Abstract
Background
At outset of the coronavirus disease 2019 (COVID-19) pandemic, the significance of bacterial and fungal coinfections in individuals with COVID-19 was unknown. Initial reports indicated that the prevalence of coinfection in the general population was low, but there was uncertainty regarding the risk of coinfection in critically ill patients.
Methods
Nine hundred critically ill adult patients with COVID-19 infection were enrolled in this observational case-control study. Patients with a coinfection (case) and patients without a coinfection (control) were compared using univariate and multivariable analyses. A subgroup analysis was performed on patients with coinfection, dividing them into early (infection within 7 days) and late (infection after 7 days) infection groups.
Results
Two hundred and thirty-three patients (25.9%) had a bacterial or fungal coinfection. Vasopressor use (P<0.001) and severity of illness (higher Acute Physiology and Chronic Health Evaluation III score, P=0.009) were risk factors for the development of a coinfection. Patients with coinfection had higher mortality and length of stay. Vasopressor and corticosteroid use and central line and foley catheter placement were risk factors for late infection (>7 days). There were high rates of drug-resistant infections.
Conclusions
Critically ill patients with COVID-19 are at risk for both community-acquired and hospital-acquired infections throughout their hospitalization for COVID-19. It is important to consider the development of a coinfection in clinically worsening critically ill patients with COVID-19 and consider the likelihood of drug-resistance when choosing an empiric regimen.
Over 400 million people have been infected with severe acute respiratory syndrome coronavirus 2 (SARS-CoV-2), the virus responsible for coronavirus disease 2019 (COVID-19), since it was first detected in the city of Wuhan in Hubei Province, China [1]. At the beginning of the COVID-19 pandemic, the significance of bacterial and fungal coinfections in individuals with COVID-19 was unknown. Prior outbreaks of respiratory viruses, such as influenza, have been associated with high rates of bacterial coinfection through viral impairment of mucociliary clearance, increased bacterial adherence, airway damage, and immune dysregulation [2,3]. Mortality attributed to coinfection in viral illness has decreased overtime since the 1918 influenza pandemic, with only 20% of influenza cases being complicated by bacterial pneumonia during the 2009 H1N1 influenza A pandemic [2].
Data collected through the first year of the pandemic suggests that the prevalence of secondary infections in patients with COVID-19 is low, with many cohorts reporting approximately 7% of patients with a coinfection, despite over 90% of patients receiving antimicrobials [4-9]. On the contrary, patients with COVID-19 admitted to the intensive care unit (ICU) are at a higher risk for developing coinfections. Studies have reported a 32.7% to 100% incidence of secondary bacterial infections [10-14]. The presence of these infections has been associated with increased morbidity and mortality [15,16]. While coinfections in patients with COVID-19 may be relatively uncommon, the high rate of antimicrobial use has likely contributed to the low rate of microbiologically confirmed infections [17].
The clinical features of COVID-19 infection are difficult to distinguish from bacterial pneumonia. Biomarkers, such as procalcitonin (PCT), have been used to determine the likelihood of bacterial infections in patients with COVID-19 infection [18]. However, elevated PCT has not been found to be correlated with the presence of a bacterial infection in COVID-19 patients, but may be an independent predictor of mortality [19].
Given the high rates of antimicrobial administration and the trend toward prolonged hospitalizations, we suspected that hospital-acquired infections (HAI) represent an important segment of coinfections in critically ill patients with COVID-19. This study aims to understand the risk factors which can help clinicians identify patients at high risk for coinfection and mortality. Unlike previously reported studies, we examined the occurrence of coinfections in a bimodal distribution; early compared to late and identified risk factors for both time periods.
This retrospective, observational case-control study was conducted at 16 hospitals within the Cleveland Clinic Health System, with locations throughout Northeastern Ohio and Florida. All adult patients with a positive polymerase chain reaction (PCR) test for COVID-19 admitted to the medical ICU between March 15, 2020, and August 31, 2020 were identified in an internal ICU registry. Ethical approval for this study was granted by the Cleveland Clinic Institutional Review Board (No. 20-404). Consent was waived in accordance with the Cleveland Clinic Institutional Review Board due to the retrospective nature of this study.
