Abstract
Background and Objectives
Considering the prevalence and health effects of obstructive sleep apnea (OSA), early diagnosis and proper treatment are essential. Polysomnography (PSG) has limitations in diagnosing or tracking large-scale OSA patients. Smartwatches (SWs) can be equipped with a photoplethysmograph (PPG) that can indirectly measure heart rate and blood oxygen saturation by detecting the difference of light absorption through blood. The purpose of this study is to compare oxygen saturation parameters of PPG-based SWs with those of PSG to determine the diagnostic accuracy for OSA.
Methods
After obtaining voluntary consent from patients who were scheduled to undergo PSG in a sleep clinic due to suspected OSA, they were randomly assigned to wear a Galaxy watch4 (GW) or Apple watch7 (AW) on their wrist. The agreement rates between the oxygen saturation parameters of the two SW types and PSG were evaluated. The accuracy, sensitivity, and specificity of the oxygen saturation parameters for diagnosis of OSA (apnea-hypopnea index [AHI] ≥5/h) were compared between the two types of SW.
Results
A total of 133 patients underwent PSG while wearing an SW. Including duplicates, 109 patients wearing a GW and 69 wearing an AW were included. The diagnostic accuracy of AHI ≥5/h according to oxygen saturation time measured by a GW was less than 90%, the respective sensitivity and specificity were 82.9% and 75.8%. The area under the curve (AUC) of the receiver operating characteristic (ROC) curve was 0.807 (p<0.001). Using the lowest oxygen saturation value of GW, the sensitivity was 81.6%, the specificity was 69.7%, and the AUC of the ROC was 0.849 (p<0.001). The diagnostic accuracy of AHI ≥5/h according to the average oxygen saturation of AW, and the sensitivity and specificity were 75.6% and 70.8%, respectively. The AUC of this ROC curve was 0.757 (p<0.001). Using the lowest oxygen saturation value of AW, the sensitivity was 71.1%, the specificity was 62.5%, and the AUC of the ROC was 0.705 (p=0.005).
Obstructive sleep apnea (OSA) is a condition in which breathing stops during sleep, which, if left untreated, can significantly reduce the quality of life by causing chronic fatigue, daytime sleepiness, and cognitive function decline [1]. The prevalence of OSA in Korea is 4% of male and 2% of female, but it is known to increase to 30% in age-specific studies [2]. OSA is potentially fatal disease because it can increase the risk of cardiovascular disease, including coronary artery disease, stroke and diabetes [3-5]. Considering the prevalence and health effects of OSA, proper diagnosis and treatment is essential. Currently, polysomnography (PSG) is the gold standard for diagnosing OSA. PSG has limitations in diagnosing or tracking large-scale OSA patients because it requires inhospital facilities and a lot of time and labor for examination and reading. Complementary to PSG, home sleep apnea test (HSAT) is less expensive than PSG, but it also requires a medical evaluation by a doctor [6] and is still limited for many patients due to cost or time issues.
Recently, digital wearable devices such as, Smartwatches (SWs) or Bands that can easily measure various health bio-signals have become common. They are equipped with a 3-axis accelerometer that distinguishes between motion and rest, and photoplethysmography (PPG) that measures peripheral blood volume by detecting the difference of light absorption. The PPG can indirectly estimate oxygen saturation and respiration rate by calculating different light absorption rates according to heart rate or oxygenated hemoglobin levels, and replace the hassle of inserting a needle into a blood vessel to measure oxygen saturation in various medical fields.
Although most digital wearable devices are not Food and Drug Administration approved and made for well-being or fitness purposes, they measure critical vital signs such as, oxygen saturation, pulse rate, and respiration that cross medical and non-medical boundaries, which increase the need to evaluate and validate the accuracy of these devices. If these devices become more accurate, the paradigm may change from doctor-and hospital-centered healthcare to patient-centered smart healthcare. Digital healthcare can provide health care services of prevention, diagnosis, treatment, and follow-up care anytime and anywhere [7].
Various digital wearable devices and apps related to sleep have been developed to analyze sleep patterns and quality. Early wearable devices analyzed sleep duration and structure based on a 3-axis accelerometer and pulse rate variability. However, compared with PSG, which is the gold standard, it was still insufficient to replace PSG due to its low specificity compared to the sensitivity except for total sleep time [8-10]. The recently introduced SWs can serially measure oxygen saturation during sleep using reflective PPG, so it theoretically possible to detect hypoxia, which is often accompanied by sleep-related breathing disorders. Although diagnosis of OSA syndrome (OSAS) is not made by the severity of oxygen desaturation, but by apnea or hypopnea episodes per hour, a few studies have suggested that the oxygen saturation-related index in patient with OSA is significantly associated with the apnea-hypopnea index (AHI), and oxygen-related parameters can be helpful in diagnosing and assessing the severity of OSAS [11-13].
Therefore, the purpose of this study is to compare oxygen saturation parameters of PPG-based SWs with the results of PSG to determine the diagnostic accuracy and how useful it tis to screen for OSA.
