Abstract
Background
Clonal hematopoiesis of indeterminate potential (CHIP), which is defined as the presence of blood cells originating from somatically mutated hematopoietic stem cells, is common among the elderly and is associated with an increased risk of hematologic malignancies. We investigated the clinical, mutational, and transcriptomic characteristics in elderly Korean individuals with CHIP mutations.
Methods
We investigated CHIP in 90 elderly individuals aged ≥60 years with normal complete blood counts at a tertiary-care hospital in Korea between June 2021 and February 2022. Clinical and laboratory data were prospectively obtained. Targeted next-generation sequencing of 49 myeloid malignancy driver genes and massively parallel RNA sequencing were performed to explore the molecular spectrum and transcriptomic characteristics of CHIP mutations.
Results
We detected 51 mutations in 10 genes in 37 (41%) of the study individuals. CHIP prevalence increased with age. CHIP mutations were observed with high prevalence in DNMT3A (26 individuals) and TET2 (eight individuals) and were also found in various other genes, including KDM6A, SMC3, TP53, BRAF, PPM1D, SRSF2, STAG1, and ZRSR2. Baseline characteristics, including age, confounding diseases, and blood cell parameters, showed no significant differences. Using mRNA sequencing, we characterized the altered gene expression profile, implicating neutrophil degranulation and innate immune system dysregulation.
Conclusions
Somatic CHIP driver mutations are common among the elderly in Korea and are detected in various genes, including DNMT3A and TET2. Our study highlights that chronic dysregulation of innate immune signaling is associated with the pathogenesis of various diseases, including hematologic malignancies.
Clonal hematopoiesis of indeterminate potential (CHIP) is characterized by a fraction of peripheral blood cells carrying somatic mutations in genes previously implicated in hematologic cancers, but without the presence of hematological diseases [1, 2]. Individuals with CHIP are at a significant risk for hematologic malignancies, cardiovascular disease, and increased mortality from non-hematological diseases [3-6].
Inflammation plays a pivotal role in the pathogenesis of CHIP caused by mutations in DNMT3A and TET2 and CHIP-associated diseases [7-9]. Macrophages and dendritic cells derived from Tet2-deficient mice exhibited aberrant production of inflammatory cytokines, such as interleukin-6, when compared with wild-type cells [8]. Mast cells from Dnmt3a-deficient mice produced high levels of interleukin-6, tumor necrosis factor α, and interleukin-13 in response to stimulation with IgE [7].
However, how CHIP mutant clones mechanistically play a role in the development and progression of the disease and their clinical implications for prognostication of disease risk are poorly understood. Furthermore, CHIP has rarely been studied in non-Caucasian populations. We investigated the clinical, mutational, and transcriptomic characteristics in elderly Korean individuals with CHIP mutations.
We included individuals aged ≥60 years with normal complete blood counts (Hb, absolute neutrophil count, and platelets) and peripheral blood cell morphology. Ninety individuals examined between June 2021 and February 2022 at the Soonchunhyang University Bucheon Hospital (a tertiary referral hospital in Bucheon, Korea) were prospectively enrolled. Peripheral blood (3–6 mL) was collected in EDTA tubes and refrigerated, and nucleic acids were extracted. Main clinical and laboratory data at the time of initial hospital visit, including age, sex, common age-related morbidities, such as hypertension and diabetes, leukocyte count, Hb level, platelet count, and mean corpuscular volume of red cells, were collected. This study was approved by the Institutional Review Board of Soonchunhyang University Bucheon Hospital (SCHBC 2021-05-009). Written informed consent was obtained from all individuals in accordance with the Declaration of Helsinki 2013.
DNA was isolated from peripheral blood leukocytes using the QIAamp DNA Mini Kit (Qiagen, Venlo, Netherlands). Targeted NGS for 49 myeloid malignancy driver genes (Supplemental Data Table S1) was performed using hybridization oligonucleotide probes on the Illumina MiSeqDx platform (Illumina, San Diego, CA, USA), producing 2×150-bp paired-end reads with a mean depth of coverage of >1,000× [10, 11]. Sequence reads were aligned against human genome build 19 (hg19) using the Burrows–Wheeler Aligner (v0.7.17, r1188). Variant calling was performed using VarScan v2.4.4 and GATK Mutect2 (v4.1.6.0), and variants were annotated using Ensembl VEP v104. After variant calling using the commercial software, all variants were manually inspected and curated based on the following criteria: ≥1% variant allele frequency (VAF) and minimum of 20 mutant reads; and in-house databases for recurrent artifacts, polymorphisms, and mutations. Variants with a low mapping quality (<20) were considered technical artifacts and excluded. Variants with a VAF of 0.4–0.6 and > 0.8 were not considered, to exclude potential germline variants. Publicly available databases, namely Catalogue of Somatic Mutations in Cancer (COSMIC) v90, ClinVar (retrieved on November 18, 2019), gnomAD (version 2.0.1), and phase 3 of the 1,000 Genome project, were used to identify rare polymorphisms, which were excluded. Our testing protocol was verified using genomic DNA samples containing different types of mutations and serially diluted 1:8-fold, 1:16-fold, and 1:32-fold with wild-type genomic DNA. The allele frequencies of mutations in genomic DNA samples diluted 1:32-fold ranged from 1.0% to 2.3%. All target mutations were detected by the targeted NGS panel at each dilution.
