Abstract
Background
Glycated hemoglobin (HbA1c), which reflects the patient's blood sugar level, can only be measured in a hospital setting. Therefore, we developed a model predicting HbA1c using personal information and self-monitoring of blood glucose (SMBG) data solely obtained by a patient.
Methods
Leave-one-out cross-validation (LOOCV) was performed at two university hospitals. After measuring the baseline HbA1c level before SMBG (Pre_HbA1c), the SMBG was recorded over a 3-month period. Based on these data, an HbA1c prediction model was developed, and the actual HbA1c value was measured after 3 months. The HbA1c values of the prediction model and actual HbA1c values were compared. Personal information was used in addition to SMBG data to develop the HbA1c predictive model.
Results
Thirty model training sessions and evaluations were conducted using LOOCV. The average mean absolute error of the 30 models was 0.659 (range, 0.005–2.654). Pre_HbA1c had the greatest influence on HbA1c prediction after 3 months, followed by post-breakfast blood glucose level, oral hypoglycemic agent use, fasting glucose level, height, and weight, while insulin use had a limited effect on HbA1c values.
Several challenges are encountered when assessing the status of patients with diabetes from a long-term perspective based only on their self-monitoring of blood glucose (SMBG). Therefore, a method for checking the patient's blood sugar management status by measuring the glycated hemoglobin (HbA1c) is currently in use.1)2) HbA1c reflects the average glycemic control over the previous 2–3 months,3) which can be easily used for blood glucose measurement in patients, as well as for the screening, diagnosis, and treatment of diabetes.4)5) Notably, HbA1c has shown a strong correlation with possible diabetic complications, such as cerebrocardiovascular diseases.6)
While SMBG can be performed at home, it may be inconvenient for patients to visit a hospital regularly and have their HbA1c levels measured. Therefore, a method for estimating the HbA1c value using SMBG at home would be convenient. Moreover, if the patient's HbA1c level is predictable, they will be able to set their own blood sugar goals and focus better on self-management.
Various HbA1c prediction models using SMBG exist7-9); therefore, we aimed to create a model that predicts HbA1c in real-life situations using other variables in combination with the SMBG data. The actual HbA1c levels measured in the hospital and the predicted HbA1c values were compared. Furthermore, we evaluated the importance of factors affecting the prediction of HbA1c. The ability to identify the factors affecting HbA1c prediction in advance will be of great help to patients in self-glycemic management.
This study included patients with type 2 diabetes who visited Korea University Ansan Hospital and Soonchunhyang University Seoul Hospital between April 1 and August 31, 2020. We included patients aged 40–80 years who had visited the hospital for at least 2 years. Patients who agreed to participate in the study and fulfilled the selection criteria were included. In contrast, those with type 1 diabetes, an estimated glomerular filtration rate <30, kidney transplant, and ongoing dialysis were excluded from this study. A basic physical examination was performed for all included patients, and they were asked to record their blood sugar levels in a diary at home for a period of 3 months. This study was approved by the Institutional Review Boards of Korea University Ansan Hospital and Soonchunhyang University Seoul Hospital (IRB No. 2019AS0226).
The variables of the HbA1c predictive model included demographic and SMBG data. Basic information, including sex, height, weight, and body mass index (BMI) of the patient at the first visit, HbA1c before SMBG (Pre_HbA1c), and insulin use were investigated. The SMBG data comprised blood glucose records from the diary maintained by the patient for 3 months, after which, the HbA1c (Post_HbA1c) was rechecked. The patient's SMBG values comprised the average values of fasting, post-breakfast, post-lunch, and post-dinner blood sugar.
In this study, the eXtreme Gradient Boosting (XGBoost)10) was used for the development of the HbA1c predictive model. LOOCV, a useful method for estimating the performance of a small dataset, was used to train and evaluate the model. LOOCV used only one of the 30 data sets as the “test set” and the remaining 29 data sets as the “training set.” Thus, the process of training and testing the model was repeated 30 times. The mean absolute error (MAE) was used as the model test indicator. The Shapley value was used to measure the feature contribution to the model prediction.11)12)
