Abstract
Purpose
The illness-death model (IDM) is a comprehensive approach to evaluate the relationship between relapse and death. This study aimed to illustrate the value of the IDM for identifying risk factors and evaluating predictive probabilities for relapse and death in patients with non–small cell lung cancer (NSCLC) in comparison with the disease-free survival (DFS) model.
Materials and Methods
We retrospectively analyzed 612 NSCLC patients who underwent a curative operation. Using the IDM, the risk factors and predictive probabilities for relapse, death without relapse, and death after relapse were simultaneously evaluated and compared to those obtained from a DFS model.
Results
The IDM provided more detailed risk factors according to the patient’s disease course, including relapse, death without relapse, and death after relapse, in patients with resected lung cancer. In the IDM, history of malignancy (other than lung cancer) was related to relapse and smoking history was associated with death without relapse; both were indistinguishable in the DFS model. In addition, the IDM was able to evaluate the predictive probability and risk factors for death after relapse; this information could not be obtained from the DFS model.
Lung cancer is one of the most common cancers in the world, and its mortality of 25% is the highest among all cancers [1]. Non–small cell lung cancer (NSCLC) accounts for approximately 85% of lung cancer cases and surgery is the mainstay treatment for patients with early-stage NSCLC [2]. Surgery is generally performed in patients with stage I to IIIA NSCLC, when the tumor is resectable in patients who are suitable for surgery [3]. The 5-year overall survival (OS) rate for NSCLC is approximately 50% for resected lung cancers [1] and approximately 30%–55% of patients with resected NSCLC eventually show relapse during follow-up [4]. There are several risk factors affecting OS or relapse-free survival (RFS) in patients with resected NSCLC. Tumor-related factors, including tumor-node-metastasis (TNM) staging, cell types, and host-related factors (i.e., sex, age, smoking history) are predictive of OS in patients with NSCLC [5]. In addition, TNM staging, tumor markers, and performance status are reported as significant factors in predicting RFS after surgical resection in patients with NSCLC [5].
In patients who have undergone NSCLC surgery, events such as relapse or metastasis are called intermediate events because they are not fatal, whereas death is called a terminal event. Studies have typically focused on whether or not a patient survives without experiencing relapse or death when analyzing cancer-related survival data. This concept is called disease-free survival (DFS). In DFS studies, the intermediate event and terminal event are considered to have a competing relationship, survival time is defined as the time of occurrence of the first of the two events, and censoring is defined as whether either of the two events has occurred or not. On the other hand, the illness-death model (IDM) [6–8] considers the intermediate event and terminal event to have a semi-competing relationship, so it has the advantage of observing the survival time from the occurrence of the intermediate event to the occurrence of the terminal event, which cannot be observed in DFS.
The purpose of this study was to identify risk factors affecting relapse and death via the IDM among NSCLC patients and evaluate personalized predictive probabilities of disease outcomes to help in establishing follow-up plans or treatment strategies.
A total of 917 patients who underwent curative surgery for primary lung cancer from 2010 to 2018 were initially included in this study. Exclusion criteria were as follows: (1) sublobar resection, (2) the presence of synchronous or metachronous lung cancer, (3) an unknown origin of cancer recurrence in patients with double primary cancer, (4) the presence of distant metastases, (5) other than adenocarcinoma or squamous cell carcinoma, and (6) pre-invasive cancers. A total of 612 consecutive patients with resected lung adenocarcinoma or squamous cell carcinoma were ultimately included in the study (Fig. 1).
Patient demographics, history of malignancy other than lung cancer, family history of lung cancer, smoking history (never smokers vs. previous or current smokers), cancer location (upper lobes, including the middle lobe vs. lower lobes), cancer type (adenocarcinoma vs. squamous cell carcinoma), TNM stage, operation method (lobectomy vs. bi-lobectomy or pneumonectomy), treatment method, and the presence of relapse were recorded from electronic medical records and radiology reports. TNM stage was determined according to the eighth edition of the American Joint Commission on Cancer staging system for lung cancer [9]. The T category was determined based on pathologic reports following lobectomy, and the N category was determined by lymph node dissection or endobronchial ultrasound-guided biopsy.
