Abstract
Background
To ensure valid results of big data research in the medical field, the input laboratory results need to be of high quality. We aimed to establish a strategy for evaluating the quality of laboratory results suitable for big data research.
Methods
We used Korean Association of External Quality Assessment Service (KEQAS) data to retrospectively review multicenter data. Seven measurands were analyzed using commutable materials HbA1c, creatinine (Cr), total cholesterol (TC), triglyceride (TG), alpha-fetoprotein (AFP), prostate-specific antigen (PSA), and cardiac troponin I (cTnI). These were classified into three groups based on their standardization or harmonization status. HbA1c, Cr, TC, TG, and AFP were analyzed with respect to peer group values. PSA and cTnI were analyzed in separate peer groups according to the calibrator type and manufacturer, respectively. The acceptance rate and absolute percentage bias at the medical decision level were calculated based on biological variation criteria.
Results
The acceptance rate (22.5%–100%) varied greatly among the test items, and the mean percentage biases were 0.6%–5.6%, 1.0%–9.6%, and 1.6%–11.3% for all items that satisfied optimum, desirable, and minimum criteria, respectively.
Conclusions
The acceptance rate of participants and their external quality assessment (EQA) results exhibited statistically significant differences according to the quality grade for each criterion. Even when they passed the EQA standards, the test results did not guarantee the quality requirements for big data. We suggest that the KEQAS classification can serve as a guide for building big data.
Research focus on big data in the healthcare systems field has been increasing because the large amounts of data generated in healthcare systems can potentially contribute to population health management and personalized medicine. The growth of healthcare data is associated with the increase in their digital availability [1]. The sources of big data in healthcare include electronic health records, clinical data (medical imaging and laboratory examination), pharmaceutical data, public records, genomic databases, and measurements made by medical devices [2]. Numerous big data projects in the healthcare systems field have focused on clinical decision support, personalized medicine, population health management, cost reduction, and improvement in the quality of healthcare [3].
Big data in healthcare exhibit distinct features, such as heterogeneity, incompleteness, privacy, and data ownership, in addition to the commonly referred “5 V” (volume, velocity, variety, veracity, and value) [3]. The accuracy, completeness, and consistency of such data are crucial to ensure the quality of the output results [4]. Input data of poor quality can lead to poor decision-making and unreliable results.
The quality of laboratory data is important for the retrospective analysis of large amounts of multicenter data in light of the quality issue of big data. According to the U.S. Centers for Disease Control and Prevention, 70% of current medical decisions rely on laboratory test results, showing the important role of clinical laboratories in current healthcare system [5, 6]. As most test results in diagnostic laboratory medicine are quantitative, the equivalence of test results among laboratories is ensured through standardization and harmonization. Despite these efforts, there remains a large bias in test results when the same sample is tested in various laboratories. If the biased test results are included in multicenter big data, the outcome results of big data research using such biased laboratory results are of no use. Thus, it is essential in big data research to assess the quality or accuracy of laboratory data using external quality assessment (EQA) results.
EQA surveys evaluate the quality of test items in a laboratory. As EQA surveys require only minimum quality criteria, for laboratory big data, it is necessary to evaluate EQA results using stricter criteria [7]. We aimed to establish a strategy for evaluating the quality of laboratory results suitable for big data research using Korean Association of External Quality Assessment Service (KEQAS) data as a surrogate for real laboratory data. The acceptance rate of participants and their EQA results were compared considering their quality grade based on the biological variation (BV) or outcome-based criteria for the total error.
This retrospective study was conducted using multicenter EQA results from clinical laboratories. We retrieved KEQAS data of commutable fresh-frozen serum samples from 2010 to 2020 and analyzed more than 30,000 EQA results for seven test items. We categorized the data into three groups depending on whether the measurement procedures had been standardized or harmonized (Fig. 1).
The first group comprised laboratory tests for HbA1c, creatinine (Cr), total cholesterol (TC), and triglyceride (TG) fully standardized in accuracy-based EQAs [8–11]. The target values of these tests were measured using reference measurement procedures in certified reference laboratories [12, 13]. According to the International Consortium for Harmonization of Clinical Laboratory Results, the tests in the second and third groups had maintained their harmonization status or were undergoing harmonization [14]. The second group comprised tests for which relevant international standards exist, including tests for alpha-fetoprotein (AFP) and prostate-specific antigen (PSA). AFP tests were calibrated against the WHO 72/225 International Standard (IS). The PSA tests were calibrated using the WHO 96/670 IS or the Hybritech standard (Beckman Coulter Inc., Brea, CA, USA) [14, 15]. The target values for this group were determined by calculating the mean in accordance with their standards. The third group comprised tests for which harmonization was still ongoing because of the lack of traceable calibrators or the use of various antibodies, such as the cardiac troponin I (cTnI) test. We analyzed the results of major instrument platforms for cTnI that were used by more than 10 EQA survey participants. These platforms included Abbott (Abbott Diagnostics, Abbott Park, IL, USA), Beckman Coulter Inc., LSI Medience (LSI Medience, Chiba, Japan), Radiometer (Radiometer Medical ApS, Brønshøj, Denmark), Roche (Roche Diagnostics, Mannheim, Germany), and Siemens (Siemens Healthineers, Erlangen, Germany). Because these cTnI tests use different calibrators and epitopes, the average value for each manufacturer was considered the target value. The manufacturer names are denoted as letters from A to F.
