Abstract
Background
Since image-based fracture prediction models using deep learning are lacking, we aimed to develop an X-ray-based fracture prediction model using deep learning with longitudinal data.
Methods
This study included 1,595 participants aged 50 to 75 years with at least two lumbosacral radiographs without baseline fractures from 2010 to 2015 at Seoul National University Hospital. Positive and negative cases were defined according to whether vertebral fractures developed during follow-up. The cases were divided into training (n=1,416) and test (n=179) sets. A convolutional neural network (CNN)-based prediction algorithm, DeepSurv, was trained with images and baseline clinical information (age, sex, body mass index, glucocorticoid use, and secondary osteoporosis). The concordance index (C-index) was used to compare performance between DeepSurv and the Fracture Risk Assessment Tool (FRAX) and Cox proportional hazard (CoxPH) models.
Results
Of the total participants, 1,188 (74.4%) were women, and the mean age was 60.5 years. During a mean follow-up period of 40.7 months, vertebral fractures occurred in 7.5% (120/1,595) of participants. In the test set, when DeepSurv learned with images and clinical features, it showed higher performance than FRAX and CoxPH in terms of C-index values (DeepSurv, 0.612; 95% confidence interval [CI], 0.571 to 0.653; FRAX, 0.547; CoxPH, 0.594; 95% CI, 0.552 to 0.555). Notably, the DeepSurv method without clinical features had a higher C-index (0.614; 95% CI, 0.572 to 0.656) than that of FRAX in women.
As fragility fractures have emerged as a major social issue from both medical and economic standpoints [1-3], it is vital to preemptively identify individuals who are likely to experience fractures in the near future. Currently, several tools exist for finding patients who are likely to have fractures, such as the Fracture Risk Assessment Tool (FRAX), dual-energy X-ray absorptiometry (DXA), quantitative computed tomography (CT), and others [4,5]. While these approaches are well-validated measures in assessing patients [6,7], DXA is still underutilized and generally available only at referral centers [8], and lack of insight among both physicians and patients leads to low screening rates of <10%, even in high-risk populations [9,10]. Therefore, cost-effective and easily accessible alternatives to improve these circumstances are needed. Opportunistically taken spine X-rays, which are widely available in clinical practice and have good image quality, can be a candidate as an alternative method to discriminate patients at high risk of fracture.
In recent years, machine learning (ML) methodologies for analyzing medical images have been introduced in various medical fields, such as diagnosing diabetic retinopathy and lung nodules [11-13]. Among various methods, convolutional neural networks (CNNs) are an emerging methodology that has demonstrated its potential in many applications [14]. Compared to previous methodologies, a strength of CNNs is that they do not require hand-crafted feature extraction or segmentation by human experts, while they are computationally more expensive and require graphical processing units due to the millions of learnable parameters to calculate [15]. In several cross-sectional studies, CNN algorithms using X-rays and CT images have been applied to assess bone mineral density (BMD) or detect fractures [16-19]. Although these studies showed acceptable performance in classification and segmentation, there is still a lack of longitudinal studies on deep learning-based vertebral fracture prediction models.
Therefore, we aimed to develop a spine radiography-based fracture prediction model using deep learning with longitudinal data. The study could be a technical leap to identify patients at high risk of fractures with spine radiography, a readily accessible and cost-effective method.
This longitudinal cohort study included the images and medical records of 7,301 patients aged over 50 years who had at least two spine radiographs in the anteroposterior and lateral positions from 2010 to 2015 taken at Seoul National University Hospital. Patients with a history of fragility fractures at baseline (n=1,982) or those who visited only once (n=2,368) were excluded, as were patients who did not have lateral X-rays in a neutral position (n=697), those whose follow-up periods were less than 6 months (n=113), patients who were prescribed antiosteoporotic drugs (such as bisphosphonates, teriparatide, denosumab, or selective estrogen receptor modulators) (n=531), and those with radiographs of poor image quality (n=439) were excluded. As a result, 1,595 participants were eligible for the final analysis (Fig. 1). The training set (n=1,416) was randomly divided at 5:1 for cross-validation. Patients with BMD data measured within 1-year before or after X-ray imaging were selected as the test set (n=179), which enabled the calculation of FRAX.
The study protocol was approved by the Institutional Review Board of Seoul National University Hospital (IRB No. H-1902-050-1008). The requirement for informed consent was waived due to the retrospective design of the study. The study was carried out according to the World Medical Association Declaration of Helsinki—Ethical Principles for Medical Research.
The primary outcome of the study was incident vertebral fracture events. Vertebral fractures were defined as morphometric fractures confirmed by X-rays. Morphometric vertebral fractures were confirmed by X-rays with measurements of anterior, middle, and posterior vertebral heights. Anterior to posterior, middle to posterior, and posterior to posterior above and below ratios were calculated. A vertebral fracture was defined as being present if any of the abovementioned ratios were more than 3 standard deviations below the normal mean for the vertebral level, as described in our previous report [20]. Paired X-rays with follow-up intervals for each participant were obtained. Baseline X-rays were used as the source of the training model, and follow-up X-rays were used for identifying the outcome.
