Abstract
Objectives
Although medical artificial intelligence (AI) systems that assist healthcare professionals in critical care settings are expected to improve healthcare, skepticism exists regarding whether their potential has been fully actualized. Therefore, we aimed to conduct a qualitative study with physicians and nurses to understand their needs, expectations, and concerns regarding medical AI; explore their expected responses to recommendations by medical AI that contradicted their judgments; and derive strategies to implement medical AI in practice successfully.
Methods
Semi-structured interviews were conducted with 15 healthcare professionals working in the emergency room and intensive care unit in a tertiary teaching hospital in Seoul. The data were interpreted using summative content analysis. In total, 26 medical AI topics were extracted from the interviews. Eight were related to treatment recommendation, seven were related to diagnosis prediction, and seven were related to process improvement.
Results
While the participants expressed expectations that medical AI could enhance their patients’ outcomes, increase work efficiency, and reduce hospital operating costs, they also mentioned concerns regarding distortions in the workflow, deskilling, alert fatigue, and unsophisticated algorithms. If medical AI decisions contradicted their judgment, most participants would consult other medical staff and thereafter reconsider their initial judgment.
Conclusions
Healthcare professionals wanted to use medical AI in practice and emphasized that artificial intelligence systems should be trustworthy from the standpoint of healthcare professionals. They also highlighted the importance of alert fatigue management and the integration of AI systems into the workflow.
The usefulness of artificial intelligence (AI) has been demonstrated in diverse industries [1,2]. AI has garnered attention in the medical field as a technology that promises improvements in efficiency, quality, and costs [3]. In particular, strategies to expand Electronic Medical Records (EMRs) [4] and the movement to comply with health data standardization [5,6] have increased the volume of quantitative data. This has launched the rapid progression of AI in medicine [7,8]. Consequently, there have been noteworthy outcomes, particularly in image data reading in dermatology, pathology, and radiology [9,10].
Patients admitted to critical care settings, such as the emergency room (ER) and intensive care unit (ICU), have high levels of urgency, severity, and complexity. Making an accurate diagnosis promptly and providing appropriate treatment are crucial for a favorable prognosis [11,12]. Accordingly, healthcare professionals working in these settings are uniquely positioned as they must make accurate decisions instantly [13]. Researchers in critical care fields have focused on predicting diagnoses and prognoses, recommending treatments, and performing triage [14,15].
Although medical AI systems are expected to enhance healthcare, whether their potential has been fully actualized is unclear. Studies have found that healthcare professionals believe that AI can improve healthcare and positively affect clinical performance [16]. Conversely, given that health information technology requires a fundamental change among stakeholders and in the surrounding environment, clinical decision support system (CDSS) researchers have closely examined the after-effects of the implementation of medical AI systems [17]. AI does not seem to have successfully become part of clinical practice, and studies have reported various unintended consequences of many CDSSs. These include patient safety threats [18], burnout with electronic health records [19,20], and alert fatigue [21,22].
Accordingly, we conducted a qualitative study among physicians and nurses working in a hospital’s ER and ICU to address the following objectives: (1) to understand the needs, expectations, and concerns of healthcare professionals related to medical AI in critical care settings; (2) to explore the anticipated responses of an AI system if it were to provide advice or recommendations contradicting a healthcare professional’s medical judgment; and (3) to derive strategies to help successfully apply medical AI in critical care settings, using insights from healthcare professionals.
The study was conducted at a 2,000-bed tertiary teaching hospital in Seoul. Eight physicians and seven nurses working in the ER and ICU were recruited via convenience sampling. Eight participants worked in the ER, and seven worked in the ICU. The participants’ mean age was 35 years, and 73.3% were women. On average, the participants had 10.2 years of healthcare experience. Five participants could explain medical AI conceptually, and four could explain it by referring to appropriate example cases. The participants’ characteristics are presented in Table 1.
One of the first authors, JY—who conducted all the interviews— is an informatician with 5 years of experience as a critical care nurse in the emergency department and cardiac intensive care unit. The other first author, SH, is a graduate student majoring in digital health and has experience in the cardiac intensive care unit and quality control section of the study’s site. Both researchers participated in seminars and workshops on qualitative research before conducting this research. The co-author, WH, is a professor in the Department of Industrial Information System Engineering, and teaches the “HCI Research Methodology” course, which primarily deals with qualitative research methods and analysis for graduate students. He has also published papers on studies that utilized qualitative research methods. The corresponding author, WCC, is a faculty member in the Department of Emergency Medicine and Digital Health.
Before the main study, a pilot study was conducted with an ER physician and an ICU nurse to finalize the semi-structured interview questionnaire (Table 2). The pilot study interviews were not included in the overall results. We focused on (1) clinical challenges that may be solved by introducing medical AI; (2) outcome predictions and data that may be used to make them; (3) the impact of medical AI implementation on patients, healthcare professionals, and the hospital; (4) the anticipated challenges in using medical AI in practice; (5) anticipated responses if AI reached a conclusion contradictory to the participants’ judgment; and (6) strategies to successfully apply medical AI in practice.
Participants were informed of the study’s purpose, and they signed a written consent form. The interviews proceeded in an open format, based on semi-structured questions. One researcher, who was a clinical informatician and a nurse with 5 years of experience working in the ER and ICU at the target hospital, conducted all the interviews. All interviews were audio-recorded, and non-verbal communication was manually recorded by a research assistant. Upon completion of the interview, the participants were paid an incentive of 50,000 KRW each (approximately 38 USD).
The interviews were conducted in the conference room of the study site. The average duration of the interviews was approximately 26 minutes per person, not including the 20 minutes that were taken for the consent acquisition process.