Patients were included if they had PCR confirmed SARS-CoV-2 and were admitted to the medical ICU during their hospital admission for COVID-19 infection. Patients were excluded if they did not have PCR confirmed COVID-19, age <18, or if they were participating in a clinical trial. Patients were divided into two groups: case group and control group. The case group was defined by the presence of a concurrent bacterial or fungal infection and the control group was defined by the absence of a concurrent infection. A coinfection was defined as a positive body fluid culture considered by the treating team as clinically relevant either per documentation or treatment with antibiotics per chart review. Cultures with pathogens considered to be contaminants were excluded (for example, Candida species [spp.] from respiratory cultures and coagulase negative Staphylococcus spp. which was not considered clinically significant). In a subgroup analysis of the patients in the case cohort, we divided the patients into early versus late infection based on the timing of infection development. The early infection group was defined as patients who were diagnosed with coinfection within 7 days of hospitalization and the late infection group was defined as patients who developed coinfection later (>7 days) in their hospital course. We chose to define late infection as 7 days after hospital admission in order to exclude pathogens acquired in the community. The risk of HAI increases with length of stay (LOS), frequently occurring after one week of hospital admission [20,21].
The following data were compiled for analysis. Demographic data collected included weight, height, age, sex, race, comorbid conditions, and Acute Physiology and Chronic Health Evaluation (APACHE) III score. Laboratory data collected included C-reactive protein (CRP), ferritin, PCT, lactate dehydrogenase, and D-dimer. The following clinical data variables were collected: use of paralytics, vasopressors, antibiotics, and COVID-19 specific therapies (corticosteroids, tocilizumab, remdesivir, etc.), presence of indwelling devices (foley catheter, endotracheal tube, and central venous access), presence of a coinfection, and presence of a drug-resistant infection.
The primary outcome was identification of risk factors for the development of coinfections among patients with COVID-19. Secondary outcomes were hospital and ICU mortality and ICU and hospital LOS, and discharge disposition. In a subgroup analysis we identified risk factors between the subset of patients who developed “early” versus “late” infection.
Descriptive statistics were used to characterize the patient cohort. Patients with a coinfection (cases) and patients without a coinfection (controls) were compared using univariate analysis and multivariable analysis. A subgroup analysis was performed on patients with coinfection. They were divided into two groups early versus late coinfection. Early coinfection was defined as within 7 days of hospital admission and late was defined as after 7 days into hospital admission. Multivariable analyses were performed comparing the early group to the controls and the late group to the controls, controlling for the same variables listed above.
The study variables were described using sample mean with standard deviation or count with percentage as appropriate. Continuous variables were compared using Wilcoxon Rank Sum test, whereas categorical variables were compared using Pearson’s chi-square test or Fisher’s exact test. Multivariable logistic regression analysis was conducted to study the associations between the predictors and the outcome. We controlled for body mass index (BMI), age, race, immune suppression, end-stage renal disease, APACHE III score, vasopressor, corticosteroid, and tocilizumab use, CRP, PCT closest to the infection, and foley and central line insertion. The variables in the multivariable model were selected based on clinical literature and experience caring for critically ill COVID-19 patients.. Association between these variables and the COVID-19 coinfection were expressed as odds ratio (OR) with its 95% confidence interval (CI). Missing values were imputed using the MissForest method using R package missForest [22]. All analyses were two-tailed and were performed at a significance level of 0.05. SAS 9.4 software (SAS Institute, Cary, NC, USA) and R version 4.0.0 (The R Foundation for Statistical Computing, Vienna, Austria) were used for all analyses.
