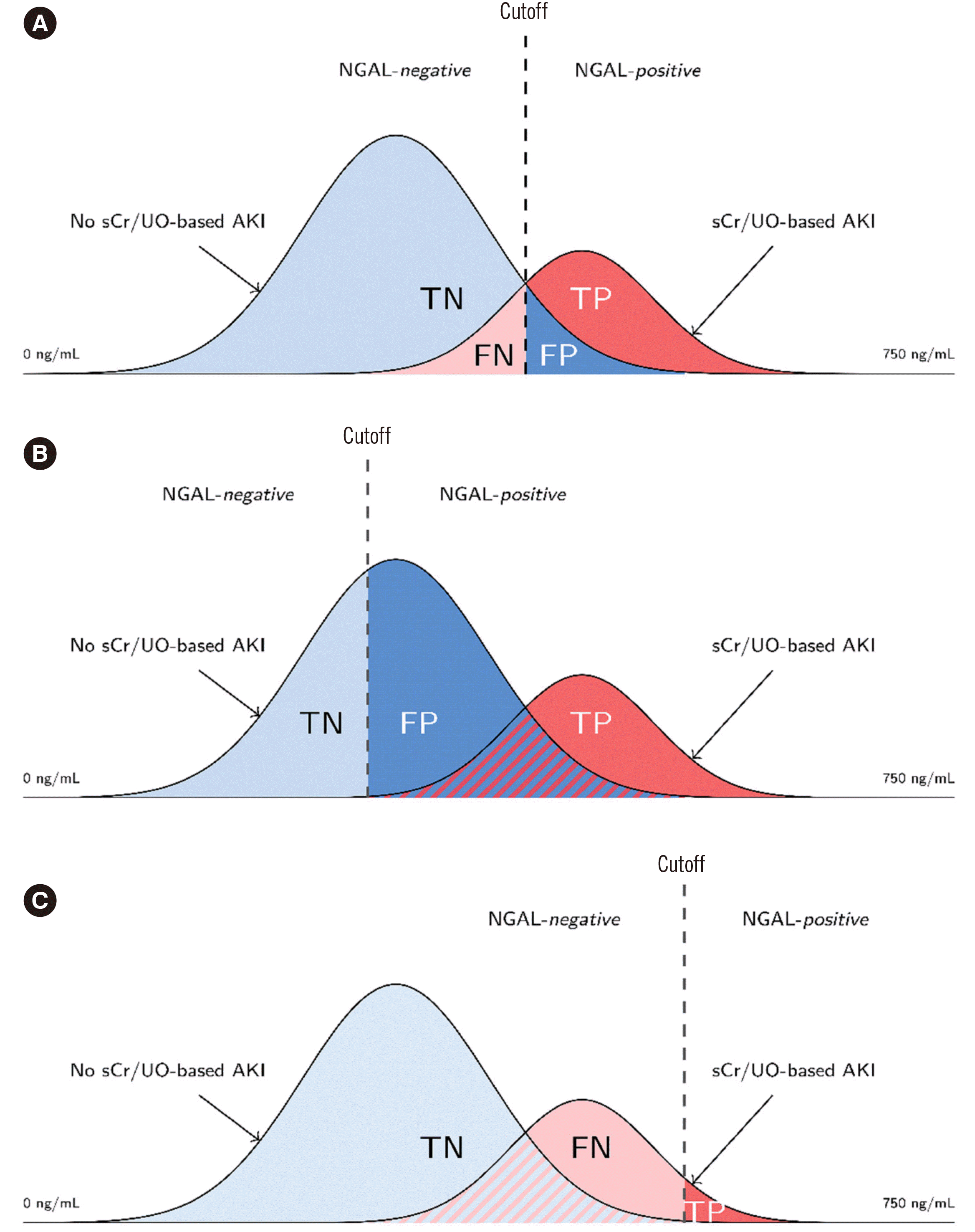
Fig. 2
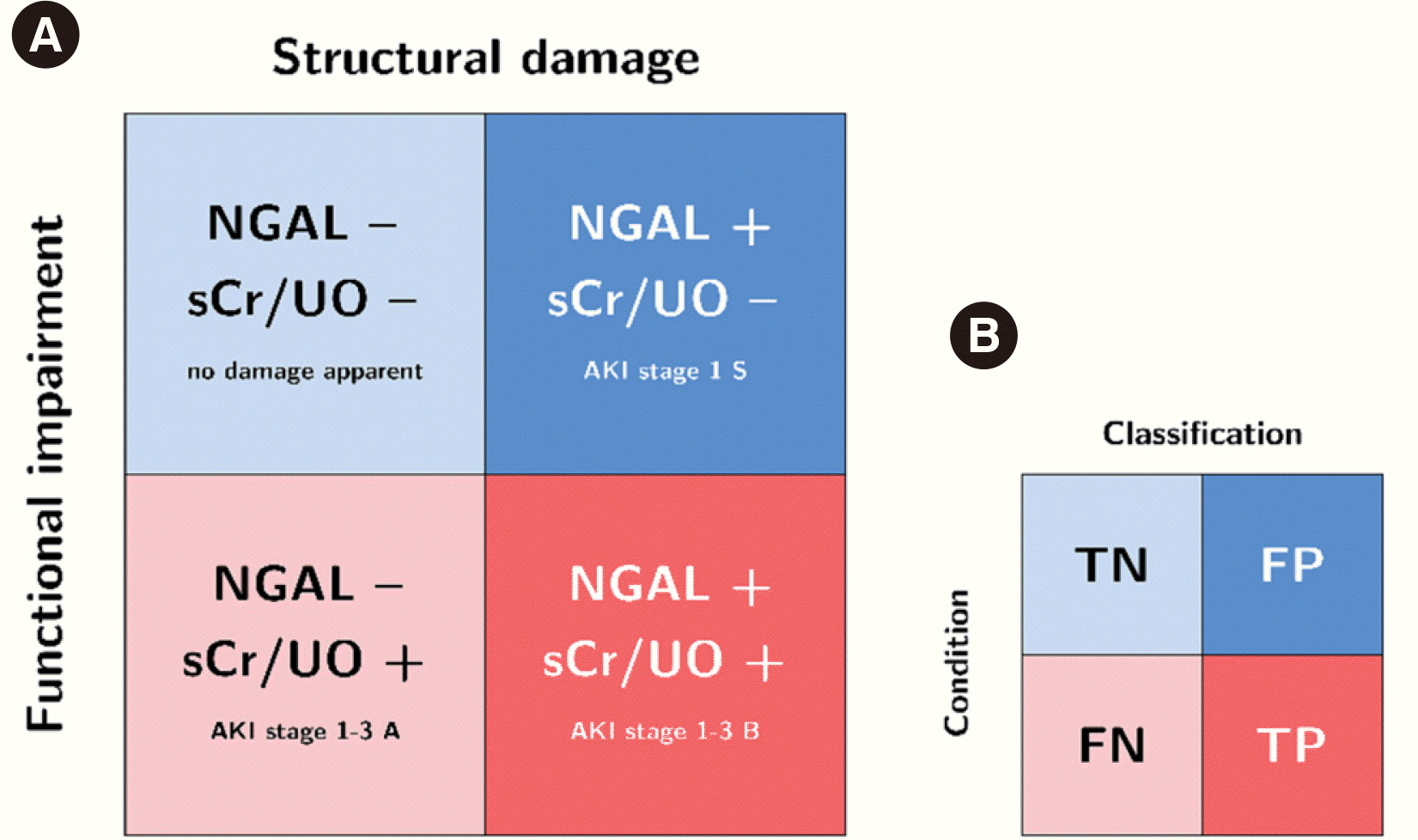
Fig. 3
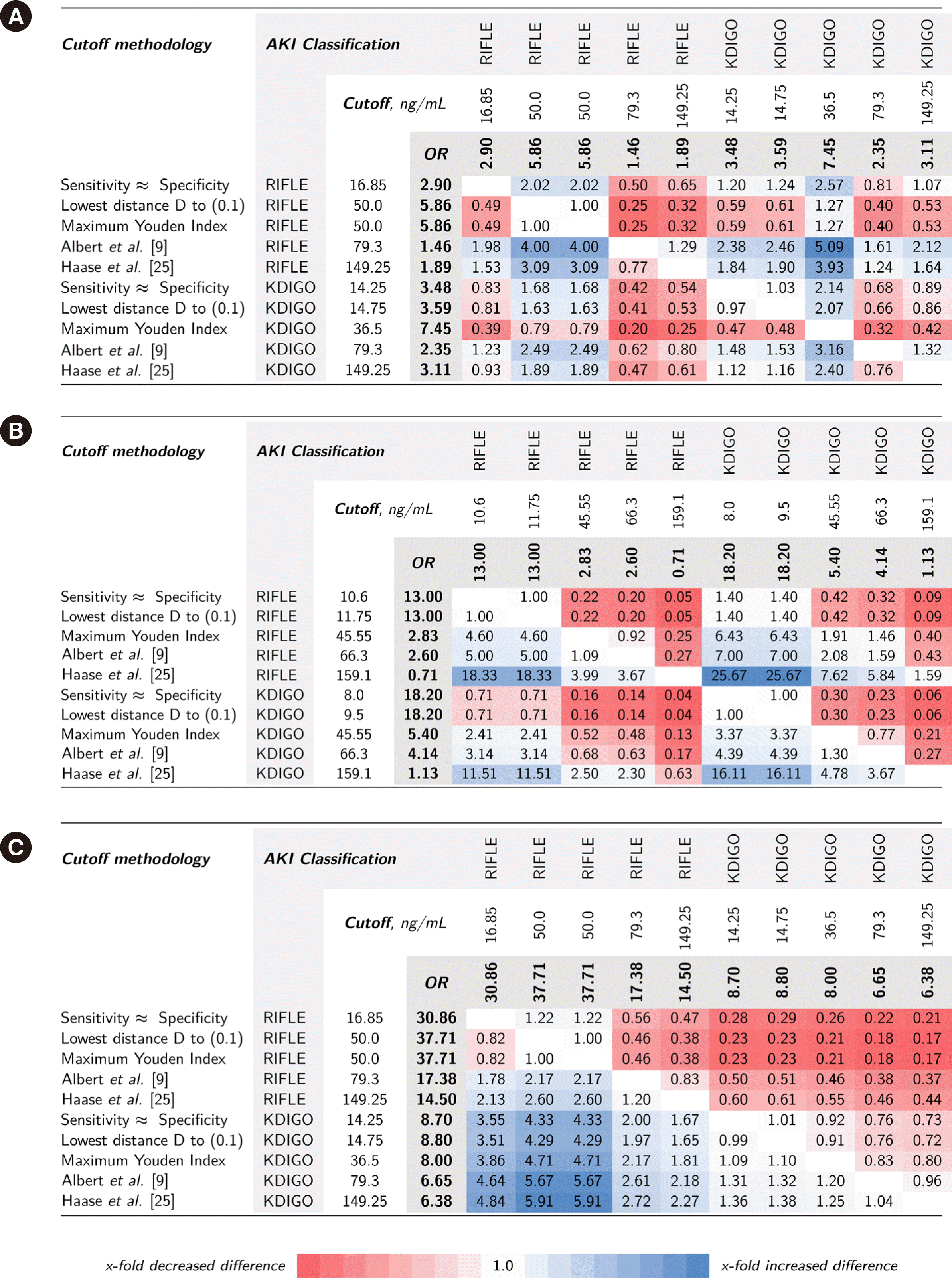
Table 1
Methodology | NGAL cutoff*, ng/mL | Sensitivity | Specificity | D† | Youden J‡ | DOR (95% CI) | LR+ (95% CI) | LR– (95% CI) | PPV (95% CI) | NPV (95% CI) | AKI prevalence, % |
---|---|---|---|---|---|---|---|---|---|---|---|
Magdeburg cohort | |||||||||||
|
|||||||||||
RIFLE AKI classification used as dependent outcome measure | |||||||||||
Sensitivity ≈ specificity | 10.6 | 0.714 | 0.709 | 0.408 | 0.423 | 6.10 (1.75–21.28) | 2.46 (1.54–3.92) | 0.40 (0.17–0.93) | 0.29 (0.14–0.44) | 0.94 (0.88–1.00) | 14.0 |
Lowest distance D to (0,1) | 11.75 | 0.714 | 0.733 | 0.391 | 0.447 | 6.85 (1.95–24.00) | 2.67 (1.65–4.32) | 0.39 (0.17–0.90) | 0.30 (0.15–0.46) | 0.94 (0.88–1.00) | 14.0 |
Maximum Youden index | 45.55 | 0.500 | 0.953 | 0.502 | 0.453 | 20.50 (4.80–87.46) | 10.75 (3.61–32.00) | 0.52 (0.31–0.89) | 0.64 (0.35–0.92) | 0.92 (0.87–0.98) | 14.0 |
Albert, et al. [9]§ | 66.3 | 0.357 | 0.965 | 0.644 | 0.322 | 15.37 (3.14–75.23) | 10.24 (2.75–38.14) | 0.67 (0.45–0.99) | 0.63 (0.29–0.96) | 0.90 (0.84–0.96) | 14.0 |
Haase, et al. [25]|| | 159.1 | 0.214 | 0.965 | 0.787 | 0.179 | 7.55 (1.35–42.12) | 6.14 (1.37–27.46) | 0.81 (0.62–1.07) | 0.50 (0.10–0.90) | 0.88 (0.82–0.95) | 14.0 |
KDIGO AKI classification used as dependent outcome measure | |||||||||||
Sensitivity ≈ specificity | 8.0 | 0.565 | 0.571 | 0.611 | 0.136 | 1.73 (0.68–4.44) | 1.32 (0.85–2.05) | 0.76 (0.46–1.26) | 0.28 (0.15–0.41) | 0.81 (0.71–0.92) | 23.0 |
Lowest distance D to (0,1) | 9.5 | 0.565 | 0.688 | 0.535 | 0.253 | 2.87 (1.10–7.46) | 1.81 (1.11–2.96) | 0.63 (0.39–1.03) | 0.35 (0.20–0.51) | 0.84 (0.75–0.93) | 23.0 |
Maximum Youden index | 45.55 | 0.348 | 0.961 | 0.653 | 0.309 | 13.16 (3.12–55.43) | 8.93 (2.58–30.93) | 0.68 (0.50–0.92) | 0.73 (0.46–0.99) | 0.83 (0.75–0.91) | 23.0 |
Albert, et al. [9]§ | 66.3 | 0.261 | 0.974 | 0.739 | 0.235 | 13.24 (2.46–71.35) | 10.04 (2.17–46.43) | 0.76 (0.59–0.97) | 0.75 (0.45–1.05) | 0.82 (0.74–0.89) | 23.0 |