Once an interview was complete, two research assistants iteratively listened to the audio recordings and transcribed them, incorporating records of non-verbal communication into it. The data were interpreted through summative content analysis [23]. We employed this method (summative content analysis) to identify and quantify concepts, based on healthcare professionals’ perspectives, that should be considered for the successful clinical implementation of medical AI. Content analysis is a method that is widely used to acquire new insights from documents or written communications by describing meaningful categories and analyzing patterns. This method consists of three phases: (1) the preparation phase (designing the study’s setting and sampling strategy), (2) the organizing phase (concept generation and developing structured matrices), and (3) the reporting phase (descriptive statistics based on the frequency of the concepts). The source data were iteratively read and coded by three researchers working independently (including a research assistant) focusing on meaningful words and phrases. After the researchers determined the final codes, which were the minimal units of analysis, another researcher re-read the source interview data to assign each of the final codes. They then operationally defined the subcategories and categories based on the coding. The frequencies of the keywords were counted for each category and subcategory to understand the data’s context, and the quantitative results were interpreted.
R version 3.6.1 and the RQDA packages were used for data coding, pattern recognition, categorization, and visualization.
Audio recordings totaling 6 hours, 36 minutes, and 49 seconds were collected from the interviews. These were transcribed into a 139-page document comprising 39,268 words.
A total of 26 topics related to AI algorithms were derived from the data. These topics were classified on two axes, comprising the objectives and procedures. The classifications are presented in Table 3. Eight topics were classified under treatment recommendations, seven were classified under diagnosis prediction, and eight were classified under process improvement.
The participants’ responses regarding medical AI systems’ potential to enhance healthcare were classified into three levels: patient, medical staff, and institution. The participants noted that patient safety would increase through fewer complications, thereby improving patient outcomes (Figure 1). They also expressed the opinion that medical AI can fulfill patients’ right to knowledge and potentially provide patients with personalized precision medicine.
“The occurrence of delirium among patients will decrease. Patients will experience less pain, and, consequently, hospital stays and unplanned intubation will also decrease. Since additional drugs are administered if delirium occurs, I think it will also prevent administering unnecessary drugs.” (ICU charge nurse)
“It will reduce the time spent pondering the correct diagnosis and performing diagnostic procedures or techniques. Overall, the greatest advantage would be faster decision-making.” (Emergency medicine resident)
“It would be good if it rapidly makes an adjustment to find the optimal values if the respirator settings are off.” (Pulmonology fellow)
Most participants stated that medical AI would also benefit medical staff (Figure 2) and that it would reduce their workload by reducing the decision-making time and managing tasks that must be repeatedly performed. This would allow them to concentrate on other essential tasks and, thus, enhance their work efficiency. Additionally, they mentioned the psychological effects resulting from the decreased burden and emotional labor by delegating monitoring work to medical AI. A few participants expressed that trust between doctors and nurses may increase through the solidification of a communication channel.
“Since managers’ workloads will drastically reduce, they can focus on other details. They could afford to allocate time to quality improvement, human resource management, or consultations. Since nurses’ workload tends to increase steadily, it would be nice if AI could assist with this.” (ER head nurse)
“Selecting appropriate empirical antibiotics requires much attention from a doctor. If such a program is developed, it would greatly lessen the time infectious disease specialists spend on simple tasks, like determining how many antibiotics individual patients currently take. They could spend more time on research or nosocomial infection management.” (Infectious disease associate)
Further, the participants thought that medical AI algorithms might help with hospital management (Figure 3) and that medical AI has the potential to reduce medical malpractice disputes, labor costs, and unnecessary procedures and treatments. They believed that the resulting cost reductions would promote financial gain and contribute to improving direct profit through an increase in the bed occupancy rate. They also stated that improved healthcare quality resulting from the use of medical AI would, in turn, increase satisfaction among their patients and their families and thereby enhance the hospital’s image. They expected improved work processes to initiate attendant effects, such as resolving over-crowdedness and increasing departmental efficiency.
“I think malpractice suits may be reduced because the unanticipated worsening of patient status would occur less often.” (Critical care medicine faculty)
“Administering one or two preventive drugs could prevent complications with blood transfusion. If a complication occurs, we are charged for the cost of the many treatments given to the patient. There would be savings in areas like these.” (ER staff nurse)
“In the ER, how long it takes to refer a patient to another department varies daily depending on how crowded the ER is. It takes three to six hours and often longer. During this time, continuing to manage the patient and holding them for a single decision to be made leaves very little room to care for new patients.” (Emergency medicine faculty)
The participants also expressed concerns regarding medical AI (Figure 4). They were primarily concerned about distortions in the workflow, such as additional work required to execute the algorithms and the possibility that healthcare professionals’ right to treat patients autonomously may be limited. Additional concerns included an overdependence on algorithms, which could cause deskilling among less experienced health professionals, alert fatigue desensitizing of medical staff to alerts, and AI’s lack of ability to incorporate information that is not in the EMR. The participants argued that discourse would be needed to determine who would be accountable if a patient’s status worsened after a medical decision was made following the guidance of a medical AI algorithm. A few participants mentioned concerns about AI damaging the rapport between patients and medical staff due to distortions in the workflow.