A prospective study was conducted with patients aged 19 years older who voluntarily participated in this study among patients who visited an Eulji University Hospital Sleep Clinic from September 1, 2021 to September 30, 2022, and underwent PSG with suspected OSA. Exclusion criteria are as follows. Patients younger than 19 years of age, patients who did not consent to the study, patients with pigmented or tattooed wrists, patients who unable to clearly communicate or respond to pain, and patients who slept less than 240 minutes.
All participants received informed consent in writing, and the study protocol was approved by the Institutional Review Board of Daejeon Eulji University Hospital (IRB no. 2022-03-010-002)
PPG is a non-invasive method for measuring blood volume in the microvascular layer of the skin based on optical properties such as, absorption and reflection of human tissues at specific light wavelengths. Deoxygenated hemoglobin absorbs more red wavelengths, and oxygenated hemoglobin absorbs more infrared wavelengths [14].
The SWs measures the infrared PPG and red PPG signals from the wrist using a reflective pulse oximetry to obtain the perfusion index, which is the ratio of pulsatile and non-pulsatile static blood flow at each wavelength. Using the R value calculated using the ratio of the perfusion index and a pretrained model, the oxygen saturation is estimated.
An Android-based Samsung Galaxy watch4 (GW, 44 mm sized) and an iPhone-based Apple watch7 (AW, 44 mm sized) were used in the study. Participants were randomly assigned to wear GW or AW on both wrists, and a transmissive pulse oximeter was worn on their fingertips to perform a nighttime PSG test at a sleep clinic.
PSG was conducted in a level I environment according to the guidelines of the American Academy of Sleep Medicine (AASM) using Embla N7000 (Natus, Kanata, Canada). Electroencephalogram (F3, F4, C3, C4, O1, O2), electrooculogram, chin and leg electromyogram, airflow signals, respiratory effort signals, electrocardiogram, snoring, and peripheral oxygen saturation were measured. Reading was performed by a sleep medicine specialist according to the AASM Manual for the Scoring of Sleep and Associated Events. An AHI of less than 5 per hour is defined as the normal, 5 or more and less than 15 as mild, 15 or more and less than 30 as moderate, and 30 or more as severe.
Statistical analysis was performed using IBM SPSS Statistics 25 (IBM Corp., Armonk, NY, USA). Bland-Altman plot and intraclass correlation coefficient (ICC) were used to evaluate the agreement between SWs and PSG. To compare the diagnostic performance of the two different SWs for OSA screening, a receiver-operating characteristic (ROC) curve was performed and the cut-off values were obtained. All statistical significance was considered when p<0.05.
A total of 133 patients who underwent PSG for suspected OSA were included in the study. Of the 133 patients, 109 performed PSG with the GW on their wrist, 69 on their wrist AW, and 45 on both wrists. There were 109 patients in the GW group and 69 patients in the AW. The mean age was 45.8 years, and there were 103 males and 30 females. There was no difference between the two groups in terms of sex, age, body mass index, daytime sleepiness questionnaire score, and presence of underlying diseases (Table 1).
The average total sleep time of GW and AW was 347.9 minutes and 338.4 minutes, and the average sleep efficiency was 84.8% and 84.4% respectively. The AHI was 21.5/h in GW and 18.0/h in AW, showing no significant difference (p=0.246). In GW, the ratio of mild/moderate/severe OSA based on AHI was 19.3%/20.2%/30.3%, and in AW, 24.6%/ 17.4%/23.2%, there was no significant difference between two groups (p= 0.627). In the oxygen saturation parameters, the average oxygen saturation was 93.9% in GW and 93.7% in AW, indicating no significant difference (p=0.585). At the lowest oxygen saturation, GW was by 81.9% and AW was 81.6%, which showed no significant difference (p=0.796) (Table 1).
Using the Bland-Altman plot, the oxygen saturation measured in PSG and SWs were compared.
For the time of oxygen saturation less than 90%, the agreement increased when the time of less than 90% was short in case of normal or mild OSA severity, whereas the agreement decreased as the time of less than 90% was high in case of severe OSA (Fig. 1A), and the ICC of this plot was 0.441, indicating a fair degree (Table 2). The lowest oxygen saturation of GW showed a difference of -2.5% on average from the PSG result, and the ICC value was 0.909, which showed a very high agreement with the PSG (Fig. 1B and Table 2).
The value of average oxygen saturation of AW differed by -1.5% from the that of PSG, and showed a relatively consistent appearance even when the mean difference increased in the Bland-Altman plot. And the ICC value was 0.793, indicating a very high agreement with the PSG (Fig. 1C and Table 2). The lowest oxygen saturation of AW showed a difference of +10% on average from the PSG result, and the ICC value was 0.397, which showed a fair agreement with the PSG (Fig. 1D and Table 2).
The severity of OSA was classified according to the AHI of PSG, and several oxygen saturation parameters of PSG, GW, and AW according to OSA severity are shown in Table 3.
When comparing GW and PSG, the lowest oxygen saturation values according to severity were relatively similar, and in normal/mild/moderate/severe cases, 1.3%, 1.7%, 2.8%, and 5.1%, respectively, showed a tendency for GW to measure slightly higher. The oxygen saturation time of less than 90% of GW was -257 seconds in mild group and -2277 seconds in severe group compared to PSG except for the normal group, showing a tendency to be underestimated as the severity increased.