There were 1,019 patients screened and after appropriate exclusion, 900 critically ill patients with COVID-19 were included in the study (Figure 1). Two hundred and thirty-three patients (25.9%) had a bacterial or fungal coinfection. There was no difference in the weight, BMI, sex, age, and race in patients with and without coinfection. Baseline characteristics are reported in Table 1. Among the 233 patients with a bacterial or fungal coinfection, there were a total of 333 infections. The distribution of coinfections are as follows: 308 bacterial (92.5%) and 25 fungal (7.5%) (Table 2). 24.1% of early infections (≤7 days from admission) were multidrug-resistant while 32.6% of late infections (>7 days from admission) were multidrug-resistant. While there was a trend towards increased resistance in the late infection group, this result was not statistically significant. Of the bacterial infections, 18 cultured pathogens produced extended spectrum beta-lactamases (ESBL). All ESBL were Escherichia coli or Klebsiella pneumoniae (24% of isolates) and six pathogens were carbapenem-resistant Enterobacteriaceae. There were 92 (27.6%) multi-drug resistant infections, resistant to three or more antimicrobial classes [23]. The most common source of coinfection was pulmonary with 141 infections (42.3%), followed by urinary (27.0%) and blood-stream infections (21.9%) (Table 3).
On univariate analysis, patients with coinfections had higher APACHE III scores (53.9 vs. 65.8, P<0.001) than those without coinfection. Patients with coinfections received corticosteroids (66.1% vs. 56.8%, P=0.013) and tocilizumab (22.7% vs. 13.2%, P=0.001) at a higher rate than those without coinfections. Among all critically ill patients with COVID-19, 749 (83.2%) received antibiotics with a mean duration of therapy of 8.7 days. Patients without a coinfection received an average of 6.7 days of antimicrobial therapy, compared with 14.4 days in those with a coinfection. Patients with coinfections had more central lines, endotracheal tubes, and indwelling foley catheters (Table 1).
Patients with coinfection had higher PCT values during their hospital admission (5.9 vs. 3.0, P<0.001). This trend was present prior to development of a coinfection, however the difference seen is unlikely to be clinically meaningful (PCT 3.6 vs. 3.1, P=0.005). Similarly, for ferritin (7,638.8 vs. 2,874.4, P<0.001) and CRP (19.8 vs. 26.7, P<0.001) the patients with coinfection had statistically significant elevations in these markers, but the elevations did not occur prior to the microbiological diagnosis of infection. Therefore, biomarkers did not predict the onset of infection (Table 1).
In the multivariable model, APACHE III score and the use of vasopressors were associated with the development of a coinfection. There was no association between elevation in PCT and subsequent development of coinfection (Table 4). The coinfection group had a higher rate of ICU mortality (15.0% vs. 29.7%, P<0.001) and hospital mortality (20.1% vs. 34.5%, P<0.001). ICU LOS (13.8 vs. 6.7 days, P<0.001) and hospital LOS (18.6 vs. 11.8 days, P<0.001) were longer in the coinfections group (Table 5). Patients with coinfections were less likely to be discharged to home (14.2% vs. 45.9%, P<0.001) and more likely to discharge to a skilled nursing facility or rehabilitation (38.8% vs. 25.1%, P<0.001) (Table 5).
Of the 233 patients with coinfection included in the subgroup analysis, 142 patients (60.9%) had their first coinfection within the first 7 days and 91 patients (39.1%) had their first coinfection after 7 days (Table 6). Seventy-one patients experienced multiple infections. Among them, 40 (56.3%) had their first infection within 7 days and 31 (43.7%) had their first infection after 7 days. Patients with early coinfection were older age and had higher APACHE III scores. There were higher rates of neuromuscular blockade, vasopressors, corticosteroids, tocilizumab, remdesivir, foley catheters, central venous lines, and mechanical ventilation in the late infection group. In the multivariable analysis of the late group, the use of vasopressors (OR, 2.71; 95% CI, 1.245–5.881) and corticosteroids (OR, 2.08; 95% CI, 1.155–3.734]) and the placement of a foley catheter (OR, 4.40; 95% CI, 1.820–10.657) and central line (OR, 2.53; 95% CI, 1.176–5.426) increased the risk for late coinfection (not shown).
Those who developed an early coinfection had a lower hospital LOS and ICU LOS compared to the late group 13.9 days vs. for 25.8 days, P<0.001 and 9.5 days vs. 20.4 days, P<0.001, respectively. There was no difference in ICU mortality, hospital mortality, or discharge disposition between the two groups.
In our study, severity of illness (higher APACHE III score) and vasopressor use were associated with higher risk of developing coinfections in critically ill COVID-19 patients. Risk factors associated with developing a late coinfection (>7 days post admission) included vasopressor and corticosteroid use and placement of a foley catheter or central line. Clinical biomarkers were not associated with the development of coinfections in critically ill patients with COVID-19. There were higher rates of drug resistance in our population than previously seen in our ICU in pre-pandemic cohorts [24]. Patients with coinfection had higher hospital and ICU mortality, hospital and ICU LOS, and were less likely to be discharged to home.
