Abstract
Background
The selective leukoreduction protocol (SLP) is limited in that patients who require it can be overlooked. We estimated SLP compliance (SLPC) using the Observational Medical Outcomes Partnership common data model (CDM).
Methods
Patients were classified into eight groups pre- and post-hematology disease (A and B), pre- and post-solid organ transplantation (C and D), solid cancer (E), immunodeficiency (F), anticancer therapy (G), and cardiovascular surgery (H). We examined the red blood cell (RBC) transfusion history from three hospital datasets comprising approximately three million patients over 20 years using CDM-based analysis. SLPC was calculated as the percentage of patients who received only leukoreduced RBCs in total patients transfused RBCs.
Results
In total, 166,641 patients from three hospitals were enrolled in this study. From 2001 to 2021, SLPC in all groups, except H, tended to increase, although there were differences among the hospitals. Based on the most recent values (2017–2021), the SLPC in groups A, B, D, and G was maintained at ≥75% until 1,095 days before or after diagnosis or treatment. Groups E, F, and H had < 50% SLPC one day after diagnosis and treatment.
Reduction of residual allogeneic leukocytes in transfused blood components is desirable to prevent HLA alloimmunization, platelet refractoriness, cell‐associated pathogens, such as cytomegalovirus (CMV) transmission, and febrile nonhemolytic transfusion reactions (FNHTRs) [1]. Universal leukoreduction (ULR) is a routine leukoreduction of all units of blood components prior to storage or transfusion [2]. Several countries, including France, Germany, and England, have implemented ULR. In other countries, such as Korea and the United States, ULR adoption has been delayed because of economic issues. Instead, they adopted selective leukoreduction protocols (SLPs) for patients considered to be at the greatest risk of experiencing adverse effects associated with the presence of leukocytes in blood components [2]. In Korea, National Health Insurance benefit criteria for selected patient populations (including patients who had a FNHTR, hematological disease, solid cancer requiring repeated platelet transfusions, previous or planned solid organ transplant, or cardiac disease, or are aged <1 year or immunocompromised) were announced in 2008 and revised in 2016. However, in Korea, leukoreduced (LR) RBCs (LRRBCs) accounted for 18.6% of total RBCs transfused in 2012 [3], whereas in the United States, LRRBCs accounted for 84% of whole blood/RBCs transfused in 2011 [4].
SLP is limited in that patients who require it can be overlooked. For example, in a trial to reduce alloimmunization to platelets, 85% of patients who received transfusion two weeks before trial entry were transfused with non-LR (NLR) blood [5]. However, there was no report of how many patients were overlooked among those who met the criteria for using LR blood components, because it is difficult to review large numbers of medical records for various patient groups. Recently, the common data model (CDM) has been used to review large datasets from multiple institutions [6]. A CDM is an informational model that allows the transformation of data contained in different databases to a common format, in which all coding and vocabulary are prespecified and standardized [7]. The Observational Medical Outcomes Partnership (OMOP) is an international collaboration that aims to create and apply open-source data analytic solutions to a large network of health databases [8]. In 2021, an OMOP-based CDM database was established in four institutions of Soonchunhyang Medical Center (Fig. 1). We aimed to estimate SLP compliance (SLPC) in three medical institutions in Korea using the CDM database analysis method.
The three hospitals belonging to the Soonchunhyang Medical Center (Soonchunhyang University Bucheon Hospital [SCHBC], Soonchunhyang University Cheonan Hospital [SCHCA], and Soonchunhyang University Seoul Hospital [SCHSU]) used a single electronic medical record (SU-III, Dongeun Information Technologies, Bucheon, Korea), converted into CDM format datasets (SCHBC_5.3.1_01, SCHCA_5.3.1_02, and SCHSU_5.3.1_
02, respectively) using FEEDER-NET (https://feedernet.com) by EvidNet (Seongnam, Korea). Therefore, data quality review was omitted. The datasets were analyzed using ATLAS version 2.7.2 (https://github.com/OHDSI/Atlas). Table 1 lists the source and mapping terms of the medical records uploaded to the database. The data collection period for each database is indicated in Fig. 2. This study was conducted in accordance with the principles of the Declaration of Helsinki (2013). The Institutional Review Board of SCHBC waived the approval of this study (file no. 2022-01-011) because we used de-identified data (Table 1, Fig. 2) [9].
