Abstract
Automated breast ultrasound (ABUS) is a novel imaging method, introduced to overcome the main limitations of traditional hand-held ultrasound, such as the lack of standardization, low reproducibility, small field of view, high operator dependency, and high commitment of physician time. ABUS is a standardized radiologic modality with many advantages in both screening and diagnostic settings. It increases the detection rate of breast cancer, improves workflow, and reduces the examination time. On the other hand, ABUS has some limitations, these include the inability to assess the axilla, vascularization, and elasticity of a lesion. With respect to the interpretation, the disadvantages of ABUS are the artifacts due to poor positioning, lack of contact, or those related to motion or some specific tumors. However, these disadvantages can be diminished by additional attention and training. ABUS can be used in clinical settings and is a promising modality in breast imaging. The purpose of this review is to present a summary of the characteristics and clinical applications of ABUS, and provide future perspectives.
유방암은 전 세계에서 가장 많이 발생하는 암으로(11.7%, 2,261,419명) 치료 기술의 발전에도 불구하고 사망률은 감소하지 않아 전체 암 사망률 5위(6.9% , 6,84,996명)를 차지하고 있다.(1) 유방암을 조기에 발견하는 것은사망률을 줄일 뿐만 아니라 항암화학요법 등의 보조 요법(adjuvant therapy)을 생략할 수 있기 때문에 유방암의 조기 발견을 위한 노력은 매우 중요하다.(2)
유방촬영술(mammography)은 유방암의 선별 검사(screening test)로써 조기 발견을 통해 유일하게 사망률을 낮출 수 있는 영상학적 검사로 알려져 있다.(3) 그러나 치밀 유방(dense breast) 환자에서 낮은 민감도(sensitivity)와 높은 위양성율(false positive rate)의 단점이 있을 수 있다. 이러한 단점으로 필요하지 않은 조직검사를 할 수 있고 환자의 불안을 높이며 방사선 노출 증가 및 검사 시 심한 통증으로 인해 유방촬영술의 시행을 꺼려할 수 있다.(4,5) 유방촬영술의 단점을 보완하기 위해 유방 초음파(ultrasound)를 사용한 연구 결과를 살펴보면 유방암을 포함한 유방 종양의 발견을 유의하게 증가시켰다.(6,7) 그러나 전통적인 수동 유방 초음파(hand-held ultrasound) 또한 표준화(standardization)의 부재, 낮은 재현성(reproducibility), 좁은 시야(field of view), 높은 검사자(operator) 의존도, 긴 검사 시간 등의 단점이 존재해 이를 극복하기 위한 새로운 초음파 장치의 개발이 요구되었다.(8)
자동 유방 초음파(automated breast ultrasound)는 이질적(heterogenous)이고 치밀한 유방을 가진 여성에서 유방촬영술에 추가적인 선별 검사로서 2012년 미국 식약청(U.S. Food and Drug Administration; FDA)의 허가를 받은 새로운 영상 기법이다.(9) 자동 유방 초음파는 방사선사(radiographer)가 촬영한 후 영상의학과 전문의가 별도의 다른 시간(separate time)에 판독하기 때문에 전문의가 소비하는 시간이 적고 검사자 의존도가 낮으며, 관상면(coronal plane) 영상을 얻을 수 있어 유방 병변의 보다 정확한 진단에 도움이 된다. 또한 넓은 시야의 표준화되고 일관성 있는 영상을 얻을 수 있는 장점이 있어 수동 유방 초음파의 단점을 보완할 수 있는 새로운 기술로 각광받고 있다.(10,11)