When comparing AW and PSG, the average oxygen saturation value tends to be measured 1%–2% higher than that of PSG, and the lowest oxygen saturation value is 10% higher than that of PSG in mild group and 15% in severe group showing a tendency to be overestimated as the severity increased.
When the cut-off value of the oxygen saturation time of less than 90% of GW was 7 seconds, the sensitivity was 82.9%, the specificity was 75.8%, and the accuracy was 80.7% (Table 4). The area under curve (AUC) value was 0.807, which could be evaluated as a ‘good’ diagnostic tool (Fig. 2A). If the cut-off value of the lowest oxygen saturation value of GW was determined as 88.0%, the sensitivity was 81.6%, the specificity was 69.7%, and the accuracy was 78% (Table 4). The AUC value was 0.849 in the ROC curve, which could be evaluated as a ‘good’ diagnostic tool (Fig. 2B).
However, in the case of AW, when the cut-off value of the average oxygen saturation was 95.7%, the sensitivity was 75.6%, the specificity was 70.8%, and the accuracy was 73.9% (Table 4). The AUC value was 0.757 in the ROC curve, which could be evaluated as a ‘fair’ diagnostic tool (Fig. 2C). If the cut-off value of the lowest oxygen saturation value of AW was determined as 93.0%, the sensitivity was 71.1%, the specificity was 62.5%, and the accuracy was 68.1% (Table 4). The AUC value was 0.705 in the ROC curve, which could be evaluated as a ‘fair’ diagnostic tool (Fig. 2D). The diagnostic performance of AW seems to be slightly inferior to that of GW.
When the cut-off value of the oxygen saturation time of less than 90% of GW was 36 seconds, the sensitivity was 72.7%, the specificity was 66.7%, and the accuracy was 69.7% (Table 5), and the AUC value was 0.722 in the ROC curve. It could be evaluated as a ‘fair’ diagnostic tool (Fig. 2A).
If the cut-off value of the lowest oxygen saturation of GW was 86.0%, the sensitivity was 70.9%, the specificity was 66.7%, and the accuracy was 68.8% (Table 5), and the AUC value was 0.762 in the ROC curve. It could be evaluated as a ‘fair’ diagnostic tool (Fig. 2B).
On the other hand, when the cut-off value of the average oxygen saturation of AW was 95.1%, the sensitivity was 75.0%, the specificity was 70.1%, and the accuracy was 72.5% (Table 5), and the AUC value was 0.753 in the ROC curve. It could be evaluated as a ‘fair’ diagnostic tool (Fig. 2C). If the cut-off value of the lowest oxygen saturation of AW was 93.0%, the sensitivity was 75.0%, the specificity was 51.2%, and the accuracy was 60.9% (Table 5), and the AUC value was 0.711 in the ROC curve. It could be evaluated as a ‘fair’ diagnostic tool (Fig. 2D).
Through this study, SWs, one of the user-friendly and popular wearable devices, was compared with PSG, the diagnostic gold standard of OSA, and the diagnostic performance and potential of SWs could be confirmed. In this study, in the case of GW, if the duration of the oxygen saturation time of less than 90% was more than 7 seconds or the lowest oxygen saturation level was less than 88.0%, it was possible to show approximately 78%–80% accuracy for estimating OSA.
AW showed approximately 68%–73% accuracy when diagnosing OSA with the average oxygen saturation was 95.7% or less or the lowest oxygen saturation was 93.0% or less.
Both GW and AW showed a significant degree of accuracy in diagnosing OSA with oxygen parameters, but the index of GW was more intuitive and accurate. In GW, if the oxygen saturation is lower than 90% regardless of time or lowest level, it can be easily seen that there is a problem with breathing during sleep. However, even the cut-off value of lowest oxygen saturation of AW is also more than 90%, so it is not easy for users to recognize their sleep disordered breathing by their oxygen saturation value. In addition, AW tends to overestimate the oxygen saturation by an average of 2%–10% compared to the PSG transmissive pulse oximeter, so there is a risk that general public may overestimate their real oxygen saturation during sleep.
The second significance of this study is that, unlike other studies that reanalyzed the data extracted from SWs [15], the oxygen saturation data displayed on the SW was directly compared with the that of PSG. Up to now, wearable devices have not been able to show an accuracy comparable to PSG due to various noises or limitation of the devices itself [8-10]. To overcome this, it was indispensable for researchers to extract raw data from SW and reanalyze it as a 30-second epoch base to reduce noises or errors. However, this reanalyze method requires time and the help of experts, so it is not practical because it is not readily available to the general public. In that regard, the authors made it easy to communicate the results of this study to the general public by comparing the accuracy of the real-world data of SWs with PSG.
OSA is also becoming a chronic disease that need to be checked frequently as the population ages to reduce the risk of OSA complications such as hypertension, ischemic heart disease, type 2 diabetes, and stroke increase. However, PSG, known as the gold standard for diagnosing OSA, is not an appropriate tool for managing OSA patients, which is increasing in cost, time, and efficiency. To supplement this, the AASM announced that the HSAT could be used under physician review for patients with suspected OSA [6]. However, it also states that ‘the HSAT should not be used for the general screening of asymptomatic populations’ [6]. However, some OSA patients are asymptomatic, some are unaware of the severity of their symptoms, and many patients are still difficult to see a doctor. Furthermore, PSG is too expensive and cumbersome to keep track of if you are getting the right treatment.
