INTRODUCTION
Staphylococcus aureus (S. aureus) is one of the significant human pathogen in clinical medicine. It is highly associated with antimicrobial resistance and has the potential to infect virtually all types of tissue causing skin infections, pneumonia, osteomyelitis, and endocarditis (1). Because of its ability to develop resistance against antibiotics treatment, new interventions like novel classes of antibiotics and vaccines are urgently needed (2).
During infection, S. aureus requires essential nutrients from host for multiplication and virulence expression. In particular, iron is a crucial element highly associated with biosynthesis of cellular building blocks and electron transport for cellular respiration (3). For efficient uptake, transport, and storage of iron, S. aureus has evolutionarily developed a wide variety of iron acquisition system (4, 5, 6).
S. aureus acquires iron from the host present as multiple types including siderophores, xenosiderophores, heme, and inorganic free iron (7). Despite the different types of iron, major acquisition system of iron is quite similar: each of the systems consists of an iron-regulated membrane protein as a major iron transporter, a high-affinity membrane-anchored lipoprotein, and several accessory proteins (8). Especially, the iron-regulated lipoproteins are essential for specific recognition and binding of the iron-associated molecules as well as interaction with its cognate iron transporter facilitating translocation of iron into the cell (9, 10, 11).
Bacterial lipoproteins are lipid-posttranslationally modified proteins that play a role in nutrient transport, signal transduction, or working as toxins. S. aureus possesses five major iron-regulated lipoproteins (IRLPs): IsdE, SstD, SirA, HtsA, and SitC (Fig. 1). IsdE is a one of the component in the iron-regulated surface determinant pathway (Isd) for iron acquisition from heme and hemoproteins (12, 13). It supports the heme shuttle system within the bacterial cell wall and membrane. SstD is a receptor for catechol siderophores via the SstABCD ABC transporter (14). The Sst locus contributes to growth promotion and in vivo colonization of the mouse liver and kidneys. SirA and HtsA are receptors for staphyloferrin siderophores that have high-affinity for ferric iron (15). The two lipoproteins provide uptake specificity for staphyloferrin molecules to their cognate receptors. SitC is the component of SitABC and also homologous to the manganese-binding MntC protein (16). It was first described as an immunogenic lipoprotein component of iron-regulated ABC transporter, strongly reacting with antibodies from pooled human peritoneal dialysate (17). These proteins have residence in extracellular locations and activate the host being as immunogenic innate immune response via Toll-like receptor-2 (TLR-2), suggesting as potent vaccine targets against S. aureus infection.