“If we follow the algorithm, we may have to measure blood glucose every hour, whereas previously it was measured four times. The workload would overwhelmingly increase. It’s not like AI can even take blood, either. Hence, people are not willing to use the currently implemented CDSS for blood glucose control.” (Intensive care medicine fellow)
“In medical decision-making, wouldn’t it be difficult for the algorithms to consider the information that medical staff members share only in conversation, minor information that did not get saved in the EMR, or emotional information?” (Emergency medicine resident)
Various opinions were expressed regarding the expected responses if a decision recommended by medical AI was contrary to the participants’ judgment. Most participants stated that they would consult with another healthcare professional for a second opinion. The subsequent most frequent response was that they would reconsider their initial opinion. Compared to participants who were supervised by other healthcare professionals (residents and staff nurses), those making self-directed decisions (faculty members, associates, head nurses, and charge nurses) more frequently stated that they would review or reconsider their opinion, rather than move forward with the decision. In particular, drawing on the fact that medical AI algorithms make decisions based only on the variables included in the training datasets, these participants expressed that they would use more information to make informed decisions.
“AI is not 100% accurate, but it has a larger volume of data than I do, doesn’t it? My clinical judgment is based on my 5-year clinical experience, so if an algorithm suggests an option conflicting with my judgment, I will seek the opinions of other medical staff members and decide to prevent complications as entirely as possible.” (ER nurse)
“I believe I would need to consult an expert in the field.” (Intensive care medicine fellow)
“I would need to make the final decision after taking into account the variables not included in the EMR.” (Emergency medicine resident)
“I don’t think algorithms compete with me. It will be a situation where I need to think, ‘This is different from what I know. What is causing the difference?’ and investigate it.” (ICU charge nurse)
“People are the gold standard.” (ER charge nurse)
“It may be different depending on the level of experience. Doctors with a lot of experience will go ahead with their decisions, and those who do not trust themselves will rely on the algorithm.” (Emergency medicine resident)
Despite the concerns regarding medical AI algorithms, all participants intended to use medical AI-based CDSS. For the successful clinical implementation of such systems, they suggested enhancing the systems’ accuracy, gradually introducing AI systems into practice, and establishing and maintaining the trust of healthcare professionals through evidence-building (Figure 5). Additionally, they emphasized that machine learning should be performed using sufficient input variables to develop algorithms and that the training datasets should contain absolutely no errors. Conversely, the importance of alert fatigue management and integration into the workflow was emphasized. Furthermore, legal issues about medical decision-making based on algorithms, the realignment of institutional culture to introduce and reinforce the use of algorithms, and manageable costs for system integration and the use of algorithms were mentioned.
“I think the use of AI would be terminated if there was no difference in outcomes when AI is used versus before, or if the outcomes were worse. It seems that AI implementation should start with where there will be no harm and be gradually increased if it is reasonably effective.” (ER staff nurse)
“Data can have errors, and it is necessary to locate such errors.” (ICU head nurse)
“People working at hospitals, particularly nurses, feel burdened by doing something new. If what has been followed a certain way is changed even slightly, the first reaction is sometimes resistance. Out of 10, two to three will strongly resist.” (ER head nurse)
“AI can help in areas like decision-making, but if it is expensive to purchase or maintain the programs, there is no reason why humans cannot do the work instead of AI.” (Emergency medicine fellow)
This study was conducted with healthcare professionals working in an acute care setting to understand their needs, expectations, and concerns regarding medical AI. We also aimed to explore the expected responses to a decision by medical AI that contradicted their judgment and derive strategies for the successful implementation of medical AI in practice. Medical AI has made a series of advances in well-defined problems based on machine learning by utilizing abundant clinical data [10]. However, it has also been challenging to actualize its influential power in clinical practice due to algorithm failures [24].
The study confirmed that healthcare professionals in critical care settings need the help of medical AI in diagnosis, treatment, and process improvement. The first step in the development and implementation of CDSSs is recognizing healthcare professionals’ clinical needs [25]. Machine learning techniques are perceived as a general-purpose technology in many fields, and their scope of implementation has been expanded. However, in medicine, the focus of medical AI is diagnosis and treatment [15]. ERs and ICUs are unique healthcare environments, where diagnoses are made and treatment is provided based on patients’ rapidly changing statuses [11,12]. Many topics in this study also pertain to diagnosis and treatment. Furthermore, the study confirmed critical care healthcare professionals’ need to improve work processes as a severe limitation in the available human resources in Korean ERs and ICUs [26,27]. Our findings present a starting point to extend the scope of future medical AI research and implementation by suggesting various topics for AI algorithms to automate and apply in the critical care field.
Several participants expected medical AI to help them perform their jobs more efficiently, while simultaneously being concerned that their workload may increase after its introduction [24]. The need to perform unnecessary assessments to operate medical AI algorithms, redundant data input due to the inability of AI algorithms to automatically access information stored in the EMR, and alert fatigue because of unsophisticated functionality have consistently been mentioned as problems both in previous studies [8,28] and in this study. These factors cause healthcare professionals to regard AI systems as cumbersome and prevent them from appreciating the potential gains offered. Therefore, medical AI researchers should consider these challenges when designing and implementing AI systems [15,29].
The importance of trust in medical AI algorithms in clinical implementation cannot be overlooked. The participants emphasized the importance of the volume and quality of transparent data and transparency in the overall process, including data acquisition and preprocessing [30]. The systems should be designed such that they can be trusted by healthcare professionals to successfully apply medical AI. The overall data-related process must be carefully managed, recorded, monitored, and evaluated when the systems are used in practice.
As this study was conducted with a convenience sample of healthcare professionals working in the ER and ICU of a tertiary teaching hospital in Korea, caution should be taken not to overinterpret its findings. Further quantitative studies are needed to confirm the effect size of each of the categories extracted in this study and develop a conceptual model. Finally, one physician working in the ED participated in both the pilot and main interviews.