Haase, et al. [25]|| | 159.1 | 0.174 | 0.974 | 0.826 | 0.148 | 7.89 (1.34–46.37) | 6.70 (1.31–34.25) | 0.85 (0.70–1.03) | 0.67 (0.29–1.04) | 0.80 (0.72–0.88) | 23.0 |
|
|||||||||||
Berlin cohort | |||||||||||
|
|||||||||||
RIFLE AKI classification used as dependent outcome measure | |||||||||||
Sensitivity ≈ specificity | 16.85 | 0.625 | 0.623 | 0.532 | 0.248 | 2.75 (1.14–6.64) | 1.66 (1.15–2.38) | 0.60 (0.35–1.02) | 0.19 (0.10–0.27) | 0.92 (0.88–0.97) | 12.1 |
Lowest distance to (1,0) | 50.0 | 0.625 | 0.771 | 0.439 | 0.396 | 5.28 (2.15–12.93) | 2.60 (1.73–3.91) | 0.49 (0.29–0.83) | 0.26 (0.15–0.38) | 0.94 (0.90–0.98) | 12.1 |
Maximum Youden index | 50.0 | 0.625 | 0.771 | 0.439 | 0.396 | 5.28 (2.15–12.93) | 2.60 (1.73–3.91) | 0.49 (0.29–0.83) | 0.26 (0.15–0.38) | 0.94 (0.90–0.98) | 12.1 |
Albert, et al. [9]§ | 79.3 | 0.542 | 0.811 | 0.495 | 0.353 | 5.09 (2.09–12.36) | 2.87 (1.78–4.64) | 0.56 (0.36–0.88) | 0.28 (0.15–0.41) | 0.93 (0.89–0.97) | 12.1 |
Haase, et al. [25]|| | 149.25 | 0.458 | 0.846 | 0.563 | 0.304 | 4.64 (1.88–11.43) | 2.97 (1.70–5.18) | 0.64 (0.44–0.93) | 0.29 (0.15–0.43) | 0.92 (0.88–0.96) | 12.1 |
KDIGO AKI classification used as dependent outcome measure | |||||||||||
Sensitivity ≈ specificity | 14.25 | 0.627 | 0.629 | 0.526 | 0.256 | 2.85 (1.52–5.34) | 1.69 (1.26–2.26) | 0.59 (0.42–0.85) | 0.42 (0.31–0.52) | 0.80 (0.73–0.87) | 29.7 |
Lowest distance to (1,0) | 14.75 | 0.627 | 0.636 | 0.521 | 0.263 | 2.93 (1.56–5.51) | 1.72 (1.28–2.31) | 0.59 (0.41–0.84) | 0.42 (0.32–0.52) | 0.80 (0.73–0.88) | 29.7 |
Maximum Youden index | 36.5 | 0.508 | 0.779 | 0.539 | 0.287 | 3.64 (1.90–6.95) | 2.30 (1.54–3.42) | 0.63 (0.48–0.83) | 0.49 (0.37–0.62) | 0.79 (0.72–0.86) | 29.7 |
Albert, et al. [9]§ | 79.3 | 0.356 | 0.821 | 0.668 | 0.177 | 2.54 (1.28–5.05) | 1.99 (1.22–3.27) | 0.78 (0.64–0.96) | 0.46 (0.31–0.60) | 0.75 (0.68–0.82) | 29.7 |
Haase, et al. [25]|| | 149.25 | 0.305 | 0.857 | 0.710 | 0.162 | 2.63 (1.27–5.46) | 2.14 (1.22–3.74) | 0.81 (0.68–0.97) | 0.47 (0.31–0.63) | 0.75 (0.68–0.81) | 29.7 |