As has been described in other recent studies, critically ill patients with COVID-19 experience a higher rate of coinfection (25.9%) than previously reported in all hospitalized patients with COVID-19 [4-9]. Our cohort shows that higher severity of illness at presentation, need for vasopressors, and use of neuromuscular blockade and indwelling devices, such as foley catheters, endotracheal tubes, and central venous access were all risk factors for development of infections. Nasir et al. similarly showed that severity of illness and the use of steroids were risk factors for the development of coinfection in critically ill COVID patients [25]. These findings are consistent with reports in other viral illness such as influenza, which have identified higher APACHE III score, older age, diabetes, and sepsis as risk factors for bacterial coinfection [26].
Our study demonstrates that there are unique differences in risk factors for coinfection among those with early versus late infection, suggesting that these are distinct populations. Interestingly, patients in the late infection group were twice as likely to develop a coinfection if they received corticosteroids. Corticosteroids are known to be associated with an increased risk of infection, but their use has increased in patients with COVID-19 since benefit was shown in the RECOVERY trial (dexamethasone in hospitalized patients with Covid-19 by the RECOVERY Collaborative Group) [27,28]. Retrospective data from New York City hospitals also found an increase in bacterial (25% vs. 13.1%, P=0.041) and fungal (12.7% vs. 0.7%, P<0.001) infections in patients with COVID-19 who received corticosteroids [29].
Unsurprisingly, the use of central lines and foley catheters was associated with an increased risk of late coinfections, further illustrating the need to remove temporary indwelling devices, when they are not clinically indicated. The analysis of early vs. late coinfections shows that critically ill patients with COVID-19 remain at high risk of infection throughout their hospital course. Therefore, evidence of worsening shock or respiratory failure should be promptly recognized with the need for empiric antibiotics addressed. In our study, there were a large number of patients diagnosed with coinfection after 7 days of admission (late group), indicating that HAI represent a significant proportion of coinfections for those with COVID-19. The increased risk of nosocomial and drug-resistance has been supported by recent data. Italian hospitals have reported 46% of critically ill patients with COVID19 develop a HAI, with high rates of ventilator-associated pneumonia and catheter-associated blood stream infections [30]. These infections have been more commonly associated with drug resistance [30,31].
Our study revealed that biomarkers (PCT, CRP, and ferritin) are poor predictors of the presence of a coinfection, as elevations did not occur reliably prior to their diagnosis. Although PCT has been used in critically ill patients with COVID-19 for antibiotic de-escalation, the role of an elevated PCT in predicting the presence of a coinfection is less clear [32]. Elevated PCT has been seen in patients with severe COVID-19, but it has not been correlated with the presence of a bacterial infection [19,33]. An elevated PCT and other biomarkers, such as CRP and ferritin, have been shown to be associated with increased mortality in COVID-19 patients and may be an independent predictor of mortality [19,34].
Overlapping clinical features between bacterial pneumonia and COVID-19 leads to near ubiquitous use of antibiotics among these patients which may lead to increased antibiotic resistance. Our cohort had 92 (27.6%) multi-drug resistant infections. The rates of ESBL among E. coli and K. pneumoniae isolates seen in critically ill COVID-19 patients were higher than previously reported in our institutions antibiogram [24]. In our population, fungal infections were relatively uncommon.
The presence of coinfections has serious implications for critically ill patients with COVID-19. Patients with coinfection had longer LOS, higher mortality, and were less likely to be discharged to home. Coinfections likely contribute to decreased quality of life for patients and increased healthcare costs due to increased LOS and the need for long-term medical care. Patients with late infection had longer hospital and ICU LOS.