Time to relapse was defined as the time from the date of operation to the date of the first recorded evidence of intra-thoracic or distant metastasis as confirmed by imaging or histology. Loss to follow-up was defined as a case where imaging follow-up was not performed after December 2018 (1 year before the end of the study). The time of censoring was determined as the date of the last imaging evaluation. Date of death and cause of death data were obtained via linkage to the Korean Statistical Information Service.
For analyses of the DFS model and the IDM, proportional hazard models were used to examine the associations of covariates (age, sex, smoking history, family history of lung cancer, history of other malignancies, nodule location, operation method, cancer type, treatment method, interaction of cancer types and treatment methods, TNM stage) with relapse or death. For the analysis of DFS, the primary endpoint was defined as the time of relapse or death. The IDM was fitted to estimate the intensities in transitions from surgery to relapse (0→1), from surgery to death (0→2), and from relapse to death (1→2), as illustrated in Fig. 2. These three transitions could occur at any time until the end of follow-up. The most widely used R package for analyzing DFS models is survival, but this package can only handle right-censored data. Therefore, the icensReg [10] R package was used instead of survival because the event of interest, such as relapse, was interval censored in the NSCLC data. In addition, the most widely used R package to analyze multi-state models is msm, but this package can only analyze right-censored data with intermediate events. Therefore, the SmoothHazard [11] R package was used to deal with interval-censored intermediate events.
In both the DFS and ID models, interval censoring was considered as the assessment of relapse was not continuous; the exact date of relapse could have occurred in the interval between two consecutive clinical examinations. As for baseline transition intensities, a Weibull distribution was assumed in both the DFS and ID models. Multivariable analyses in both the DFS and ID models were conducted to select the most parsimonious model that would reduce overfitting. Risk factors included in initial multivariable analyses were selected based on a p-value < 0.2 in TNM-adjusted univariable analyses because TNM stage is considered a primary risk factor. The Akaike information criterion (AIC) was used to decide on final models (i.e., models with the smallest AIC value), thus indicating the best model through a backward elimination strategy.
The IDM can discern four different predictive probabilities for patients who underwent surgery, while the DFS model includes only two predictions. For the former, the probabilities of being alive after relapse, being dead after relapse, being dead without relapse, and being alive without relapse are predictable at each elapsed time since surgery; for the latter, we calculated the probabilities of being relapsed or dead and being alive without experiencing any event. As an illustration, we examined a patient with the following risk factors: age, 65 years; cancer type, adenocarcinoma; sex, male; treatment method, operation only; history of malignancy other than lung cancer, no; smoking history, previous or current smoker; nodule location, upper lobes, and middle lobes; and operation method, lobectomy. Those values correspond to the sample median or mode of each risk factor. We estimated the predictive probabilities of survivorship and relapse by TNM stage (1A, 2A, or 3A) in order to investigate characteristics within each stage and to compare changes in trends according to TNM stage.
Among the 612 included patients with resected lung adeno-carcinoma or squamous cell carcinoma, 69.3% (424/612) were men (mean age±standard deviation, 66.3±9.6 years) and 30.7% (188/612) were women (mean age±standard deviation, 65.1±8.9 years). Sixteen percent (99/612) had a history of malignancy other than lung cancer, 4% (26/612) had a family history of lung cancer, and 56% (345/612) were previous or current smokers. Forty percent (246/612) of the lung tumors were located in the lower lobes. With respect to TNM staging, 48% (294/612) of cases were 1A, 12.3% (75/612) were 1B, 5.9% (36/612) were 2A, 15.8% (97/612) were 2B, 14.4% (88/612) were 3A, 3.4% (21/612) were 3B, and 0.2% (1/612) were 3C. A combined TNM stage (3B and 3C) was used in the analysis because there was only one patient with stage 3C cancer. Sixty-two percent (382/612) of the patients had adenocarcinoma, and 38% (230/612) had squamous cell carcinoma. Fifty-three percent (323/612) underwent surgery without additional treatment modalities, and 47% (289/612) received chemotherapy or radiation therapy in addition to surgery. With respect to relapse, 51 of the 612 patients (8.3%) were lost to follow-up, 68% (415/612) did not experience relapse, and 24% did experience relapse (146/612). Seventy percent (426/612) were alive, 25% (155/612) of the included patients died from lung cancer, and 5% (31/612) died from causes other than lung cancer during the course of the study. Detailed results are described in Table 1.