We selected EQA samples with concentrations close to medical decision levels according to corresponding clinical guidelines and subsequently calculated the absolute percentage bias for each test item (Supplemental Data Table S1) [17–23]. We analyzed 10 samples for HbA1c, Cr, and TC; five for TG and PSA; four for AFP; and eight for cTnI. The analytical performance specifications (APSs) were the optimum, desirable, and minimum goal of total error (TE) based on the BV of the measurand using the latest European Federation of Clinical Chemistry and Laboratory Medicine data [24]. The acceptance criteria are summarized in Supplemental Data Table S2. In addition to the BV, an outcome-based criterion for TE (6.7%) was used for HbA1c [25]. Results that did not meet the minimum criteria (outcome-based criterion for HbA1c) were considered unacceptable.
Microsoft Office Excel 2021 (Microsoft Co., Redmond, WA, USA) and MedCalc version 19.2.6 for Windows (MedCalc Software, Ostend, Belgium) were used for statistical analysis. The mean percentage bias for the BV criteria was compared between groups using one-way ANOVA followed by the Student–Newman–Keuls and Kruskal–Wallis tests and then Dunn’s post-hoc test. Statistical significance was defined as P<0.05. To detect outliers, the distributions of the total sample results from each participating laboratory and test were visually observed; a value >3 SDs from the target mean concentration was considered an outlier. The 95% confidence intervals (CIs) of the mean percentage bias for the BV criteria were calculated from all samples after outlier elimination.
Fig. 2 shows the acceptance rates and concentrations expressed in National Glycohemoglobin Standardization Program (NGSP) units of 10 HbA1c samples. The concentrations ranged from 5.8% to 7.1%. Conversion between NGSP (%) and International Federation of Clinical Chemistry (IFCC) (mmol/mol) units requires a linear equation: IFCC unit=10.93×NGSP unit–23.5. The mean acceptance rates were 95.2%, 67.5%, 42.9%, and 22.9% within the outcome-based, minimum, desirable, and optimum criteria, respectively.
The mean acceptance rates for the first group for various APSs are presented in Fig. 3A. For Cr, the average acceptance rates for 10 samples with concentrations ranging from 0.66 to 1.40 mg/dL were 70.9%, 56.3%, and 34.4% within the minimum, desirable, and optimum criteria, respectively. The Cr concentration can be converted from mg/dL to the SI unit (µmol/L) by multiplying the value with 88.42. The average acceptance rates for 10 TC samples with concentrations ranging from 197.2 to 246.4 mg/dL were 100.0%, 99.1%, and 86.0%, respectively. TC and TG concentrations can be converted from mg/dL to the SI unit (µmol/L) by multiplying the values with 0.0259 and 0.0113, respectively. The TG data were divided into two groups based on whether or not the test method included free glycerol blanking, and the concentrations of the five samples ranged from 93.3 to 205.0 mg/dL. Within the minimum, desirable, and optimum criteria, the average acceptance rates were 100.0%, 100.0%, and 99.5%, respectively, for the non-free glycerol-blanking method, 100.0%, 100.0%, and 99.7%, respectively, for the free glycerol blanking method, and 100.0%, 100.0%, and 99.6%, respectively, for both methods combined.
The AFP data from the 2019–2020 survey demonstrated that the four samples had concentrations ranging from 11.6 to 87.8 ng/mL, which were close to the clinical threshold. Based on the minimum, desirable, and optimum criteria, the average acceptance rates were 99.8%, 99.6%, and 94.7%, respectively.
The PSA data were divided into two groups according to the calibrator. According to the WHO 96/670 IS, the average acceptance rates for five samples with concentrations ranging from 4.011 to 12.989 ng/mL were 99.1%, 92.4%, and 60% within the minimum, desirable, and optimum criteria, respectively. According to the Hybritech standard, the average acceptance rates for five samples with concentrations ranging from 4.176 to 14.329 ng/mL were 98.4%, 89.9%, and 50.3% within the minimum, desirable, and optimum criteria, respectively (Fig. 3B).
We analyzed the survey data for cTnI using samples with values close to the concentrations at which the CV was 20% in the manufactures’ package inserts. The concentrations of the eight samples ranged from 0.106 to 2.006 ng/mL. The mean acceptance rates for the six manufacturers are shown in Fig. 3C. The mean acceptance rates within the minimum criteria for TE were >95.0% for all manufacturers, except one (F; 89.1%). For manufacturers A, B, and D, the mean acceptance rates were all >95.0% within the desirable bounds. The mean acceptance rates within the optimum criteria were 92.0%, 82.5%, 72.9%, 91.4%, 77.4%, and 50.4% for manufacturers A–F, respectively.
Figs. 4 and 5 and Supplemental Data Fig. S1 show box and whisker plots of the mean percentage bias for all analyte items according to the performance criteria. The mean percentage bias for the BV criteria showed significant differences between the groups (P<0.0001, one-way ANOVA) for all analyte items. The mean percentage bias (95% CI) for each analyte is summarized in Table 1.
The mean percentage bias did not significantly differ between the two calibrator types based on the APS groups for PSA (P=0.236, 0.325, 0.522, and 0.603 for the optimum, desirable, minimum, and unacceptable criteria, respectively) (Supplemental Data Fig. S1). Conversely, for cTnI, the mean percentage bias differed significantly (P<0.05) among the platforms based on the APS groups (Fig. 5). According to the optimum, desirable, minimum, and unacceptable criteria, the mean percentage bias (range) for cTnI for the six platforms was 4.4% (3.9%–5.5%), 6.5% (4.8%–9.6%), 7.2% (4.8%–11.3%), and 46.0% (41.0%–99.0%), respectively.
Big data research using unreliable laboratory results can result in poor medical decisions, improper risk stratification, inappropriate management, and increased costs for the patient [28, 29]. We investigated the eligibility of test results that met the EQA criteria to be included in big data based on the BV or outcome-based criteria.
We selected seven test items that were measured using commutable frozen human serum pools in the KEQAS program. According to the test item, the acceptance rates for EQA results were 67.5%–100%, 42.9%–100%, and 22.9%–99.5% within the minimum, desirable, and optimum criteria, respectively. Among the seven test items, HbA1c and Cr showed low acceptance rates. Based on the minimum criteria, the mean acceptance rates for HbA1c and Cr were 67.5% and 70.9%, respectively, which we attribute to the minimum criteria for HbA1c (3.3%) and Cr (11.1%) being lower than the KEQAS acceptable bias criteria for HbA1c (6.7%) and Cr (11.4%). The acceptance rate was analyzed using all participants with acceptable and unacceptable results in KEQAS in this study. Therefore, few participants showed mean percentage biases between 11.1% and 11.4%.
The minimum criterion for HbA1c was 3.3%, which is 15.8 times more stringent than that of APF (52.2%); therefore, it had the lowest acceptance rate among the seven test items. The minimum criterion of Cr was 11.1%, which is three times higher than that of HbA1c, while the acceptance rate was comparable to that of HbA1c, which can be attributed to the analytical interference in routine Cr methods. Because the minimum criterion for Cr is high, it is necessary to deduce through discussions with data scientists whether any of the criteria based on the BV can be applied to the big data criterion for Cr. Unlike for Cr, it may be possible to use the minimum criterion for HbA1c for use in big data, unless HbA1c big data require very high accuracy.