RNA was isolated using the QIAamp RNA Blood Mini Kit (Qiagen). mRNA libraries were prepared using an Illumina TruSeq Stranded Total RNA with Ribo-Zero Globin kit following the manufacturer’s recommendation. Sequencing was performed using the NovaSeq 6000 platform (Illumina), generating paired-end 101-bp reads. STAR (v2.5.3a) [12] was used to align the reads to hg19 and DESeq2 (v3.5e) [13] was used to find differentially expressed genes (DEGs), with default parameters. Significant DEGs were identified based on adjusted P<0.05 and a log2 fold change >1 or <–1. The list of DEGs was subjected to Gene Ontology (GO) and Protein Analysis Through Evolutionary Relationships (PANTHER) pathway analyses using the Homo sapiens reference list (20,589 genes) and the overrepresentation test (released on February 2, 2022) in PANTHER v17.0 with the default setting (http://www.pantherdb.org/; released on February 23, 2022) [14]. Reactome pathway analysis using the Reactome Pathway Database v65 (released on October 1, 2021) was used to investigate whether dysregulated genes were enriched in any particular gene category [15]. Gene set enrichment analysis (GSEA, v4.2.3) was used to verify the interpretation of gene expression data [16].
Baseline characteristics are described as median and range for continuous variables and as frequency and percentage for categorical variables. Comparisons between study subsets were made using the chi-square, Fisher’s exact, or Mann–Whitney U-test, as appropriate. The Cochran–Armitage test was used to test the CHIP mutation frequency for an age trend. For the association between CHIP and age, odds ratios with 95% confidence intervals (CIs) were analyzed using univariable logistic regression. The PANTHER overrepresentation test incorporates Fisher’s exact test with false discovery rate multiple test correction, and adjusted P<0.05 was set as the cutoff value. Statistical analyses were performed using SPSS Statistics for Windows v26.0 (SPSS, Chicago, IL, USA). Data images were generated using GraphPad Prism 9 software (GraphPad Software, San Diego, CA, USA). Two-sided P<0.05 was considered statistically significant.
The study cohort included 90 individuals with a median age of 67 years (range, 60–86 years). Sixty-six percent (59/90) of the cohort was male. Table 1 summarizes the demographic, clinical, and hematological data for the entire cohort, as well as for CHIP mutation carriers and noncarriers. Individuals with CHIP did not differ from those without CHIP in various characteristics, including age, sex, hypertension, and blood count.
A targeted NGS panel was available for all 90 individuals. CHIP was detected in 37 individuals (41%), who carried a total of 51 mutations in 10 genes (Fig. 1A). The prevalence of CHIP increased with age (Fig. 1B) (P<0.001). We detected at least one mutation in 35% (19/54) of individuals between the ages of 60–69 years, 46% (13/28) of individuals between the ages of 70–79 years, and 63% (5/8) of individuals older than 80 years. Thirteen individuals (14%) had two or more mutations, which also increased in prevalence with age (Fig. 1B) (P=0.001). The increase in CHIP prevalence with age was predominantly driven by mutated TET2 clones (odds ratio, 1.2; 95% CI, 1.09–1.45; P=0.003) (Fig. 1C). Among individuals with CHIP, 65% (24/37) had a driver mutation in only one gene and 35% (13/37) of individuals showed two or more mutations, including one individual who carried three mutations (Fig. 1D). Supplemental Data Table S2 lists all CHIP mutations detected.
The most frequently mutated genes (Fig. 1A) were DNMT3A (32 mutations, affecting 26 individuals, 29% of the cohort) and TET2 (eight mutations, affecting eight individuals, 9%). Two individuals presented mutations in both DNMT3A and TET2. In total, 32 individuals (36%) presented DNMT3A and/or TET2 mutations. Of 26 individuals with a DNMT3A mutation, five (19%) carried multiple mutations in this gene, including four with two mutations (15%, 4/26) and one with three mutations (4%, 1/26). Cohesin complex (SMC3 and STAG1, 3% of the cohort), spliceosome (SRSF2 and ZRSR2, 2%), and TP53 (2%) mutations were observed in considerably lower proportions of the cohort.
The median VAF of total CHIP mutations was 2.0% (range, 1.0%–22.2%) (Fig. 1E). The median VAFs of mutations in DNMT3A, TET2, and other genes were 2.0%, 2.5%, and 2.0%, respectively (Fig. 1F). The majority (82%, 42/51) of CHIP mutations were small mutant clones with a VAF<10%, which was the threshold used in a previous study to distinguish CHIP with small versus large mutant clones [4]. In total, 84% (27/32) of DNMT3A mutations and 75% (6/8) of TET2 mutations had a VAF<10%, and 82% (9/11) of mutations in other genes had a VAF below this threshold.
Massively parallel RNA sequencing was available for 12 individuals, including six CHIP-positive and six CHIP-negative individuals. The 31 most significant DEGs (adjusted P<0.05) are shown in a heatmap with sample-wise dendrograms by hierarchical clustering in Fig. 2. CHIP-positive samples are largely segregated from CHIP-negative samples, except for an outlier. Samples of individuals carrying DNMT3A mutation alone or in combination with TET2 mutations are also segregated from those of individuals carrying TET2 mutations alone or other gene mutations (Fig. 2).
In reactome pathway analysis, the “innate immune system” pathway was enriched in CHIP-positive samples, and its subfamily pathway, the “neutrophil degranulation” pathway, was also enriched in this cohort (Table 2 and Fig. 3). In GO enrichment analysis of cellular components, GO terms related to granules or vesicles were overrepresented among the DEGs (log2 fold change >1 or <–1 and adjusted P<0.05), whereas GO analysis of biological process or molecular function did not yield any significant results (Table 2). GSEA confirmed that the pathway of neutrophil degranulation was enriched in the CHIP-positive cohort, albeit not significantly (normalized enrichment score of 1.16 and nominal P=0.34) (Fig. 3).