이에 저자들은 유방암 선별 검사 및 임상 진료에 현재 자동 유방 초음파가 얼마나 유용하게 이용되는지 살펴보고 미래의 자동 유방 초음파 적용 가능성을 전망하여 유방암 환자의 적절하고 효율적인 진료에 도움이 되고자 한다.
자동 유방 초음파는 압박판으로 유방을 고정한 후 넓은 탐촉자가 압박판 위를 가로지르며 자동으로 영상을 획득하는 컴퓨터-기반 시스템(computer-based system)이다. 주파수 6-14 MHz의 선형 탐촉자(linear probe)로 양측 유방의 횡단면(transverse plane), 종단면(longitudinal plane), 관상면(coronal plane)의 세 영상을 얻어 작업단말기(workstation)로 전송한 후 전체 유방을 볼 수 있도록 3차원 영상으로 변환되어 다면재구성(multiplanar reconstruction) 방식으로 보여진다(Fig. 1). 일반적으로 누운(supine) 자세에서 시행하는데 최근에는 엎드린(prone) 자세로 검사하는 장치도 개발되었다.(12)
스캔의 깊이(depth of scan)는 주변부를 포함한 유방 전체를 모두 확인하기 위해 유방의 크기에 따라 3.5-5.0 cm까지 다양하게 적용하며 큰 유방을 가진 환자의 경우 위와 아래면(superior and inferior plane)을 추가로 촬영한다. 평균적으로 한쪽 유방을 검사하는데 3-5분, 한 명의 환자를 검사하는데 10-15분의 시간이 소요된다.(13)
유방암의 선별 검사로써 시행하는 유방촬영술의 단점을 극복하기 위해 자동 유방 초음파를 적용하는 연구는 1980년대부터 이루어졌다.(14) 이후 특히 치밀 유방에서 유방암 선별 검사로서 자동 유방 초음파의 유용성은 많은 연구에서 보고되었다. 1,000명 이상의 많은 수를 대상으로 시행한 기존의 연구 결과들을 요약해 보면 유방촬영술에 추가적으로 자동 유방 초음파를 적용하였을 때 유방촬영술만 시행한 경우보다 높은 민감도(sensitivity)와 동등한 특이도(specificity)를 보였다(Table 1).(10,15-17)
치밀 유방을 가진 4,419명의 여성을 대상으로 다기관에서 시행한 Kelly 등(15)의 보고에 따르면 유방촬영술에 자동 유방 초음파를 추가로 적용하는 경우 민감도와 특이도에서 각각 41%와 3.5%의 이점을 보였으며 자동 유방 초음파 판독 시간도 평균 7분 58초로 매우 짧았다. Brem 등(10)이 발표한 SomoInsight study는 선별 검사로서 자동 유방 초음파의 유용성을 평가한 가장 대규모의 연구이다. 치밀 유방을 가진 무증상의 15,318명의 여성을 대상으로 분석하였을 때 유방촬영술에 자동 유방 초음파를 추가로 적용하는 경우 재검률(recall rate)의 증가를 보였지만(1,000명 당 284.9 vs. 150.2) 26.8%의 민감도 증가와 1,000명 당 발견율 1.9의 증가 소견을 보였다. 이러한 결과들은 자동 유방 초음파에 대한 영상의학과 전문의의 경험이 쌓이고 확신을 통해 불필요한 재검률을 낮춘다면 더욱 증진될 것으로 예상한다.
1,376명의 환자에서 1,527 병변을 대상으로 최근에 발표된 메타 분석(meta-analysis)에 따르면 자동 유방 초음파는 유방암의 선별 검사로써 수동 유방 초음파와 비교해 진단 정확도와 발견율에서 차이를 보이지 않았으며 특히 젊고, 치밀 유방을 가진 환자에게 유용한 것으로 보고하였다.(18)
Wang 등(19)의 보고에 따르면 수동 유방 초음파에 비해 자동 유방 초음파가 낮은 특이도를 보였지만(82.5% vs. 80.5%) 민감도는 4.7% 더 좋은 결과를 보였고(90.6% vs. 95.3%), 5,566명의 대규모 환자를 대상으로 한 Choi 등(21)의 보고에 따르면 유방암의 진단 정확도(97.7% vs. 96.5%)와 특이도(97.8% vs. 96.7%)에서 모두 자동 유방 초음파가 수동 유방 초음파에 비해 우월한 결과를 보였고, 재검률도 자동 유방 초음파 (2.57/1,000예)가 수동 유방 초음파(3.57/1,000예)에 비해 통계적으로 낮은 결과를 보였다. 398명의 여성 환자에서 599개의 유방 병변을 대상으로 연구한 최근 발표에 따르면 자동 유방 초음파가 수동 유방 초음파 에 비해 통계학적으로 의미 있게 좋은 민감도를 보였지만(92.2% vs. 82.5%) 진단 정확도, 특이도, 양성과 음성 예측률에서는 차이를 보이지 않았다.(24)