The BV criteria of TC were similar to those of Cr, but its acceptance rates were 1.4, 1.8, and 2.5 times higher than those of Cr for the minimum, desirable, and optimal criteria, respectively. Unlike that for Cr, the KEQAS acceptable bias criterion for TC was 9%, which was higher than the desirable criterion (8.7%) and lower than the minimum criterion (13.0%). The difference in medical decision levels between Cr (0.7–1.0 mg/dL) and TC (200–240 mg/dL) was another factor contributing to the higher acceptance rate of TC. Even when the absolute difference was small, a low Cr value was more likely to cause a large relative difference (%bias). Fully automated enzymatic methods, less interference, and standardization of measurement procedures for cholesterol quantification were additional contributing factors. The mean percentage biases for TC were <3% based on the minimum, desirable, and optimum criteria. Given the high acceptance rates and low mean percentage biases for TC based on all criteria, we can apply any criterion according to the needs in term of accuracy and size of the TC big data.
TG showed a substantially wider BV criterion than other lipids owing to its high intraindividual BV, which is approximately three times that of TC [30]. The optimum criterion for TG was 13.5%, which is close to the KEQAS acceptable bias criterion for TC (15%). Few EQA results for TG were outside the optimum criterion, regardless of free glycerol blanking. Therefore, for TG, it is crucial to set a new criterion other than the BV-based criterion for use in big data.
The average acceptance rate of AFP was approximately 95.0% based on the optimum criterion (17.4%). Despite using target values for AFP that were derived from all methods used in different platforms, the acceptance rate was high. The mean percentage bias for AFP did not significantly differ (approximately 6%) among the three BV groups. The average acceptance rates of the two PSA calibrator types were approximately 60.0% based on the optimum criterion, which was lower than that of AFP. The difference in the BV criteria was one cause of this discrepancy; the optimum criterion of PSA was 8.1%, which is less than half of that of AFP (17.4%). Accordingly, the mean percentage bias of PSA was <4.0% and that of AFP was 5.6%. The WHO calibrator yields 2%–14% lower PSA value than the Hybritech calibrator [31, 32]. According to the optimum, desirable, and minimum criteria, there were 3.0%, 14.3%, and 14.7% differences, respectively, in mean percentage bias between the two calibrator types. Therefore, it is essential to construct big data according to the type of calibrator used in the PSA test. Recently, the APSs derived from state-of-the-art tests were shown to be the most suitable because of the lack of high-quality BV data for tumor markers [33]. The average acceptance rate for PSA was approximately 90.0% when applying the 15% criterion recommended in earlier studies [33, 34]. Further research is needed to decide the criteria to be set for AFP or PSA big data.
The acceptance rates for cTnI were 89.1%–99.3%, 81.7%–99.4%, and 50.4%–92.0% for the minimum, desirable, and optimum criteria, respectively. The results varied among manufacturers owing to differences in calibration and antibody specificity [35]; moreover, the bias was calculated using the mean value of each instrument peer group. However, the acceptance rate varied significantly among peer groups; particularly, the acceptance rate according to the optimal criteria ranged from 50.4% to 92.0%. If the overall mean of the six cTnI tests was used as target value and all EQA results were simultaneously analyzed, the acceptance rates based on the BV criteria were 44.3%, 33.0%, and 17.2% for the minimum, desirable, and optimum criteria, respectively. For the TnI test, acceptance rates should be determined separately for the different manufacturers. The mean percentage biases for cTnI among six platforms were 3.9%–5.5%, 4.8%–9.6%, and 4.8%–11.3% for the optimum, desirable, and minimum criteria, respectively. The mean percentage bias was significant for the desirable and minimum criteria but relatively small for the optimum criterion. The results in the unacceptable group showed a mean percentage bias of 40.9%–99.0% among the six platforms. For big data construction, one must consider the platform used for the TnI test to improve clinical outcomes in patients with various cardiovascular conditions. Further research may be needed to decide an outcome-based criterion for TnI big data.
There have been numerous studies on standardizing terms, result formats, statistical techniques, and data categorization or mapping tools to improve the quality of big data [23, 36–38]. However, big data researchers, not clinical pathologists, may wrongly believe that all quantified data can be aggregated without any quality-assurance checks [39, 40]. We used EQA results as a surrogate for real laboratory data, and we compared and analyzed participants’ EQA results considering their quality grade based on the TE, which revealed statistically significant differences. Even test results that passed the EQA did not guarantee the quality for inclusion in big data. Therefore, in big data research, it is essential for laboratory medicine experts to ensure that the data meet quality standards; particularly, the reliability of test results should be considered [12]. Big data should be classified according to the state of harmonization or standardization; however, no study has been conducted on this. EQAs evaluate test results using categorization based on the standardization or harmonization status. This classification can guide building big data for each test item.
One potential study limitation is that we only used BV based on the test items as the acceptance criteria. Because standards or guidelines for QC of laboratory data are lacking, further research is needed to establish criteria and evaluate the data quality according to test items, test characteristics, and the purpose and amount of big data. According to Kim, et al. [12], cumulative EQA data can be used to evaluate a laboratory’s reliability over time. As EQA can only guarantee a laboratory’s performance at a given point and big data in healthcare include longitudinal patient records, it is desirable to analyze accumulated EQA results from each laboratory to determine whether its test results can be included in big data.
Notes
REFERENCES
1. Wang L, Alexander CA. 2020; Big data analytics in medical engineering and healthcare: methods, advances and challenges. J Med Eng Technol. 44:267–83. DOI: 10.1080/03091902.2020.1769758. PMID: 32498594.