The strengths of our analysis include the inclusion of multiple medical centers which allowed for recruitment of a large number of patients and examining a protracted period of the pandemic. We performed multivariable analysis to account for confounders and determine which variables are associated with coinfection. Additionally, our study is unique in that it is the first case-control series that examines infections in a bimodal model (early vs. late infection) as distinct entities with unique risk factors. Limitations of this study include the retrospective nature of the analysis and possible poor generalizability to ICUs in resource-limited environments. Another limitation of our analysis is that the true prevalence of coinfection is difficult to elucidate. High rates of early antibiotic exposure decrease the likelihood of a positive culture despite true coinfection and accurately determining contaminant versus pathogen in a patient with a compatible clinical picture is nearly impossible. While there is no gold standard to determine true coinfection, and false positive (contaminant) and false negative results were possible, these data demonstrate the poor clinical outcomes for critically ill patients with COVID-19 and coinfection.
The presence of coinfections has serious implications for critically ill patients with COVID-19. Severity of illness and vasopressor use was associated with higher risk of developing coinfection in critically ill patients with COVID-19. Coinfections occur in a bimodal distribution and patients remain at high risk of developing coinfections throughout their hospital course. While biomarkers are elevated in patients with COVID-19, they cannot be reliably used to predict the presence or likelihood of developing a coinfection.
▪ Bacterial and fungal coinfections occur more commonly in critically ill patients with coronavirus disease 2019 (COVID-19) than non-critically ill hospitalized patients.
▪ Clinicians should maintain a high index of suspicion for hospital-acquired infections in COVID-19 patients with increased severity of illness, indwelling medical devices, and prolonged hospital length of stay.
ACKNOWLEDGMENTS
This project was supported by the SMARRT T32 which is funded by the National Institutes of Heart, Lung and Blood grant, T32HL155005. The content is solely the responsibility of the authors and do not necessarily represent the official views of the NIH.
REFERENCES
1. Johns Hopkins University and Medicine. COVID-19 Map: Johns Hopkins coronavirus resource center [Internet]. Baltimore (MD): Johns Hopkins University and Medicine;2021. [cited 2022 May 3]. Available from: https://coronavirus.jhu.edu/map.html.
2. Metersky ML, Masterton RG, Lode H, File TM Jr, Babinchak T. Epidemiology, microbiology, and treatment considerations for bacterial pneumonia complicating influenza. Int J Infect Dis. 2012; 16:e321–31.
3. Bakaletz LO. Viral-bacterial co-infections in the respiratory tract. Curr Opin Microbiol. 2017; 35:30–5.
4. Hughes S, Troise O, Donaldson H, Mughal N, Moore L. Bacterial and fungal coinfection among hospitalized patients with COVID-19: a retrospective cohort study in a UK secondary-care setting. Clin Microbiol Infect. 2020; 26:1395–9.
5. Garcia-Vidal C, Sanjuan G, Moreno-García E, Puerta-Alcalde P, Garcia-Pouton N, Chumbita M, et al. Incidence of co-infections and superinfections in hospitalized patients with COVID-19: a retrospective cohort study. Clin Microbiol Infect. 2021; 27:83–8.
6. Karaba SM, Jones G, Helsel T, Smith LL, Avery R, Dzintars K, et al. Prevalence of co-infection at the time of hospital admission in COVID-19 patients, a multicenter study. Open Forum Infect Dis. 2020; 8:ofaa578.
7. Youngs J, Wyncoll D, Hopkins P, Arnold A, Ball J, Bicanic T. Improving antibiotic stewardship in COVID-19: Bacterial co-infection is less common than with influenza. J Infect. 2020; 81:e55–7.
8. Sepulveda J, Westblade LF, Whittier S, Satlin MJ, Greendyke WG, Aaron JG, et al. Bacteremia and blood culture utilization during COVID-19 surge in New York City. J Clin Microbiol. 2020; 58:e00875–20.
9. Rawson TM, Moore L, Zhu N, Ranganathan N, Skolimowska K, Gilchrist M, et al. Bacterial and fungal coinfection in individuals with coronavirus: a rapid review to support COVID-19 antimicrobial prescribing. Clin Infect Dis. 2020; 71:2459–68.
10. Bhatt PJ, Shiau S, Brunetti L, Xie Y, Solanki K, Khalid S, et al. Risk factors and outcomes of hospitalized patients with severe coronavirus disease 2019 (COVID-19) and secondary bloodstream infections: a multicenter case-control study. Clin Infect Dis. 2021; 72:e995–1003.
11. Sharifipour E, Shams S, Esmkhani M, Khodadadi J, Fotouhi-Ardakani R, Koohpaei A, et al. Evaluation of bacterial co-infections of the respiratory tract in COVID-19 patients admitted to ICU. BMC Infect Dis. 2020; 20:646.
12. Zhang H, Zhang Y, Wu J, Li Y, Zhou X, Li X, et al. Risks and features of secondary infections in severe and critical ill COVID-19 patients. Emerg Microbes Infect. 2020; 9:1958–64.
13. Baskaran V, Lawrence H, Lansbury LE, Webb K, Safavi S, Zainuddin NI, et al. Co-infection in critically ill patients with COVID-19: an observational cohort study from England. J Med Microbiol. 2021; 70:001350.