Level III HSAT lacks electroencephalography detection and can measure airflow, respiratory effort, and blood oxygenation. Recently, SWs have a built-in actigraphy and oxygen saturation sensors PPG-based, which can indirectly estimate airflow and breathing rate and intensity, and they are evolving to the level comparable to the level IV HSAT [15-17].
Although AHI is the only criterion for diagnosing OSA and assessing its severity, but oxygen saturation can also be a useful parameter, as hypoxia is known to impair physiological and cognitive function [13]. In supporting studies, there are reports that there is a correlation between oxygen desaturation index (ODI) and AHI, or that oxygen desaturation may better reflect the severity of OSA symptoms [11,12]. Using PSG and survey methods in 178 subjects, OSA severity based on AHI classification was correlated with various oxygen desaturation indices, and total and respiratory arousal indices were directly correlated with OSA severity [11]. A study using PSG and multiple sleep latency test in 362 patients with suspected OSA reported that an increase in the severity of 10% oxygen desaturation might significantly increase OSA-related daytime sleepiness risk than an increase in 10% AHI [12]. There is a study comparing ODI obtained with a single transmissive pulse oximeter with PSG and HSAT. The result of ODI by Single pulse oximeter had a significant correlation with RDI by HSAT and AHI by PSG. And the AUC of ROC for AHI ≥5 by PSG was reported to be 0.83 (confidence interval, 0.73–0.93), which could provide similar prediction of OSA [18]. The AUC of ROC in the above study is similar to the our GW predictive power.
There are two types of pulse oximeter: transmissive and reflectance.
The transmissive type has relatively high accuracy because it is attached to a thin area such as a fingertip or an earlobe in a clip method and emits light from one side and detects the light from the other side. However, wearable devices mainly worn on the wrist, such as SW, are a reflectance type that emits light from one side and detects it from the same side, so if it does not adhere well to the interface of the device and the skin of the wrist, it may occur noises or errors. In addition, errors may occur depending on the thickness of the wrist skin, color, and development of subcutaneous blood vessels. Clip type transmissive pulse oximeter is accurate but it limits finger movement, causing discomfort and may make long-term use difficult. However, the wrist-type reflectance oximeter is easy to wear and has the advantage of being able to measure multiple times or continuously for a long time.
Recently, a study comparing the GW and PSG transmissive pulse oximeter was reported in Korea. From the raw data of GW, ODI was derived as the number of episode of oxygen desaturation divided by the total sleep time. The sensitivity, specificity, and accuracy of ODI ≥5/h of GW for OSA diagnosis were 89.7%, 64.1%, and 79.4%, similar to our results [15]. In this study, it was confirmed that the ODI of GW had a strong positive correlation (r=0.918) with the AHI of PSG [15]. Our study is different in that it directly compared the parameters displayed in SW with PSG rather than recalculated parameters.
Similar study have been reported in China comparing OSA diagnosis with Chinese SW and PSG or HSAT. 119 patients were included in the study, and when SW compared to the PSG, the OSA prediction (AHI ≥5/h) accuracy, sensitivity, and specificity were 81.1%, 76.5%, and 100%, respectively. In addition, when SW compared to the HSAT, moderate OSA prediction (AHI ≥15/h) accuracy, sensitivity, and specificity were 87.9%, 89.7%, and 86.0%, respectively [16].
In our study, the diagnosis accuracy of GW using an oxygen saturation time of less than 90% was mild (80.7%), moderate (69.7%), and severe (63.3%) of OSA. As the severity of OSA increased, there was a large difference between the saturation value measured by PSG and that by GW, and the diagnostic accuracy decreased, showing a different patten from the study in China. In the Chinese study, indirect AHI was derived by estimating the PPG-based respiration rate using its own algorithm as well as the oxygen saturation. However, it did not describe in detail how to calculate the respiratory rate and how to distinguish between apnea and hypopnea.
Since this study mainly conducted at a sleep clinic that mainly examines OSA, the prevalence of OSA is very high, so it is difficult to say that it reflects the real world. It is necessary to study whether the false-positive rate in the OSA diagnosis of SW increases when a large number of normal subject are included, and whether this error can be overcome by repeated SW measurement for several nights.
Through this study, we were able to check the accuracy for diagnosis of OSA using SWs that anyone can use easily. Since digital health wearable devices such as SWs can easily measure and manage health-related bio-signals, it is expected that they will contribute to the diagnosis and evaluation of chronic sleep disorder such as OSA.
Notes
Availability of Data and Material
The datasets generated or analyzed during the study are available from the corresponding author on reasonable request.
References
1. Lee YJ. Updates of diagnosis and treatment of sleep-related breathing disorders-focusing on obstructive sleep apnea. J Korean Neuropsychiatr Assoc. 2020; 59(1):20–4.