*Considered a positive result if greater than or equal to the cutoff; †D2=(1–TPF)2+FPF2=(1–sensitivity)2+(1–specificity)2; ‡Youden index (J) defined as TPF–FPF=sensitivity+specificity−1; §We chose the value closest to that suggested by Albert, et al. [9] (81 ng/mL); ||We chose the value closest to that suggested by Haase, et al. [25] (150 ng/mL).
Abbreviations: AKI, acute kidney injury; KDIGO, Kidney Disease Improving Global Outcomes; NGAL, neutrophil gelatinase-associated lipocalin; NPV, negative predictive value; PPV, positive predictive value; RIFLE, Risk, Injury, Failure, Loss, End-stage; TPF, true positive fraction; FPF, false positive fraction.
Table 2
Cutoff methodology | sCr/UO-AKI | NGAL cutoff* (ng/mL) | NGAL(–)/ sCr/UO(–)† | NGAL(+)/ sCr/UO(–)‡ | NGAL(–)/ sCr/UO(+)§ | NGAL(+)/ sCr/UO(+)ll |
---|---|---|---|---|---|---|
Allocation | ||||||
|
||||||
Magdeburg cohort | ||||||
Sensitivity ≈ specificity | RIFLE | 10.6 | 61 (61.0) | 25 (25.0) | 4 (4.0) | 10 (10.0) |
Lowest distance D to (0,1) | RIFLE | 11.75 | 63 (63.0) | 23 (23.0) | 4 (4.0) | 10 (10.0) |
Maximum Youden index | RIFLE | 45.55 | 82 (82.0) | 4 (4.0) | 7 (7.0) | 7 (7.0) |
Albert, et al. [9]¶ | RIFLE | 66.3 | 83 (83.0) | 3 (3.0) | 9 (9.0) | 5 (5.0) |
Haase, et al. [25]** | RIFLE | 159.1 | 83 (83.0) | 3 (3.0) | 11 (11.0) | 3 (3.0) |
Sensitivity ≈ specificity | KDIGO | 8.0 | 44 (44.0) | 33 (33.0) | 10 (10.0) | 13 (13.0) |
Lowest distance D to (0,1) | KDIGO | 9.5 | 53 (53.0) | 24 (24.0) | 10 (10.0) | 13 (13.0) |
Maximum Youden index | KDIGO | 45.55 | 74 (74.0) | 3 (3.0) | 15 (15.0) | 8 (8.0) |
Albert, et al. [9]¶ | KDIGO | 66.3 | 75 (75.0) | 2 (2.0) | 17 (17.0) | 6 (6.0) |
Haase, et al. [25]** | KDIGO | 159.1 | 75 (75.0) | 2 (2.0) | 19 (19.0) | 4 (4.0) |
Berlin cohort | ||||||
Sensitivity ≈ specificity | RIFLE | 16.85 | 109 (54.8) | 66 (33.2) | 9 (4.5) | 15 (7.5) |
Lowest distance D to (0,1) | RIFLE | 50.0 | 133 (66.8) | 42 (21.1) | 9 (4.5) | 15 (7.5) |
Maximum Youden index | RIFLE | 50.0 | 133 (66.8) | 42 (21.1) | 9 (4.5) | 15 (7.5) |
Albert, et al. [9]¶ | RIFLE | 79.3 | 142 (71.4) | 33 (16.6) | 11 (5.5) | 13 (6.5) |
Haase, et al. [25]** | RIFLE | 149.25 | 148 (74.4) | 27 (13.6) | 13 (6.5) | 11 (5.5) |