In univariable analyses of the DFS and ID models adjusted for TNM stage, the p-values of all variables except family history of lung cancer were < 0.20. Those variables were considered for multivariable analyses. S1 Table shows the detailed results of univariable analyses.
The DFS model accounting for age, smoking history, history of other malignancies, operation method, TNM stage, cancer type, treatment method, and the interaction of cancer type and treatment method had the lowest AIC value (AIC, 980.4) (Table 2).
The IDM (AIC, 1,868.3) included age, smoking history, history of other malignancies, nodule location, operation method, TNM stage, cancer type, treatment method, and the interaction of cancer type and treatment method (Table 3). Pneumonectomy against lobectomy had a statistically significantly higher risk within all three transitions (relapse, 0→1: hazard ratio [HR], 1.97; p=0.007; death without relapse, 0→2: HR, 2.58; p=0.009; death following relapse, 1→2: HR, 1.84; p=0.039). Risks for relapse and death without relapse increased substantially with an increase in TNM stage. Smoking history was a statistically significant predictor of death without relapse, with an HR of 4.05 (p < 0.001), though there was no statistically significant association with relapse or death after relapse. History of other malignancies was observed as a statistically significant predictor of relapse, though not of other transitions. There was a statistically significant interaction effect between cancer type and treatment method for relapse (p < 0.001) and death without relapse (p < 0.001), as in the multivariable DFS analysis (p < 0.001). For death following relapse, adenocarcinoma showed substantially less risk compared to squamous cell carcinoma (HR, 0.52; p=0.006). Both the DFS and ID models indicated that family history of lung cancer and sex were not statistically significant predictors of disease outcomes. The IDM revealed a statistically significant relative risk of nodule location on disease outcomes that was not observed in the DFS model.
Fig. 3 displays predictive probabilities by TNM stage, as estimated from both the ID and DFS models. For the IDM, the height of the sum of the two bottom regions represents the probability of relapse, and the sum of the two middle regions represents the probability of death. Moreover, the height of the sum of the colored regions in the IDM corresponds to the probability of death or relapse in the DFS model.
As expected, a patient diagnosed with a TNM stage of 1A had the lowest estimated probability of being alive after relapse (5-year probability, 5.1%) as well as of death after relapse (2.7%) and death without relapse (5.5%). Probabilities were highest for patients with a TNM stage of 3A (with 5-year probabilities of 14.4%, 9.1%, and 42.8%, respectively). Moreover, the sum of three 5-year probabilities in the IDM gave similar results for the probability of death or relapse as compared to the DFS model (1A, 13.3 vs. 14.2%; 2A, 32.1 vs. 38.5%; 3A, 66.3 vs. 67.8%).
In our study, we demonstrated that it is possible to evaluate detailed predictive probabilities of disease outcomes via the IDM in NSCLC patients who received surgical resection. The main reason for this finding is that the IDM evaluates the occurrences of relapse and death simultaneously as well as in conjunction with interactions between these outcomes. As compared to the DFS model, the IDM can identify statistically significant risk factors for disease outcomes according to the patient’s disease course, including relapse, death without relapse, and death after relapse. To our knowledge, this is the first study to apply the IDM for an epidemiological investigation of lung cancer. Our findings facilitate an understanding of multi-state processes in the post-surgery follow-up period for lung cancer patients.
The IDM proposed in our study showed several strengths as compared to the DFS model. First, history of malignancy other than lung cancer (HR, 1.44; p=0.049) and smoking history (HR, 1.68; p=0.001) were statistically significant risk factors related to decreased DFS; the IDM demonstrated that a history of malignancy other than lung cancer was related to relapse (HR, 1.67; p=0.023) and smoking history was related to death without relapse (HR, 4.05; p < 0.001). Second, the location of the lung tumor was not a statistically significant risk factor in the DFS model; however, lower lobe location was positively associated with death without relapse (HR, 1.97; p=0.011) and negatively associated with relapse (HR, 0.63; p=0.009) according to the IDM. This finding suggests that the DFS model offsets these effects. Third, we observed a statistically significant interaction between cancer type and treatment methods in both the DFS and the IDM. However, the IDM could additionally differentiate the magnitude of interaction between cancer type and treatment methods in death without relapse from death after relapse.