Fig. 1
Schematic diagram of IRLPs. IsdE, SstD, SirA/HtsA, and SitC are lipoproteins involved in iron acquisition systems for heme, catechol siderophores, staphyloferrin siderophores, and free iron, respectively.
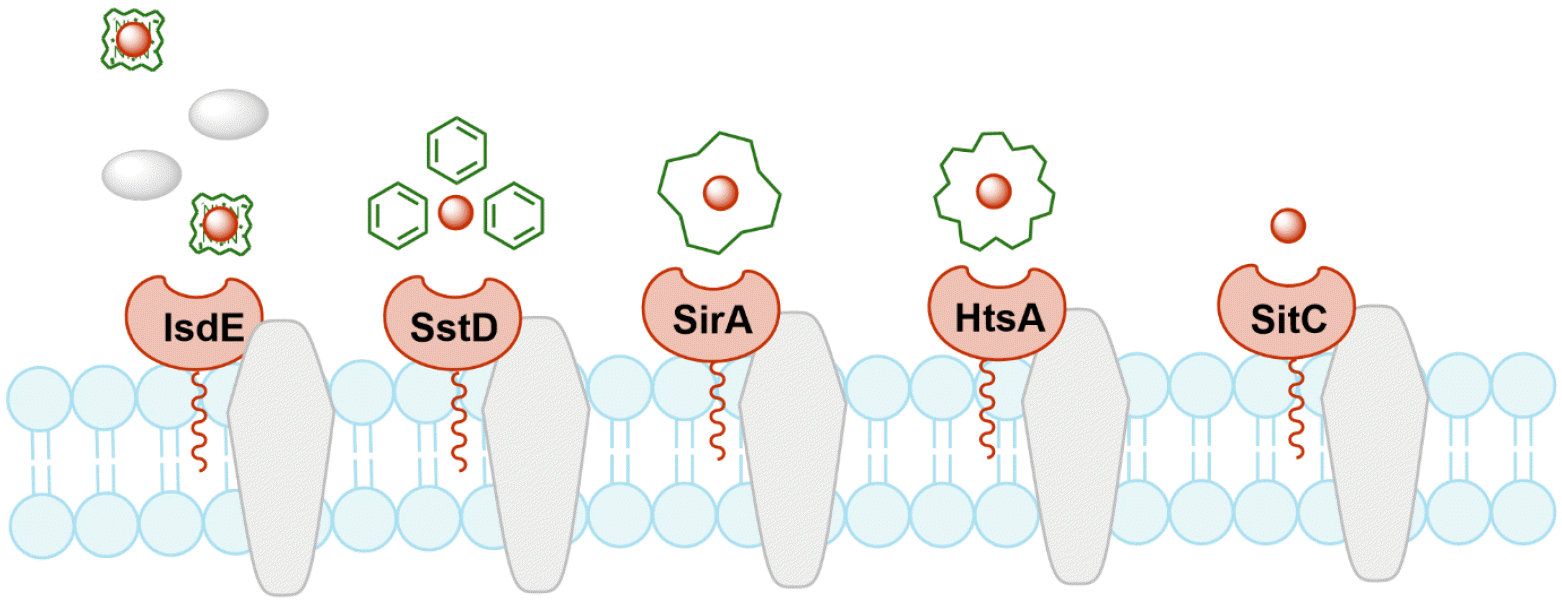
To date, many vaccine models against S. aureus have been developed or in the evaluation stages (18, 19, 20, 21). However, most of the vaccines have failed resulting from severe toxicity or allergic reactions (22, 23). Immunoinformatics is a computational immunology playing a great role in simulating immune responses and defining new vaccine targets (24). In this study, we designed multi-epitope vaccines targeting S. aureus IRLPs, IsdE, SstD, SirA, HtsA, and SitC, and evaluated their predicted physicochemical properties, effectiveness and safety based on the immunoinformatic approaches.
MATERIALS AND METHODS
Sequence retrieval
Amino acid sequences of the five IRLPs were retrieved from National Center for Biotechnology Information with ID UFK83736, WP_000754443, WP_001045111, WP_001214661, and WP_000737654 for IsdE, SstD, SirA, HtsA, and SitC, respectively.
B cell epitope prediction
BepiPred-2.0 was used for prediction of the B cell epitopes (https://services.healthtech.dtu.dk/service.php?BepiPred-2.0) (25). Predicted epitopes greater than a threshold of 0.6 were selected potent epitopes.
T cell epitope prediction
For T cell epitope prediction, most frequent alleles in Korean were considered including HLA-A*24:02, HLA-A*02:01, and HLA-DRB*09:01 (26). HLA-I epitopes of IRLPs were predicted with NetCTLpan 1.1 server with the threshold value of 1.0 for HLA-A*24:02, HLA-A*02:01 (https://services.healthtech.dtu.dk/service.php?NetCTLpan-1.1) (27). HLA-II epitopes of IRLPs were predicted with NetMHCIIpan 4.0 server with the threshold value (% Rank) of 1 and 5 for strong and weak binders, respectively, against HLA-DRB*09:01 (https://services.healthtech.dtu.dk/service.php?NetMHCIIpan-4.0) (28).
Vaccine construction
Multi-epitope sequences for B cell and T cell recognition were generated with linkers and adjuvant sequences for each IRLPs. At the N-terminal end, β-defensin and pan DR-binding epitope (PADRE) sequences were used as adjuvant with the EAAAK linker, which are well known to improve immunogenicity of the vaccine as an anti-microbial peptide involved in innate immunity and as an epitope with high affinity to human HLA-DR types, respectively (29, 30). AAY, GPGPG, and KK sequences were added to join cytotoxic T cell, helper T cell, and B cell epitopes, respectively. At the C-terminal end, six histidine tag was added to aid protein purification. Prediction of physicochemical properties of the vaccine construct was performed by ProtParam (https://web.expasy.org/protparam) (31).