Sensitivity ≈ specificity | KDIGO | 14.25 | 88 (44.2) | 52 (26.1) | 22 (11.1) | 37 (18.6) |
Lowest distance D to (0,1) | KDIGO | 14.75 | 89 (44.7) | 51 (25.6) | 22 (11.1) | 37 (18.6) |
Maximum Youden index | KDIGO | 36.5 | 109 (54.8) | 31 (15.6) | 29 (14.6) | 30 (15.1) |
Albert, et al. [9]¶ | KDIGO | 79.3 | 115 (57.8) | 25 (12.6) | 38 (19.1) | 21 (10.6) |
Haase, et al. [25]** | KDIGO | 149.25 | 120 (60.3) | 20 (10.1) | 41 (20.6) | 18 (9.1) |
|
||||||
KRT initiation | ||||||
|
||||||
Magdeburg cohort | ||||||
Sensitivity ≈ specificity | RIFLE | 10.6 | 0 (0.0) | 0 (0.0) | 0 (0.0) | 6 (60.0) |
Lowest distance D to (0,1) | RIFLE | 11.75 | 0 (0.0) | 0 (0.0) | 0 (0.0) | 6 (60.0) |
Maximum Youden index | RIFLE | 45.55 | 0 (0.0) | 0 (0.0) | 2 (28.6) | 4 (57.1) |
Albert, et al. [9]¶ | RIFLE | 66.3 | 0 (0.0) | 0 (0.0) | 3 (33.3) | 3 (60.0) |
Haase, et al. [25] ** | RIFLE | 159.1 | 0 (0.0) | 0 (0.0) | 5 (45.5) | 1 (33.3) |
Sensitivity ≈ specificity | KDIGO | 8.0 | 0 (0.0) | 0 (0.0) | 0 (0.0) | 6 (46.2) |
Lowest distance D to (0,1) | KDIGO | 9.5 | 0 (0.0) | 0 (0.0) | 0 (0.0) | 6 (46.2) |
Maximum Youden index | KDIGO | 45.55 | 0 (0.0) | 0 (0.0) | 2 (13.3) | 4 (50.0) |
Albert, et al. [9]¶ | KDIGO | 66.3 | 0 (0.0) | 0 (0.0) | 3 (17.6) | 3 (50.0) |
Haase, et al. [25]** | KDIGO | 159.1 | 0 (0.0) | 0 (0.0) | 5 (26.3) | 1 (25.0) |
Berlin cohort | ||||||
Sensitivity ≈ specificity | RIFLE | 16.85 | 2 (1.8) | 3 (4.5) | 1 (11.1) | 7 (46.7) |
Lowest distance D to (0,1) | RIFLE | 50.0 | 2 (1.5) | 3 (7.1) | 1 (11.1) | 7 (46.7) |
Maximum Youden index | RIFLE | 50.0 | 2 (1.5) | 3 (7.1) | 1 (11.1) | 7 (46.7) |
Albert, et al. [9]¶ | RIFLE | 79.3 | 4 (2.8) | 1 (3.0) | 1 (9.1) | 7 (53.8) |
Haase, et al. [25]** | RIFLE | 149.25 | 4 (2.7) | 1 (3.7) | 2 (15.4) | 6 (54.5) |
Sensitivity ≈ specificity | KDIGO | 14.25 | 0 (0.0) | 0 (0.0) | 3 (13.6) | 10 (27.0) |
Lowest distance D to (0,1) | KDIGO | 14.75 | 0 (0.0) | 0 (0.0) | 3 (13.6) | 10 (27.0) |
Maximum Youden index | KDIGO | 36.5 | 0 (0.0) | 0 (0.0) | 3 (10.3) | 10 (33.3) |
Albert, et al. [9]¶ | KDIGO | 79.3 | 0 (0.0) | 0 (0.0) | 5 (13.2) | 8 (38.1) |