Patients were classified into eight groups according to the Ministry of Health and Welfare notice on leukoreduction: pre- and post-hematology disease (A and B), pre- and post-solid organ transplantation (SOT) (C and D), solid cancer (E), immunodeficiency (F), anticancer therapy (G), and cardiovascular surgery (H). The initial occurrence of at least one of the Korean Standard Classification of Diseases (KCD) or electronic data interchange (EDI) codes in each group was defined as a cohort entry event, and repeated codes for the same patient were excluded. The following data sources and codes were used to define each group: A and B (KCD7): C90–96 and D61; C and D (EDI): R3280, Q8040, Q8042, Q8046–8049, and Q8140; E (KCD7): C00–26, C30–34, C37–46, C48–58, C60–78, C80–88, and C97; F (KCD7): B20–24, D80–84, and D86; G (KCD7): Z51.0–51.2; H (EDI): O1890, O1640–1644, O1646, O1648, O1649, O0162–0174, O1671–1672, O1690, O1701, O1701, O1710, O1711, O1721, O1722, O1750, O1770, O1781–1783, O1791–1798, O1799, O1800, O1810, O1821–1823, O1825, O1830, O1841, O1843, O1844, O1861, O1875, and O1878.
Each group was analyzed independently. For example, if a patient diagnosed as having kidney cancer (C64, malignant neoplasm of the kidney, except the kidney pelvis) received anticancer therapy (Z51.1, chemotherapy session for neoplasm) a month later and a kidney transplant (R3280, kidney transplantation) 10 years later, the patient was entered into groups E (on the date of the initial diagnosis of kidney cancer), G (on the date of initial chemotherapy), and C and D (on the date of kidney transplantation). However, if the patient received a kidney transplant again after 5 years, the re-transplantation patient was not entered into groups C and D. The observation times were set to 1, 7, 30, 90, 180, 365, 730, and 1,095 days after the entry event occurred.
SLPC was calculated as the percentage of patients who received only LRRBCs in total patients transfused RBCs. At each time point, the number of patients who received all types of RBCs was counted. SLPC was calculated as follows:
For example, in the pre-SOT group, the numbers of patients who received LRRBC and NLRRBC transfusions at least once during the year prior to SOT surgery were obtained through the CDM, and SLPC was calculated (Fig. 2). Differences in percentages between the three institutions were analyzed using a linear-by-linear association test and the chi-square test. All statistical analyses were performed using IBM SPSS Statistics version 22.0 (IBM Corporation, Armonk, NY, USA). All tests were two-tailed, and statistical significance was set at P<0.05.
In total, 166,641 patients, including 65,856 in SCHBC, 57,346 in SCHCA, and 43,439 in SCHSU, were enrolled. The numbers of patients in each group (SCHBC, SCHCA, and SCHSU) were as follows: group A: 1,242, 974, and 656; group B: 1,373, 1,065, and 697; group C: 247, 76, and 208; group D: 247, 76, and 209; group E: 48,305, 41,595, and 30,573; group F: 1,413, 979, and 2,827; group G: 12,039, 12,358, and 7,760; group H: 990, 223, and 509.
SLPC for each patient group at the three institutions is shown in Fig. 3. In groups A and B (pre- and post-hematology disease), SCHBC and SCHSU recently showed >75% SLPC over 1,095 days. Although SCHCA recently had a section with an SLPC <75%, it has shown a trend of gradual improvement. In group C (pre-SOT), SCHSU recently showed >50% SLPC up to 1,095 days prior to transplantation. Although SCHBC showed an improving trend, the SLPC was <50% after 180 days of SOT. The SLPC of SCHCA was still low, at <25%. In group D (post-SOT), SCHBC and SCHSU recently showed >75% SLPC up to 1,095 days after transplantation, whereas SCHCA showed <50% SLPC. Group E showed <50% SLPC in all three institutions but gradually improved. In group F, SCHCA recently showed an SLPC >50%, whereas the other two institutions showed an SLPC <50%. In group G, SCHBC recently showed >75%, SCHSU approximately 75%, and SCHCA approximately 50% SPCL, which gradually improved. In group H, only SCHCA recently showed >50% SLPC, whereas SCHSU and SCHBC showed <25% SLPC.
Recently, SLPC of SCHCA was 50% lower in groups C and D and 50% higher in group H than those of SCHBC or SCHSU. In these three institutions, only kidney and liver transplantations were performed among the SOT procedures. Before and after transplantation, SLPC in the liver transplant patient group differed significantly among the three institutions at all time points, but the kidney transplant patient group did not show a significant difference at any time point (Fig. 4A–D). Group H was classified as neonates and non-neonates of 1 year. Cardiovascular surgery in neonates was performed only at SCHBC and SCHCA, showing significant differences in SLPC at all time points (Fig. 4E). As for non-neonates, the only significant difference between institutions was at 730 days after surgery (P=0.02) (Fig. 4F).
LR blood components are recommended to reduce the risk of FNHTR, transfusion-mediated CMV infection, and HLA alloimmunization [10, 11]. NLRRBC transfusion in patients subjected to SLP can be considered for reporting to the hemovigilance system as a prescription error of inappropriate blood components. However, passive surveillance is limited by underreporting [6, 12], and NLRRBC transfusion has never been reported as a prescription error in the three institutions involved this study. We adopted CDM analysis to evaluate the success rate of SLP using real-world data. The traditional method of reviewing medical records is labor-intensive and prone to human error [13]. To evaluate SLPC of several groups, including patients not yet recognized as having an indication of SLP requirement, we analyzed the medical records of 166,641 patients from three institutions over 20 years, which would be impossible without CDM analysis. Our findings confirm the applicability of CDM analysis to transfusion safety and suggest the possibility of future CDM-based distributed network research using transfusion prescription data [14, 15].