We found that 41% (37/90) of the elderly Korean individuals examined had single or multiple CHIP driver mutations. Biological pathways enriched in the CHIP-positive cohort were neutrophil degranulation and innate immunity. This study was the first to estimate the CHIP mutation load and to present its transcriptomic characteristics in Koreans.
This study provided several important results. First, the most commonly mutated genes in CHIP were DNMT3A and TET2, similar to findings in previous studies [2, 4, 6]. However, we also found mutations in other genes, revealing a diverse molecular spectrum. In previous studies [2, 4, 6], CHIP mutations were commonly observed in ASXL1 and JAK2 after DNMT3A and TET2, but we found no mutations in these genes. Rather, CHIP mutations were more frequently observed in genes related to the cohesion complex (SMC3 and STAG1) and spliceosome (SRSF2 and ZRSR2), and in various other genes, such as TP53, PPM1D, and BRAF. Mutations in cohesion complex genes, such as SMC1, SMC3, RAD21, STAG1, and STAG2, lead to transcriptional dysregulation from defective chromatin accessibility and impaired differentiation of hematopoietic stem and progenitor cells [17, 18]. Additionally, recent data have revealed that cohesion plays a role in controlling the inflammatory response, which is associated with myeloid cell differentiation [17, 19]. Recurrent somatic mutations in the cohesion complex are frequent in hematologic malignancies, including myelodysplastic syndromes (11%–17%) [20] and acute myeloid leukemia (6%–13%) [21–23].
Second, our transcriptomic results support a previous report that CHIP has a dysregulation role in inflammation and immunity [24]. In our study, of the differentially upregulated genes in the CHIP-positive cohort, 23 were recognized by the PANTHER overrepresentation test (adjusted P<0.05) as being involved in pathways related to neutrophil degranulation and/or innate immunity (Table 2, Supplemental Data Table S3). However, neutrophil counts and lymphocyte counts did not significantly differ between the CHIP-positive and CHIP-negative groups (Table 1). Neutrophils are key cells in the innate immune system that mediate host defense through various effector functions, including phagocytosis and degranulation [25]. Although neutrophil granules are a major attribute of antimicrobial function, studies have suggested that excessive degranulation is associated with the pathogenesis of various inflammatory diseases, including cancer [26-28]. Individuals with a CHIP mutation have increased serum levels of certain pro-inflammatory cytokines and chemokines compared to those without mutation [29]. Altered gene expression in the innate immune system may be associated with chronic inflammatory responses and the dysregulated granulocyte maturation observed in myelodysplasia.
Of note, we lowered the threshold of CHIP mutation calling to a VAF of 1% in this study. The generally accepted threshold for CHIP variant calling is a VAF of 2% [3], but recent studies have reported on the clinical implications for CHIP of low VAFs of <2% [30, 31]. In 399 individuals with chronic heart failure, Kiefer, et al. [30] demonstrated that individuals carrying a CHIP mutation with low a VAF of 0.5%–2.0% showed significantly poorer survival than those without mutations. Another study revealed that CHIP mutations at a VAF of ≥1% were more frequently detected in elderly individuals with anemia (46.6%) than in control individuals (39.1%) [31]. In our study, 49% (25/51) of CHIP mutations were small mutant clones, with VAFs between 1% and 2%. Further studies using a large population-based cohort with long-term follow-up are required to investigate the effects and prognostic value of CHIP mutations detected in this study.
The strengths of the present study include the clinical characterization of elderly individuals with or without CHIP mutation and comprehensive genomic and transcriptomic sequencing analyses. This study was limited mainly by its small sample size, which needs to be considered when interpreting our results. Another limitation is that prognostic analysis of disease risk was not possible because of the short follow-up period. Nevertheless, our results expand on previous data from the Caucasian population and lend support to the hypothesis that CHIP mutations are associated with immune system dysfunction.
Taken together, our results demonstrate that CHIP driver mutations are common in the elderly Korean population and occur in various genes, with loss-of-function mutations being the most common in DNMT3A and TET2. Our study also indicates that the most prominent gene expression differences in individuals with and without CHIP involve neutrophil degranulation and the innate immune system.
ACKNOWLEDGEMENTS
We appreciate GC Genome and Macrogen for supporting targeted NGS panel and RNA sequencing services, respectively.
Notes
AUTHOR CONTRIBUTIONS
Jang MA and Suh J contributed to study conception and design. Moon I, Kong MG, Ji YS, Kim SH, and Park SK were involved in clinical evaluation. Jang MA conducted the genetic analysis. Jang MA, Moon I, and Kong MK drafted the manuscript. All authors have read and approved the final manuscript.
REFERENCES
1. Busque L, Patel JP, Figueroa ME, Vasanthakumar A, Provost S, Hamilou Z, et al. 2012; Recurrent somatic TET2 mutations in normal elderly individuals with clonal hematopoiesis. Nat Genet. 44:1179–81. DOI: 10.1038/ng.2413. PMID: 23001125. PMCID: PMC3483435.