Prediction of antigenicity, allergenicity, interferon-γ inducibility and toxicity
Antigenicity of the in silico processed vaccine constructs was predicted by VaxiJen with the threshold value of 0.5 for a probable antigen (http://www.ddg-pharmfac.net/vaxijen/VaxiJen/VaxiJen.html) (32). Allergenicity of the constructs was determined by AllerTOP 2.0 (https://www.ddg-pharmfac.net/AllerTOP/index.html) (33). To predict the interferon-γ (IFN- γ ) inducibility of the epitopes, IFNepitope, a server for predicting interferon-γ inducing regions in a protein, was used with motif and support vector machine (SVM) hybrid (https://webs.iiitd.edu.in/raghava/ifnepitope/scan.php) (34). Possible toxicity of the construct was predicted by ToxinPred (https://webs.iiitd.edu.in/raghava/toxinpred/) (35).
Immune simulation
Immune simulation was performed using the C-IMMSIM server for prediction of immune cell population and immune responses (https://150.146.2.1/C-IMMSIM/index.php?page=1) (36). For simulating immune responses, host HLA alleles were selected based on the most frequent alleles in Korean including HLA-A*24:02, HLA-A*02:01, HLA-B*51:01, HLA-B*15:01, HLA-DRB1*09:01, and HLA-DRB1*04:05. Three injections of the vaccine construct without LPS were administered at intervals of approximately 4 weeks by following one-time step as 8 hours (time step of injection: 9, 93, and 177). Random seed, simulation volume, and simulation steps were wet to 12345, 10 μL, and 1050, respectively.
Structure prediction and molecular docking
The three-dimensional structures of IRLPs vaccine constructs were modelled using AlphaFold2.0 based on the Colab server (https://colab.research.google.com/github/sokrypton/ColabFold/blob/main/AlphaFold2.ipynb) (37). To analyze the possible interaction between the vaccine ligands and a receptor protein, TLR1-TLR2 heterodimer complex was selected as a receptor protein based on its possible interaction with IRLPs (PDB ID: 2Z80) (38). Molecular docking was carried out using the PatchDock server (https://bioinfo3d.cs.tau.ac.il/PatchDock) (39). The top 10 models were selected and refined using the FireDock server (https://bioinfo3d.cs.tau.ac.il/FireDock) and global binding energy was predicted.
RESULTS
Prediction of B cell and T cell epitopes
IRLPs are surface-exposed bacterial lipoproteins which have roles in iron acquisition and virulence expression. In order to design vaccine constructs targeting S. aureus IRLPs, multi-epitope sequences against immune cells were evaluated. B cell epitope is defined as an antigenic determinant that is recognized by the B cell receptor or antibodies. By BepiPred server, one or two B cell epitopes were predicted for each IRLPs: PEIGQPMEP/PEEVKKM for IsdE, KEQSKSETKGSKDTVKIENNYKMRGEK/KVSNSNH for SstD, NKQSSDNK/ KDLQKQVDNGKD for SirA, NSSKKESST/SDDVTKGLSKYLKG for HtsA, and KQGTPEQMR for SitC.
T cell recognize epitopes presented by major histocompatibility complex (MHC) molecules on the surface of antigen- presenting cells (40). MHC class I and II epitopes from the IRLPs were predicted targeting most frequently present alleles, which are HLA-A*24:02, HLA-A*02:01, and HLA-DRB*09:01 in Korean (Table 1) (26). Among the five IRLPs, the HLA-I epitopes from SitC showed higher prediction score compared with other proteins. On the other hand, IsdE showed higher prediction score as HLA-II epitopes that others while SirA and SitC were predicted to only have weak binding affinities. These results imply that multi-epitope structures derived from each IRLPs would have different immunogenic responses based on their sequences.
Table 1.
Prediction of T cell epitopes
Construction of vaccine antigens
Based on the prediction of immune cell epitopes, vaccine constructs of each IRLPs were designed (Table 2). To improve the immunogenic responses, β-defensin and PADRE sequences were added at the N-terminus, and specific linkers such as EAAAK, AAY, GPGPG, and KK were used for linking each peptide.
Table 2.
Vaccine construction with linkers (red), adjuvants (green), T cell epitopes (black), B cell epitopes (blue), and 6x Histidine tag (purple)
Sequence of the vaccine constructs |
![]() |
Table 3 presents physicochemical properties of each vaccine peptides calculated by ProtParam. The vaccine proteins are composed of 150-190 amino acids ranging 16-90 kDa molecular weight. Despite the different amino acid sequences, isoelectric points of the proteins were similar (9.7-9.9) which show the basic nature of the designed vaccine proteins. Grand average of hydropathicity (GRAVY) indices were all negative indicating that the vaccine structures are hydrophilic and interact well with water molecules. Instability of the designed proteins, an estimate of the stability of protein in a test tube, were reported to be 18 to 35 that is predicted as stable when its instability index is smaller than 40. Overall, the vaccine constructs of the five IRLPs have similar physicochemical properties with respect to molecular weight and isoelectric point and are considered to be hydrophilic and stable in water molecules.