Haase, et al. [25]** | KDIGO | 149.25 | 0 (0.0) | 0 (0.0) | 6 (14.6) | 7 (38.9) |
|
||||||
In-hospital mortality | ||||||
|
||||||
Magdeburg cohort | ||||||
Sensitivity ≈ specificity | RIFLE | 10.6 | 0 (0.0) | 0 (0.0) | 0 (0.0) | 3 (30.0) |
Lowest distance D to (0,1) | RIFLE | 11.75 | 0 (0.0) | 0 (0.0) | 0 (0.0) | 3 (30.0) |
Maximum Youden index | RIFLE | 45.55 | 0 (0.0) | 0 (0.0) | 1 (14.3) | 2 (28.6) |
Albert, et al. [9]¶ | RIFLE | 66.3 | 0 (0.0) | 0 (0.0) | 2 (22.2) | 1 (20.0) |
Haase, et al. [25]** | RIFLE | 159.1 | 0 (0.0) | 0 (0.0) | 2 (18.2) | 1 (33.3) |
Sensitivity ≈ specificity | KDIGO | 8.0 | 0 (0.0) | 0 (0.0) | 0 (0.0) | 3 (23.1) |
Lowest distance D to (0,1) | KDIGO | 9.5 | 0 (0.0) | 0 (0.0) | 0 (0.0) | 3 (23.1) |
Maximum Youden index | KDIGO | 45.55 | 0 (0.0) | 0 (0.0) | 1 (6.7) | 2 (25.0) |
Albert, et al. [9]¶ | KDIGO | 66.3 | 0 (0.0) | 0 (0.0) | 2 (11.8) | 1 (16.7) |
Haase, et al. [25]** | KDIGO | 159.1 | 0 (0.0) | 0 (0.0) | 2 (10.5) | 1 (25.0) |
Berlin cohort | ||||||
Sensitivity ≈ specificity | RIFLE | 16.85 | 1 (0.9) | 4 (6.1) | 2 (22.2) | 6 (40.0) |
Lowest distance D to (0,1) | RIFLE | 50.0 | 1 (0.8) | 4 (9.5) | 2 (22.2) | 6 (40.0) |
Maximum Youden index | RIFLE | 50.0 | 1 (0.8) | 4 (9.5) | 2 (22.2) | 6 (40.0) |
Albert, et al. [9]¶ | RIFLE | 79.3 | 3 (2.1) | 2 (6.1) | 3 (27.3) | 5 (38.5) |
Haase, et al. [25]** | RIFLE | 149.25 | 3 (2.0) | 2 (7.4) | 3 (23.1) | 5 (45.5) |
Sensitivity ≈ specificity | KDIGO | 14.25 | 1 (1.1) | 2 (3.8) | 2 (9.1) | 8 (21.6) |
Lowest distance D to (0,1) | KDIGO | 14.75 | 1 (1.1) | 2 (3.9) | 2 (9.1) | 8 (21.6) |
Maximum Youden index | KDIGO | 36.5 | 1 (0.9) | 2 (6.5) | 2 (6.9) | 8 (26.7) |
Albert, et al. [9]¶ | KDIGO | 79.3 | 2 (1.7) | 1 (4.0) | 4 (10.5) | 6 (28.6) |
Haase, et al. [25]** | KDIGO | 149.25 | 2 (1.7) | 1 (5.0) | 4 (9.8) | 6 (33.3) |
|
||||||
KRT initiation or in-hospital mortality | ||||||
|
||||||
Magdeburg cohort | ||||||
Sensitivity ≈ specificity | RIFLE | 10.6 | 0 (0.0) | 0 (0.0) | 0 (0.0) | 6 (60.0) |
Lowest distance D to (0,1) | RIFLE | 11.75 | 0 (0.0) | 0 (0.0) | 0 (0.0) | 6 (60.0) |