2. Xie M, Lu C, Wang J, McLellan MD, Johnson KJ, Wendl MC, et al. 2014; Age-related mutations associated with clonal hematopoietic expansion and malignancies. Nat Med. 20:1472–8. DOI: 10.1038/nm.3733. PMID: 25326804. PMCID: PMC4313872.


3. Steensma DP, Bejar R, Jaiswal S, Lindsley RC, Sekeres MA, Hasserjian RP, et al. 2015; Clonal hematopoiesis of indeterminate potential and its distinction from myelodysplastic syndromes. Blood. 126:9–16. DOI: 10.1182/blood-2015-03-631747. PMID: 25931582. PMCID: PMC4624443.


4. Jaiswal S, Fontanillas P, Flannick J, Manning A, Grauman PV, Mar BG, et al. 2014; Age-related clonal hematopoiesis associated with adverse outcomes. N Engl J Med. 371:2488–98. DOI: 10.1056/NEJMoa1408617. PMID: 25426837. PMCID: PMC4306669.


5. Evans MA, Sano S, Walsh K. 2020; Cardiovascular disease, aging, and clonal hematopoiesis. Annu Rev Pathol. 15:419–38. DOI: 10.1146/annurev-pathmechdis-012419-032544. PMID: 31689371. PMCID: PMC7104598.


6. Jaiswal S. 2020; Clonal hematopoiesis and nonhematologic disorders. Blood. 136:1606–14. DOI: 10.1182/blood.2019000989. PMID: 32736379. PMCID: PMC8209629.


7. Leoni C, Montagner S, Rinaldi A, Bertoni F, Polletti S, Balestrieri C, et al. 2017; Dnmt3a restrains mast cell inflammatory responses. Proc Natl Acad Sci U S A. 114:E1490–9. DOI: 10.1073/pnas.1616420114. PMID: 28167789. PMCID: PMC5338402.
8. Zhang Q, Zhao K, Shen Q, Han Y, Gu Y, Li X, et al. 2015; Tet2 is required to resolve inflammation by recruiting Hdac2 to specifically repress IL-6. Nature. 525:389–93. DOI: 10.1038/nature15252. PMID: 26287468. PMCID: PMC4697747.


9. Jaiswal S, Ebert BL. 2019; Clonal hematopoiesis in human aging and disease. Science. 366:eaan4673. DOI: 10.1126/science.aan4673. PMID: 31672865. PMCID: PMC8050831.


10. Zhong Y, Xu F, Wu J, Schubert J, Li MM. 2021; Application of next generation sequencing in laboratory medicine. Ann Lab Med. 41:25–43. DOI: 10.3343/alm.2021.41.1.25. PMID: 32829577. PMCID: PMC7443516.


11. Park HS, Son SM, Kwon J. 2022; Serial analysis and comparison of mutation profiles in decitabine-treated myeloid sarcoma and subsequent acute myeloid leukemia using next-generation sequencing. Ann Lab Med. 42:602–5. DOI: 10.3343/alm.2022.42.5.602. PMID: 35470279. PMCID: PMC9057818.


12. Dobin A, Davis CA, Schlesinger F, Drenkow J, Zaleski C, Jha S, et al. 2013; STAR: ultrafast universal RNA-seq aligner. Bioinformatics. 29:15–21. DOI: 10.1093/bioinformatics/bts635. PMID: 23104886. PMCID: PMC3530905.


13. Love MI, Huber W, Anders S. 2014; Moderated estimation of fold change and dispersion for RNA-seq data with DESeq2. Genome Biol. 15:550. DOI: 10.1186/s13059-014-0550-8. PMID: 25516281. PMCID: PMC4302049.