The hematology disease and solid cancer patient groups, which are expected to receive platelet transfusions, require continuous leukoreduction, unless the underlying disease is resolved. In SCHBC, in the hematology disease group, SLPC was >75% in the 3 years before and after diagnosis (Fig. 3A and B), whereas in the solid cancer group, it was <50% (Fig. 3E). In contrast, in the anticancer therapy group, including both hematological disease and solid cancer patients, SLPC was >50% (Fig. 3G). Two possibilities can be considered to explain this difference. First, because solid cancer surgery is performed by surgical staff and chemotherapy by internal medicine staff, the difference may result from a different understanding in the medical staff. Second, it is possible that there was no SLP indication because platelet transfusion was not required for patients with solid cancer.
SOT candidates should avoid transfusion but should apply for LR blood if transfusion is unavoidable [16]. As HLA antibodies are closely related to kidney transplantation prognosis [17], we expected SLPC to be higher in kidney transplant patients than in liver transplant patients. However, as shown in Fig. 4A and B, SLPC was slightly higher in the pre-liver transplant subgroup than in the pre-kidney transplant subgroup. The longer transplant waiting period and more transfusion requirements in kidney transplantation may be one reason for the difference. After the SOT period, SLPC in kidney transplant patients was lower than that in liver transplant patients (Fig. 4C and D). In SCHBC, RBC transfusion preparation before kidney transplant surgery is unusual, whereas liver transplant surgery adapted a blood preparation protocol that includes six units of pre-LRRBC, LR platelets, and fresh-frozen plasma. Recently, protocol changes have been proposed as blood transfusions in liver transplant patients have decreased; however, this protocol seems to have at least the effect of preventing NLRRBC exposure in the liver transplant group.
Since the leukoreduction indication of newborns was presented, the blood banks of the three institutions released LRRBC to all neonates <1 year of age. In the cardiovascular surgery group (Fig. 4E and F), SLPC in neonates at SCHCA and SCHBC was 100% and 75%, respectively. It is presumed that this is because the leukoreduction indication was announced as <4 months of age in 2008 and was changed to <1 year of age in 2016. However, as the CDM analysis module used in this study, ATLAS, can only distinguish patient age, it was impossible to determine whether neonates transfused with NLRRBC were 4 months to 1 year of age.
LR blood transfusion is recommended to reduce ischemia-reperfusion injury in patients who have undergone cardiopulmonary bypass and postoperative morbidity and mortality in patients with cardiovascular diseases [18]. Leukocyte reduction filters connected to the cardiopulmonary bypass circuit (arterial line filter) are widely used in cardiovascular surgery, and the leukocyte removal rate reportedly is 96.8% [19]. However, the arterial line filter did not affect the results of this study on leukocyte exposure because it was not used in the three institutions.
Although the CDM analysis used in this study is useful for reviewing large datasets, it has several limitations. First, as only KCD and EDI code data were uploaded, the available data were limited. Accordingly, the main indications for SLP, such as exothermic non-blood transfusion abnormalities, could not be analyzed because there was no KCD code. Second, medical information, such as the diagnostic records of other institutions, cannot be used in the analysis of the CDM database for each institution. If CDM databases containing national information, such as National Health Insurance or Health Insurance Review and Assessment service, are used, this limitation may be overcome [20, 21]. Third, SLPC might be determined by the attending physicians’ preferences and/or awareness of the use of LR blood. In CDM version 5.3 used in this study, provider identification information of procedures, such as transfusion, can be uploaded to the database. However, these data were not uploaded to our CDM database; therefore, differences between physicians could not be analyzed.
In summary, to protect patients from the adverse effects of allogeneic leukocytes, continuous efforts are essential to recognize the limitations of the SLP and to improve compliance. Using CDM analysis, we showed that SLPC was low even in patients who qualified for leukoreduction. Although compliance tended to improve in most groups in recent years, additional education and monitoring are needed for groups for which SLPC remains low, such as patients with solid cancer, immunodeficiency, or cardiovascular surgery.
Notes
REFERENCES
1. Ratko TA, Cummings JP, Oberman HA, Crookston KP, DeChristopher PJ, Eastlund DT, et al. 2001; Evidence‐based recommendations for the use of WBC‐reduced cellular blood components. Transfusion. 41:1310–9. DOI: 10.1046/j.1537-2995.2001.41101310.x. PMID: 11606834.