14. d'Humières C, Patrier J, Lortat-Jacob B, Tran-Dinh A, Chemali L, Maataoui N, et al. Two original observations concerning bacterial infections in COVID-19 patients hospitalized in intensive care units during the first wave of the epidemic in France. PLoS One. 2021; 16:e0250728.
15. Lansbury L, Lim B, Baskaran V, Lim WS. Co-infections in people with COVID-19: a systematic review and meta-analysis. J Infect. 2020; 81:266–75.


16. Langford BJ, So M, Raybardhan S, Leung V, Westwood D, MacFadden DR, et al. Bacterial co-infection and secondary infection in patients with COVID-19: a living rapid review and meta-analysis. Clin Microbiol Infect. 2020; 26:1622–9.


17. Chang CY, Chan KG. Underestimation of co-infections in COVID-19 due to non- discriminatory use of antibiotics. J Infect. 2020; 81:e29–30.
18. Han J, Gatheral T, Williams C. Procalcitonin for patient stratification and identification of bacterial co-infection in COVID-19. Clin Med (Lond). 2020; 20:e47.


19. Vanhomwegen C, Veliziotis I, Malinverni S, Konopnicki D, Dechamps P, Claus M, et al. Procalcitonin accurately predicts mortality but not bacterial infection in COVID-19 patients admitted to intensive care unit. Ir J Med Sci. 2021; 190:1649–52.