Various events can occur in the disease course of a patient who has undergone surgery for NSCLC. Usually, researchers perform separate analyses for each event setting as a primary endpoint, considering the other endpoints as censoring. For example, studies of OS aim to estimate the probability of death by considering relapse as censoring. Conversely, evaluating RFS aims to estimate the probability of relapse by considering death as censoring. However, those separate analyses do not provide completely satisfying results due to a failure to reveal associations between relapse and death [12,13]. In contrast, the IDM simultaneously deals with endpoints focusing on transitioning from one state to another. Moreover, the IDM provides predictions of patient clinical prognoses at specific points in their relapse or death process. Several medical articles have estimated event-related predictive probabilities via the IDM in patients with breast cancer [12,14,15], ovarian cancer [16], or colon cancer [13]. However, to our knowledge, the IDM has not been implemented in patients with lung cancer.
As mentioned above, the IDM allowed us to explore the effects of risk factors on relapse leading to death. Regarding the NSCLC patients who experienced relapse in our study, we found that pneumonectomy and squamous cell carcinoma cancer subtype were unfavorably associated with death after relapse. Sekihara et al. [17] reported that female gender, adenocarcinoma histology, and absence of distant metastasis were favorably associated with post-recurrence survival (PRS), and Shimada et al. [18] revealed that adenocarcinoma showed a favorable PRS; however, an unfavorable PRS was observed among patients with pneumonectomy as well as adjuvant therapy. Our study identified risk factors from the IDM that were similar to previous results obtained with the PRS model, though these two models have different starting points. Specifically, the PRS model examines survival probability only among patients who experienced relapse, and the survival rate is calculated from the time of relapse [19]. In contrast, the IDM evaluates the probability of death after relapse starting from the time of lung cancer surgery. We believe that the IDM shows a more comprehensive view of risk factors for mortality as well as other patient outcomes experienced during lung cancer follow-up.
As shown in Fig. 3, the predictive probability of relapse or death obtained from the DFS model can be decomposed into three parts in the IDM (i.e., the predictive probabilities of being alive after relapse and of having died with or without relapse). This decomposition enables us to investigate the evolving process of events such as relapse and death [20–22]. For example, for a patient diagnosed with lung cancer with a TNM stage of 3A, there is a 67.8% probability of cancer relapse or death within five years of a lung cancer operation based on the results of the DFS model. We report the following separate five-year probabilities for survival after relapse, death after relapse, and death without relapse via the IDM: 14.4%, 9.1%, and 42.8%, respectively. Moreover, the sum of these probabilities corresponds to the predictive probability of relapse (23.5%) and the two mortality probabilities (51.9%) within 5 years following the operation.
Our study has several limitations. First, this was a retrospective single-center study and our results were evaluated within a small number of included patients. Our primary focus was to introduce the IDM and to compare it with the typical DFS model among patients with surgically resected NSCLC. As a further step, implementing the IDM within a larger multi-institutional cohort will help us to better under-stand the association between relapse and death among NSCLC patients. Second, our analysis was limited to patients with resected adenocarcinoma and squamous cell carcinoma, which are two of the most common histologic NSCLC types. Further studies including other cancer subtypes and advanced-stage lung cancers are warranted to gain a comprehensive understanding of this issue. Third, loss to follow-up was defined as a case where imaging follow-up was not performed as of a year before the end of the study, and patients who died of causes other than lung cancer were regarded as being censored at the time of death. Fourth, because the follow-up interval is longer in patients undergoing surgery at an earlier stage, there is a possibility that the date of relapse may not be accurate for early-stage patients, which had a total follow-up period of 8 years. Fifth, although treatment methods change before and after relapse in lung cancer patients, we assumed models in which the effects of treatment methods do not change during follow-up. However, if there are risk factors that may show altered effects before and after relapse, such as treatment methods, a model that considers the effects of these risk factors as time-varying would be more appropriate.