Maximum Youden index | RIFLE | 45.55 | 0 (0.0) | 0 (0.0) | 2 (28.6) | 4 (57.1) |
Albert, et al. [9]¶ | RIFLE | 66.3 | 0 (0.0) | 0 (0.0) | 3 (33.3) | 3 (60.0) |
Haase, et al. [25]** | RIFLE | 159.1 | 0 (0.0) | 0 (0.0) | 5 (45.5) | 1 (33.3) |
Sensitivity ≈ specificity | KDIGO | 8.0 | 0 (0.0) | 0 (0.0) | 0 (0.0) | 6 (46.2) |
Lowest distance D to (0,1) | KDIGO | 9.5 | 0 (0.0) | 0 (0.0) | 0 (0.0) | 6 (46.2) |
Maximum Youden index | KDIGO | 45.55 | 0 (0.0) | 0 (0.0) | 2 (13.3) | 4 (50.0) |
Albert, et al. [9]¶ | KDIGO | 66.3 | 0 (0.0) | 0 (0.0) | 3 (17.6) | 3 (50.0) |
Haase, et al. [25]** | KDIGO | 159.1 | 0 (0.0) | 0 (0.0) | 5 (26.3) | 1 (25.0) |
Berlin cohort | ||||||
Sensitivity ≈ specificity | RIFLE | 16.85 | 3 (2.8) | 5 (7.6) | 2 (22.2) | 11 (73.3) |
Lowest distance D to (0,1) | RIFLE | 50.0 | 3 (2.3) | 5 (11.9) | 2 (22.2) | 11 (73.3) |
Maximum Youden index | RIFLE | 50.0 | 3 (2.3) | 5 (11.9) | 2 (22.2) | 11 (73.3) |
Albert, et al. [9]¶ | RIFLE | 79.3 | 6 (4.2) | 2 (6.1) | 3 (27.3) | 10 (76.9) |
Haase, et al. [25] ** | RIFLE | 149.25 | 6 (4.1) | 2 (7.4) | 4 (30.8) | 9 (81.8) |
Sensitivity ≈ specificity | KDIGO | 14.25 | 1 (1.1) | 2 (3.8) | 4 (18.2) | 14 (37.8) |
Lowest distance D to (0,1) | KDIGO | 14.75 | 1 (1.1) | 2 (3.9) | 4 (18.2) | 14 (37.8) |
Maximum Youden index | KDIGO | 36.5 | 1 (0.9) | 2 (6.5) | 4 (13.8) | 14 (46.7) |
Albert, et al. [9]¶ | KDIGO | 79.3 | 2 (1.7) | 1 (4.0) | 7 (18.4) | 11 (52.4) |
Haase, et al. [25]** | KDIGO | 149.25 | 2 (1.7) | 1 (5.0) | 8 (19.5) | 10 (55.6) |
*A positive test result is determined if greater than or equal to the cutoff value; †No damage or functional impairment [10, 16]; ‡Subclinical AKI (stage 1 S); kidney tubular injury indicated by positive NGAL, no functional impairment detected [10, 16]; §Hemodynamic AKI (stage 1–3A); no tubular injury detected, functional impairment present [10, 16]; llAKI stage 1–3B; tubular injury and functional impairment present [10, 16]; ¶We chose the value closest to that suggested by Albert, et al. [9] (81 ng/mL); **We chose the value closest to that suggested by Haase, et al. [25] (150 ng/mL).