2. Bassuni WY, Blajchman MA, Al-Moshary MA. 2008; Why implement universal leukoreduction? Hematol. Oncol Stem Cell Ther. 1:106–23. DOI: 10.1016/S1658-3876(08)50042-2.


3. Choi SJ, Kim S, Kim HO, Kwon JR, Lee SW, Shin YH. 2012; The status of use of leukoreduced blood products in Korean hospitals. Lab Med Online. 2:204–8. DOI: 10.3343/lmo.2012.2.4.204.


4. Whitaker BI, Hinkins S. 2011; The 2011 national blood collection and utilization survey report. United States Department of Health and Human Services. 1–88.
5. Trial to Reduce Alloimmunization to Platelets Study Group. 1997; Leukocyte reduction and ultraviolet B irradiation of platelets to prevent alloimmunization and refractoriness to platelet transfusions. N Engl J Med. 337:1861–9. DOI: 10.1056/NEJM199712253372601. PMID: 9417523.
6. Choi S, Choi SJ, Kim JK, Nam KC, Lee S, Kim JH, et al. 2021; Preliminary feasibility assessment of CDM-based active surveillance using current status of medical device data in medical records and OMOP-CDM. Sci Rep. 11:24070. DOI: 10.1038/s41598-021-03332-6. PMID: 34911976. PMCID: PMC8674329.


7. Hripcsak G, Duke JD, Shah NH, Reich CG, Huser V, Schuemie MJ, et al. 2015; Observational Health Data Sciences and Informatics (OHDSI): opportunities for observational researchers. Stud Health Technol Inform. 216:574–8.
8. Park RW. 2019; The distributed research network, observational health data sciences and informatics, and the South Korean research network. Korean J Med. 94:309–14. DOI: 10.3904/kjm.2019.94.4.309.


9. Seong Y, You SC, Ostropolets A, Rho Y, Park J, Cho J, et al. 2021; Incorporation of Korean electronic data interchange vocabulary into observational medical outcomes partnership vocabulary. Healthc Inform Res. 27:29–38. DOI: 10.4258/hir.2021.27.1.29. PMID: 33611874. PMCID: PMC7921574.


10. Koo BN, Kwon MA, Kim SH, Kim JY, Moon YJ, Park SY, et al. 2019; Korean clinical practice guideline for perioperative red blood cell transfusion from Korean Society of Anesthesiologists. Korean J Anesthesiol. 72:91–118. DOI: 10.4097/kja.d.18.00322. PMID: 30513567. PMCID: PMC6458508.


11. National organ tissue blood management agency. 2022. Korean national transfusion guideline. 5th ed. National organ tissue blood management agency;Seoul: p. 56–8.
12. Choi S, Hyun J, Yu H, Cho D. 2021; ABO-incompatible transfusion events reported in written judgments and in the Korean hemovigilance system. Ann Lab Med. 41:493–8. DOI: 10.3343/alm.2021.41.5.493. PMID: 33824239. PMCID: PMC8041592.


13. Sarkar S, Seshadri D. 2014; Conducting record review studies in clinical practice. J Clin Diagn Res. 8:JG01–04. DOI: 10.7860/JCDR/2014/8301.4806. PMID: 25386466. PMCID: PMC4225918.


14. Song MJ, Kim S, Boo D, Park C, Yoo S, Yoon HI, et al. 2021; Comparison of proton pump inhibitors and histamine 2 receptor antagonists for stress ulcer prophylaxis in the intensive care unit. Sci Rep. 11:18467. DOI: 10.1038/s41598-021-98069-7. PMID: 34531488. PMCID: PMC8446063.


15. Josephson CD, Glynn S, Mathew S, Birch R, Bakkour S, Baumann Kreuziger L, et al. 2022; The Recipient Epidemiology and Donor Evaluation Study‐IV‐Pediatric (REDS‐IV‐P): a research program striving to improve blood donor safety and optimize transfusion outcomes across the lifespan. Transfusion. 62:982–99. DOI: 10.1111/trf.16869. PMID: 35441384.


16. Leffell MS, Kim D, Vega RM, Zachary AA, Petersen J, Hart JM, et al. 2014; Red blood cell transfusions and the risk of allosensitization in patients awaiting primary kidney transplantation. Transplantation. 97:525–33. DOI: 10.1097/01.tp.0000437435.19980.8f. PMID: 24300013.


17. Choi S, Lee KW, Park JB, Kim K, Jang HR, Huh W, et al. 2020; C3d-positive preformed DSAs tend to persist and result in a higher risk of AMR after kidney transplants. J Clin Med. 9:375. DOI: 10.3390/jcm9020375. PMID: 32019081. PMCID: PMC7073748.


18. Wallis JP, Chapman CE, Orr KE, Clark SC, Forty JR. 2002; Effect of WBC reduction of transfused RBCs on postoperative infection rates in cardiac surgery. Transfusion. 42:1127–34. DOI: 10.1046/j.1537-2995.2002.00181.x. PMID: 12430668.