The mean age of the patients was 66.2±8.0 years, and 63.3% (19/30) were male (Table 2). The mean BMI was 26±2.8 kg/m2, Pre_HbA1c was 7.3%±1.1%, and Post_HbA1c was 7.2%±1.0% (Table 1). Moreover, 43.3% (13/30) of the patients were taking oral hypoglycemic agents (OHA) and 56.7% (17/30) were taking insulin. The mean fasting blood glucose level was 123±17 mg/dL, whereas the mean blood glucose values post-breakfast, -lunch, and -dinner were 177±44 mg/dL, 179±60 mg/dL, and 170±58 mg/dL, respectively. The average number of fasting blood glucose measurements conducted over the 3-month period was 59.4±37.4, while the average number of blood glucose measurements conducted post-breakfast, -lunch, and -dinner over the 3-month period was 25.7±32.8, 20.8±35.5, and 30.2±37.8, respectively.
In this study, 30 model training and evaluations were conducted using LOOCV (Table 2); the average MAE of the 30 models was 0.659 (range, 0.005–2.654). We visualized and compared the predicted HbA1c values of the XGBoost models and the true HbA1c values (Figure 1). If the model's predicted value and true value match, the data were located on the green dotted line. The solid blue line represents the “line of best fit” for the point, showing that the slope is close to the green dotted line.
Among the 30 model predictions, the 10 variables that contributed the most to the predicted HbA1c values were selected (Figure 2). Pre_HbA1c had the greatest effect on HbA1c prediction after 3 months, followed by the post-breakfast blood glucose level, OHA use, fasting glucose level, height, and weight, while insulin use had a limited effect on the HbA1c prediction.
In this study, a model was developed to predict the HbA1c value using the patient's SMBG data. Moreover, the factors affecting it were identified. By providing this information to the patient, it is possible to predict the HbA1c value without the need for frequent hospital visits to undergo a separate test. Thus, this study provides a convenient method for the SMBG in patients by enabling easy prediction of HbA1c values.
A model that enables the prediction of HbA1c using SMBG data recorded in the patients' homes was developed. The SMBG data were divided into four types: fasting glucose, postbreakfast, post-lunch, and post-dinner glucose levels. Predictive models depend on how much SMBG data must be obtained to produce relevant results16)17) and the limit of missing data allowed; this is because it is inconvenient for patients to measure all of the required blood glucose values daily.18) Moreover, in this study, the proportion of missing data was 20.0–46.7%, even though the average value of each postprandial glucose value was used. Therefore, we used XGBoost for the HbA1c prediction model.10)19) XGBoost not only shows excellent performance in standardized data classification and prediction problems, but also permits cross-validation, and the missing values can be handled by themselves. Although a significant amount of postprandial glucose values were missing in this study, it was possible to proceed with the study using XGBoost without separate imputation.
Our study included 30 patients, which is a small number for creating a predictive model. Moreover, the LOOCV method used in this study requires a long time to develop the model because it depended on the amount of data.13-15)20) Hence, LOOCV is often used to measure the performance of a relatively small data sample.13-15) LOOCV uses one of the N datasets as the “test set” and the remaining N-1 data sets as the “training set”21); this process is repeated N times. The advantage of LOOCV is that since all samples are tested once, randomization is inexistent, and unlike the validation set approach, it is possible to obtain very reliable results. Furthermore, because only one sample was used as a “test set,” it was possible to create a model using a large amount of training data. However, it is difficult to include model diversity in LOOCV. Although, considering that our study is a pilot study concept, the use of LOOCV seemed appropriate.
Various models have been developed for predicting HbA1c.7-9) One such model predicted HbA1c based on the lifestyle, clinical, and biochemical information obtained at a health checkup center,7) while another predicted HbA1c after 6 years using various laboratory findings.8) Recently, given the diversity in the HbA1c prediction models, various laboratory findings are commonly used rather than simple SMBG data.9) Therefore, in this study, SMBG and simple personal information were included in the prediction model. Additionally, the pre-HbA1c value was added to reflect the patient's past self-glycemic control status.