Table 3
Cutoff methodology | sCr/UO-AKI | NGAL(+)/sCr/UO(–)* vs. reference† | NGAL(–)/sCr(+)‡ vs. reference | NGAL(+)/sCr/UO(+)§ vs. reference | NGAL(+)/sCr(+) vs. NGAL(–)/sCr/UO(+) |
---|---|---|---|---|---|
KRT initiation | |||||
|
|||||
Magdeburg cohort | |||||
Sensitivity ≈ specificity | RIFLE | NC | NC | 177.67 (8.57–3,682.96) | 13.00 (0.55–306.22) |
Lowest distance D to (0,1) | RIFLE | NC | NC | 183.44 (8.85–3,801.49) | 13.00 (0.55–306.22) |
Maximum Youden index | RIFLE | NC | 75.00 (3.19–1,762.00) | 212.14 (9.45–4,763.31) | 2.83 (0.37–21.89) |
Albert, et al. [9]¶ | RIFLE | NC | 89.92 (4.18–1,934.88) | 233.80 (9.35–5,847.08) | 2.60 (0.33–20.79) |
Haase, et al. [25]** | RIFLE | NC | 141.31 (7.01–2,847.09) | 100.20 (3.22–3,121.90) | 0.71 (0.07–7.22) |
Sensitivity ≈ specificity | KDIGO | NC | NC | 77.13 (3.92–1,516.64) | 18.20 (0.88–374.91) |
Lowest distance D to (0,1) | KDIGO | NC | NC | 92.73 (4.73–1,818.94) | 18.20 (0.88–374.91) |
Maximum Youden index | KDIGO | NC | 27.59 (1.25–607.18) | 149.00 (6.90–3,218.77) | 5.40 (0.83–35.33) |
Albert, et al. [9]¶ | KDIGO | NC | 36.45 (1.79–743.94) | 151.00 (6.46–3,527.54) | 4.14 (0.63–27.32) |
Haase, et al. [25]** | KDIGO | NC | 57.28 (3.00–1,093.38) | 64.71 (2.21–1,891.82) | 1.13 (0.13–9.70) |
Berlin cohort | |||||
Sensitivity ≈ specificity | RIFLE | 2.55 (0.41–15.66) | 6.69 (0.55–81.94) | 46.81 (8.32–263.50) | 7.00 (0.69–70.75) |
Lowest distance D to (0,1) | RIFLE | 5.04 (0.81–31.24) | 8.19 (0.67–100.18) | 57.31 (10.20–321.99) | 7.00 (0.69–70.75) |
Maximum Youden index | RIFLE | 5.04 (0.81–31.24) | 8.19 (0.67–100.18) | 57.31 (10.20–321.99) | 7.00 (0.69–70.75) |
Albert, et al. [9]¶ | RIFLE | 1.08 (0.12–9.97) | 3.45 (0.35–33.85) | 40.25 (9.20–176.03) | 11.67 (1.14–119.55) |
Haase, et al. [25]** | RIFLE | 1.38 (0.15–12.89) | 6.55 (1.08–39.79) | 43.20 (9.19–203.09) | 6.60 (0.97–44.93) |
Sensitivity ≈ specificity | KDIGO | NC | 31.77 (1.58–640.36) | 67.58 (3.83–1,190.96) | 2.13 (0.56–8.14) |
Lowest distance D to (0,1) | KDIGO | NC | 32.13 (1.59–647.54) | 68.35 (3.88–1,204.32) | 2.13 (0.56–8.14) |
Maximum Youden index | KDIGO | NC | 28.92 (1.45–577.18) | 112.17 (6.32–1,990.32) | 3.88 (1.02–14.81) |
Albert, et al. [9]¶ | KDIGO | NC | 37.93 (2.04–703.57) | 145.44 (7.94–2,662.96) | 3.84 (1.10–13.32) |
Haase, et al. [25]** | KDIGO | NC | 44.13 (2.43–802.56) | 157.17 (8.43–2,931.68) | 3.56 (1.03–12.35) |
|
|||||
In-hospital mortality | |||||
|
|||||
Magdeburg cohort | |||||
Sensitivity ≈ specificity | RIFLE | NC | NC | 57.40 (2.69–1,222.58) | 4.20 (0.17–101.54) |
Lowest distance D to (0,1) | RIFLE | NC | NC | 59.27 (2.78–1,261.93) | 4.20 (0.17–101.54) |
Maximum Youden index | RIFLE | NC | 38.08 (1.41–1,031.09) | 60.00 (2.40–1,497.18) | 1.58 (0.14–17.41) |
Albert, et al. [9]¶ | RIFLE | NC | 55.67 (2.44–1,269.49) | 37.11 (1.09–1,259.86) | 0.67 (0.05–8.95) |
Haase, et al. [25]** | RIFLE | NC | 43.95 (1.96–985.05) | 66.80 (1.79–2,495.20) | 1.52 (0.10–22.74) |
Sensitivity ≈ specificity | KDIGO | NC | NC | 29.67 (1.42–619.31) | 7.00 (0.32–152.96) |
Lowest distance D to (0,1) | KDIGO | NC | NC | 35.67 (1.71–742.79) | 7.00 (0.32–152.96) |
Maximum Youden index | KDIGO | NC | 15.41 (0.60–397.42) | 57.31 (2.48–1,324.62) | 3.72 (0.40–34.43) |
Albert, et al. [9]¶ | KDIGO | NC | 24.35 (1.11–532.69) | 41.18 (1.50–1,134.17) | 1.69 (0.18–15.98) |
Haase, et al. [25]** | KDIGO | NC | 21.57 (0.99–469.65) | 64.71 (2.21–1,891.82) | 3.00 (0.29–30.74) |
Berlin cohort | |||||
Sensitivity ≈ specificity | RIFLE | 6.97 (0.76–63.74) | 30.86 (2.48–383.23) | 72.00 (7.79–665.30) | 2.33 (0.36–15.30) |
Lowest distance D to (0,1) | RIFLE | 13.89 (1.51–128.04) | 37.71 (3.04–467.80) | 88.00 (9.54–811.96) | 2.33 (0.36–15.30) |