19. Gu YJ, devries AJ, Boonstra PW, van Oeveren W. 1995; Clinical performance of a high-efficiency rapid flow leucocyte removal filter for leucocyte depletion of heparinized cardiopulmonary bypass perfusate. Perfusion. 10:425–30. DOI: 10.1177/026765919501000606. PMID: 8747899.


20. Kim HI, Yoon JY, Kwak MS, Cha JM. 2021; Real-world use of colonoscopy in an older population: A nationwide standard cohort study using a common data model. Dig Dis Sci. 66:2227–34. DOI: 10.1007/s10620-020-06494-x. PMID: 32691386.


21. Yoon JY, Kwak MS, Kim HI, Cha JM. 2021; Seasonal variations in the diagnosis of the top 10 cancers in Korea: a nationwide population‐based study using a common data model. J Gastroenterol Hepatol. 36:3371–80. DOI: 10.1111/jgh.15634. PMID: 34293206.


Fig. 1
Overview of the data from the three hospitals uploaded to the CDM database and examples of the data conversion process.
Abbreviations: CDM, common data model; RBC, red blood cell; SNOMED, systematized nomenclature of medicine; ICDO3, International Classification of Diseases for Oncology, 3rd edition; CPT4, current procedural terminology fourth edition; LOINC, logical observation identifier names and codes.
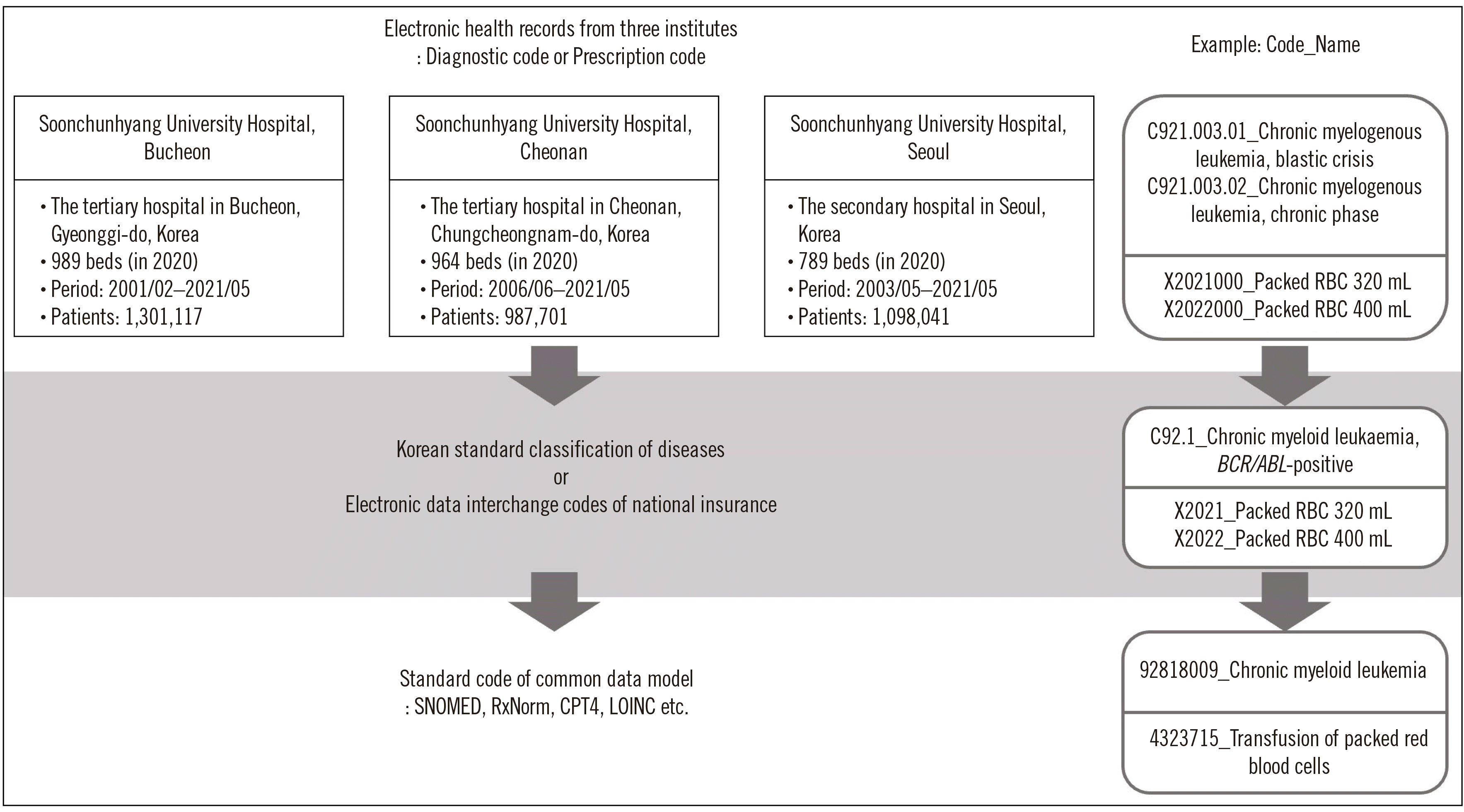
Fig. 2
Calculation process of SLPC using the CDM analysis program. (A) Setting the concept for patients group and RBC transfusion, (B) setting cohort entry event definition and inclusion criteria, (C) NRRBC and/or NLRRBC transfusion data generation using CDM, (D) NLRRBC transfusion data generation using CDM, and (E) SLPC calculation in preSOT group for a year.
Abbreviations: SLPC, selective LR protocol compliance; LR, leukoreduced; RBC, red blood cell; CDM, common data model; LRRBC, LR RBC; NLRRBC, non-LR RBC; SOT, solid organ transplantation.
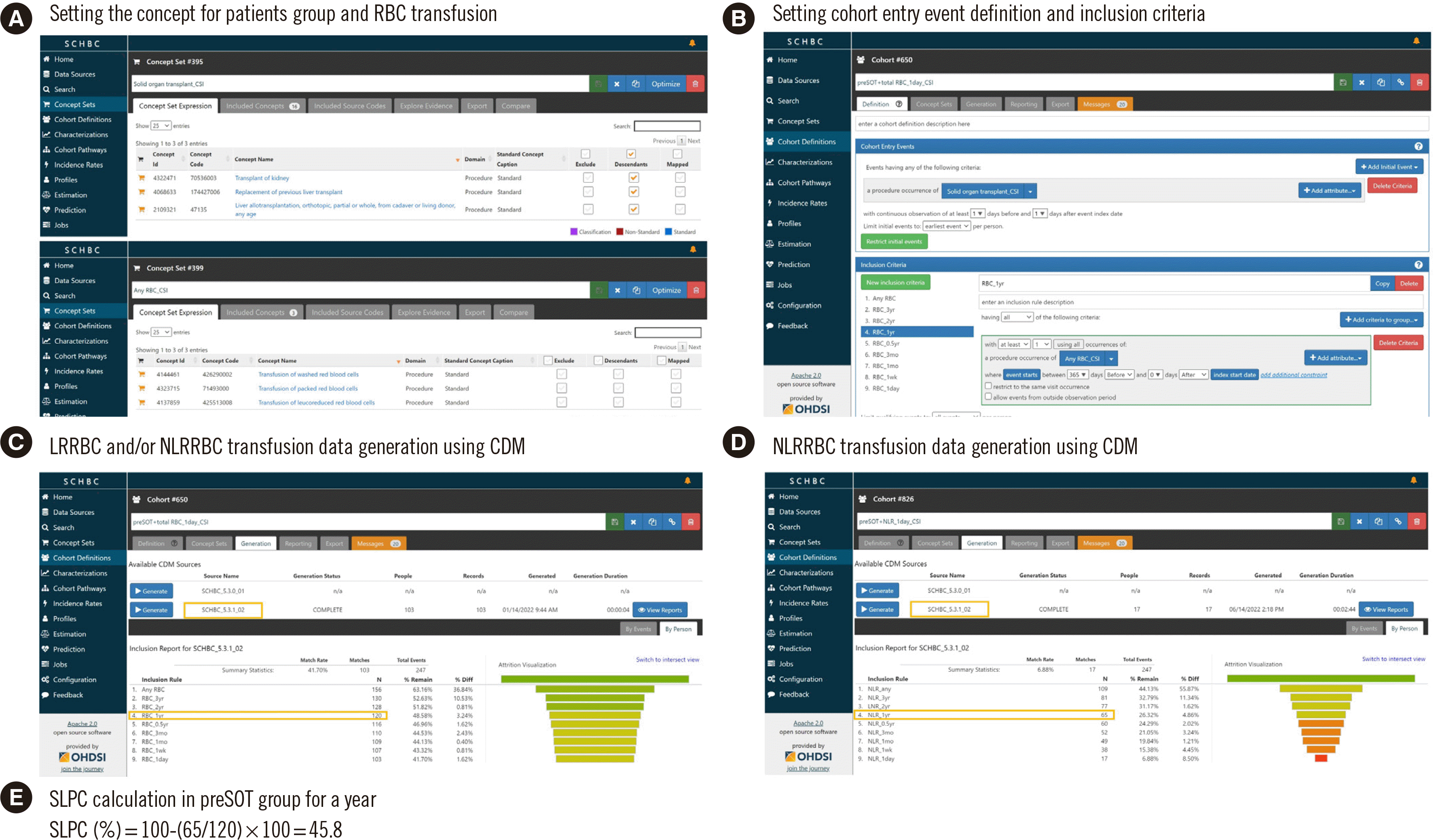
Fig. 3