2. Kim J, In K, Kim J, You S, Kang K, Shim J, et al. Prevalence of sleepdisordered breathing in middle-aged Korean men and women. Am J Respir Crit Care Med. 2004; 170(10):1108–13.
3. Gilman MP, Floras JS, Usui K, Kaneko Y, Leung RS, Bradley TD. Continuous positive airway pressure increases heart rate variability in heart failure patients with obstructive sleep apnoea. Clin Sci (Lond). 2008; 114(3):243–9.


4. Shahar E, Whitney CW, Redline S, Lee ET, Newman AB, Nieto FJ, et al. Sleep-disordered breathing and cardiovascular disease: cross-sectional results of the sleep heart health study. Am J Respir Crit Care Med. 2001; 163(1):19–25.


5. Harding SM. Complications and consequences of obstructive sleep apnea. Curr Opin Pulm Med. 2000; 6(6):485–9.


6. Caples SM, Anderson WM, Calero K, Howell M, Hashmi SD. Use of polysomnography and home sleep apnea tests for the longitudinal management of obstructive sleep apnea in adults: an American Academy of Sleep Medicine clinical guidance statement. J Clin Sleep Med. 2021; 17(6):1287–93.


7. Free C, Phillips G, Felix L, Galli L, Patel V, Edwards P. The effectiveness of M-health technologies for improving health and health services: a systematic review protocol. BMC Res Notes. 2010; 3:250.