20. Loke HY, Kyaw WM, Chen M, Lim JW, Ang B, Chow A. Length of stay and odds of MRSA acquisition: a dose-response relationship? Epidemiol Infect. 2019; 147:e223.


21. Tess BH, Glenister HM, Rodrigues LC, Wagner MB. Incidence of hospital-acquired infection and length of hospital stay. Eur J Clin Microbiol Infect Dis. 1993; 12:81–6.


22. Stekhoven DJ, Bühlmann P. MissForest: non-parametric missing value imputation for mixed-type data. Bioinformatics. 2012; 28:112–8.


23. Magiorakos AP, Srinivasan A, Carey RB, Carmeli Y, Falagas ME, Giske CG, et al. Multidrug-resistant, extensively drug-resistant and pandrug-resistant bacteria: an international expert proposal for interim standard definitions for acquired resistance. Clin Microbiol Infect. 2012; 18:268–81.


24. Cleveland Clinic Foundation. Cumulative antimicrobial susceptibility of bacteria from cultures at CCF main campus. Cleveland: Cleveland Clinic Intranet.
25. Nasir N, Rehman F, Omair SF. Risk factors for bacterial infections in patients with moderate to severe COVID-19: a case-control study. J Med Virol. 2021; 93:4564–9.


26. Klein EY, Monteforte B, Gupta A, Jiang W, May L, Hsieh YH, et al. The frequency of influenza and bacterial coinfection: a systematic review and meta-analysis. Influenza Other Respir Viruses. 2016; 10:394–403.


27. RECOVERY Collaborative Group, Horby P, Lim WS, Emberson JR, Mafham M, Bell JL, et al. Dexamethasone in hospitalized patients with COVID-19. N Engl J Med. 2021; 384:693–704.


28. George MD, Baker JF, Winthrop K, Hsu JY, Wu Q, Chen L, et al. Risk for serious infection with low-dose glucocorticoids in patients with rheumatoid arthritis : a cohort study. Ann Intern Med. 2020; 173:870–8.


29. Obata R, Maeda T, Rizk D, Kuno T. Increased secondary infection in COVID-19 patients treated with steroids in New York City. Jpn J Infect Dis. 2021; 74:307–15.


30. Bassetti M, Kollef MH, Timsit JF. Bacterial and fungal superinfections in critically ill patients with COVID-19. Intensive Care Med. 2020; 46:2071–4.


31. Grasselli G, Pesenti A, Cecconi M. Critical care utilization for the COVID-19 outbreak in Lombardy, Italy: early experience and forecast during an emergency response. JAMA. 2020; 323:1545–6.


32. Heesom L, Rehnberg L, Nasim-Mohi M, Jackson A, Celinski M, Dushianthan A, et al. Procalcitonin as an antibiotic stewardship tool in COVID-19 patients in the intensive care unit. J Glob Antimicrob Resist. 2020; 22:782–4.


Figure 1.
Flowchart of patients included in the study. PCR: polymerase chain reaction; COVID-19: coronavirus disease 2019.
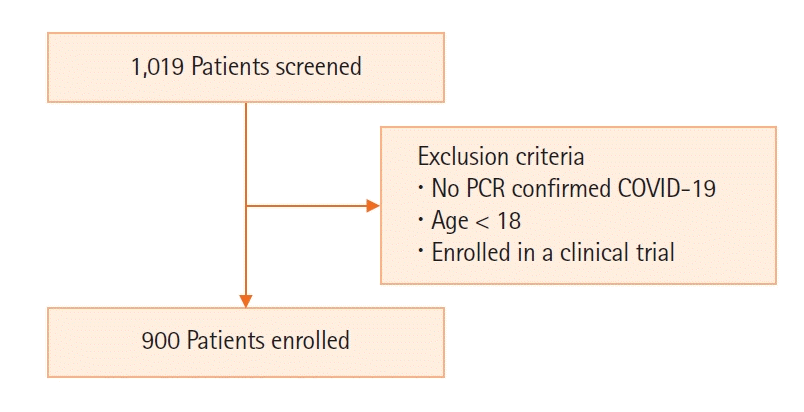
Table 1.
Baseline characteristics of critically Ill COVID-19 patients
Values are presented as mean±standard deviation or number (%).
COVID-19: coronavirus disease 2019; BMI: body mass index; COPD: chronic obstructive pulmonary disease; ESRD: end-stage renal disease; APACHE: Acute Physiology and Chronic Health Evaluation; ICU: intensive care unit; CRP: C-reactive protein; LDH: lactate dehydrogenase.
Table 2.
Early versus late pathogens resulting in coinfection
Table 3.
Sources of coinfection in critically Ill COVID-19 patients
Source | Value (n=333) |
---|---|
Sputum/pulmonary | 141 |
Urine | 90 |
Blood | 73 |
Stool | 13 |
Wound | 13 |
Abdominal fluid | 2 |
Sinus | 1 |
Table 4.
Multivariable analysis: risk factors for development of coinfection in critically Ill COVID-19 patients
Table 5.
Outcomes of critically Ill COVID-19 patients
Table 6.
Comparison of early versus late infection baseline characteristics