2. Rumsfeld JS, Joynt KE, Maddox TM. 2016; Big data analytics to improve cardiovascular care: promise and challenges. Nat Rev Cardiol. 13:350–9. DOI: 10.1038/nrcardio.2016.42. PMID: 27009423.


3. Hong L, Luo M, Wang R, Lu P, Lu W, Lu L. 2018; Big data in health care: applications and challenges. Data Inf Manag. 2:175–97. DOI: 10.2478/dim-2018-0014.


4. Mashoufi M, Ayatollahi H, Khorasani-Zavareh D. 2018; A review of data quality assessment in emergency medical services. Open Med Inform J. 12:19–32. DOI: 10.2174/1874431101812010019. PMID: 29997708. PMCID: PMC5997849.


5. Hallworth MJ. 2011; The '70% claim': what is the evidence base? Ann Clin Biochem. 48:487–8. DOI: 10.1258/acb.2011.011177. PMID: 22045648.


6. Division of Laboratory Systems (DLS), Centers for Disease Control and Prevention. https://www.cdc.gov/csels/dls/strengthening-clinical-labs.html. Updated on Jan 2023.
7. Kim S, Lee K, Park HD, Lee YW, Chun S, Min WK. 2021; Schemes and Performance Evaluation Criteria of Korean Association of External Quality Assessment (KEQAS) for Improving Laboratory Testing. Ann Lab Med. 41:230–9. DOI: 10.3343/alm.2021.41.2.230. PMID: 33063686. PMCID: PMC7591290.


8. John WG, Mosca A, Weykamp C, Goodall I. 2007; HbA1c standardisation: history, science and politics. Clin Biochem Rev. 28:163–8.
9. Nakamura M, Iso H, Kitamura A, Imano H, Kiyama M, Yokoyama S, et al. 2015; Total cholesterol performance of Abell-Levy-Brodie-Kendall reference measurement procedure: certification of Japanese in-vitro diagnostic assay manufacturers through CDC's cholesterol Reference Method Laboratory Network. Clin Chim Acta. 445:127–32. DOI: 10.1016/j.cca.2015.03.026. PMID: 25818239. PMCID: PMC4579524.


10. Nakamura M, Iso H, Kitamura A, Imano H, Noda H, Kiyama M, et al. 2016; Comparison between the triglycerides standardization of routine methods used in Japan and the chromotropic acid reference measurement procedure used by the CDC Lipid Standardization Programme. Ann Clin Biochem. 53:632–9. DOI: 10.1177/0004563215624461. PMID: 26680645. PMCID: PMC5695560.


11. Myers GL. 2008; Standardization of serum creatinine measurement: theory and practice. Scand J Clin Lab Invest Suppl. 241:57–63. DOI: 10.1080/00365510802149887. PMID: 18569966.


12. Kim S, Cho EJ, Jeong TD, Park HD, Yun YM, Lee K, et al. 2023; Proposed model for evaluating real-world laboratory results for big data research. Ann Lab Med. 43:104–7. DOI: 10.3343/alm.2023.43.1.104. PMID: 36045065. PMCID: PMC9467825.


13. Kim JH, Cho Y, Lee SG, Yun YM. 2019; Report of Korean association of external quality assessment service on the accuracy-based lipid proficiency testing (2016-2018). J Lab Med Qual Assur. 41:121–9. DOI: 10.15263/jlmqa.2019.41.3.121.


14. International Consortium for Harmonization of Clinical Laboratory Results (ICHCLR). www.harmonization.net. Updated on Nov 2021.
15. Ferraro S, Panzeri A, Braga F, Panteghini M. 2019; Serum α-fetoprotein in pediatric oncology: not a children's tale. Clin Chem Lab Med. 57:783–97. DOI: 10.1515/cclm-2018-0803. PMID: 30367785.


16. Ferraro S, Bussetti M, Rizzardi S, Braga F, Panteghini M. 2021; Verification of harmonization of serum total and free prostate-specific antigen (PSA) measurements and implications for medical decisions. Clin Chem. 67:543–53. DOI: 10.1093/clinchem/hvaa268. PMID: 33674839.


17. American Diabetes Association 6. 2021; Glycemic targets: standards of medical care in Diabetes-2021. Diabetes Care. 44:S73–84. DOI: 10.2337/dc21-S006. PMID: 33298417.
18. Park EY, Kim TY. 2010; Where are cut-off values of serum creatinine in the setting of chronic kidney disease? Kidney Int. 77:645–6. DOI: 10.1038/ki.2009.529. PMID: 20224585.


19. Grundy SM, Cleeman JI, Merz CN, Brewer HB Jr, Clark LT, Hunninghake DB, et al. 2004; Implications of recent clinical trials for the National Cholesterol Education Program Adult Treatment Panel III guidelines. Circulation. 110:227–39. DOI: 10.1161/01.CIR.0000133317.49796.0E. PMID: 15249516.


20. Gambarin-Gelwan M, Wolf DC, Shapiro R, Schwartz ME, Min AD. 2000; Sensitivity of commonly available screening tests in detecting hepatocellular carcinoma in cirrhotic patients undergoing liver transplantation. Am J Gastroenterol. 95:1535–8. DOI: 10.1111/j.1572-0241.2000.02091.x. PMID: 10894592.


21. Trevisani F, D'Intino PE, Morselli-Labate AM, Mazzella G, Accogli E, Caraceni P, et al. 2001; Serum alpha-fetoprotein for diagnosis of hepatocellular carcinoma in patients with chronic liver disease: influence of HBsAg and anti-HCV status. J Hepatol. 34:570–5. DOI: 10.1016/S0168-8278(00)00053-2. PMID: 11394657.
22. Carter HB, Albertsen PC, Barry MJ, Etzioni R, Freedland SJ, Greene KL, et al. 2013; Early detection of prostate cancer: AUA Guideline. J Urol. 190:419–26. DOI: 10.1016/j.juro.2013.04.119. PMID: 23659877. PMCID: PMC4020420.


23. Contemporary cardiac troponin I and T assay analytical characteristics designated by manufacturer IFCC committee on clinical applications of cardiac bio-markers (C-CB) v052022. https://ifcc.org/ifcc-education-division/emd-committees/committee-on-clinical-applications-of-cardiac-bio-markers-c-cb/biomarkers-reference-tables/. Updated on Jan 2023.
24. Aarsand AK, Fernandez-Calle P, Webster C, Coskun A, Gonzales-Lao E, Diaz-Garzon J, et al. The EFLM Biological Variation Database. https://biologicalvariation.eu/. Updated on Nov 2021.
25. Weykamp CW, Mosca A, Gillery P, Panteghini M. 2011; The analytical goals for hemoglobin A(1c) measurement in IFCC units and National Glycohemoglobin Standardization Program Units are different. Clin Chem. 57:1204–6. DOI: 10.1373/clinchem.2011.162719. PMID: 21571810.