This study confirmed that healthcare professionals in ERs and ICUs have high expectations that the introduction of medical AI technology will perform a beneficial role in their work and benefit their patients. However, they have concerns regarding diverse issues, ranging from fundamental problems in machine learning to implementation problems and legal, institutional, and ethical issues. The study is significant as it presents insights into the expectations and concerns about medical AI among healthcare professionals in critical care settings who will use AI algorithms in practice. The study also suggests strategies for the successful clinical implementation of medical AI.
Acknowledgments
This research was supported by the Korea Health Technology Research and Development Project through the Korea Health Industry Development Institute, funded by the Ministry of Health and Welfare, Republic of Korea (No. HI19C0275) and the Basic Science Research Program through the National Research Foundation of Korea (NRF), funded by the Ministry of Education (No. 2021R1F1A1052072).
References
1. Wuest T, Weimer D, Irgens C, Thoben KD. Machine learning in manufacturing: advantages, challenges, and applications. Prod Manuf Res. 2016; 4(1):23–45.
https://doi.org/10.1080/21693277.2016.1192517
.
2. Henrique BM, Sobreiro VA, Kimura H. Literature review: machine learning techniques applied to financial market prediction. Expert Syst Appl. 2019; 124:226–51.
https://doi.org/10.1016/j.eswa.2019.01.012
.
3. Matheny ME, Whicher D, Thadaney Israni S. Artificial intelligence in health care: a report from the National Academy of Medicine. JAMA. 2020; 323(6):509–10.
https://doi.org/10.1001/jama.2019.21579
.
4. Chae YM, Yoo KB, Kim ES, Chae H. The adoption of electronic medical records and decision support systems in Korea. Healthc Inform Res. 2011; 17(3):172–7.
https://doi.org/10.4258/hir.2011.17.3.172
.
5. Bender D, Sartipi K. HL7 FHIR: An Agile and REST-ful approach to healthcare information exchange. In : Proceedings of the 26th IEEE International Symposium on Computer-based Medical Systems; 2013 Jun 20–22; Porto, Portugal. p. 326–31.
https://doi.org/10.1109/CBMS.2013.6627810
.
6. Klann JG, Joss MA, Embree K, Murphy SN. Data model harmonization for the All of Us Research Program: transforming i2b2 data into the OMOP common data model. PLoS One. 2019; 14(2):e0212463.
https://doi.org/10.1371/journal.pone.0212463
.
7. Ahuja AS. The impact of artificial intelligence in medicine on the future role of the physician. PeerJ. 2019; 7:e7702.
https://doi.org/10.7717/peerj.7702
.
8. Kruse CS, Goswamy R, Raval Y, Marawi S. Challenges and opportunities of big data in health care: a systematic review. JMIR Med Inform. 2016; 4(4):e38.
https://doi.org/10.2196/medinform.5359
.
9. Loh E. Medicine and the rise of the robots: a qualitative review of recent advances of artificial intelligence in health. BMJ Lead. 2018; 1–5.
https://doi.org/10.1136/leader-2018-000071
.
10. Buch VH, Ahmed I, Maruthappu M. Artificial intelligence in medicine: current trends and future possibilities. Br J Gen Pract. 2018; 68(668):143–4.
https://doi.org/10.3399/bjgp18X695213
.
11. Cha WC, Cho JS, Shin SD, Lee EJ, Ro YS. The impact of prolonged boarding of successfully resuscitated out-of-hospital cardiac arrest patients on survival-to-discharge rates. Resuscitation. 2015; 90:25–9.
https://doi.org/10.1016/j.resuscitation.2015.02.004
.
12. Kang J, Kim J, Jo YH, Kim K, Lee JH, Kim T, et al. ED crowding and the outcomes of out-of-hospital cardiac arrest. Am J Emerg Med. 2015; 33(11):1659–64.
https://doi.org/10.1016/j.ajem.2015.08.002
.
13. Florkowski C, Don-Wauchope A, Gimenez N, Rodriguez-Capote K, Wils J, Zemlin A. Point-of-care testing (POCT) and evidence-based laboratory medicine (EBLM): does it leverage any advantage in clinical decision making? Crit Rev Clin Lab Sci. 2017; 54(7–8):471–94.
https://doi.org/10.1080/10408363.2017.1399336
.
14. Hong WS, Haimovich AD, Taylor RA. Predicting hospital admission at emergency department triage using machine learning. PLoS One. 2018; 13(7):e0201016.
https://doi.org/10.1371/journal.pone.0201016
.
15. Johnson AE, Ghassemi MM, Nemati S, Niehaus KE, Clifton DA, Clifford GD. Machine learning and decision support in critical care. Proc IEEE Inst Electr Electron Eng. 2016; 104(2):444–66.
https://doi.org/10.1109/JPROC.2015.2501978
.
16. Pinto Dos Santos D, Giese D, Brodehl S, Chon SH, Staab W, Kleinert R, et al. Medical students’ attitude towards artificial intelligence: a multicentre survey. Eur Radiol. 2019; 29(4):1640–6.
https://doi.org/10.1007/s00330-018-5601-1
.
17. Zheng K, Haftel HM, Hirschl RB, O’Reilly M, Hanauer DA. Quantifying the impact of health IT implementations on clinical workflow: a new methodological perspective. J Am Med Inform Assoc. 2010; 17(4):454–61.
https://doi.org/10.1136/jamia.2010.004440
.
18. Ash JS, Berg M, Coiera E. Some unintended consequences of information technology in health care: the nature of patient care information system-related errors. J Am Med Inform Assoc. 2004; 11(2):104–12.
https://doi.org/10.1197/jamia.M1471
.