Table 3.
Prediction of physicochemical properties of the vaccine constructs
Evaluation of the vaccine constructs
To predict the immunological properties of the vaccine constructs, antigenicity, allergenicity, toxicity, and IFN- γ inducibility were evaluated (Table 4). Antigenicity predicted by the VaxiJen v.2.0 server showed a score range of 0.6 to 1.0, which confirms that the constructs are antigenic. Allergenicity predicted by AllerTOP v.2.0 server indicated that all of the five vaccine constructs are non-allergen, as the native IRLPs are also not allergenic. None of the constructs were predicted to be toxic.
Table 4.
Prediction of antigenicity, allergenicity, toxicity and interferon-γ inducibility
Protein | Antigenicity | Allergenicity | Toxicity | Interferon-γ inducibility |
IsdE | 0.63 | Non-allergen | Non-toxic |
2/7 (epitopes 3, 6)a |
SstD | 1.06 | Non-allergen | Non-toxic |
2/8 (epitopes 7, 8) |
SirA | 0.92 | Non-allergen | Non-toxic |
3/8 (epitopes 4, 5, 7) |
HtsA | 0.77 | Non-allergen | Non-toxic |
3/6 (epitopes 1, 2, 4) |
SitC | 0.81 | Non-allergen | Non-toxic |
2/7 (epitopes 1, 3) |
IFN- γ is a soluble cytokine in type Ⅱ class of interferons and critical for innate and adaptive immunity against infections. IFN- γ inducibility was predicted by IFNepitope identifying MHC class Ⅱ binders that can activate IFN- γ inducing T-helper cells (34). As shown in Table 3, 2 or 3 epitopes of each vaccine constructs were predicted to be inducible for IFN- γ. Most of the constructs had similar level of IFN- γ inducible for their epitopes, and HtsA showed relatively higher positivity for IFN- γ inducing compared with other IRLP vaccines. Taken together, all of the multi-epitope IRLP vaccines designed in the present study would be antigenic, non-allergenic, non-toxic, and IFN- γ inducible.
In silico immune simulation
The immunogenic profile of the multi-epitope vaccines was simulated by the C-IMMSIM server shown in Figs. 2, 3, 4, 5, 6. The immune simulation results showed that the secondary and tertiary immune responses were more immunogenic than the primary one. For specific subclasses of antibodies, SstD, HtsA, and SitC vaccines showed a potently higher IgM production than IsdE or SitC vaccine constructs, although production profile of other types of antibodies was similar. In general, IgM is known as the primary antibody produced during an initial antigen challenge. Upon subsequent antigen exposure, IgG is highly expressed undergoing isotype switching. It is noted that the immune simulator predicted higher production of IgM in the secondary and tertiary immune responses compared to the IgG1 production, requiring further validation of the in silico immune simulation results. Total B cell counts increased during the secondary and tertiary immune responses, while the rise in B cells was more remarkable for IsdE and SirA vaccines. T-helper cell population and cytokines levels similarly increased among the five vaccine constructs, except that the immunoregulatory interleukin-2 (IL-2) level was lower in SstD and HtsA. These immune response profiles demonstrate that the clearance of antigen and development of immune responses by the IRLPs vaccines are expected.
Fig. 2
Immune response simulation of IsdE to the vaccine constructs. (A) Antigen count (per mL) and antibody levels (IgM, IgG1, and IgG2), (B) B-cell population (per mm3), (C) T-helper cell population (per mm3), and (D) cytokines and interleukin levels (ng/mL).