The results of this study showed that the Pre_HbA1c value had the greatest influence on the HbA1c prediction model (Figure 2). Theoretically, Pre_HbA1c and Post_HbA1c are independent variables that do not affect HbA1c prediction. Although careful interpretation can be conducted in various ways, the most important reason for using SMBG data obtained from a patient's diary is that it is recorded in real time.17)22) Pre_HbA1c reflects the patient's past blood sugar management pattern and status. Although only the patient's average blood glucose value for 3 months can affect the prediction of HbA1c, management pattern and habits cannot be changed easily, which is thought to influence HbA1c prediction. This is considered a relevant finding. The prediction of the HbA1c value was only affected by fasting blood glucose or postprandial blood glucose levels; however, Pre_HbA1c demonstrated a rather significant effect, which may be related to the missing blood glucose values of the patients. This finding suggests that the Pre_HbA1c value compensates for the missing values; therefore, Pre_HbA1c was presumed to be the most powerful predictor of HbA1c in this study.
However, there are certain limitations in applying the results of this study. When patients make strong decisions about their blood sugar management and change their diet/exercise management rapidly, there is a high possibility that the predicted HbA1c value may be inaccurate.
Various HbA1c prediction models are continuously being developed,7-9) but researchers who wish to develop a prediction model in the future will have to consider several factors. For instance, it is necessary to consider whether to include personal information, such as Pre_HbA1c, as a variable rather than SMBG data only. Ultimately, the answer depends on the purpose of the study,16) and it seems that a broad definition of predicted HbA1c is required.
If the main purpose of developing a prediction model for HbA1c is to improve the patient's blood glucose level or if the amount of SMBG data is sufficient, it would be more appropriate to use simple SMBG data. However, if there are few SMBG data or no significant difference occurred in the patients' willingness to manage blood sugar, it would be better to include data on personal information in addition to simple SMBG data in the predictive model. The fact that Pre_HbA1c had a significant effect in this predictive model suggests that there was almost no change in the pattern of the patient's blood glucose management. Given the retrospective nature of the cohort study, which can only estimate correlation and not causation,16)17) we can assume that the study was conducted with patients exhibiting limited changes in blood glucose patterns.
Among the other factors influencing HbA1c prediction, the influence of post-breakfast blood glucose and fasting blood glucose was high in this study. This is theoretically consistent with the results of the original HbA1c prediction model, wherein SMBG correlated with the predicted HbA1c to some extent.23)24) The influence of each postprandial glucose level on the prediction of HbA1c should be studied using large samples in the future. Furthermore, OHA use had a much greater influence than insulin use. For the latter, it is presumed that the predicted HbA1c value was affected by blood glucose changes as the insulin dose was gradually adjusted.
This pilot study was conducted with a small sample size; therefore, several limitations may have occurred. Considering that Pre_HbA1c is included as a variable, it is necessary to thoroughly evaluate the interpretation, which is different from the actual result of the patient. The results of this study may contribute to the development of various predictive models in the future, although it is difficult to generalize the research results. Hence, future studies should include larger samples and more variables.
Despite its limitations, the results of this study showed that self-management could be facilitated by allowing the patient to check their HbA1c level without visiting a hospital. By enabling easy prediction of HbA1c, early recognition of the degree of blood sugar control and blood sugar management status can be achieved, which would help patients in managing their blood sugar levels voluntarily and actively. Thus, we look forward to the creation of more diverse and sophisticated predictive models, and that more studies will be conducted to help patients manage their blood glucose levels. The existing results are insufficient, and it will be necessary to develop a model with high potential for practical use in the future by securing a large sample and more sophisticated methods of analysis.
Notes
REFERENCES
1. Sacks DB, Bruns DE, Goldstein DE, Maclaren NK, McDonald JM, Parrott M. Guidelines and recommendations for laboratory analysis in the diagnosis and management of diabetes mellitus. Clin Chem. 2002; 48:436–72.