14. Mi H, Ebert D, Muruganujan A, Mills C, Albou LP, Mushayamaha T, et al. 2021; PANTHER version 16: a revised family classification, tree-based classification tool, enhancer regions and extensive API. Nucleic Acids Res. 49:D394–403. DOI: 10.1093/nar/gkaa1106. PMID: 33290554. PMCID: PMC7778891.


15. Fabregat A, Sidiropoulos K, Viteri G, Marin-Garcia P, Ping P, Stein L, et al. 2018; Reactome diagram viewer: data structures and strategies to boost performance. Bioinformatics. 34:1208–14. DOI: 10.1093/bioinformatics/btx752. PMID: 29186351. PMCID: PMC6030826.


16. Subramanian A, Tamayo P, Mootha VK, Mukherjee S, Ebert BL, Gillette MA, et al. 2005; Gene set enrichment analysis: a knowledge-based approach for interpreting genome-wide expression profiles. Proc Natl Acad Sci U S A. 102:15545–50. DOI: 10.1073/pnas.0506580102. PMID: 16199517. PMCID: PMC1239896.


17. Cuartero S, Innes AJ, Merkenschlager M. 2019; Towards a better understanding of cohesin mutations in AML. Front Oncol. 9:867. DOI: 10.3389/fonc.2019.00867. PMID: 31552185. PMCID: PMC6746210.


18. Viny AD, Bowman RL, Liu Y, Lavallée VP, Eisman SE, Xiao W, et al. 2019; Cohesin members Stag1 and Stag2 display distinct roles in chromatin accessibility and topological control of HSC Self-renewal and differentiation. Cell Stem Cell. 25:682–96.e8. DOI: 10.1016/j.stem.2019.08.003. PMID: 31495782. PMCID: PMC6842438.


19. Cuartero S, Weiss FD, Dharmalingam G, Guo Y, Ing-Simmons E, Masella S, et al. 2018; Control of inducible gene expression links cohesin to hematopoietic progenitor self-renewal and differentiation. Nat Immunol. 19:932–41. DOI: 10.1038/s41590-018-0184-1. PMID: 30127433. PMCID: PMC6195188.


20. Thota S, Viny AD, Makishima H, Spitzer B, Radivoyevitch T, Przychodzen B, et al. 2014; Genetic alterations of the cohesin complex genes in myeloid malignancies. Blood. 124:1790–8. DOI: 10.1182/blood-2014-04-567057. PMID: 25006131. PMCID: PMC4162108.


21. Ley TJ, Miller C, Ding L, Raphael BJ, Mungall AJ, et al. Cancer Genome Atlas Research Network. 2013; Genomic and epigenomic landscapes of adult de novo acute myeloid leukemia. N Engl J Med. 368:2059–74. DOI: 10.1056/NEJMoa1301689. PMID: 23634996. PMCID: PMC3767041.


22. Welch JS, Ley TJ, Link DC, Miller CA, Larson DE, Koboldt DC, et al. 2012; The origin and evolution of mutations in acute myeloid leukemia. Cell. 150:264–78. DOI: 10.1016/j.cell.2012.06.023. PMID: 22817890. PMCID: PMC3407563.


23. Thol F, Bollin R, Gehlhaar M, Walter C, Dugas M, Suchanek KJ, et al. 2014; Mutations in the cohesin complex in acute myeloid leukemia: clinical and prognostic implications. Blood. 123:914–20. DOI: 10.1182/blood-2013-07-518746. PMID: 24335498.


24. Barreyro L, Chlon TM, Starczynowski DT. 2018; Chronic immune response dysregulation in MDS pathogenesis. Blood. 132:1553–60. DOI: 10.1182/blood-2018-03-784116. PMID: 30104218. PMCID: PMC6182269.


25. Mantovani A, Cassatella MA, Costantini C, Jaillon S. 2011; Neutrophils in the activation and regulation of innate and adaptive immunity. Nat Rev Immunol. 11:519–31. DOI: 10.1038/nri3024. PMID: 21785456.


26. Rawat K, Syeda S, Shrivastava A. 2021; Neutrophil-derived granule cargoes: paving the way for tumor growth and progression. Cancer Metastasis Rev. 40:221–44. DOI: 10.1007/s10555-020-09951-1. PMID: 33438104. PMCID: PMC7802614.


27. Filep JG. 2022; Targeting neutrophils for promoting the resolution of inflammation. Front Immunol. 13:866747. DOI: 10.3389/fimmu.2022.866747. PMID: 35371088. PMCID: PMC8966391.


28. Liew PX, Kubes P. 2019; The neutrophil's role during health and disease. Physiol Rev. 99:1223–48. DOI: 10.1152/physrev.00012.2018. PMID: 30758246.


29. Jaiswal S, Natarajan P, Silver AJ, Gibson CJ, Bick AG, Shvartz E, et al. 2017; Clonal hematopoiesis and risk of atherosclerotic cardiovascular disease. N Engl J Med. 377:111–21. DOI: 10.1056/NEJMoa1701719. PMID: 28636844. PMCID: PMC6717509.