26. Myers GL, Miller WG, Coresh J, Fleming J, Greenberg N, Greene T, et al. 2006; Recommendations for improving serum creatinine measurement: a report from the Laboratory Working Group of the National Kidney Disease Education Program. Clin Chem. 52:5–18. DOI: 10.1373/clinchem.2005.0525144. PMID: 16332993.


27. Warnick GR, Kimberly MM, Waymack PP, Leary ET, Myers GL. 2008; Standardization of measurements for cholesterol, triglycerides, and major lipoproteins. Lab Med. 39:481–90. DOI: 10.1309/6UL9RHJH1JFFU4PY.


28. Hripcsak G, Knirsch C, Zhou L, Wilcox A, Melton G. 2011; Bias associated with mining electronic health records. J Biomed Discov Collab. 6:48–52. DOI: 10.5210/disco.v6i0.3581. PMID: 21647858. PMCID: PMC3149555.


29. Weiskopf NG, Hripcsak G, Swaminathan S, Weng C. 2013; Defining and measuring completeness of electronic health records for secondary use. J Biomed Inform. 46:830–6. DOI: 10.1016/j.jbi.2013.06.010. PMID: 23820016. PMCID: PMC3810243.


30. Marcovina SM, Gaur VP, Albers JJ. 1994; Biological variability of cholesterol, triglyceride, low- and high-density lipoprotein cholesterol, lipoprotein(a), and apolipoproteins A-I and B. Clin Chem. 40:574–8. DOI: 10.1093/clinchem/40.4.574. PMID: 8149613.


31. Vignati G, Giovanelli L. 2007; Standardization of PSA measures: a reappraisal and an experience with WHO calibration of Beckman Coulter Access Hybritech total and free PSA. Int J Biol Markers. 22:295–301. DOI: 10.1177/172460080702200409. PMID: 18161661.


32. Stephan C, Bangma C, Vignati G, Bartsch G, Lein M, Jung K, et al. 2009; 20-25% lower concentrations of total and free prostate-specific antigen (PSA) after calibration of PSA assays to the WHO reference materials-analysis of 1098 patients in four centers. Int J Biol Markers. 24:65–9. DOI: 10.5301/JBM.2009.1349. PMID: 19634108.


33. Marques-Garcia F, Boned B, González-Lao E, Braga F, Carobene A, Coskun A, et al. 2022; Critical review and meta-analysis of biological variation estimates for tumor markers. Clin Chem Lab Med. 60:494–504. DOI: 10.1515/cclm-2021-0725. PMID: 35143717.


34. Carobene A, Guerra E, Locatelli M, Cucchiara V, Briganti A, Aarsand AK, et al. 2018; Biological variation estimates for prostate specific antigen from the European Biological Variation Study; consequences for diagnosis and monitoring of prostate cancer. Clin Chim Acta. 486:185–91. DOI: 10.1016/j.cca.2018.07.043. PMID: 30063887.


35. Christenson RH, Jacobs E, Uettwiller-Geiger D, Estey MP, Lewandrowski K, Koshy TI, et al. 2017; Comparison of 13 commercially available cardiac troponin assays in a multicenter North American study. J Appl Lab Med. 2:134. DOI: 10.1373/jalm.2017.023903. PMID: 33636962.


36. Kim HS, Kim DJ, Yoon KH. 2019; Medical big data is not yet available: why we need realism rather than exaggeration. Endocrinol Metab (Seoul). 34:349–54. DOI: 10.3803/EnM.2019.34.4.349. PMID: 31884734. PMCID: PMC6935779.


37. Dash S, Shakyawar SK, Sharma M, Kaushik S. 2019; Big data in healthcare: management, analysis and future prospects. J Big Data. 6:54. DOI: 10.1186/s40537-019-0217-0.


38. Shi X, Prins C, Van Pottelbergh G, Mamouris P, Vaes B, De Moor B. 2021; An automated data cleaning method for electronic health records by incorporating clinical knowledge. BMC Med Inform Decis Mak. 21:267. DOI: 10.1186/s12911-021-01630-7. PMID: 34535146. PMCID: PMC8449435.


39. Vesper HW, Myers GL, Miller WG. 2016; Current practices and challenges in the standardization and harmonization of clinical laboratory tests. Am J Clin Nutr. 104(Suppl 3):907S–12S. DOI: 10.3945/ajcn.115.110387. PMID: 27534625. PMCID: PMC5004491.


40. Panteghini M. 2012; Implementation of standardization in clinical practice: not always an easy task. Clin Chem Lab Med. 50:1237–41. DOI: 10.1515/cclm.2011.791. PMID: 22850055.