2. American Diabetes Association. Standards of medical care in diabetes. Diabetes Care. 2004; 27 Suppl 1:S15–35.
3. Nathan DM, Kuenen J, Borg R, Zheng H, Schoenfeld D, Heine RJ; A1c-Derived Average Glucose Study Group. Translating the A1C assay into estimated average glucose values. Diabetes Care. 2008; 31:1473–8.


4. Nathan DM, Genuth S, Lachin J, Cleary P, Crofford O, Davis M, Rand L, Siebert C; Diabetes Control and Complications Trial Research Group. The effect of intensive treatment of diabetes on the development and progression of long-term complications in insulin-dependent diabetes mellitus. N Engl J Med. 1993; 329:977–86.


5. UK Prospective Diabetes Study (UKPDS) Group. Intensive blood-glucose control with sulphonylureas or insulin compared with conventional treatment and risk of complications in patients with type 2 diabetes (UKPDS 33). Lancet. 1998; 352:837–53.
6. Singer DE, Nathan DM, Anderson KM, Wilson PW, Evans JC. Association of HbA1c with prevalent cardiovascular disease in the original cohort of the Framingham Heart Study. Diabetes. 1992; 41:202–8.


7. Chien KL, Lin HJ, Lee BC, Hsu HC, Chen MF. Prediction model for high glycated hemoglobin concentration among ethnic Chinese in Taiwan. Cardiovasc Diabetol. 2010; 9:59.


8. Huang CL, Iqbal U, Nguyen PA, Chen ZF, Clinciu DL, Hsu YH, Hsu CH, Jian WS. Using hemoglobin A1C as a predicting model for time interval from pre-diabetes progressing to diabetes. PLoS One. 2014; 9:e104263.


9. Rauh SP, Heymans MW, Koopman AD, Nijpels G, Stehouwer CD, Thorand B, Rathmann W, Meisinger C, Peters A, de Las Heras Gala T, Glümer C, Pedersen O, Cederberg H, Kuusisto J, Laakso M, Pearson ER, Franks PW, Rutters F, Dekker JM. Predicting glycated hemoglobin levels in the non-diabetic general population: Development and validation of the DIRECT-DETECT prediction model - a DIRECT study. PLoS One. 2017; 12:e0171816.


10. Tianqi C, Guestrin C. Xgboost: a scalable tree boosting system. In : Proceedings of the 22nd ACM SIGKDD International Conference on Knowledge Discovery and Data Mining; 2016 Aug 13-17; San Francisco, CA. New York, NY. Association for Computing Machinery. 2016. p. 785–94.
11. Lundberg SM, Lee SI. A unified approach to interpreting model predictions. In : Proceedings of the 31st International Conference on Neural Information Processing Systems; 2017 Dec 4-9; Long Beach, CA. Red Hook, NY. Curran Associates Inc.2017. p. 4768–77.
12. Lundberg SM, Erion G, Chen H, DeGrave A, Prutkin JM, Nair B, Katz R, Himmelfarb J, Bansal N, Lee SI. From local explanations to global understanding with explainable AI for trees. Nat Mach Intell. 2020; 2:56–67.


13. Shavitt I, Segal E. Regularization learning networks: deep learning for tabular datasets. In : Proceedings of the 32nd International Conference on Neural Information Processing Systems 2018; 2018 Dec 3-8; Montréal, Canada. Red Hook, NY. Curran Associates Inc.2018. p. 1386–96.
14. Abou Omar KB. XGBoost and LGBM for Porto Seguro's Kaggle challenge: a comparison semester project [Internet]. Zürich: ETH Zurich;2018. [cited 2021 Sep 10]. Available from: https://pub.tik.ee.ethz.ch/students/2017-HS/SA-2017-98.pdf.
15. Cai H, Zhong R, Wang C, Zhou R, Zhou K, Lee H, Xu K, Gao Z, Zhong R, Luo J, Zhou Y, Ding M, Li L, Li Q, Li D, Jiang N, Cheng X, Cui S, Ye H, Shen J. KDD CUP 2017 travel time prediction [Internet]. KDD;2017. [cited 2021 Sep 10]. Available from: https://www.kdd.org/kdd2017/files/Task1_3rdPlace.pdf.
16. Kim HS, Kim DJ, Yoon KH. Medical big data is not yet available: why we need realism rather than exaggeration. Endocrinol Metab (Seoul). 2019; 34:349–54.