30. Kiefer KC, Cremer S, Pardali E, Assmus B, Abou-El-Ardat K, Kirschbaum K, et al. 2021; Full spectrum of clonal haematopoiesis-driver mutations in chronic heart failure and their associations with mortality. ESC Heart Fail. 8:1873–84. DOI: 10.1002/ehf2.13297. PMID: 33779075. PMCID: PMC8120376.


31. van Zeventer IA, de Graaf AO, Wouters HJCM, van der Reijden BA, van der Klauw MM, de Witte T, et al. 2020; Mutational spectrum and dynamics of clonal hematopoiesis in anemia of older individuals. Blood. 135:1161–70. DOI: 10.1182/blood.2019004362. PMID: 32243522.


Fig. 1
Number and distribution of CHIP mutations in the study cohort. (A) Number of CHIP mutations identified using a targeted NGS panel of 49 myeloid malignancy driver genes. (B) The frequency of CHIP mutation increases with age. (C) Forest plot showing the odds ratio with 95% CI for the presence of CHIP mutation according to a 1-year increase in age. (D) Numbers of CHIP mutations per individual. (E) VAFs of CHIP mutations detected in the study cohort. Mutations in DNMT3A, TET2, and other genes are indicated by specific symbols. (F) Distribution of the VAF of CHIP mutations per gene. Dotted lines indicate median VAFs of 2.0%, 2.5%, and 2.0% mutations in DNMT3A, TET2, and other genes, respectively.
Abbreviations: CI, confidence interval; CHIP, clonal hematopoiesis of indeterminate potential; NGS, next-generation sequencing; VAF, variant allele frequency.
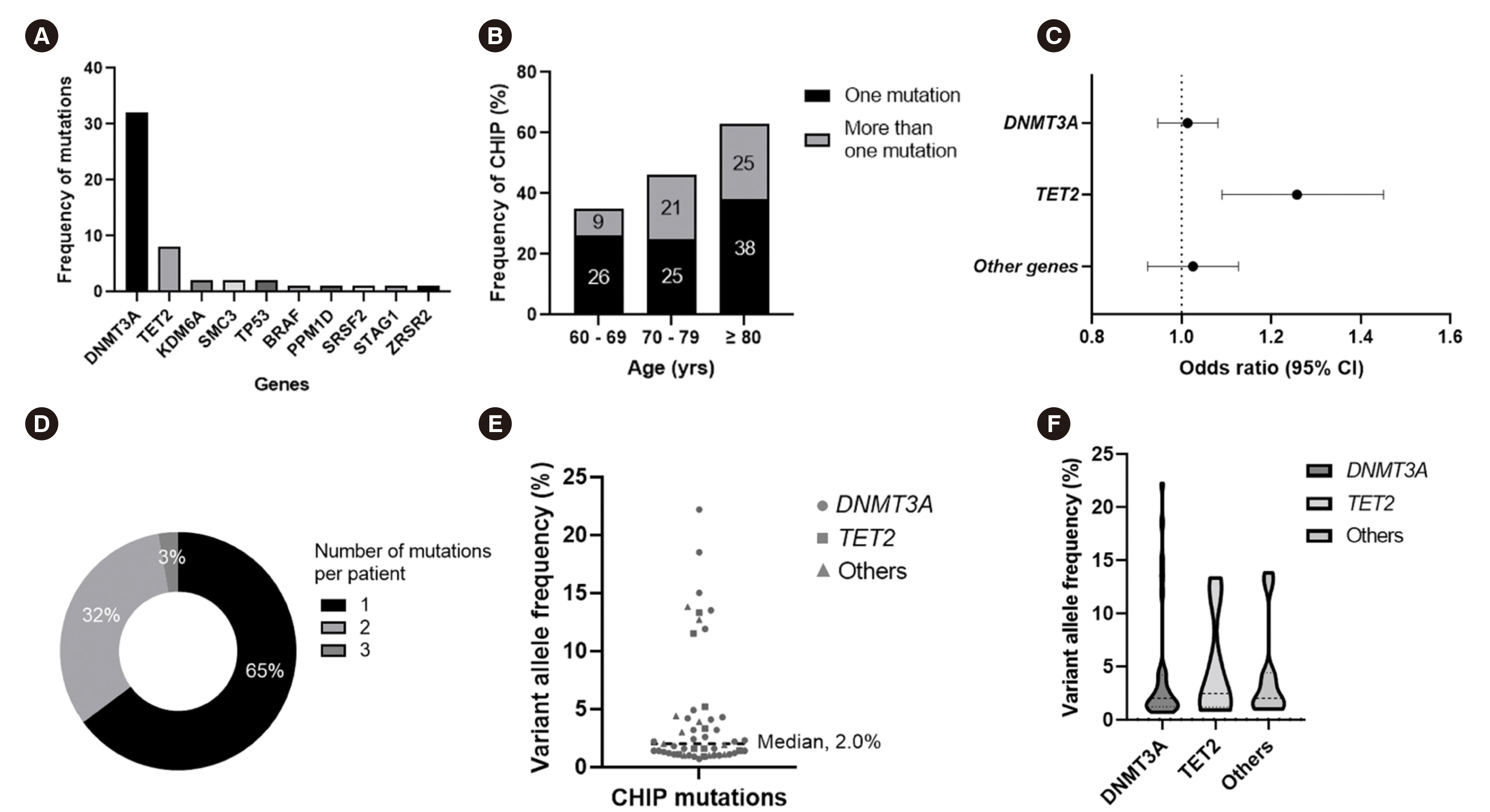
Fig. 2
Heatmap and hierarchical clustering of the 31 most significant DEGs by CHIP status. CHIP-positive samples (purple; N=6) are segregated from CHIP-negative samples (light purple; N=6), except for an outlier. All genes listed had a log2 fold change >1 or <–1 and adjusted P<0.05 in DESeq2 (v3.5e) analysis. The heatmap color range is from positive (red) to negative (blue) Z-score values.
Abbreviations: DEG, differentially expressed gene; CHIP, clonal hematopoiesis of indeterminate potential.
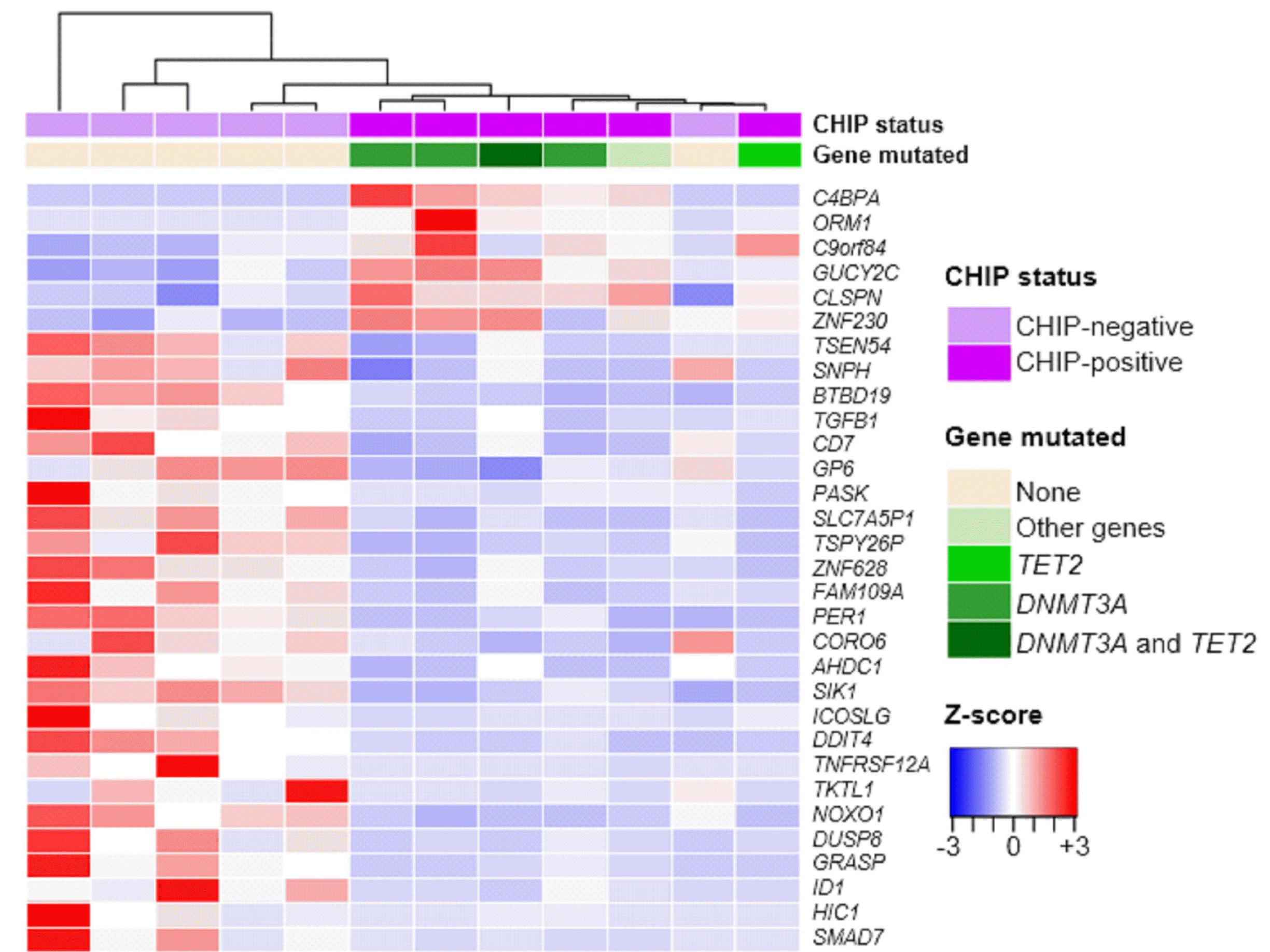
Fig. 3