19. Shanafelt TD, Dyrbye LN, West CP. Addressing physician burnout: the way forward. JAMA. 2017; 317(9):901–2.
https://doi.org/10.1001/jama.2017.0076
.
20. Sequeira L, Almilaji K, Strudwick G, Jankowicz D, Tajirian T. EHR “SWAT” teams: a physician engagement initiative to improve Electronic Health Record (EHR) experiences and mitigate possible causes of EHR-related burnout. JAMIA Open. 2021; 4(2):ooab018.
https://doi.org/10.1093/jamiaopen/ooab018
.
21. Sendelbach S, Funk M. Alarm fatigue: a patient safety concern. AACN Adv Crit Care. 2013; 24(4):378–86.
https://doi.org/10.1097/NCI.0b013e3182a903f9
.
22. Yoo J, Lee J, Rhee PL, Chang DK, Kang M, Choi JS, et al. Alert override patterns with a medication clinical decision support system in an academic emergency department: retrospective descriptive study. JMIR Med Inform. 2020; 8(11):e23351.
https://doi.org/10.2196/23351
.
23. Bengtsson M. How to plan and perform a qualitative study using content analysis. NursingPlus Open. 2016; 2:8–14.
https://doi.org/10.1016/j.npls.2016.01.001
.
24. Yu KH, Kohane IS. Framing the challenges of artificial intelligence in medicine. BMJ Qual Saf. 2019; 28(3):238–41.
https://doi.org/10.1136/bmjqs-2018-008551
.
25. Shiffman RN. Best practices for implementation of clinical decision support. Berner E, editor. Clinical decision support systems. Cham, Switzerland: Springer;2016. p. 99–109.
https://doi.org/10.1007/978-3-319-31913-1_6
.
26. Lee YJ, Shin SD, Lee EJ, Cho JS, Cha WC. Emergency department overcrowding and ambulance turnaround time. PLoS One. 2015; 10(6):e0130758.
https://doi.org/10.1371/journal.pone.0130758
.
27. Offenstadt G, Moreno R, Palomar M, Gullo A. Intensive care medicine in Europe. Crit Care Clin. 2006; 22(3):425–32.
https://doi.org/10.1016/j.ccc.2006.03.007
.
28. Ranji SR, Rennke S, Wachter RM. Computerised provider order entry combined with clinical decision support systems to improve medication safety: a narrative review. BMJ Qual Saf. 2014; 23(9):773–80.
https://doi.org/10.1136/bmjqs-2013-002165
.
29. Sittig DF, Wright A, Coiera E, Magrabi F, Ratwani R, Bates DW, et al. Current challenges in health information technology-related patient safety. Health Informatics J. 2020; 26(1):181–9.
https://doi.org/10.1177/1460458218814893
.
30. He J, Baxter SL, Xu J, Xu J, Zhou X, Zhang K. The practical implementation of artificial intelligence technologies in medicine. Nat Med. 2019; 25(1):30–6.
https://doi.org/10.1038/s41591-018-0307-0
.
Figure 1
Critical care providers’ expectations regarding AI’s impact on patients. AI: artificial intelligence.
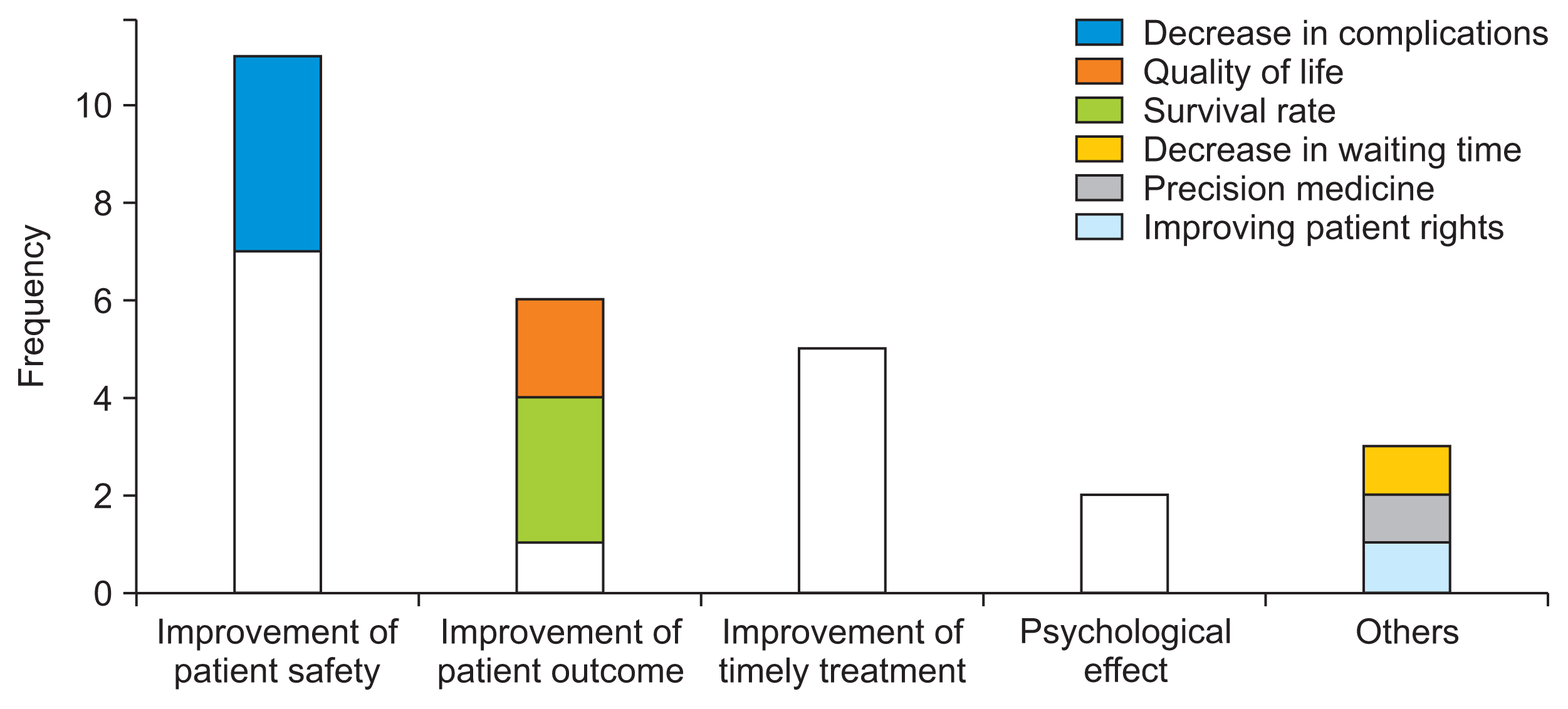
Figure 2
Critical care providers’ expectations regarding AI’s impact on caregivers. AI: artificial intelligence.