Sociodemographic factors were obtained from a review of electronic medical records, including age, sex, and previous medical history at baseline. The use of glucocorticoids was defined as the patient currently using oral glucocorticoids or having been exposed to oral glucocorticoids for more than 3 months at a dose of prednisolone >5 mg or its equivalent. Secondary osteoporosis included a history of type 1 diabetes mellitus, osteogenesis imperfecta, untreated hyperthyroidism, hypogonadism or premature menopause, chronic malnutrition or malabsorption, and chronic liver disease. Height and body weight were measured based on standard methods by trained staff using a scale and a wall-mounted extensometer while the participants were wearing light-weight clothes. Body mass index (BMI) was calculated as the weight divided by height squared (kg/m2).
The baseline BMD (g/cm2) of skeletal sites (lumbar spine, femoral neck, and total hip) and muscle mass were measured using DXA (GE Prodigy, GE Healthcare, Chicago, IL, USA) and analyzed (EnCORE Software version 11.0, GE Healthcare) according to the manufacturer’s guidance. The BMD precision error (% of the coefficient of variation) was 1.7% for the lumbar spine, 1.8% for the femoral neck, and 1.7% for the total hip. For the lumbar spine BMD, the L1–4 values were chosen for analysis. When an area of the spine was not suitable for analysis due to a compression fracture or severe sclerotic change, values from the rest of the spine were used (e.g., if L1 was not suitable, L2–4 was used). Instruments were calibrated using anthropomorphic phantoms.
The 10-year absolute risks of hip and osteoporotic fracture (FRAX scores) were calculated using the University of Sheffield’s online Korea-specific FRAX tool (https://www.sheffield.ac.uk/FRAX/tool.aspx?country=25). The FRAX algorithm includes the following parameters: femoral neck BMD T-score, age, sex, BMI, previous history of fracture, parental history of hip fracture, secondary osteoporosis, current smoking status, recent use of corticosteroids, presence of rheumatoid arthritis, and consumption of ≥3 alcoholic beverages per day.
The proposed deep learning-based lumbar spine fracture prediction framework comprises two main steps: (1) keypoint detection and (2) survival analysis. For each step, we applied deep CNNs for data-driven learning. A keypoint detection model was employed to extract and isolate the region including the vertebral bodies (L1–L5) from the original radiographs, followed by a survival model to predict the fracture risk score from the extracted region. Fig. 2 shows an overview of our framework. Preprocessing was done with both training and test sets.
First, the keypoint detection model was performed to extract the spatial region of interest (ROI) from lateral spine radiographs to remove irrelevant structures such as bowel gas [21,22]. The model localized five center points corresponding to each of the L1–L5 lumbar vertebral bodies. For the training keypoint detection model, all center key points of each vertebral body in the training and test dataset were manually annotated and validated by the authors, including a musculoskeletal radiologist (J.K.S., and K.H.J.). To evaluate the accuracy of the keypoint localization, the object keypoint similarity-based average precision (AP) metric, which is calculated from a distance between predicted points and ground truth points, was applied. Our keypoint detection model achieved a 0.971±0.020 mean AP score in five-fold cross-validation. Based on the extracted key points of the vertebra bodies, alignment of the original radiographs was performed. Rotation and translation transformations were performed so that each of the two points (L1 and L5) was always in the same position around all images. Then, the ROI was extracted from the area around the keypoints with an external margin. For training, input images were rescaled with min-max normalization and resized into a uniform size of 384×384 pixels with zero paddings. We trained our keypoint detection model on the training set using similar settings as previously described [22] with an ImageNet pre-trained HRNet-W32 backbone: data augmentations with random rotation, scale, and flipping. The Adam optimizer was used with an initial learning rate of 1e-3 that dropped to 1e-5, and there were 200 training epochs.
Six preprocessing methods, as shown in Supplemental Fig. S1, were tested to determine how best to manipulate X-rays for fracture prediction: full images containing the L1–L5 or L1–L4 vertebral bodies with and without masks, and individual patches of the bodies with and without masks. The masks used were manually annotated. A heatmap visualization of X-rays with and without fractures is depicted in Supplemental Fig. S2.
In baseline characteristics, depending on the distribution, continuous parameters are presented as means with standard deviations, and categorical data are presented as proportions. Comparisons between groups were analyzed by performing the Student t test, whereas the chi-square test was used for categorical variables. The area under the receiver operating characteristic curve (AUROC) was calculated for comparisons among preprocessed images. Cases that were predicted to have and experienced actual fracture events during the follow-up were defined as true positive (TP), while false positive (FP) cases were defined as those that were predicted to have but did not experience fracture (FP). Cases that were predicted to be free of fracture events but had one during follow-up were defined as false negative (FN). True negative (TN) cases were defined as those that were predicted to be and were free of fracture events during the follow-up. Sensitivity and specificity were calculated for each time series as follows: sensitivity=TP/(TP+FN) and specificity=TN/(TN+FP).