Breast Imaging Reporting and Data System (BI-RADS) 범주(category)에 따른 유방 병변의 진단 정확도도 두 검사 방법간에 동등한 결과를 보였다. Kim 등(25)의 연구에서 두 검사의 BI-RADS 범주 일치율은 0.773 (k= 0.773 ± 0.104)이었고 ‘orientation (wider-than-tall, taller-than-wide, same)’ 항목에서 가장 높은 일치율을 보였으며(k= 0.608 ± 0.210), ‘posterior echo feature’ 항목에서 가장 낮은 일치율을 보였다(k = 0.371 ± 0.225). 최근에 발표된 Zhang 등(26)의 연구에 따르면 자동 유방 초음파가 수동 유방 초음파에 비해 특히 BI-RADS 범주 4, 5에서 더 좋은 진단 정확도를 보였고(78.6% vs. 71.4%), BI-RADS 범주 1, 2에서 두 검사 방법간의 위음성율(false-negative rate)은 통계학적으로 차이를 보이지 않았다.
유방의 악성 석회화는 크기가 작은 미세석회화가 많아 초음파에서 후방음향 감소(posterior acoustic shadow)를 관찰하기 어려워 유방촬영술에 비해 큰 제한점으로 알려져 왔다. 그러나 자동 유방 초음파는 수동 유방 초음파에 비해 다양한 각도의 영상을 선택적으로 볼 수 있어 석회와의 위치와 수 그리고 모양을 좀 더 정확하게 관찰함으로써 유방의 석회화 병변을 진단하는데 매우 유용한 것으로 알려져 있다.(27)
최근에 발표된 유방 병변에 대한 자동 유방 초음파와 수동 유방 초음파의 유용성을 분석한 체계적 고찰(systemic review)을 살펴보면 자동 유방 초음파는 수동 유방 초음파와 비교해 유방의 양성과 악성 병변을 감별하는데 정확도 면에서 차이를 보이지 않았다.(28)
유방암 병기 결정을 위해 가장 신뢰할 만한 영상 검사는 유방 자기공명영상(magnetic resonance imaging; MRI)이다. 그러나 유방 MRI는 특이도가 낮은 단점이 있는데 자동 유방 초음파는 이러한 MRI의 낮은 특이도를 보완하는데 매우 유용하다. Schmachtenberg 등(29)은 유방 병변의 진단에 있어 자동 유방 초음파가 MRI와 훌륭한 상관 관계(k = 0.83)를 보인다고 하였고 Girometti 등(30)은 MRI를 시행한 환자 중 유방암의 고위험군, BI-RADS 범주 3, 선행항암화학요법 후 반응 평가를 받은 131명의 환자를 대상으로 이차 추적(second-look) 초음파로서 자동 유방 초음파가 수동 유방 초음파 와 유사한 발견율(69.3% vs. 71.5%)과 거의 완벽한 일치율(k = 0.94)을 보인다고 하였다.
수술 전 유방 MRI를 시행한 58명의 환자에서 총 80개의 추가 병변이 확인되었고 추가 병변에 대한 이차 추적 초음파 검사에서 자동 유방 초음파는 70개, 수동 유방 초음파는 65개의 병변을 확인함으로써 MRI와의 일치율이 자동 유방 초음파가 더 높았으며 자동 유방 초음파에서 발견된 병변의 10%는 수동 유방 초음파에서 보이지 않았다.(31) 또한 Kim 등(32)의 전향적 연구에서도 MRI후 시행한 이차 추적 초음파에서 자동 유방 초음파가 수동 유방 초음파보다 통계학적으로 의미 있게 더 높은 발견율(94.7% vs. 86.8%; P < 0.05)을 보였다.
자동 유방 초음파의 가장 큰 장점은 수술대에서 보여지는 유방의 관상면 영상을 얻을 수 있다는 점이다. 관상면 영상을 통해 병변의 변연부(edge) 평가를 증진시킬 수 있으며 유방암을 감별하는데 중요한 ‘뒷당김 현상(retraction phenomenon)’을 좀 더 명확히 관찰할 수 있다.(19) 뒷당김 현상은 병변 주위 조직의 수렴 경향(convergence tendency)으로 정의할 수 있는데 Van Zelst 등(33)은 관상면에서의 뒷당김 현상과 유방암 사이에 상관 관계가 있음을 보고하였고, 뒷당김 현상은 특히 작은 크기의 종양, 낮은 조직학적 등급, 여성 호르몬 수용체의 존재와 통계학적으로 의미 있는 관련이 있었다. 또한 관상면을 통해 피부부터 흉벽까지 해부학적 구조를 연속적이고 체계적으로 볼 수 있기 때문에 유방 수술 시 효과적인 정보를 제공해 준다.
정기적인 추적관찰을 시행하는 유방 병변을 가진 환자에서 자동 유방 초음파는 병변의 위치, 크기, 특징을 비교할 수 있는 재현 가능한 영상을 제공한다. 영상의학과 전문의가 평균 20분에 걸쳐 시행하는 수동 유방 초음파에 비해 방사선사가 평균 10분 정도에 걸쳐 촬영하는 자동 유방 초음파는 시간 절약 차원에서 월등한 이점을 보인다.(34)
유방의 변연부(peripheral)에 위치한 병변의 경우 자동 유방 초음파로 발견하지 못할 가능성이 있고 자동 유방 초음파의 촬영 특성 상 겨드랑이는 촬영 범위에 포함할 수 없기 때문에 겨드랑이 림프절 전이 여부에 대한 정보를 얻을 수 없어 유방암 환자의 수술 전 검사로 제한점이 있다. 최근 자동 유방 초음파의 영상 특징과 Ki-67을 이용하여 겨드랑이 림프절 전이 상태를 예측하는 모델에 관한 연구도 발표되었지만 겨드랑이 부위를 검사할 수 있는 방법의 개발이 필요한 상황이다.(35) 자동 유방 초음파는 병변의 혈관 분포(vascularization)와 탄성도(elasticity)를 평가할 수 없다는 단점이 있으며 자동 유방 초음파 유도 하에 조직 검사 등의 침습적 시술을 시행할 수 없어 자동 유방 초음파에서 의심스러운 병변이 관찰된 경우 수동 유방 초음파로 재평가 후 시술을 시행해야 한다.(34)