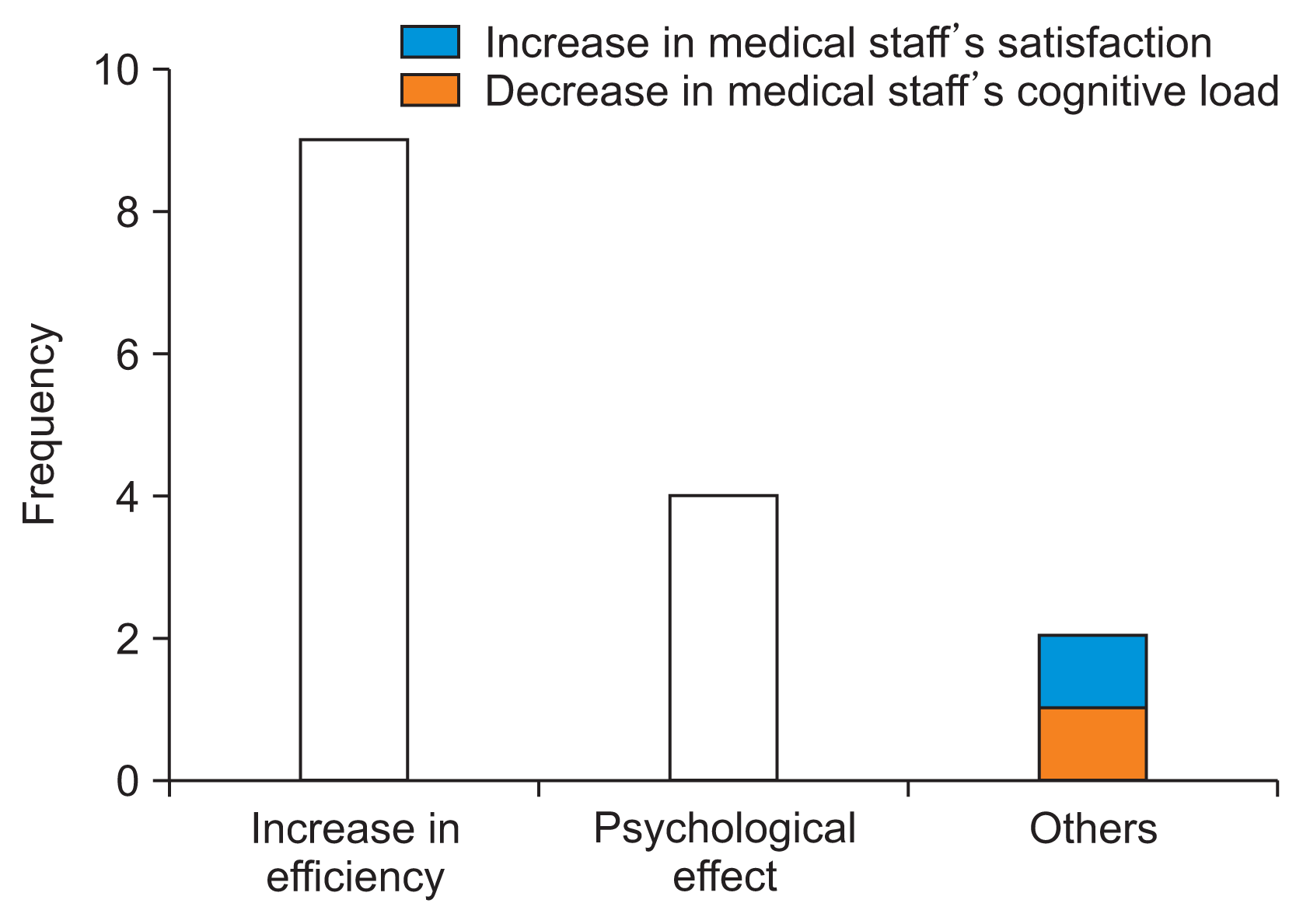
Figure 3
Critical care providers’ expectations regarding AI’s impact on institutions. AI: artificial intelligence.