8. Kim K, Park DY, Song YJ, Han S, Kim HJ. Consumer-grade sleep trackers are still not up to par compared to polysomnography. Sleep Breath. 2022; 26(4):1573–82.


9. Stone JD, Rentz LE, Forsey J, Ramadan J, Markwald RR, Finomore VS, et al. Evaluations of commercial sleep technologies for objective monitoring during routine sleeping conditions. Nat Sci Sleep. 2020; 12:821–42.
10. Moreno-Pino F, Porras-Segovia A, López-Esteban P, Artés A, Baca-García E. Validation of Fitbit Charge 2 and Fitbit Alta HR against polysomnography for assessing sleep in adults with obstructive sleep apnea. J Clin Sleep Med. 2019; 15(11):1645–53.


11. Wali SO, Abaalkhail B, AlQassas I, Alhejaili F, Spence DW, Pandi-Perumal SR. The correlation between oxygen saturation indices and the standard obstructive sleep apnea severity. Ann Thorac Med. 2020; 15(2):70–5.


12. Kainulainen S, Töyräs J, Oksenberg A, Korkalainen H, Sefa S, Kulkas A, et al. Severity of desaturations reflects OSA-related daytime sleepiness better than AHI. J Clin Sleep Med. 2019; 15(8):1135–42.


13. Rashid NH, Zaghi S, Scapuccin M, Camacho M, Certal V, Capasso R. The value of oxygen desaturation index for diagnosing obstructive sleep apnea: a systematic review. Laryngoscope. 2021; 131(2):440–7.


14. Zijlstra WG, Buursma A, Meeuwsen-van der Roest WP. Absorption spectra of human fetal and adult oxyhemoglobin, de-oxyhemoglobin, carboxyhemoglobin, and methemoglobin. Clin Chem. 1991; 37(9):1633–8.


15. Jung H, Kim D, Lee W, Seo H, Seo J, Choi J, et al. Performance evaluation of a wrist-worn reflectance pulse oximeter during sleep. Sleep Health. 2022; 8(5):420–8.


16. Chen Y, Wang W, Guo Y, Zhang H, Chen Y, Xie L. A single-center validation of the accuracy of a photoplethysmography-based smartwatch for screening obstructive sleep apnea. Nat Sci Sleep. 2021; 13:1533–44.


17. Papini GB, Fonseca P, van Gilst MM, Bergmans JWM, Vullings R, Overeem S. Wearable monitoring of sleep-disordered breathing: estimation of the apnea-hypopnea index using wrist-worn reflective photoplethysmography. Sci Rep. 2020; 10(1):13512.


18. Dawson A, Loving RT, Gordon RM, Abel SL, Loewy D, Kripke DF, et al. Type III home sleep testing versus pulse oximetry: is the respiratory disturbance index better than the oxygen desaturation index to predict the apnoea-hypopnoea index measured during laboratory polysomnography? BMJ Open. 2015; 5(6):e007956.