In conclusion, our results demonstrate the IDM can be used as a complementary tool to simultaneously evaluate the predictive probabilities of relapse and death during the post-operation follow-up of NSCLC patients. Furthermore, the IDM may help in establishing follow-up plans or treatment strategies according to individual risk prediction. We believe that the IDM provides a more comprehensive picture of risk factors as compared to the DFS model and may facilitate an improved understanding of the multi-state disease processes occurring among lung cancer patients.
Electronic Supplementary Material
Supplementary materials are available at Cancer Research and Treatment website (https://www.e-crt.org).
Notes
Ethical Statement
This retrospective preliminary study was approved (approval number: CUH-2020-11-004) by the institutional review board of the Chonbuk National University Hospital (Jeonju, Korea). The requirement for informed consent was waived due to the retrospective nature of this study. This research was conducted in accordance with the principles of the Declaration of Helsinki and its later amendments.
Acknowledgments
This research was supported by Basic Science Research Program through the National Research Foundation of Korea (NRF) funded by the Ministry of Education (NRF-2020R1F1A1A01048397).
References
1. Siegel RL, Miller KD, Fuchs HE, Jemal A. Cancer statistics, 2021. CA Cancer J Clin. 2021; 71:7–33.
2. Mitsudomi T, Suda K, Yatabe Y. Surgery for NSCLC in the era of personalized medicine. Nat Rev Clin Oncol. 2013; 10:235–44.
3. Zappa C, Mousa SA. Non-small cell lung cancer: current treatment and future advances. Transl Lung Cancer Res. 2016; 5:288–300.
4. Uramoto H, Tanaka F. Recurrence after surgery in patients with NSCLC. Transl Lung Cancer Res. 2014; 3:242–9.
5. Brundage MD, Davies D, Mackillop WJ. Prognostic factors in non-small cell lung cancer: a decade of progress. Chest. 2002; 122:1037–57.
6. Fine JP, Jiang H, Chappell R. On semi-competing risks data. Biometrika. 2001; 88:907–19.
7. Wang W. Estimating the association parameter for Copula models under dependent censoring. J R Stat Soc Series B Stat Methodol. 2003; 65:257–73.
8. Xu J, Kalbfleisch JD, Tai B. Statistical analysis of illness-death processes and semicompeting risks data. Biometrics. 2010; 66:716–25.
9. Travis WD, Asamura H, Bankier AA, Beasley MB, Detterbeck F, Flieder DB, et al. The IASLC Lung Cancer Staging Project: proposals for coding T categories for subsolid nodules and assessment of tumor size in part-solid tumors in the forthcoming eighth edition of the TNM classification of lung cancer. J Thorac Oncol. 2016; 11:1204–23.
10. Anderson-Bergman C. icenReg: regression models for interval censored data in R. J Stat Softw. 2017; 81:1–23.
11. Touraine C, Gerds TA, Joly P. SmoothHazard: an R package for fitting regression models to interval-censored observations of illness-death models. J Stat Softw. 2017; 79:1–22.
12. Meier-Hirmer C, Schumacher M. Multi-state model for studying an intermediate event using time-dependent covariates: application to breast cancer. BMC Med Res Methodol. 2013; 13:80.
13. Alafchi B, Roshanaei G, Tapak L, Abbasi M, Mahjub H. Joint modelling of colorectal cancer recurrence and death after resection using multi-state model with cured fraction. Sci Rep. 2021; 11:1016.
14. Putter H, van der Hage J, de Bock GH, Elgalta R, van de Velde CJ. Estimation and prediction in a multi-state model for breast cancer. Biom J. 2006; 48:366–80.
15. Hajihosseini M, Faradmal J, Sadighi-Pashaki A. Survival analysis of breast cancer patients after surgery with an intermediate event: application of illness-death model. Iran J Public Health. 2015; 44:1677–84.
16. Eulenburg C, Mahner S, Woelber L, Wegscheider K. A systematic model specification procedure for an illness-death model without recovery. PLoS One. 2015; 10:e0123489.
17. Sekihara K, Hishida T, Yoshida J, Oki T, Omori T, Katsumata S, et al. Long-term survival outcome after postoperative recurrence of non-small-cell lung cancer: who is ‘cured’ from postoperative recurrence? Eur J Cardiothorac Surg. 2017; 52:522–8.