Fig. 1
Overview of the approach used to categorize the data into groups and establish the target values.
Abbreviations: KEQAS, Korean Association of External Quality Assessment Service; EQA, External Quality Assessment.
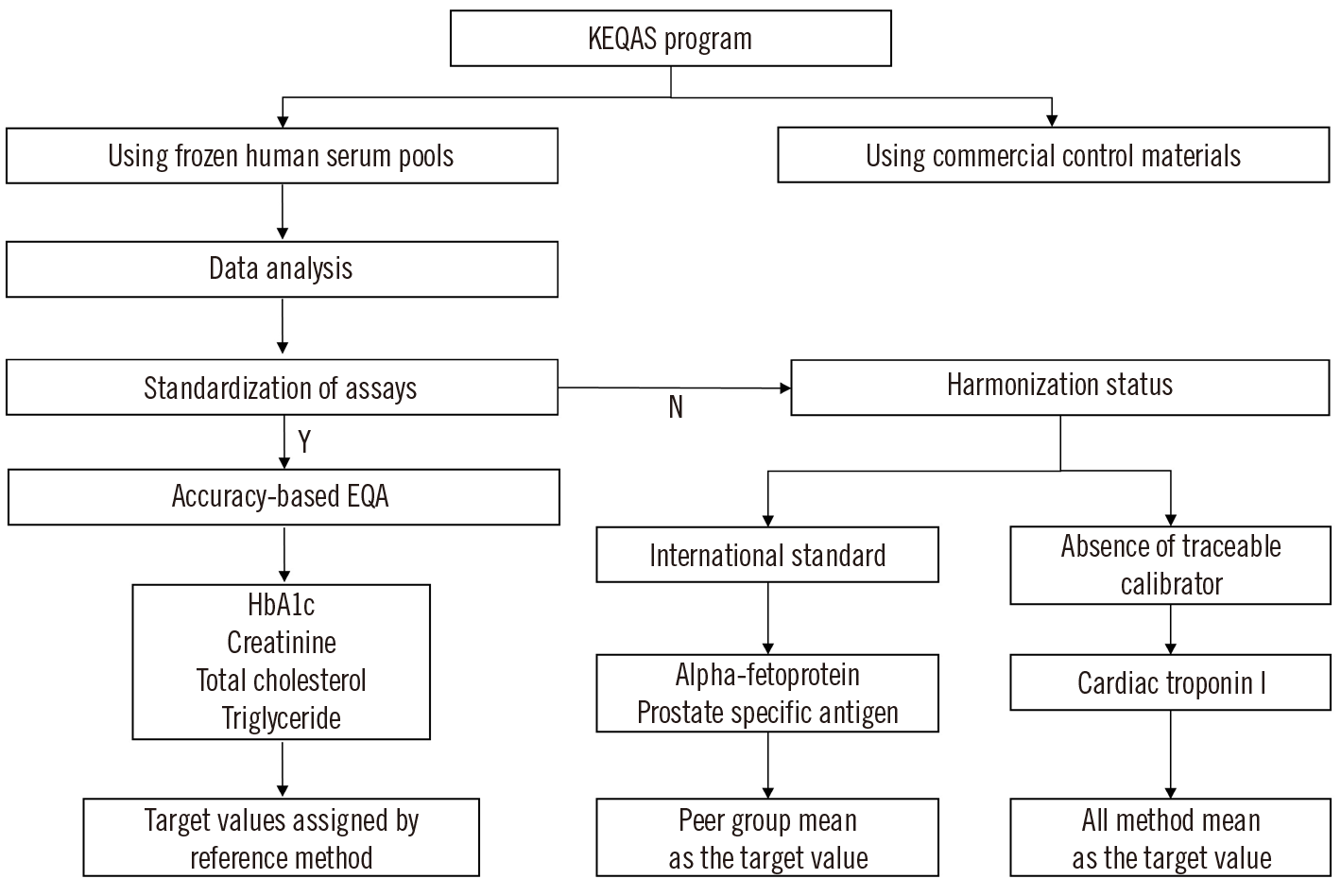
Fig. 2
Percentages of acceptable performances and sample concentrations ranging from approximately 6.0% to 7.0% obtained from 10 EQA samples for HbA1c according to different performance goals.
Abbreviation: EQA, external quality assessment.
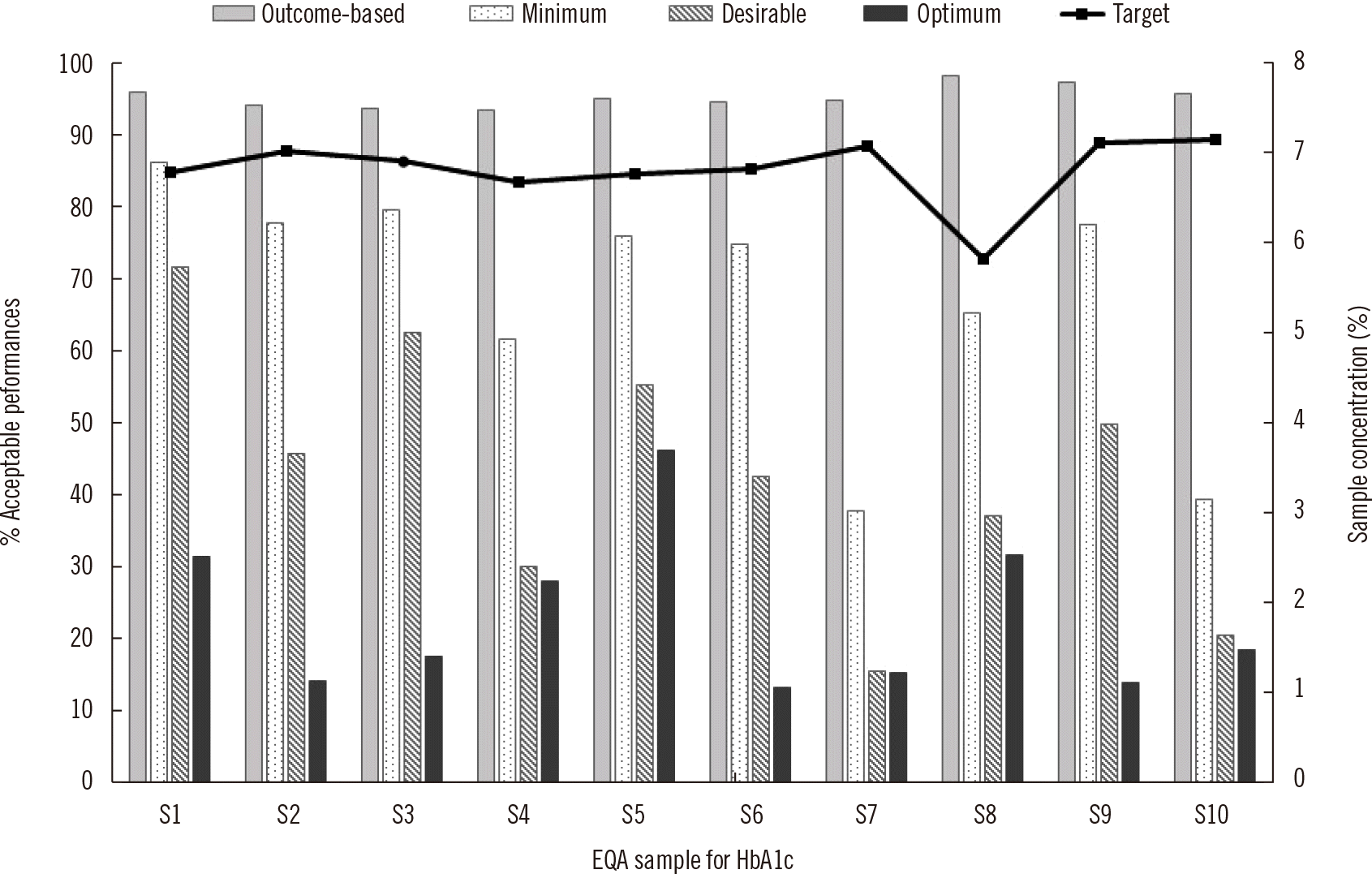
Fig. 3
Mean percentages of acceptable performances considering the participants’ EQA results according to different performance goals. Data categorized into three groups are shown for each test