Maximum Youden index | RIFLE | 13.89 (1.51–128.04) | 37.71 (3.04–467.80) | 88.00 (9.54–811.96) | 2.33 (0.36–15.30) |
Albert, et al. [9]¶ | RIFLE | 2.99 (0.48–18.65) | 17.38 (3.01–100.17) | 28.96 (5.85–143.28) | 1.67 (0.29–9.45) |
Haase, et al. [25]** | RIFLE | 3.87 (0.61–24.32) | 14.50 (2.59–81.29) | 40.28 (7.75–209.29) | 2.78 (0.48–16.03) |
Sensitivity ≈ specificity | KDIGO | 3.48 (0.31–39.35) | 8.70 (0.75–100.74) | 24.00 (2.88–200.14) | 2.76 (0.53–14.38) |
Lowest distance D to (0,1) | KDIGO | 3.59 (0.32–40.63) | 8.80 (0.76–101.89) | 24.28 (2.91–202.41) | 2.76 (0.53–14.38) |
Maximum Youden index | KDIGO | 7.45 (0.65–85.05) | 8.00 (0.70–91.53) | 39.27 (4.67–330.09) | 4.91 (0.94–25.53) |
Albert, et al. [9]¶ | KDIGO | 2.35 (0.21–27.03) | 6.65 (1.17–37.88) | 22.60 (4.18–122.30) | 3.40 (0.84–13.84) |
Haase, et al. [25]** | KDIGO | 3.11 (0.27–35.95) | 6.38 (1.12–36.24) | 29.50 (5.35–162.61) | 4.63 (1.11–19.19) |
|
|||||
KRT initiation or in-hospital mortality | |||||
|
|||||
Magdeburg cohort | |||||
Sensitivity ≈ specificity | RIFLE | NC | NC | 177.67 (8.57–3,682.96) | 13.00 (0.55–306.22) |
Lowest distance D to (0,1) | RIFLE | NC | NC | 183.44 (8.85–3,801.49) | 13.00 (0.55–306.22) |
Maximum Youden index | RIFLE | NC | 75.00 (3.19–1,762.00) | 212.14 (9.45–4,763.31) | 2.83 (0.37–21.89) |
Albert, et al. [9]¶ | RIFLE | NC | 89.92 (4.18–1,934.88) | 233.80 (9.35–5,847.08) | 2.60 (0.33–20.79) |
Haase, et al. [25]** | RIFLE | NC | 141.31 (7.01–2,847.09) | 100.20 (3.22–3,121.90) | 0.71 (0.07–7.22) |
Sensitivity ≈ specificity | KDIGO | NC | NC | 77.13 (3.92–1,516.64) | 18.20 (0.88–374.91) |
Lowest distance D to (0,1) | KDIGO | NC | NC | 92.73 (4.73–1,818.94) | 18.20 (0.88–374.91) |
Maximum Youden index | KDIGO | NC | 27.59 (1.25–607.18) | 149.00 (6.90–3,218.77) | 5.40 (0.83–35.33) |
Albert, et al. [9]¶ | KDIGO | NC | 36.45 (1.79–743.94) | 151.00 (6.46–3,527.54) | 4.14 (0.63–27.32) |
Haase, et al. [25]** | KDIGO | NC | 57.28 (3.00–1,093.38) | 64.71 (2.21–1,891.82) | 1.13 (0.13–9.70) |
Berlin cohort | |||||
Sensitivity ≈ specificity | RIFLE | 2.90 (0.67–12.54) | 10.10 (1.44–70.66) | 97.17 (19.22–491.30) | 9.63 (1.38–67.25) |
Lowest distance D to (0,1) | RIFLE | 5.86 (1.34–25.65) | 12.38 (1.77–86.51) | 119.17 (23.62–601.29) | 9.63 (1.38–67.25) |
Maximum Youden index | RIFLE | 5.86 (1.34–25.65) | 12.38 (1.77–86.51) | 119.17 (23.62–601.29) | 9.63 (1.38–67.25) |
Albert, et al. [9]¶ | RIFLE | 1.46 (0.28–7.59) | 8.50 (1.79–40.39) | 75.56 (16.40–348.05) | 8.89 (1.40–56.58) |
Haase, et al. [25]** | RIFLE | 1.89 (0.36–9.92) | 10.52 (2.51–44.10) | 106.50 (18.76–604.57) | 10.13 (1.47–69.94) |
Sensitivity ≈ specificity | KDIGO | 3.48 (0.31–39.35) | 19.33 (2.04–183.31) | 52.96 (6.61–423.97) | 2.74 (0.77–9.76) |
Lowest distance D to (0,1) | KDIGO | 3.59 (0.32–40.63) | 19.56 (2.06–185.40) | 53.57 (6.69–428.79) | 2.74 (0.77–9.76) |
Maximum Youden index | KDIGO | 7.45 (0.65–85.05) | 17.28 (1.85–161.36) | 94.50 (11.62–768.34) | 5.47 (1.53–19.59) |
Albert, et al. [9]¶ | KDIGO | 2.35 (0.21–27.03) | 12.76 (2.52–64.53) | 62.15 (12.06–320.26) | 4.87 (1.49–15.95) |
Haase, et al. [25]** | KDIGO | 3.11 (0.27–35.95) | 14.30 (2.90–70.62) | 73.75 (13.76–395.16) | 5.16 (1.54–17.27) |
*NGAL(+)/sCr/UO(–), Subclinical AKI (stage 1 S); kidney tubular injury indicated by positive NGAL; †The reference group was NGAL(–)/sCr/UO(–), No damage or functional impairment [10, 16]. ‡NGAL(–)/sCr(+), Hemodynamic AKI (stage 1–3A); §NGAL(+)/sCr(+), AKI stage 1–3B; tubular injury and functional impairment present [10, 16]; ¶We chose the value closest to that suggested by Albert, et al. [9] (81 ng/mL); **We chose the value closest to that suggested by Haase, et al. [25] (150 ng/mL).