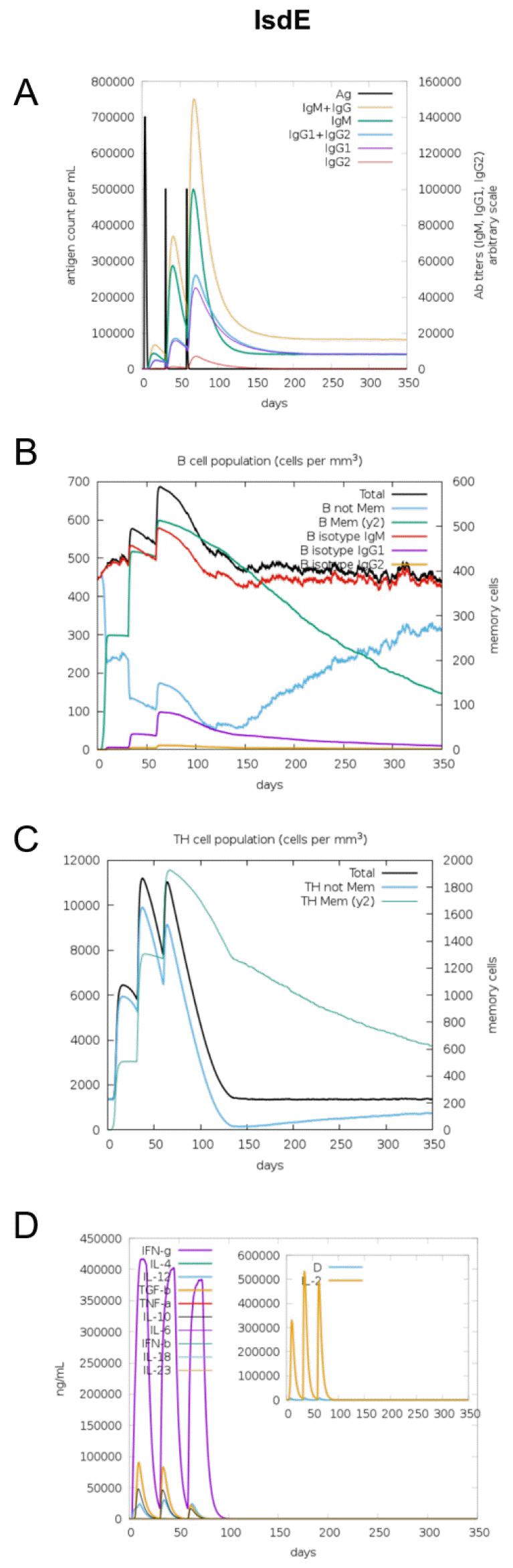
Fig. 3
Immune response simulation of SstD to the vaccine constructs. (A) Antigen count (per mL) and antibody levels (IgM, IgG1, and IgG2), (B) B-cell population (per mm3), (C) T-helper cell population (per mm3), and (D) cytokines and interleukin levels (ng/mL).
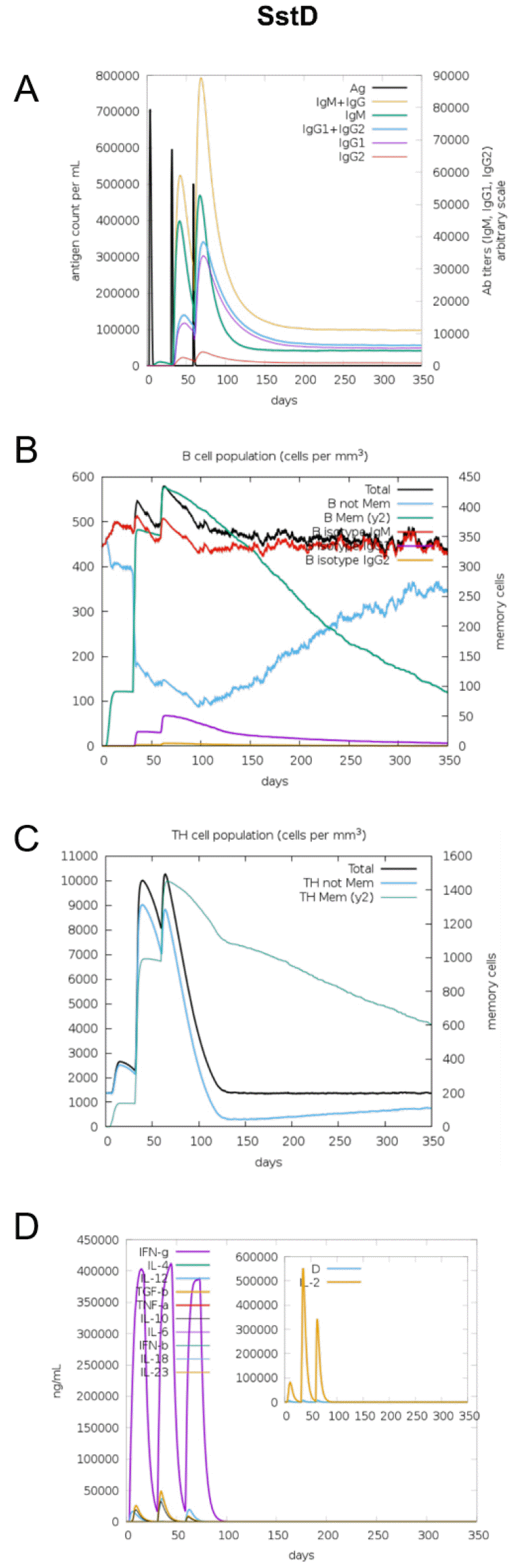
Fig. 4
Immune response simulation of SirA to the vaccine constructs. (A) Antigen count (per mL) and antibody levels (IgM, IgG1, and IgG2), (B) B-cell population (per mm3), (C) T-helper cell population (per mm3), and (D) cytokines and interleukin levels (ng/mL).