18. Shimada Y, Saji H, Yoshida K, Kakihana M, Honda H, Nomura M, et al. Prognostic factors and the significance of treatment after recurrence in completely resected stage I non-small cell lung cancer. Chest. 2013; 143:1626–34.
19. Choi PJ, Jeong SS, Yoon SS. Prediction and prognostic factors of post-recurrence survival in recurred patients with early-stage NSCLC who underwent complete resection. J Thorac Dis. 2016; 8:152–60.
20. Keiding N, Klein JP, Horowitz MM. Multi-state models and outcome prediction in bone marrow transplantation. Stat Med. 2001; 20:1871–85.
21. Touraine C, Helmer C, Joly P. Predictions in an illness-death model. Stat Methods Med Res. 2016; 25:1452–70.
22. Andersen PK, Pohar Perme M. Inference for outcome probabilities in multi-state models. Lifetime Data Anal. 2008; 14:405–31.
Fig. 2
Comparison of disease-free survival and illness-death models and their progression paths and counts for lung cancer patients.
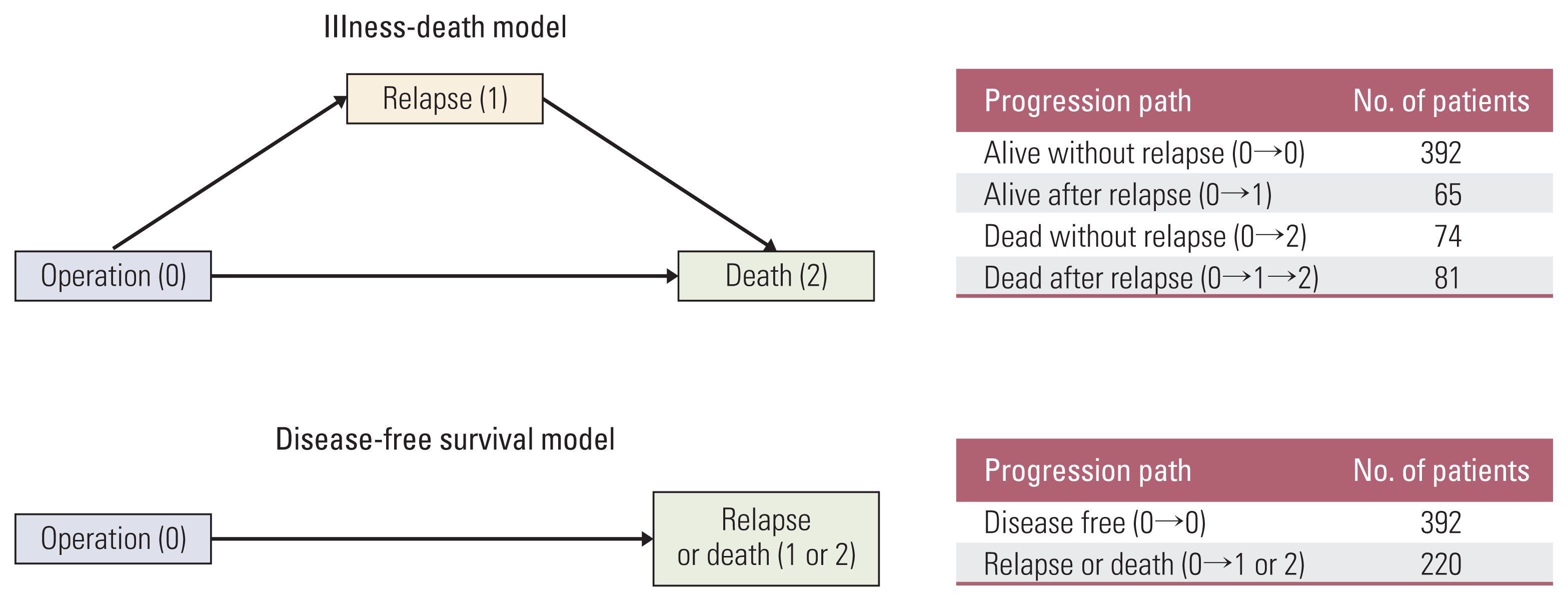
Fig. 3
Predictive probabilities for an illustrative patienta) with the sample median or mode for each risk factor presented according to TNM stage (1A [A, B], 2A [C, D], 3A [E, F]), as estimated from the illness-death model (left) and the disease-free survival model (right). a)This patient’s risk factors were set to age=65, cancer type=adenocarcinoma, sex=male, treatment method=operation only, history of malignancy other than lung cancer=no, smoking history=previous or current smoker, nodule location=upper lobes and middle lobes, and operation method=lobectomy.