Cumulative SLPC change before hematology disease diagnosis (A), after hematology disease diagnosis (B), before SOT (C), after SOT (D), after solid cancer diagnosis (E), after immunodeficiency diagnosis (F), after anticancer therapy (G), and after cardiovascular surgery (H) in patient groups exposed to NLRRBCs in SCHBC, SCHCA, and SCHSU from 2001 to 2021. SLPC was calculated as the percentage of patients who received only leukoreduced RBCs in total patients transfused RBCs.
Abbreviations: SLPC, selective LR protocol compliance; LR, leukoreduced; SOT, solid organ transplantation; NLRRBCs, non-LR red blood cells; SCHBC, Soonchunhyang University Bucheon Hospital; SCHCA, Soonchunhyang University Cheonan Hospital; SCHSU, Soonchunhyang University Seoul Hospital.
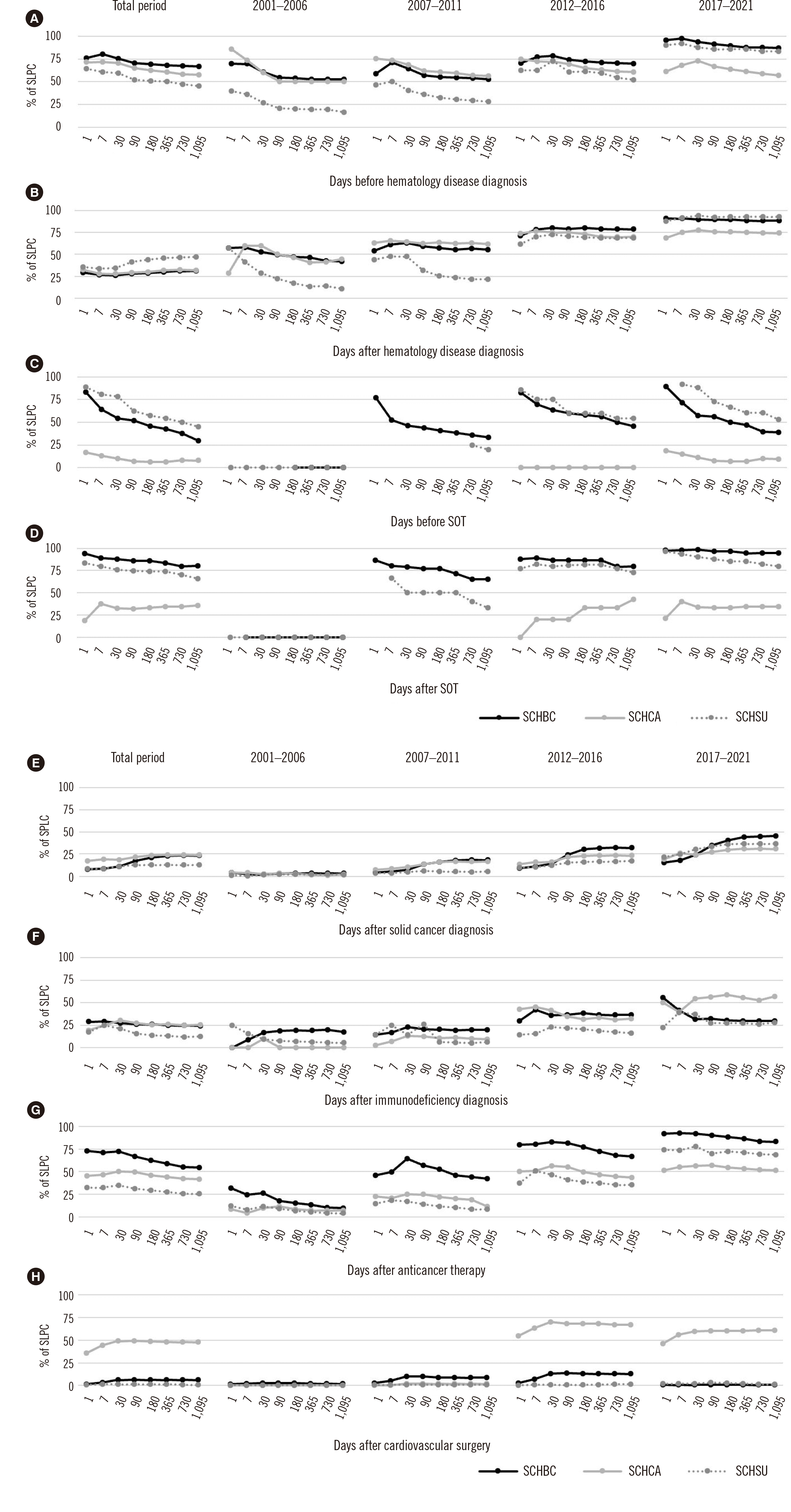
Fig. 4
Subgroup analysis of SLPC in (A) pre-liver, (B) pre-kidney, (C) post-liver, and (D) post-kidney transplantation subgroups of SOT and (E) neonate and (F) non-neonate subgroups of cardiovascular surgery.