압박이 적절하게 이루어지지 않을 때 허상(artifact)이 보일 수 있는데 이는 공기, 젤(gel), 유두 또는 탐촉자의 부적절한 이동이나 환자의 빠르고 깊은 호흡에 의해서도 나타날 수 있다.(36,37) 자동 유방 초음파 영상이 단면적(sectional)이고 정적(static)이기 때문에 복합 낭성 병변(complex cystic lesion)의 경우에 위양성(false positive)결과를 보일 수 있다. 반대로 작은 크기의 병변, 엽상 종양(phyllodes tumor) 또는 수질암(medullary carcinoma) 등에서 보이는 국한된 테두리(circumscribed edge)는 위음성 결과를 낳을 수 있다.(19)
이러한 자동 유방 초음파의 단점들은 방사선사의 축적된 경험, 정확한 탐촉자와 피부 접촉, 충분한 젤의 사용, 변연부 지역을 포함한 전 유방 스캔 등의 노력을 통해 충분히 극복될 수 있다. 또한 전문의들은 환자들에게 병력 청취를 자세히 하고 임상 자료 특히 이전에 시행한 영상 검사 등을 잘 확보해 정확한 진단을 내리기 위해 힘써야 한다.
자동 유방 초음파의 실제 임상 적용을 위해 높은 판독자간 신뢰도(inter-observer reliability)는 필수적이다. 많은 연구들에서 자동 유방 초음파의 판독자간 신뢰도가 높음을 보고하였으나 병변의 모양과 경계 측면에서 판독자간 신뢰도가 낮다는 보고도 있었다.(38) 최근에 판독자간의 신뢰도와 관련된 체계적 고찰을 시행한 Meng 등(39)에 따르면 자동 유방 초음파는 양성과 악성 유방 병변을 감별하는 민감도(92.0%)와 특이도(84.9%)는 높지만 판독자간의 신뢰도는 각 검사마다 매우 이질적이라고 보고해 자동 유방 초음파의 판독자간의 신뢰도에 관해서는 잘 고안된 전향적 연구가 필요할 것으로 생각한다.(40)
최근 연구 결과에 따르면 자동 유방 초음파의 영상학적 특성과 유방암의 분자적 아형(molecular subtype)이 상관 관계가 있다는 보고가 있다. 303개의 유방암 병변을 대상으로 한 연구에서 Zheng 등(41)은 특히 뒷당김 현상과 유방암 아형 사이에 긴밀한 연관이 있음을 보고하였고, Jiang 등(42)도 유사한 결과를 보고해 이에 관련된 좀 더 많은 연구 결과가 축적된다면 향후 유방암에 대한 자동 유방 초음파의 진단적 가치는 더욱 향상될 것으로 생각한다.
또한 선행항암화학요법 후 반응 평가에 있어서도 자동 유방 초음파가 유용하다는 보고가 있었는데 선행항암화학요법 후 병리학적 완전 관해(complete pathological response)를 예측하는데 85.7%–88.1%의 민감도와 81.5%-85.1%의 특이도를 보였다.(43)
자동 유방 초음파를 위해 최근에 개발된 computer-aided detection software(QVCADTM, QView Medical, Los Altos, CA, USA)가 FDA 승인을 받았는데 이를 이용한 후향적 연구에 따르면 18명의 영상의학과 전문의 중 33%가 진단의 정확도에 영향을 미치지 않고 판독 시간의 감소를 보였다. 또한 QVCADTM을 실제 임상에 적용해 보았을 때 1분 이상의 평균 판독 시간 감소를 보였다.(3.33 min vs. 2.24 min) (44) 향후 자동 유방 초음파와 라디오믹스(radiomics), 딥 러닝(deep learning) 그리고 인공 지능(artificial intelligence)과의 통합(integration)이 이루어진다면 더욱 정확한 유방 병변의 진단 방법이 될 것으로 예상한다.(45)
REFERENCES
1. International Agency for Research on Cancer. 2022. Cancer today: data visualization tools for exploring the global cancer burden in 2020 [Internet]. International Agency for Research on Cancer;Lyon: Available from: http://gco.iarc.fr/today/home. cited 2022 September 19.
2. Chow LW, Yip AY, Ng EL. 2012; Prevention of oncological diseases: primary and secondary prevention. Int J Biol Markers. 27:e337–43. DOI: 10.5301/JBM.2012.10370. PMID: 23250774.