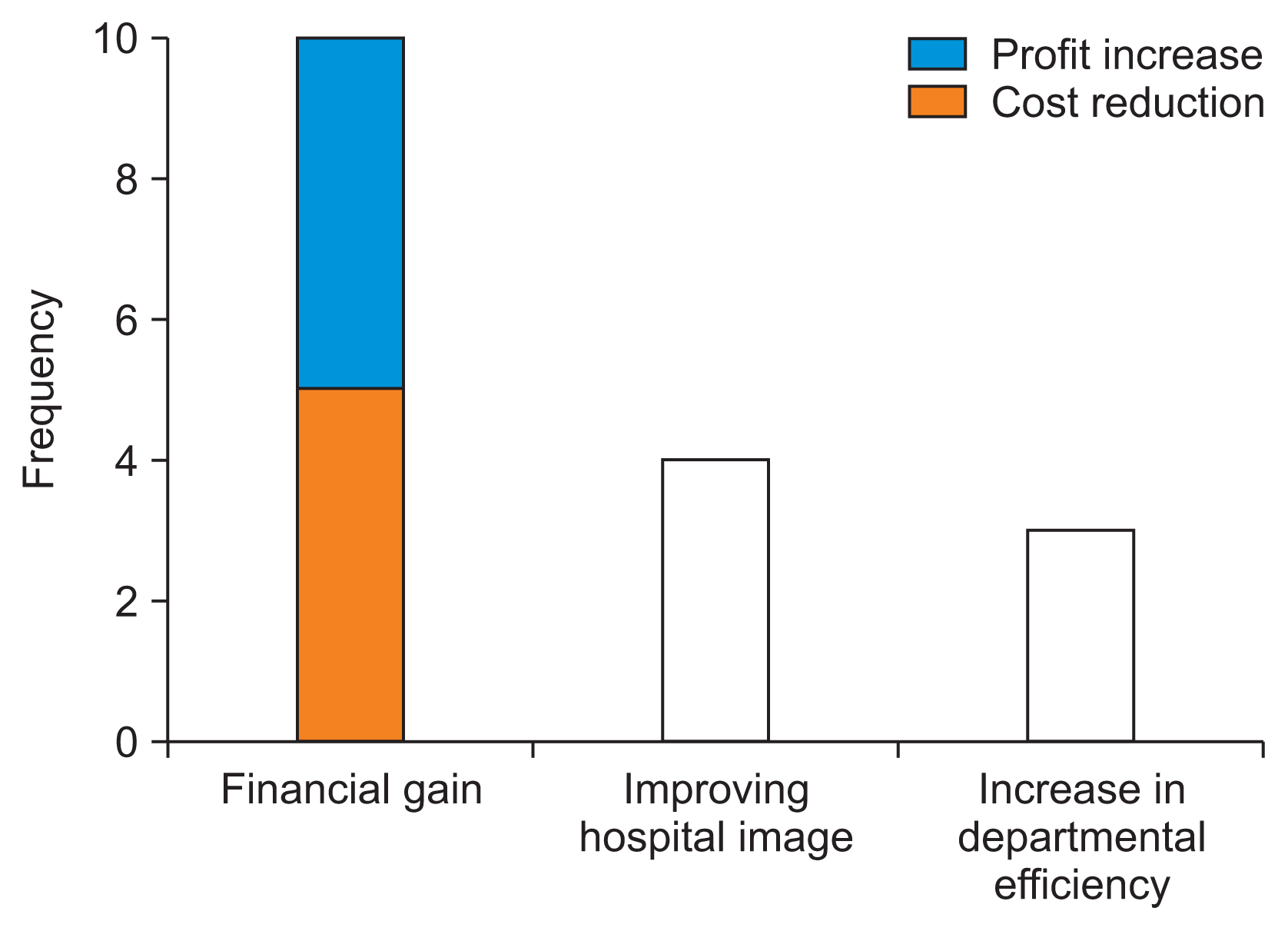
Figure 4
Critical care providers’ concerns regarding the impact of medical AI (artificial intelligence).
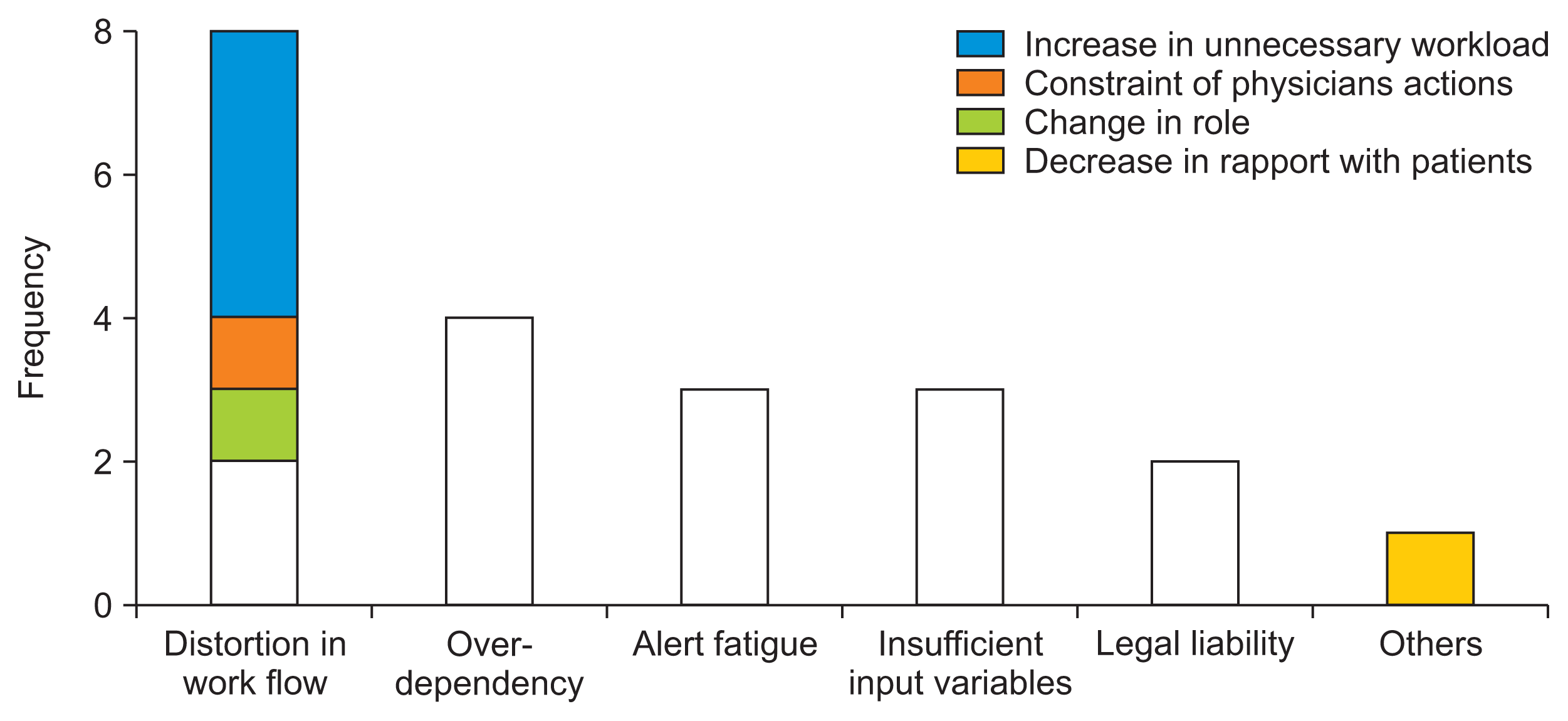
Figure 5
Strategies for the successful clinical implementation of medical AI (artificial intelligence).
