Dear Editor,
Real-world laboratory results are generated for clinical purposes rather than for research purposes. It is not possible to conduct proper big data research by combining non-validated real-world laboratory results. Although refining standardized terms and appropriate formats is essential to build big data with real-world laboratory results, data reliability is a more critical factor.
Many laboratory tests lack standardization or harmonization, and results vary among laboratories. Even if test items are standardized or harmonized, big data results may be biased if unreliable test results are included. For example, with the well-standardized HbA1c test, 5% of laboratories participating in a European trial reported a result exceeding the quality target from the actual value [1]. The reliability of laboratory results can also change over time. Lot changes of calibrators and reagents are frequent challenges that limit a laboratory’s ability to produce consistent results over time [2]. When the HbA1c reagent lot was changed, the HbA1c result increased by 0.5% (5.5 mmol/mol) on average [3]. In the case of non-standardized or non-harmonized tests, results may vary according to different test principles, or among different instruments or reagents even within the same test principles.
It is therefore a challenge to select research-level reliable real-world laboratory results obtained for a clinical purpose for secondary use in big data analysis. We propose reanalyzing the external quality assessment (EQA) model for evaluating real-world laboratory results for big data research (Fig. 1).
The first step is to check if the laboratory tests are harmonized because the results of different institutions should not be recklessly aggregated otherwise. The “International Consortium for Harmonization of Clinical Laboratory Results” provides a resource center for global activities to harmonize and standardize clinical laboratory measurement procedures [4]. The second step is to evaluate whether the laboratory results are sufficiently reliable using the laboratory’s cumulative EQA results. EQA providers accumulate data regarding each test’s standardization or harmonization level in participants [5, 6]. Reanalyzing the EQA results of participants could be the most convenient and useful method for this purpose. For harmonized tests, if the evaluation results are within the acceptance criteria, the laboratory results could be considered sufficiently reliable for data aggregation. For non-harmonized tests, data aggregation would only be suitable within the peer group, comprising laboratories that use the same methods or analyzers from the same manufacturer [6]. We suggest basing the evaluation on the following principles and verify the detailed protocol; the results should be transparently disclosed by each EQA provider (Fig. 2).
Principle 1: Cumulative EQA data (e.g., data collected over several years) should be used for evaluation to reflect the laboratory’s reliability over time, because EQA reflects only the performance of a laboratory at a certain time point, whereas big data analysis is based on longitudinally collected data. It is preferable to include at least 24 samples to enable minimal imprecision evaluation [7].
Principle 2: Set the acceptance criteria as the total error. The total error is derived from bias and imprecision [7]. Bias can be calculated based on the target value, participants’ mean value, or the peer group mean value of the multi-year EQA according to standardization or harmonization. Imprecision could be calculated as the new protocol for the HbA1c imprecision certificate by the International Federation of Clinical Chemistry and Laboratory Medicine [8]. The acceptance criteria could be outcome-based, biological variation-based, or state-of-the art–based according to the characteristics of the test item.
Principle 3: Evaluate each test item. The performance of each test differs for each test item even in the same laboratory.
Principle 4: Evaluate periodically (e.g., annually); even in the same laboratory, instruments/reagents can change over time, or the level of quality control may vary.
Principle 5: Take a conservative approach for evaluation (i.e., evaluate strictly). If big data research is biased by including unreliable results, significant side effects (e.g., incorrect medical practices based on incorrect research results) can occur in the long run.
Two basic laboratory quality conditions should be observed as prerequisites: only an appropriate laboratory level (e.g., accredited laboratory) and only the results generated by the instruments/reagents authorized by the regulatory body should be included.
We emphasize the reliability of laboratory test results in data quality management for big data research and propose reanalyzing the EQA model for evaluating real-world laboratory results for big data research. Simply declaring an assessment of data quality in a research paper will not solve the quality issue of big data research using real-world laboratory results. We recommend certification or management of data quality at the national/organizational level. Our model based on cumulative EQA data offers a good option for evaluating medical institutions with real-world laboratory results that can be included in big data research. Since this article does not present a specific methodology, a more in-depth statistical approach is needed in the future to fully address this issue.
Notes
REFERENCES
1. EurA1c Trial Group. 2018; EurA1c: the European HbA1c trial to investigate the performance of HbA1c assays in 2166 laboratories across 17 countries and 24 manufacturers by use of the IFCC model for quality targets. Clin Chem. 64:1183–92. DOI: 10.1373/clinchem.2018.288795. PMID: 29921723.
2. Thompson S, Chesher D. 2018; Lot-to-lot variation. Clin Biochem Rev. 39:51–60.
3. Thaler MA, Iakoubov R, Bietenbeck A, Luppa PB. 2015; Clinically relevant lot-to-lot reagent difference in a commercial immunoturbidimetric assay for glycated hemoglobin A1c. Clin Biochem. 48:1167–70. DOI: 10.1016/j.clinbiochem.2015.07.018. PMID: 26187005.


4. ICHCLR. The International Consortium for Harmonization of Clinical Laboratory Results. https://www.harmonization.net. Updated on Apr 2022.
5. Jeong TD, Cho EJ, Lee K, Lee W, Yun YM, Chun S, et al. 2021; Recent trends in creatinine assays in Korea: long-term accuracy-based proficiency testing survey data by the Korean Association of External quality assessment service (2011-2019). Ann Lab Med. 41:372–9. DOI: 10.3343/alm.2021.41.4.372. PMID: 33536355. PMCID: PMC7884186.