3. Drukteinis JS, Mooney BP, Flowers CI, Gatenby RA. 2013; Beyond mammography: new frontiers in breast cancer screening. Am J Med. 126:472–9. DOI: 10.1016/j.amjmed.2012.11.025. PMID: 23561631. PMCID: PMC4010151.


4. Kolb TM, Lichy J, Newhouse JH. 2002; Comparison of the performance of screening mammography, physical examination, and breastUS and evaluation of factors that influence them: an analysis of 27,825 patient evaluations. Radiology. 225:165–75. DOI: 10.1148/radiol.2251011667. PMID: 12355001.


5. Feig SA. 2004; Adverse effects of screening mammography. Radiol Clin North Am. 42:807–19. DOI: 10.1016/j.rcl.2004.06.013. PMID: 15337417.


6. Burkett BJ, Hanemann CW. 2016; A review of supplemental screening ultrasound for breast cancer: certain populations of women with dense breast tissue may benefit. Acad Radiol. 23:1604–9. DOI: 10.1016/j.acra.2016.05.017. PMID: 27374700.
7. Berg WA, Blume JD, Cormack JB, Mendelson EB, Lehrer D, Böhm-Vélez M, et al. 2008; Combined screening with ultrasound and mammography vs mammography alone in women at elevated risk of breast cancer. JAMA. 299:2151–63. DOI: 10.1001/jama.299.18.2151. PMID: 18477782. PMCID: PMC2718688.


8. Zanotel M, Bednarova I, Londero V, Linda A, Lorenzon M, Girometti R, et al. 2018; Automated breast ultrasound: basic principles and emerging clinical applications. Radiol Med. 123:1–12. DOI: 10.1007/s11547-017-0805-z. PMID: 28849324.


9. Giger ML, Inciardi MF, Edwards A, Papaioannou J, Drukker K, Jiang Y, et al. 2016; Automated breast ultrasound in breast cancer screening of women with dense breasts: reader study of mammography-negative and mammography-positive cancers. AJR Am J Roentgenol. 206:1341–50. DOI: 10.2214/AJR.15.15367. PMID: 27043979.


10. Brem RF, Tabár L, Duffy SW, Inciardi MF, Guingrich JA, Hashimoto BE, et al. 2015; Assessing improvement in detection of breast cancer with three-dimensional automated breast US in women with dense breast tissue: the SomoInsight Study. Radiology. 274:663–73. DOI: 10.1148/radiol.14132832. PMID: 25329763.


11. Chang JM, Moon WK, Cho N, Park JS, Kim SJ. 2011; Radiologists' performance in the detection of benign and malignant masses with 3D automated breast ultrasound (ABUS). Eur J Radiol. 78:99–103. DOI: 10.1016/j.ejrad.2011.01.074. PMID: 21330080.


12. Kaplan SS. 2014; Automated whole breast ultrasound. Radiol Clin North Am. 52:539–46. DOI: 10.1016/j.rcl.2014.01.002. PMID: 24792655.


13. Nicosia L, Ferrari F, Bozzini AC, Latronico A, Trentin C, Meneghetti L, et al. 2020; Automatic breast ultrasound: state of the art and future perspectives. Ecancermedicalscience. 14:1062. DOI: 10.3332/ecancer.2020.1062. PMID: 32728378. PMCID: PMC7373644.


14. Maturo VG, Zusmer NR, Gilson AJ, Smoak WM, Janowitz WR, Bear BE, et al. 1980; Ultrasound of the whole breast utilizing a dedicated automated breast scanner. Radiology. 137:457–63. DOI: 10.1148/radiology.137.2.6254110. PMID: 6254110.


15. Kelly KM, Dean J, Lee SJ, Comulada WS. 2010; Breast cancer detection: radiologists' performance using mammography with and without automated whole-breast ultrasound. Eur Radiol. 20:2557–64. DOI: 10.1007/s00330-010-1844-1. PMID: 20632009. PMCID: PMC2948156.


16. Giuliano V, Giuliano C. 2013; Improved breast cancer detection in asymptomatic women using 3D-automated breast ultrasound in mammographically dense breasts. Clin Imaging. 37:480–6. DOI: 10.1016/j.clinimag.2012.09.018. PMID: 23116728.