item. (A) Standardization of laboratory tests, including HbA1c, creatinine, total cholesterol, and triglyceride. (B) Harmonization category, including AFP and PSA classified according to the calibrator (Hybritech standard or WHO 96/670 IS). (C) Lack-of-harmonization category, including cardiac troponin I, according to six manufacturers (A–F).
Abbreviations: EQA, external quality assessment; AFP, alpha-fetoprotein; PSA, prostate-specific antigen; IS, international standard.
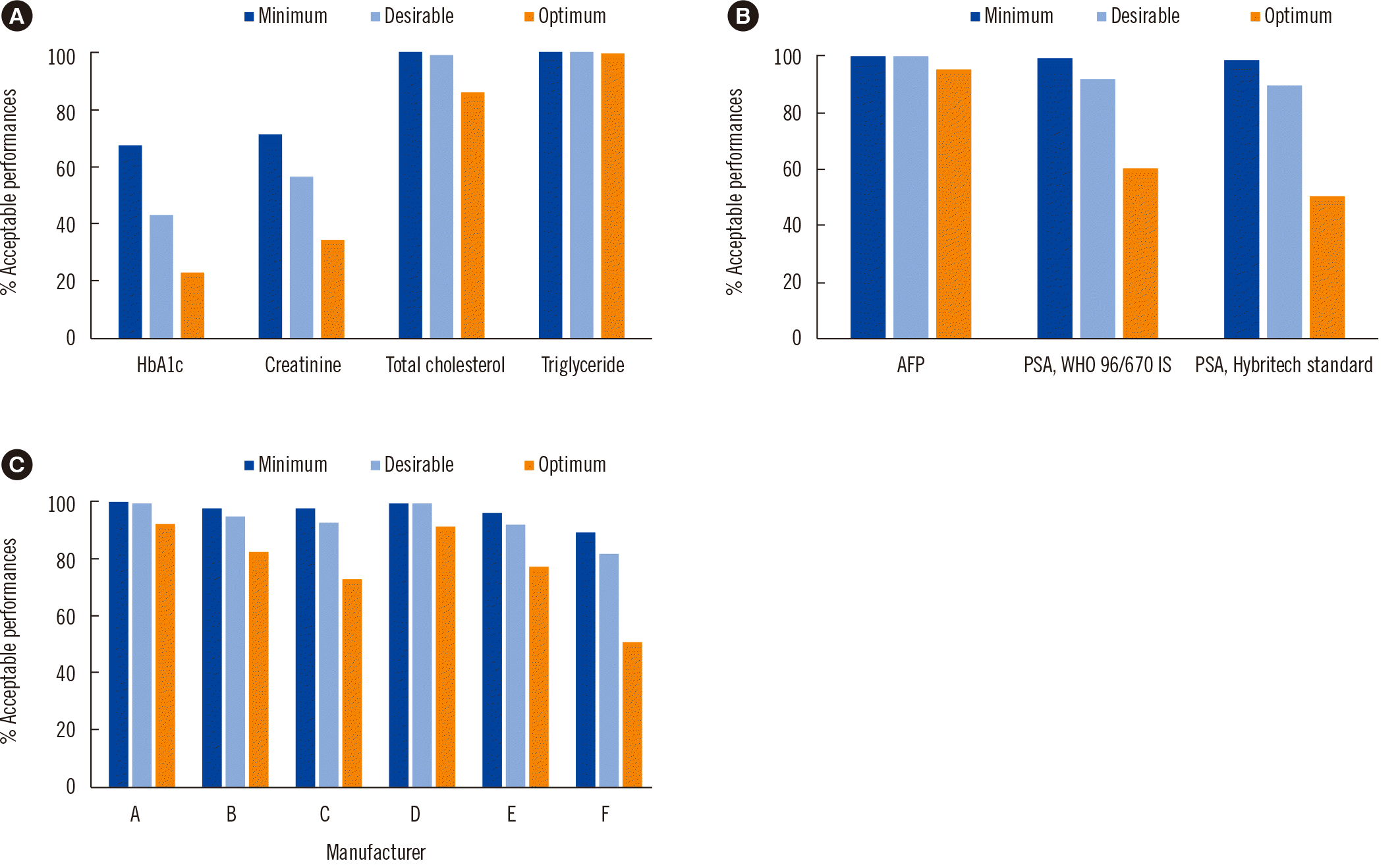
Fig. 4
Box plot analysis of mean percentage bias for total samples for each test according to different performance goals. (A) HbA1c, (B) creatinine, (C) total cholesterol, (D) triglyceride, and (E) alpha-fetoprotein. The gray box plot shows