*Cardiovascular surgery for neonates is not performed at SCHSU.
Abbreviations: SLPC, selective LR protocol compliance; SOT, solid organ transplantation; SCHBC, Soonchunhyang University Bucheon Hospital; SCHCA, Soonchunhyang University Cheonan Hospital; SCHSU, Soonchunhyang University Seoul Hospital.
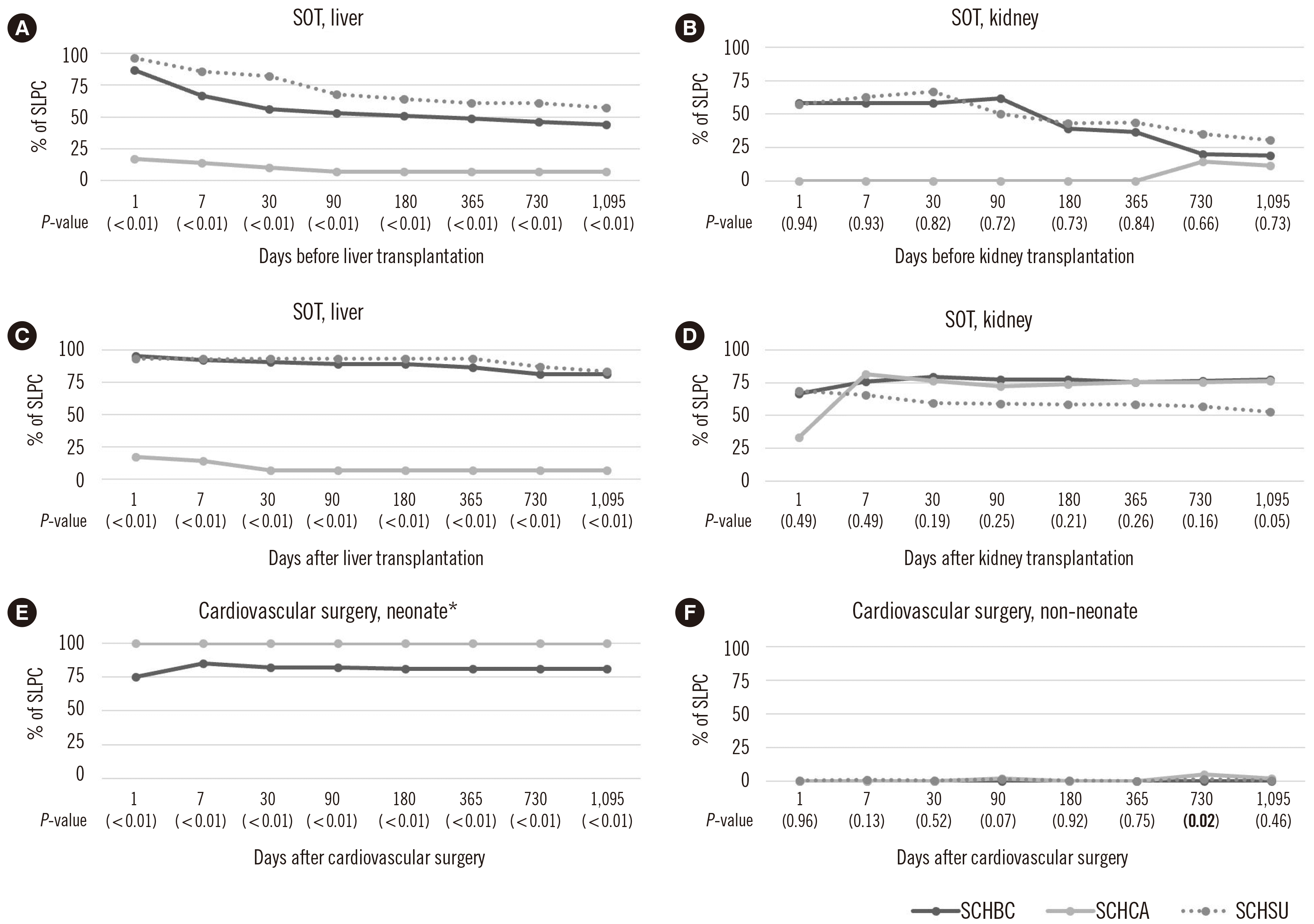
Table 1
Classification and description of medical records uploaded to the CDM database
Abbreviations: CDM, common data model; KCD, Korean Standard Classification of Diseases; SNOMED, systematized nomenclature of medicine; ICDO3, International Classification of Diseases for Oncology, 3rd edition; EDI code, electronic data interchange codes of national health insurance; CVX, vaccine administered; CPT4, current procedural terminology fourth edition; HCPCS, healthcare common procedure coding system; ICD-10-PCS, International Classification of Diseases 10th Procedure Coding System; LOINC, logical observation identifier names and codes.