17. Wilczek B, Wilczek HE, Rasouliyan L, Leifland K. 2016; Adding 3D automated breast ultrasound to mammography screening in women with heterogeneously and extremely dense breasts: report from a hospital-based, high-volume, single-center breast cancer screening program. Eur J Radiol. 85:1554–63. DOI: 10.1016/j.ejrad.2016.06.004. PMID: 27501888.


18. Zhang X, Chen J, Zhou Y, Mao F, Lin Y, Shen S, et al. 2019; Diagnostic value of an automated breast volume scanner compared with a hand-held ultrasound: a meta-analysis. Gland Surg. 8:698–711. DOI: 10.21037/gs.2019.11.18. PMID: 32042678. PMCID: PMC6989916.


19. Wang HY, Jiang YX, Zhu QL, Zhang J, Dai Q, Liu H, et al. 2012; Differentiation of benign and malignant breast lesions: a comparison between automatically generated breast volume scans and handheld ultrasound examinations. Eur J Radiol. 81:3190–200. DOI: 10.1016/j.ejrad.2012.01.034. PMID: 22386134.


20. Chen L, Chen Y, Diao XH, Fang L, Pang Y, Cheng AQ, et al. 2013; Comparative study of automated breast 3-D ultrasound and handheld B-mode ultrasound for differentiation of benign and malignant breast masses. Ultrasound MedBiol. 39:1735–42. DOI: 10.1016/j.ultrasmedbio.2013.04.003. PMID: 23849390.


21. Choi WJ, Cha JH, Kim HH, Shin HJ, Kim H, Chae EY, et al. 2014; Comparison of automated breast volume scanning and hand- held ultrasound in the detection of breast cancer: an analysis of 5,566 patient evaluations. Asian Pac J Cancer Prev. 15:9101–5. DOI: 10.7314/APJCP.2014.15.21.9101. PMID: 25422185.
22. Jeh SK, Kim SH, Choi JJ, Jung SS, Choe BJ, Park S, et al. 2016; Comparison of automated breast ultrasonography to handheld ultrasonography in detecting and diagnosing breast lesions. Acta Radiol. 57:162–9. DOI: 10.1177/0284185115574872. PMID: 25766727.


23. Hellgren R, Dickman P, Leifland K, Saracco A, Hall P, Celebioglu F. 2017; Comparison of handheld ultrasound and automated breast ultrasound in women recalled after mammography screening. Acta Radiol. 58:515–20. DOI: 10.1177/0284185116665421. PMID: 27565633.


24. Niu L, Bao L, Zhu L, Tan Y, Xu X, Shan Y, et al. 2019; Diagnostic performance of automated breast ultrasound in differentiating benign and malignant breast masses in asymptomatic women: a comparison study with handheld ultrasound. J Ultrasound Med. 38:2871–80. DOI: 10.1002/jum.14991. PMID: 30912178.


25. Kim H, Cha JH, Oh HY, Kim HH, Shin HJ, Chae EY. 2014; Comparison of conventional and automated breast volume ultrasound in the description and characterization of solid breast masses based on BI-RADS features. Breast Cancer. 21:423–8. DOI: 10.1007/s12282-012-0419-1. PMID: 23086698.


26. Zhang X, Lin X, Tan Y, Zhu Y, Wang H, Feng R, et al. 2018; A multicenter hospital-based diagnosis study of automated breast ultrasound system in detecting breast cancer among Chinese women. Chin J Cancer Res. 30:231–9. DOI: 10.21147/j.issn.1000-9604.2018.02.06. PMID: 29861608. PMCID: PMC5953959.


27. Xiao YM, Chen ZH, Zhou QC, Wang Z. 2015; The efficacy of automated breast volume scanning over conventional ultrasonography among patients with breast lesions. Int J Gynaecol Obstet. 131:293–6. DOI: 10.1016/j.ijgo.2015.05.036. PMID: 26493011.


28. Ibraheem SA, Mahmud R, Mohamad Saini S, Abu Hassan H, Keiteb AS, Dirie AM. 2022; Evaluation of diagnostic performance of automatic breast volume scanner compared to handheld ultrasound on different breast lesions: a systematic review. Diagnostics (Basel). 12:541. DOI: 10.3390/diagnostics12020541. PMID: 35204629. PMCID: PMC8870745.


29. Schmachtenberg C, Fischer T, Hamm B, Bick U. 2017; Diagnostic performance of automated breast volume scanning (ABVS) compared to handheld ultrasonography with breast MRI as the gold standard. Acad Radiol. 24:954–61. DOI: 10.1016/j.acra.2017.01.021. PMID: 28336007.