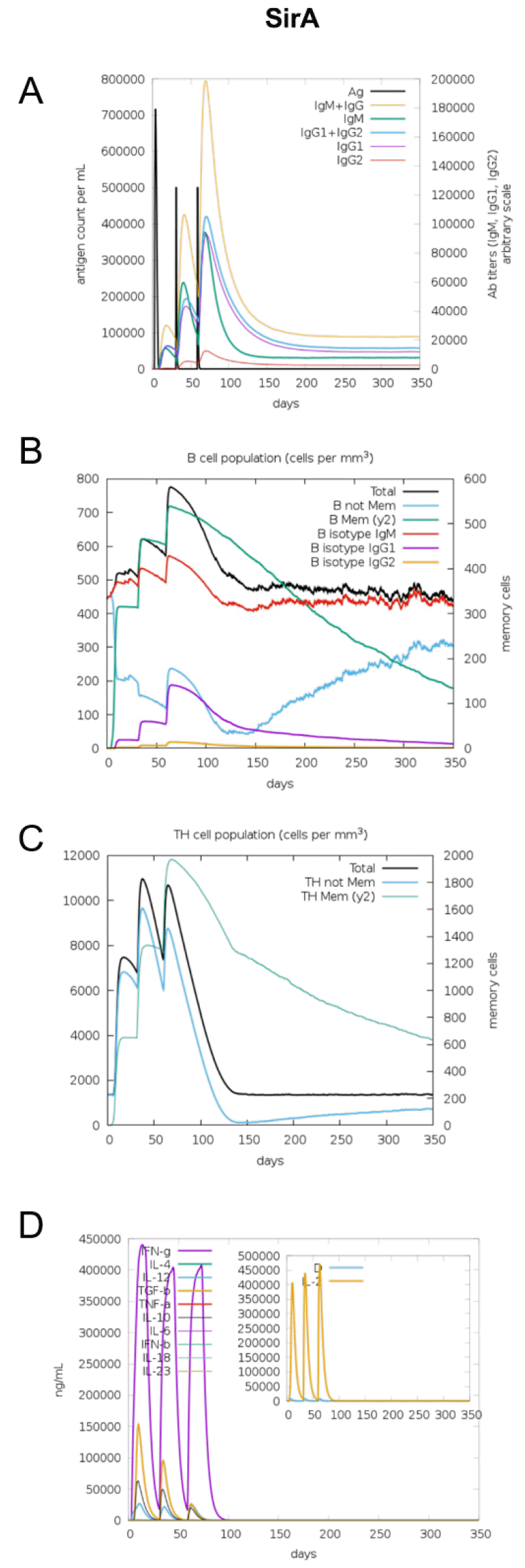
Molecular docking of the multi-epitope vaccine constructs on TLR2
TLR2 associated with TLR1 recognizes microbial lipoproteins and lipopeptides, inducing strong proinflammatory signals in macrophages (41). TLR1-TLR2 as lipopeptide receptors and vaccine peptides as ligands were docked using the PatchDock server. TLR1-TLR2 proteins have characteristic horseshoe-like structures with parallel β-strands and usually the lipopeptide-binding site is located in the concave surfaces of the horseshoe-like structures (38). The best models of each IRLPs vaccine ligands were demonstrated by the PatchDock and FireDock servers with various range of global energy (kCal·Mol-1) by the ascending order: -10.01 for IsdE, -0.52 for SirA, 2.29 for HtsA, 3.54 for SstD, and 13.53 for SitC (Fig. 7). While IsdE vaccine peptide showed the lowest global energy for binding, SitC had the largest positive energy. These results suggest that the IRLPs-originated multi-epitope vaccines would have different binding affinity and possible immune stimulation efficacy via TLR2 except for SitC.