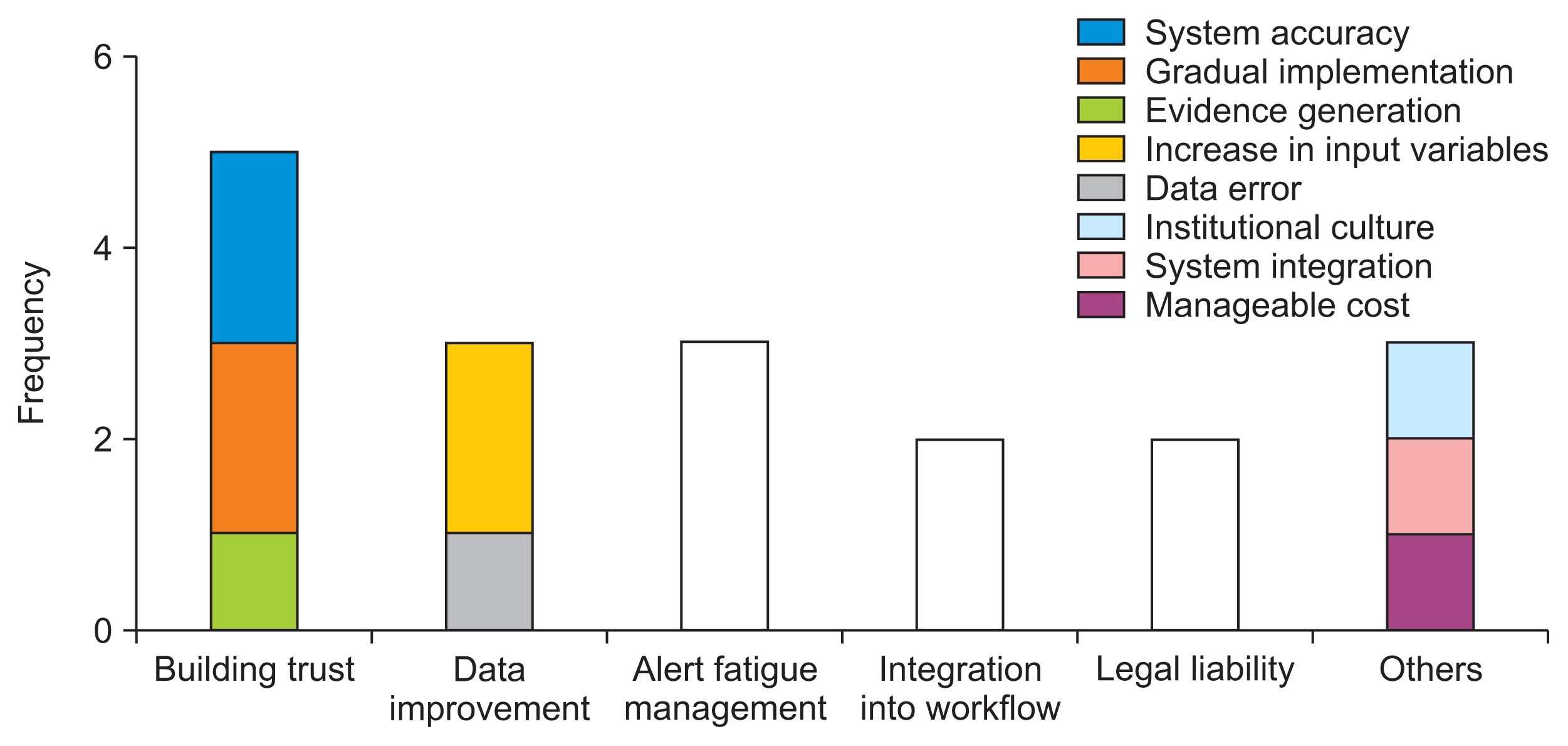
Table 1
Participants’ characteristics
Table 2
Semi-structured interview questions
Table 3
Classification of the topics on medical AI derived from the clinical providers