30. Girometti R, Zanotel M, Londero V, Bazzocchi M, Zuiani C. 2017; Comparison between automatedbreast volume scanner (ABVS) versus hand-held ultrasound as a second look procedure after magnetic resonance imaging. Eur Radiol. 27:3767–75. DOI: 10.1007/s00330-017-4749-4. PMID: 28120030.


31. Chae EY, Shin HJ, Kim HJ, Yoo H, Baek S, Cha JH, et al. 2013; Diagnostic performance of automated breast ultrasound as a replacement for a hand-held second-look ultrasound for breast lesions detected initially on magnetic resonance imaging. Ultrasound MedBiol. 39:2246–54. DOI: 10.1016/j.ultrasmedbio.2013.07.005. PMID: 24035627.


32. Kim Y, Kang BJ, Kim SH, Lee EJ. 2016; Prospective study comparing two second-look ultrasound techniques: handheld ultrasound and an automated breast volume scanner. J Ultrasound Med. 2103–12. DOI: 10.7863/ultra.15.11076. PMID: 27503758.
33. Van Zelst JC, Platel B, Karssemeijer N, Mann RM. 2015; Multiplanar reconstructions of 3D automated breast ultrasound improve lesion differentiation by radiologists. Acad Radiol. 22:1489–96. DOI: 10.1016/j.acra.2015.08.006. PMID: 26345538.


34. Boca Bene I, Ciurea AI, Ciortea CA, Dudea SM. 2021; Pros and cons for Automated Breast Ultrasound (ABUS): a narrative review. J Pers Med. 703. DOI: 10.3390/jpm11080703. PMID: 34442347. PMCID: PMC8400952.
35. Wang Q, Li B, Liu Z, Shang H, Jing H, Shao H, et al. 2022; Prediction model of axillary lymph node status using automated breast ultrasound (ABUS) and ki-67 status in early-stage breast cancer. BMC Cancer. 22:929. DOI: 10.1186/s12885-022-10034-3. PMID: 36031602. PMCID: PMC9420256.


36. Xiao Y, Zhou Q, Chen Z. 2015; Automated breast volume scanning versus conventional ultrasound in breast cancer screening. Acad Radiol. 22:387–99. DOI: 10.1016/j.acra.2014.08.013. PMID: 25620036.


37. Isobe S, Tozaki M, Yamaguchi M, Ogawa Y, Homma K, Satomi R, et al. 2011; Detectability of breast lesions under the nipple using an automated breast volume scanner: comparison with handheld ultrasonography. Jpn J Radiol. 29:361–5. DOI: 10.1007/s11604-010-0555-5. PMID: 21717306.


38. Kim SH, Kang BJ, Choi BG, Choi JJ, Lee JH, Song BJ, et al. 2013; Radiologists' performance for detecting lesions and the interobserver variability of automated whole breast ultrasound. Korean J Radiol. 14:154–63. DOI: 10.3348/kjr.2013.14.2.154. PMID: 23482698. PMCID: PMC3590325.


39. Meng Z, Chen C, Zhu Y, Zhang S, Wei C, Hu B, et al. 2015; Diagnostic performance of the automated breast volume scanner: a systematic review of inter-rater reliability/agreement and meta-analysis of diagnostic accuracy for differentiating benign and malignant breast lesions. Eur Radiol. 25:3638–47. DOI: 10.1007/s00330-015-3759-3. PMID: 25916389.


40. Kang SY, Lee SJ, Youn HJ, Jung SH. 2017; Clinical significance of automated breast ultrasound. J Surg Ultrasound. 4:12–7.
41. Zheng FY, Lu Q, Huang BJ, Xia HS, Yan LX, Wang X, et al. 2017; Imaging features of automated breast volume scanner: correlation with molecular subtypes of breast cancer. Eur J Radiol. 86:267–75. DOI: 10.1016/j.ejrad.2016.11.032. PMID: 28027759.


42. Jiang J, Chen YQ, Xu YZ, Chen ML, Zhu YK, Guan WB, et al. 2014; Correlation between three-dimensional ultrasound features and pathological prognostic factors in breast cancer. Eur Radiol. 24:1186–96. DOI: 10.1007/s00330-014-3135-8. PMID: 24723231.


43. Wang X, Huo L, He Y, Fan Z, Wang T, Xie Y, et al. 2016; Early prediction of pathological outcomes to neoadjuvant chemotherapy in breast cancer patients using automated breast ultrasound. Chin J Cancer Res. 28:478–85. DOI: 10.21147/j.issn.1000-9604.2016.05.02. PMID: 27877006. PMCID: PMC5101221.


44. Jiang Y, Inciardi MF, Edwards AV, Papaioannou J. 2018; Interpretation time using a concurrent-read computer-aided detection system for automated breast ultrasound in breast cancer screening of women with dense breast tissue. AJR Am J Roentgenol. 211:452–61. DOI: 10.2214/AJR.18.19516. PMID: 29792747.