Fig. 1.
Bland-Altman plots comparing SpO2 parameters between each Smartwatch and PSG. A: GW showed good agreement results when the period of desaturation less than 90% was small, whereas the agreement rate decreased as the difference increased. B: The lowest recorded SpO2 parameter of GW showed a difference of -2.5% on average with the PSG result, and showed a good agreement in all sections. C: The parameter of average SpO2 of AW showed a difference of -1.5% with the PSG result, and showed a good agreement. D: The lowest SpO2 parameter of AW showed a difference of 10% with the PSG result, and showed a fair agreement. GW, Galaxy watch; AW, Apple watch; PSG, polysomnography; SpO2, oxygen saturation.
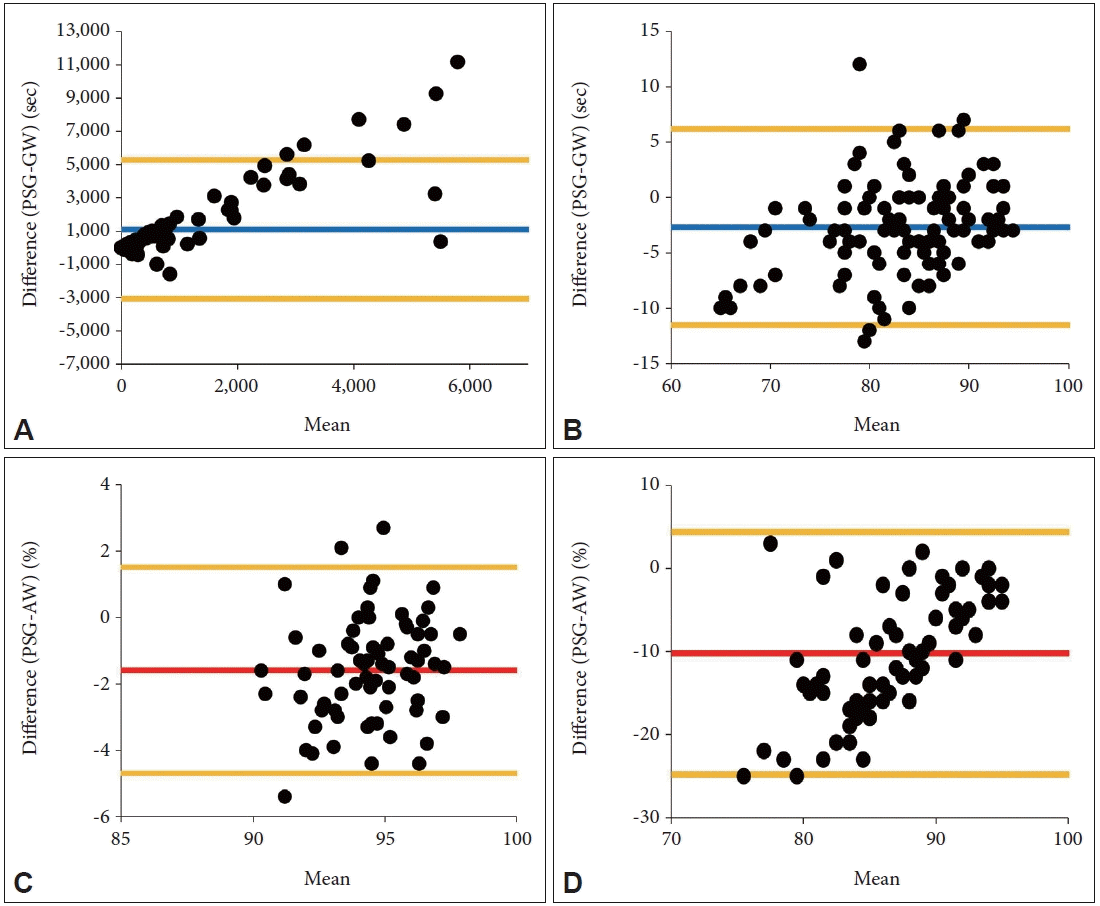
Fig. 2.
Receiver-operating characteristic curves of the various oxygen parameters of each Smartwatch in predicting AHI ≥5, AHI ≥15, and AHI ≥30. A: The duration of saturation less than 90% parameter of GW. B: The lowest oxygen saturation parameter of GW. C: The average oxygen saturation parameter of AW. D: The lowest oxygen saturation parameter of AW. GW, Galaxy watch; AW, Apple watch; AHI, apnea-hypopnea index.
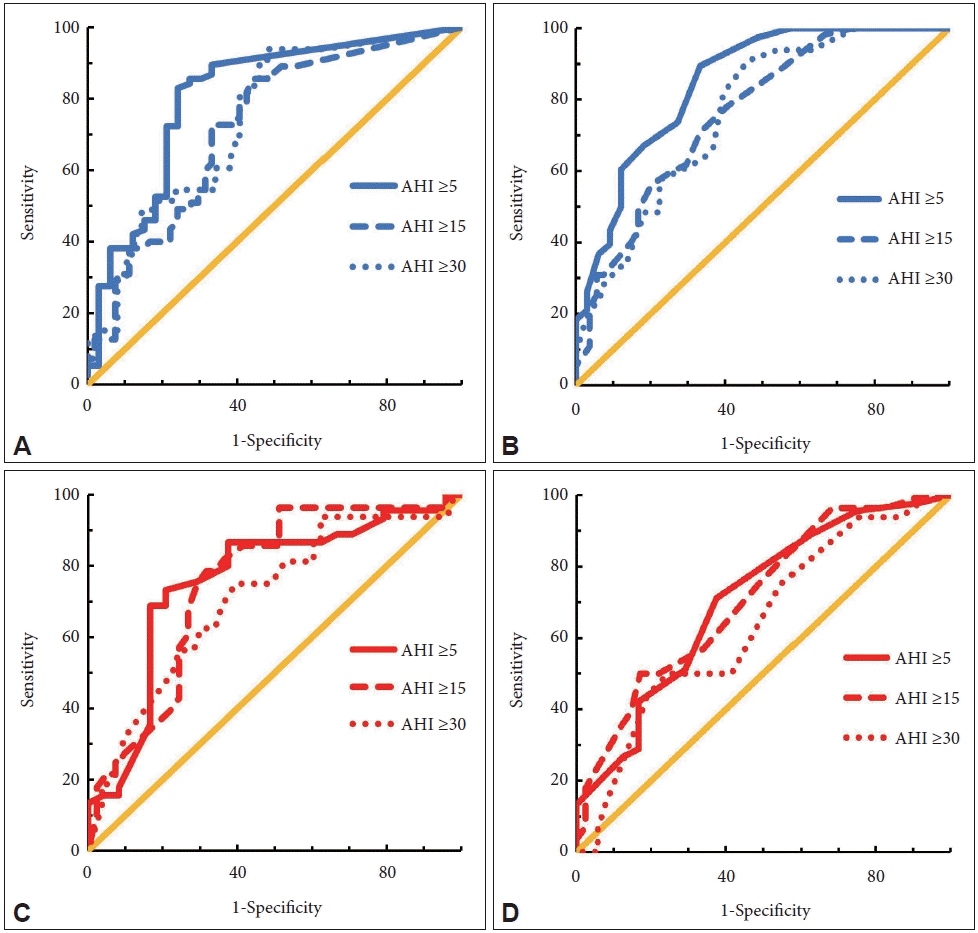
Table 1.
Patient demographics & comparison of PSG parameters between different Smartwatch wearers
Data are presented as mean±standard deviation or n (%). PSG, polysomnography; GW, Galaxy watch; AW, Apple watch; BMI, body mass index; ESS, Epworth Sleepiness Scale; HTN, hypertension; DM, diabetes mellitus; CVD, cerebrovascular disease; TST, total sleep time; AHI, apnea-hypopnea index; OSA, obstructive sleep apnea; SpO2, oxygen saturation