17. Kim HS, Kim JH. Proceed with caution when using real world data and real world evidence. J Korean Med Sci. 2019; 34:e28.


18. Hu ZD, Zhang KP, Huang Y, Zhu S. Compliance to self-monitoring of blood glucose among patients with type 2 diabetes mellitus and its influential factors: a real-world cross-sectional study based on the Tencent TDF-I blood glucose monitoring platform. mHealth. 2017; 3:25.


19. Friedman JH. Greedy function approximation: a gradient boosting machine. Ann Stat. 2001; 29:1189–232.


20. Yelin I, Snitser O, Novich G, Katz R, Tal O, Parizade M, Chodick G, Koren G, Shalev V, Kishony R. Personal clinical history predicts antibiotic resistance of urinary tract infections. Nat Med. 2019; 25:1143–52.


21. DeCoste D, Wagstaff K. Alpha seeding for support vector machines. In : Proceedings of the Sixth ACM SIGKDD International Conference on Knowledge Discovery and Data Mining; 2000 Aug 20-23; Boston, MA. New York, NY. Association for Computing Machinery. 2000. p. 345–9.
22. Kim HS, Lee S, Kim JH. Real-world evidence versus randomized controlled trial: clinical research based on electronic medical records. J Korean Med Sci. 2018; 33:e213.


Figure 1.
Plot of the predicted and observational glycated hemoglobin data. Solid blue line represents the “line of best fit” for the point.
HbA1c = glycated hemoglobin.
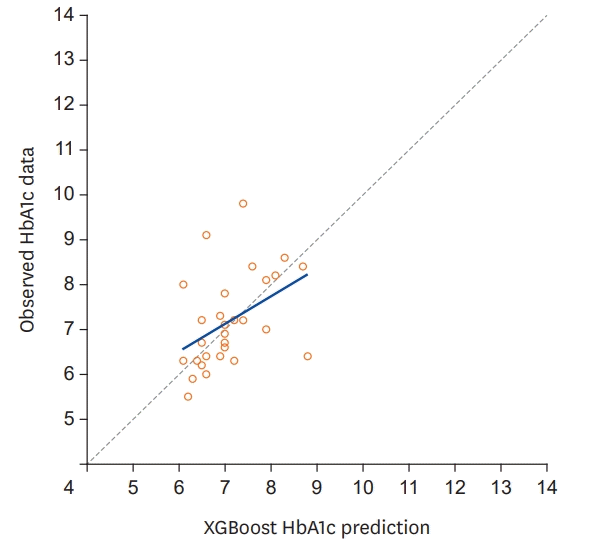
Figure 2.
The contributors to model predictions.
BMI = body mass index; HbA1c = glycated hemoglobin; OHA = oral hypoglycemic agents.
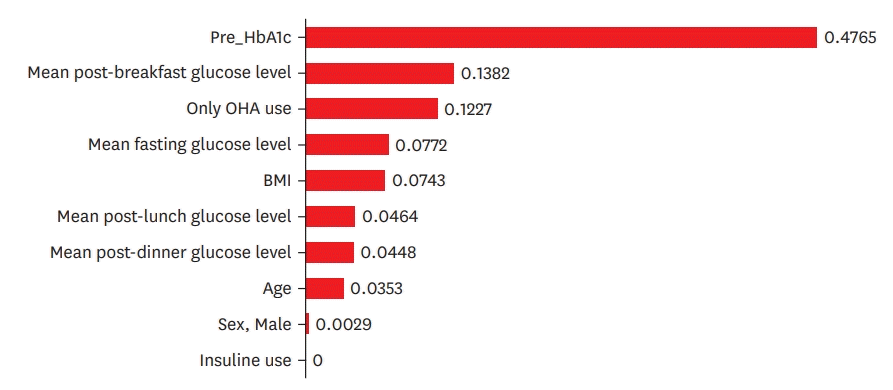
Table 1.
Baseline characteristics
Table 2.
Performance evaluation of each model using the leave-one-out cross-validation method