45. Liao WX, He P, Hao J, Wang XY, Yang RL, An D, et al. 2020; Automatic identification of breast ultrasound image based on supervised block-based region segmentation algorithm and features combination migration deep learning model. IEEE J Biomed Health Inform. 24:984–93. DOI: 10.1109/JBHI.2019.2960821. PMID: 31869809.


Fig. 1
Automated breast ultrasoundimage of a 40-year-old woman with 0.5 cm sized benign mass. (A) Coronal view (B) Longitudinal view (C) Transverse view The lesion is marked as a dotted circle.
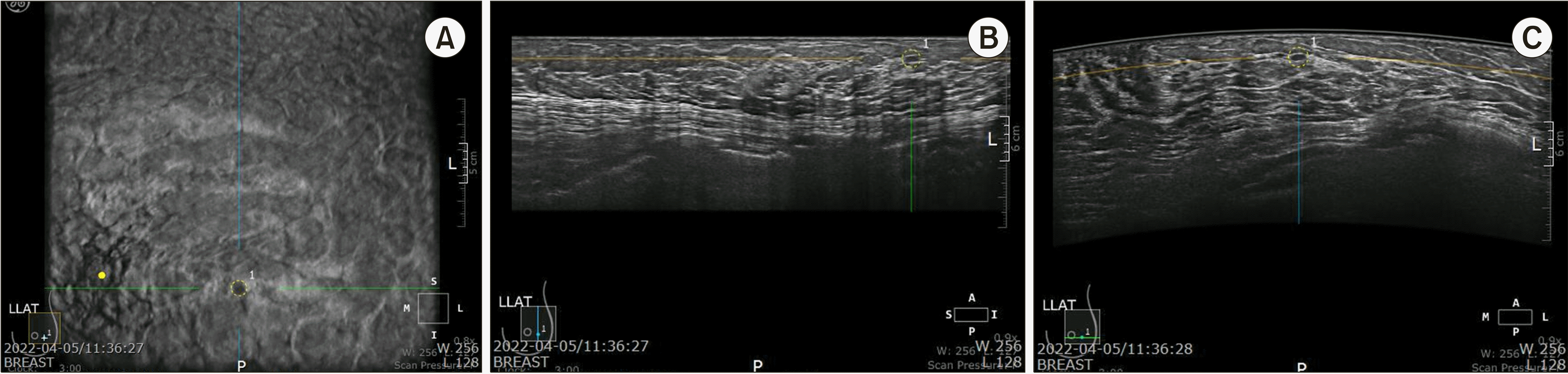
Table 1
Diagnostic Value of Automated Breast Ultrasound in Screening Program
Author |
Year of publication |
Number of patients |
Sensitivity (%) | Specificity (%) | Detection rate per 1,000 | |||
---|---|---|---|---|---|---|---|---|
|
|
|
||||||
MMG | MMG + ABUS | MMG | MMG + ABUS | MMG | MMG + ABUS | |||
Kelly et al. (15) | 2010 | 4,419 | 40 | 81 | 95.2 | 98.7 | 2.6 | 6.5 |
Giuliano and Giuliano (16) | 2012 | 3,418 | 76 | 96.7 | 99.7 | 98.2 | 4.6 | 12.3 |
Brem et al. (10) | 2014 | 15,318 | 73.2 | 100 | 85.4 | 72 | 5.4 | 7.3 |
Wilczek et al. (17) | 2016 | 1,668 | 63.6 | 100 | 99 | 98.4 | 4.2 | 6.6 |
Table 2
Diagnostic Accuracy of Automated Breast Ultrasound and Hand-Held Ultrasound Regarding Breast Cancer Detection
Author | Year of publication | Number of patients | Sensitivity (%) | Specificity (%) | |||
---|---|---|---|---|---|---|---|
|
|
|
|||||
ABUS | HHUS | ABUS | HHUS | ||||
Wang et al. (19) | 2012 | 213 | 95.3 | 90.6 | 80.5 | 82.5 | |
Chen et al. (20) | 2013 | 175 | 92.5 | 88.1 | 86.2 | 87.5 | |
Choi et al. (21) | 2014 | 5,566 | 77.8 | 62.5 | 97.8 | 96.7 | |
Jeh et al. (22) | 2016 | 173 | 88 | 95.7 | 76.2 | 49.4 | |
Hellgren et al. (23) | 2017 | 113 | 88 | 88 | 89.2 | 93.5 | |
Niu et al. (24) | 2019 | 398 | 92.2 | 82.5 | 77.6 | 80.2 |