Enrichment of the neutrophil degranulation pathway in CHIP-positive samples. (A) Differential expression of neutrophil/innate immunity-related genes, compared between individuals with CHIP mutations and those without. Data are presented as the median and interquartile range. (B) Upper panel: enrichment plot of the neutrophil degranulation pathway shown as a snapshot of the GSEA. Lower panel: heatmap showing the expression of genes belonging to the set with the annotation of genes ordered. *P<0.05, **P<0.01.
Abbreviations: CHIP, clonal hematopoiesis of indeterminate potential; GSEA, gene set enrichment analysis.
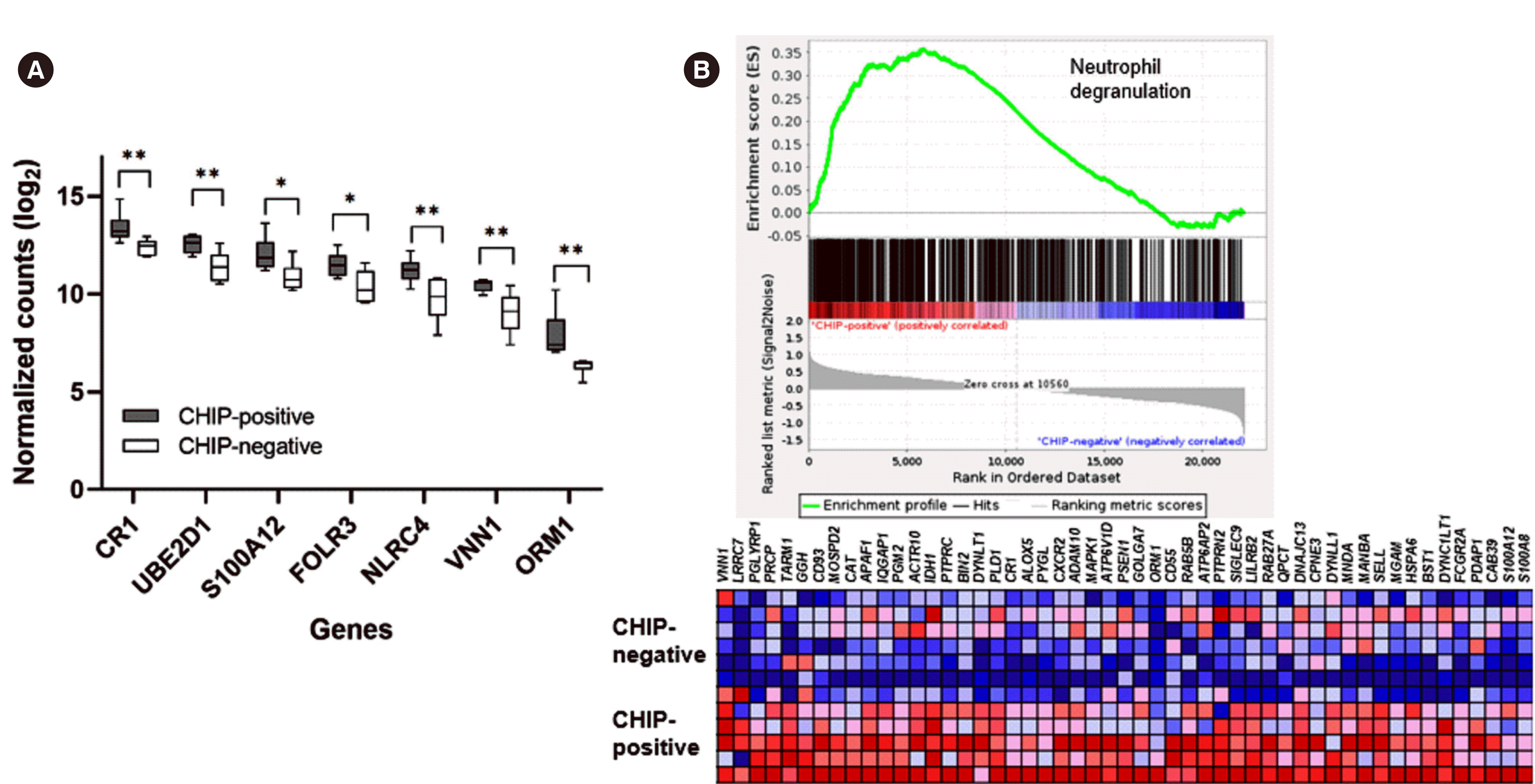
Table 1
Demographic, clinical, and hematological data according to the presence of CHIP mutations
Table 2
Results of GO and PANTHER pathway enrichment analyses in CHIP-positive individuals
Abbreviations: FDR, false discovery rate; GO, Gene Ontology; GO:CC, GO cellular component; CHIP, clonal hematopoiesis of indeterminate potential; PANTHER, Protein Analysis Through Evolutionary Relationships (http://www.pantherdb.org/).