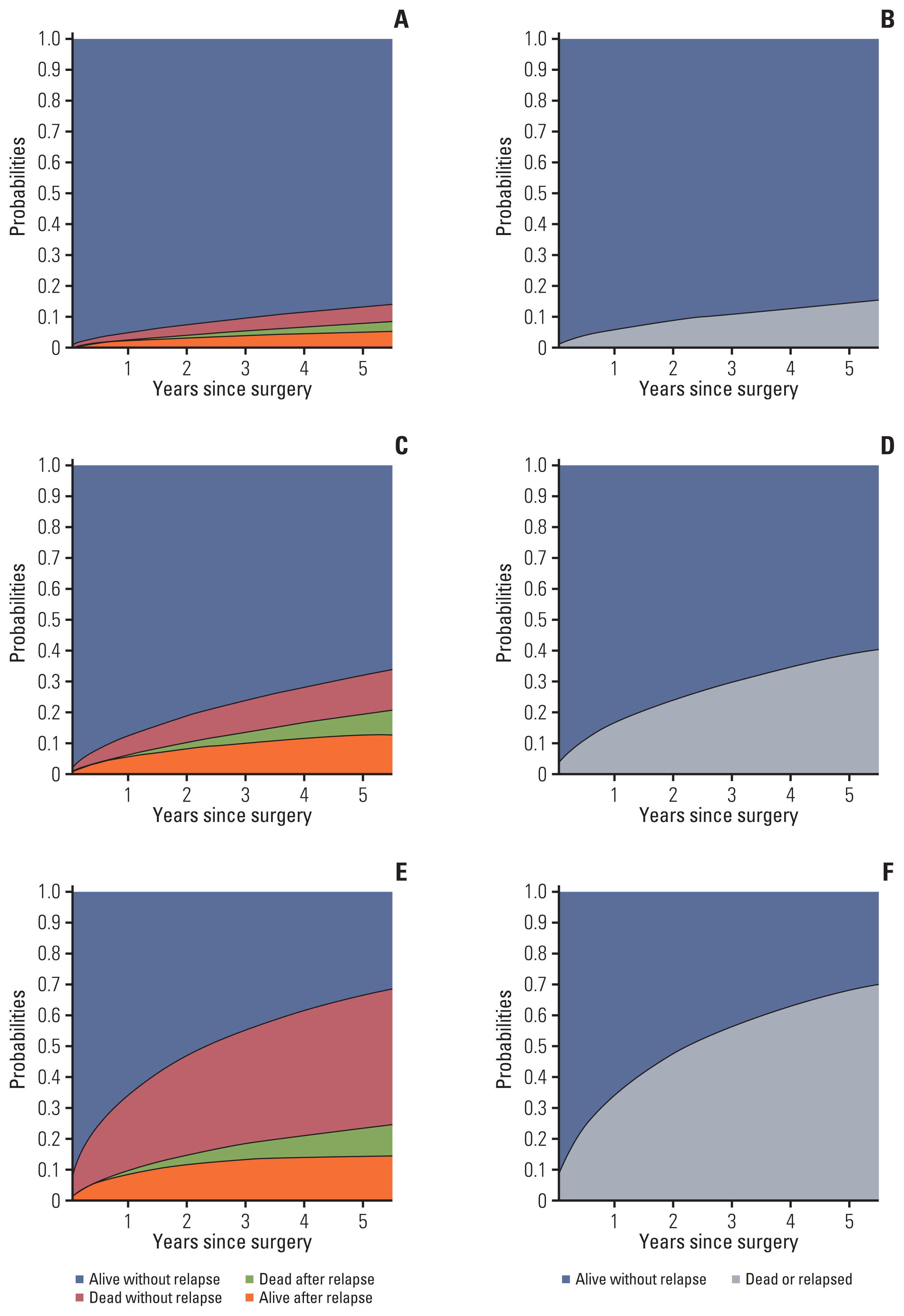
Table 1
Patient demographic and medical characteristics
Variable | Included patients (n=612) |
---|---|
Sex | |
Female | 188 (30.7) |
Male | 424 (69.3) |
Age (yr) 66.0±9.4 | |
History of malignancy other than lung cancer | |
No | 513 (83.8) |
Yes | 99 (16.2) |
Family history of lung cancer | |
No | 586 (95.8) |
Yes | 26 (4.2) |
Smoking history | |
Never smoker | 267 (43.6) |
Former or current smoker | 345 (56.4) |
Nodule location | |
Upper and middle lobes | 366 (59.8) |
Lower lobes | 246 (40.2) |
TNM stagea) | |
1A | 294 (48.0) |
1B | 75 (12.3) |
2A | 36 (5.9) |
2B | 97 (15.8) |
3A | 88 (14.4) |
3B | 21 (3.4) |
3C | 1 (0.2) |
Pathologic diagnosis | |
Squamous cell carcinoma | 230 (37.6) |
Adenocarcinoma | 382 (62.4) |
Operation method | |
Lobectomy | 559 (91.3) |
Bilobectomy or pneumonectomy | 53 (8.7) |
Treatment | |
Operation only | 323 (52.8) |
Adjuvant chemo- or radiation therapy | 289 (47.2) |
Relapse | |
No | 415 (67.8) |
Yes | 146 (23.9) |
Loss to follow-up | 51 (8.3) |
Death | |
Alive | 426 (69.6) |
Death (lung cancer) | 155 (25.3) |
Death (with a cause other than lung cancer) | 31 (5.1)b) |
Table 2
Multivariable analysis for the disease-free survival model based on the Akaike information criterion in the patients with lung cancer
Table 3
Multivariable analysis for the illness-death model based on the Akaike information criterion in patients with lung cancer
Variable | Relapse | Death without relapse | Death after relapse | |||