DISCUSSION
Prevention of S. aureus infections are urgently needed especially against the widespread virulent and multi-drug resistant strains. Accordingly, it is important to develop effective and successful vaccines against S. aureus strains. In the present study, we designed five types of multi-epitope vaccine constructs, targeting lipoproteins associated bacterial iron metabolism, namely IsdE, SstD, SirA, HtsA, and SitC. Using immunoinformatic approaches, we evaluated their physicochemical and structural properties. In addition, the immunologic, allergenic, IFN-γ inducing, and toxic features of each construct were predicted, and their effectiveness as vaccine candidates were compared.
Immunoinformatics, also known as computational immunology, is an approach for understanding immunological information and defining new hypotheses related to immune responses (24). Traditional vaccine development against infectious diseases was largely dependent on inactivation or live attenuation of the pathogen. However, these vaccine platforms may cause allergic and toxic responses due to the nature of the vaccine origins (42). To overcome these limitations, recombinant vaccine technologies such as subunit, toxoid, viral vector, and messenger RNA vaccines have been developed based on a rationale design (43). Recently, immunoinformatics-based vaccine development has been widely applied to support the discovery of recombinant vaccine targets encompassing high-throughput bioinformatics approaches to immunology, providing rapid and cost-effective vaccine development (44).
In this study, five multi-epitope vaccine constructs against S. aureus IRLPs were assessed for their physicochemical and immunologic properties. The constructs had similar physicochemical characteristics with respect to molecular weights and isoelectric points. These multi-epitopes were also antigenic, non-allergenic, and non-toxic. On the contrary to the similar features, specific constructs showed distinct immune-stimulatory properties. For example, IsdE vaccine showed increased B cell population after injection and higher predictive binding affinity for the TLR1-TLR2 complex. Similarly, SirA vaccine showed increased B cell production. The vaccine construct against HtsA, high-affinity heme uptake system protein, showed higher positivity for IFN-γ production as well as higher IgM production in secondary and tertiary stages of the immune response. Based on the findings, IsdE or HtsA vaccines would be good choice in terms with the immune stimulation and protective immunity.
Development of S. aureus has been attempted for over a century with a number of candidate antigens. However, the immunizations with the candidate vaccines taken to clinical trials have failed so far (21). One of the well-known target antigen of S. aureus is IsdB, iron-regulated surface determinant protein B (45). Although it was highly immunogenic and effective to the laboratory animals, its use as a vaccine target did not reduce the rate of S. aureus infections and was associated with increased mortality (45). Possible explanations have been recently reported that non-protective antibodies can be imprinted by prior S. aureus exposure and the IsdB vaccine recalls non-protective humoral memory and further its efficacy is reduced by antibody competition (46). Also, the functional redundancy in terms of bacterial iron transport and strain variation in iron-regulated proteins would result in the ineffectiveness of the IsdB vaccine (8).
Iron metabolism is central in bacterial persistence and, therefore, consists of highly complex and functionally redundant protein complexes (47). Most of these complexes contain IRLPs in the recognition and binding of iron. It has been demonstrated that mutation of IRLPs induces expression of a different array of cytokines and weakens the host antimicrobial response (48). In this study, we evaluated potent vaccine targets based on the IRLPs highly associated with various iron acquisition systems. Each of the multi-epitope IRLPs vaccine constructs showed similar physicochemical properties but also distinct simulated immune responses. In order to overcome the functional redundancy and maximize the immunization efficiency, we also can develop a multi-valent vaccine with a combination of the IRLPs.
CONCLUSION
In this study, we designed multi-epitope vaccines targeting S. aureus IRLPs, IsdE, SstD, SirA, HtsA, and SitC, and evaluated their immunological properties using immunoinformatics. Based on the immune simulation studies, it was clear that the designed vaccine constructs would trigger both of B cell and T cell responses and stimulate primary, secondary, and tertiary immune responses. Further in vivo test for efficacy of the vaccine constructs is required for developing new vaccines against pathogenic S. aureus infection.