the minimum, first quartile, median, third quartile, and maximum values. The blue line indicates the mean and the blue diamond the confidence interval of the data. Groups were compared using the Student–Newman–Keuls multiple-comparison test. **P≤0.001 and *P≤0.05.
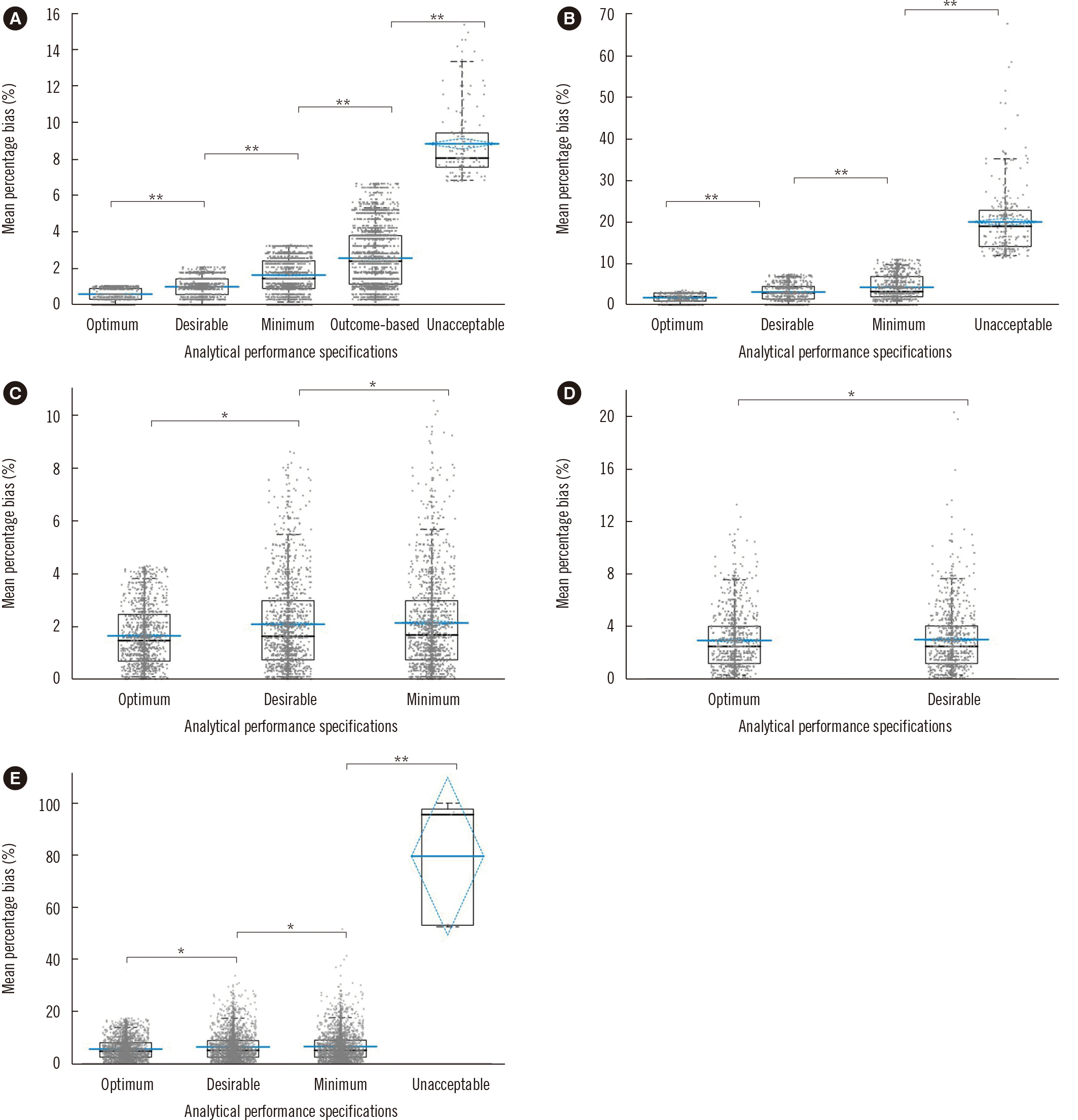
Fig. 5
Box plot analysis of mean percentage bias for cardiac troponin I grouped into six manufacturers (A–F) according to different performance goals. Bars represent means, and error bars represent 95% confidence intervals. Groups were compared using the Student–Newman–Keuls multiple-comparison test. **P≤0.001 and *P≤0.05.
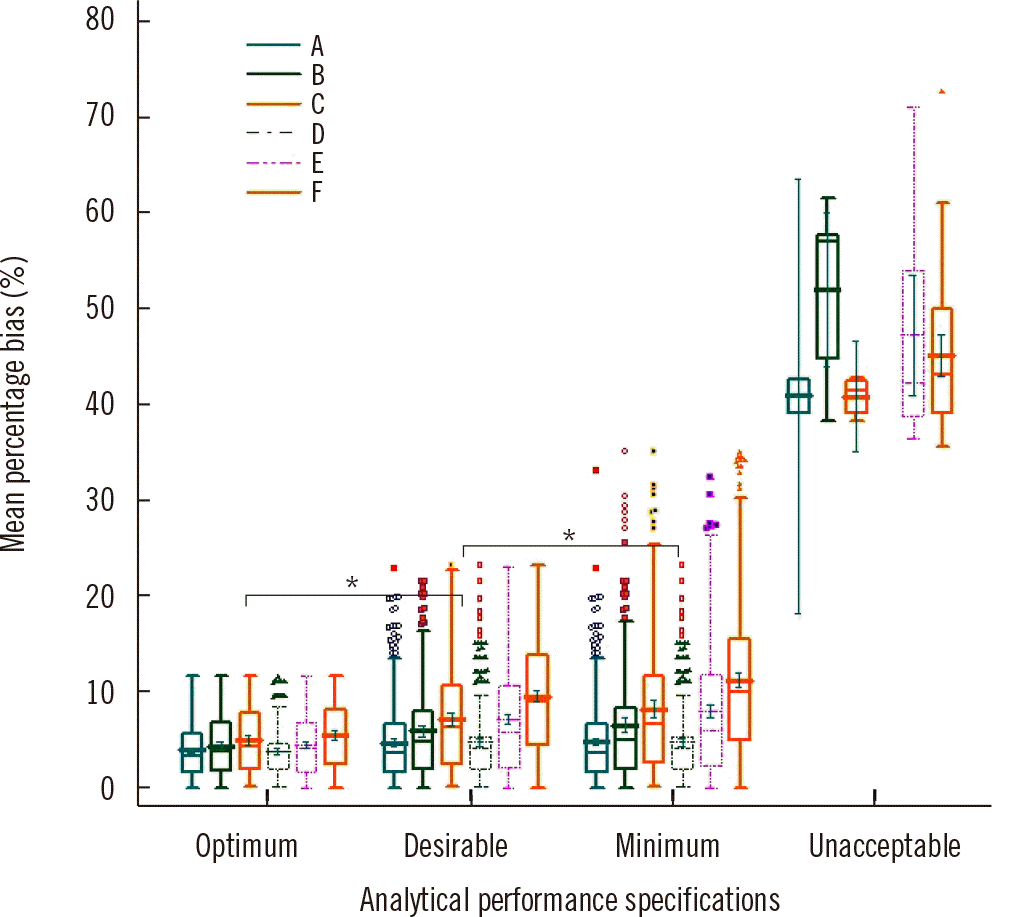
Table 1
Mean percentage bias according to analytical performance criteria