---|---|---|---|---|---|---|
|
|
|
||||
HR (95% CI) | p-value | HR (95% CI) | p-value | HR (95% CI) | p-value | |
Age (yr) | 1.02 (1.00–1.04) | 0.115 | 1.07 (1.03–1.11) | < 0.001 | - | - |
|
||||||
Former or current smoker | - | - | 4.05 (1.95–8.39) | < 0.001 | - | - |
|
||||||
History of malignancy other than lung cancer | 1.67 (1.07–2.6) | 0.023 | - | - | - | - |
|
||||||
Location at a lower lobe | 0.63 (0.44–0.89) | 0.009 | 1.97 (1.17–3.32) | 0.011 | - | - |
|
||||||
Pneumonectomy (reference: lobectomy) | 1.97 (1.20–3.23) | 0.007 | 2.58 (1.26–5.28) | 0.009 | 1.84 (1.03–3.28) | 0.039 |
|
||||||
TNM stage (reference: 1A) | ||||||
|
||||||
1B | 1.19 (0.58–2.42) | 0.632 | 2.42 (1.1–5.32) | 0.027 | - | - |
|
||||||
2A | 2.98 (1.32–6.73) | 0.009 | 2.30 (0.71–7.44) | 0.164 | - | - |
|
||||||
2B | 3.55 (1.80–7.01) | < 0.001 | 3.16 (1.38–7.23) | 0.007 | - | - |
|
||||||
3A | 5.26 (2.61–10.59) | < 0.001 | 11.20 (4.61–27.21) | < 0.001 | - | - |
|
||||||
3B or 3C | 12.06 (5.35–27.22) | < 0.001 | 16.35 (5.47–48.91) | < 0.001 | - | - |
|
||||||
Interaction of cancer type and treatment (reference: SqCC and operation only) | ||||||
|
||||||
SqCC and adjuvant therapy | 0.62 (0.29–1.32) | 0.214 | 0.10 (0.05–0.23) | < 0.001 | - | - |
|
||||||
ADC and operation only | 0.44 (0.21–0.92) | 0.029 | 0.44 (0.22–0.89) | 0.022 | 0.52 (0.33–0.83)a) | 0.006 |
|
||||||
ADC and adjuvant therapy | 1.65 (0.83–3.28) | 0.157 | 0.17 (0.07–0.40) | < 0.001 |