6. Kim S, Lee K, Park HD, Lee YW, Chun S, Min WK. 2021; Schemes and performance evaluation criteria of Korean Association of External Quality Assessment (KEQAS) for improving laboratory testing. Ann Lab Med. 41:230–9. DOI: 10.3343/alm.2021.41.2.230. PMID: 33063686. PMCID: PMC7591290.


7. Weykamp C, John G, Gillery P, English E, Ji L, Lenters-Westra E, et al. 2015; Investigation of 2 models to set and evaluate quality targets for hb a1c: biological variation and sigma-metrics. Clin Chem. 61:752–9. DOI: 10.1373/clinchem.2014.235333. PMID: 25737535. PMCID: PMC4946649.


8. IFCC (International Federation of Clinical Chemistry and Laboratory Medicine). IFCC network for standardization of HbA1c. Updated on May 2022. https://ifcchba1c.org/.
Fig. 1
EQA results reanalysis model for determining test results that are sufficiently reliable for big data research. *“Harmonized” refers to whether or not the laboratory test item is harmonized based on the “Harmonization Status of Measurand” on the ICHCLR website (https://www.harmonization.net/); †A convenient and effective method of evaluation is to use cumulative data of external quality assurance for each laboratory. For the harmonized tests, if the evaluation results are within the acceptable criteria, the laboratory results could be considered to be sufficiently reliable for big data research and data aggregation. For non-harmonized tests, if the evaluation results are within acceptable criteria, the laboratory results could be sufficiently reliable for big data research and data aggregation, but only within the peer group.
Abbreviations: EQA, external quality assessment; ICHCLR, International Consortium for Harmonization of Clinical Laboratory Results.
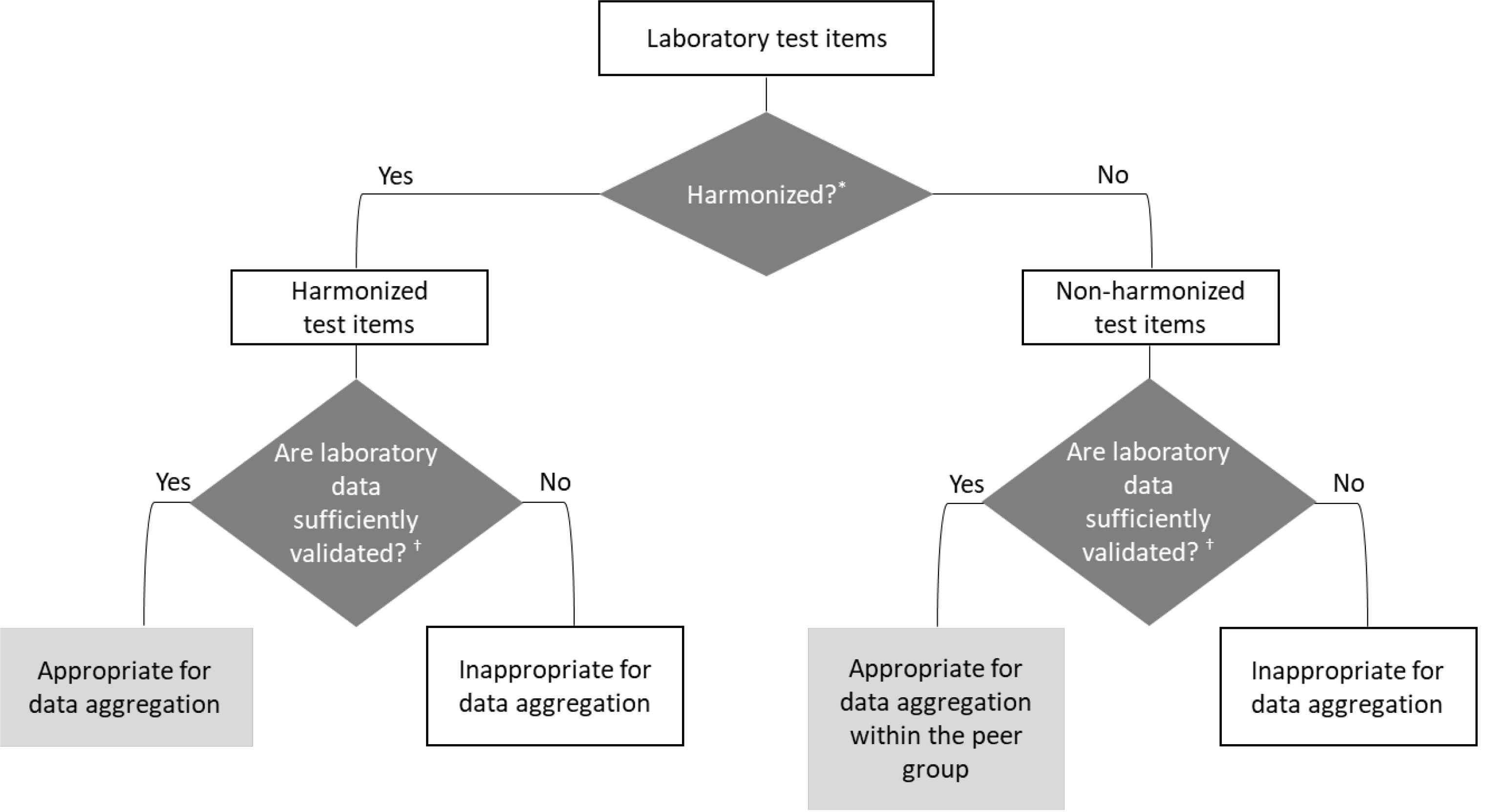