Table 2.
Agreement rate of SpO2 parameters between polysomnography and Smartwatches
Index | ICC | 95% CI | p-value |
---|---|---|---|
GW | |||
Duration of desaturation <90% | 0.441 | 0.184–0.618 | 0.001 |
SpO2 nadir | 0.909 | 0.866–0.937 | <0.001 |
AW | |||
Average SpO2 | 0.793 | 0.666–0.872 | <0.001 |
SpO2 nadir | 0.397 | 0.025–0.626 | 0.019 |
Table 3.
Oxygen saturation parameters among the PSG, GW, and AW according to the severity of OSA
Controls (AHI <5) | Mild OSA (5≤ AHI <15) | Moderate OSA (15≤ AHI <30) | Severe OSA (AHI ≥30) | p-value | ||
---|---|---|---|---|---|---|
PSG | ||||||
Average SpO2 (%) | 95.18±1.68*†‡ | 94.00±1.29§ | 93.63±1.63ǁ | 92.37±2.07 | <0.001 | |
Duration of desaturation <90% (sec) | 55.64±121.91 | 488.05±966.58¶§ | 1013.87±1541.73** | 2933.42±3379.59 | <0.001 | |
SpO2 nadir (%) | 88.53±4.81 | 82.41±5.64§ | 80.83±6.18** | 75.37±7.14 | <0.001 | |
GW | ||||||
Duration of desaturation <90% (sec) | 84.55±289.05*†‡ | 231.67±359.77 | 214.36±346.50** | 656.64±1166.58 | <0.001 | |
SpO2 nadir (%) | 89.82±4.54*†‡ | 84.05±5.62¶ | 83.64±5.91 | 80.52±6.40 | <0.001 | |
AW | ||||||
Average SpO2 (%) | 96.25±1.50‡ | 95.56±1.44‡‡ | 94.31±1.19 | 94.27±1.90 | 0.002 | |
SpO2 nadir (%) | 93.73±2.60‡ | 92.88±2.22 | 89.78±5.63 | 90.67±3.33 | 0.007 |
Table 4.
Diagnostic accuracy of GW and AW for diagnosis of OSA (AHI ≥5)
Table 5.
Diagnostic accuracy of GW and AW for diagnosis of OSA (AHI ≥15)