We built a Cox proportional hazard (CoxPH) model and DeepSurv model in the training set and predicted survival in the test set. CoxPH and DeepSurv survival models were compared from either only clinical data or with an additional baseline X-ray image. Clinical variables in CoxPH were selected from variables included in the FRAX model [5]. Both models measure hazard rates as the log-risk function. DeepSurv [23] is a multilayer perceptron that predicts the hazard rate based on both clinical information with image data and only clinical information (age, sex, BMI, previous fracture history, secondary osteoporosis, rheumatoid arthritis, and glucocorticoid usage). For images, a deep CNN was used. We evaluated the prediction models’ performance in terms of the concordance index (C-index), which can be regarded as the fractures of all pairs of individuals whose predicted survival times were correctly ordered. This metric is based on the Harrell C statistic, as described in previous studies [24-26]. However, although the C-index is easily implementable using available statistical packages and algorithms, it has an inherent limitation in its unclear validity/reliability [27] in datasets with censored data, as in our study, due to the possibility of inflated type 1 error [27].
Model 1 was adjusted for age and sex, model 2 was additionally adjusted for BMI, and model 3 was additionally adjusted for the use of glucocorticoids and secondary osteoporosis. The DeepSurv package from R and PyTorch from Python were used in the analyses. A P value <0.05 was considered significant. Statistical analyses were performed using R (The R Foundation, www.r-project.org) and Python version 3.9.4 (Python Software Foundation, https://www.python.org).
A total of 1595 participants were included in the final analysis. The mean follow-up duration was 3.4 years. The average age was 60.4 years old, and 1,188 (74.4%) participants were female (Table 1). The participants were divided into a training set (n=1,416) and a test set (n=179). The participants included in the training set were more likely to be female (P=0.020), had a higher BMI (P=0.002), and were less likely to have secondary osteoporosis (P=0.031) than those in the test set. During follow-up, vertebral fractures occurred in 120 (7.5%) of the participants.
Before training, we compared the performance of various types of preprocessed images, such as L1–L5, L1–4, and L1–L5 patches with and without masks (Table 2, Supplemental Fig. S1). L1–L5 spine images showed similar performance in discriminating those who were likely to develop a fracture in the future, regardless of the presence of masks for vertebral bodies (AUROC, 0.778 and 0.787, respectively). When images were cropped to include L1–L4, the performance was similar between those without and with masks for vertebral bodies (AUROC, 0.783 and 0.734, respectively), and similar to images including L1–L5. The best performance was observed in images with L1–L5 patches without a mask, with an AUROC of 0.802, while images with L1–L5 patches with masks showed the lowest AUROC (0.672). Therefore, we implemented images with L1–L5 patches without masks (Supplemental Fig. S1E) as image data in further prediction models.
In the training set, compared to conventional methods such as the CoxPH, both DeepSurv methods (with and without images) had higher C-index values in predicting fractures in women (model 3: CoxPH, 0.712; 95% confidence interval [CI], 0.654 to 0.770; DeepSurv without images, 0.740; 95% CI, 0.686 to 0.795; DeepSurv with images, 0.764; 95% CI, 0.739 to 0.789) (Fig. 3A). However, there was no significant difference according to whether spine X-ray images were used in DeepSurv. Consistent trends were observed in models 1, 2, and 3, which adjusted for age, additionally adjusted for BMI, and additionally adjusted for glucocorticoid use and secondary osteoporosis, respectively.
When we compared clinical models within the analytic methods, there were no significant differences among clinical models 1, 2, and 3 in all analytical techniques, including CoxPH, DeepSurv with and without images, and DeepSurv with image only (C-index, 0.748; 95% CI, 0.699 to 0.797) (Fig. 3B).
In the female test set, compared to the CoxPH method, DeepSurv with images had higher performance in predicting fractures than FRAX models 2, and 3, as represented by C-index values (FRAX, 0.547); model 2 (CoxPH, 0.553; 95% CI, 0.552 to 0.555; DeepSurv without images, 0.558; 95% CI, 0.521 to 0.595; DeepSurv with images, 0.610; 95% CI, 0.576 to 0.644); model 3 (CoxPH, 0.594; 95% CI, 0.584 to 0.604; DeepSurv without images, 0.433; 95% CI, 0.510 to 0.579; DeepSurv with images, 0.612; 95% CI, 0.571 to 0.653) (Fig. 4A). The DeepSurv method with images had a higher C-index than the DeepSurv method without images in model 3. However, there was no significant difference between CoxPH and the DeepSurv method without images in all clinical models. In addition, the C-index was similar between DeepSurv methods with and without images in models 1 and 2.
As described in Fig. 4B, when we compared clinical models using the CoxPH method, model 3 had a higher C-index than FRAX, model 1, and model 2. However, model 3 had a higher C-index than model 1 using the DeepSurv method with images. Notably, when using the DeepSurv method with images without clinical features, the C-index (0.614; 95% CI, 0.572 to 0.656) was higher than that of FRAX. There were no significant differences in the C-index among clinical models when applying the DeepSurv method without images.
In this study, we found that the CNN-based DeepSurv prediction model using baseline spine X-rays provided comparable vertebral fracture risk prediction with the well-established clinical standard of FRAX in longitudinal data. Among various preprocessed image models, L1–5 patches without masks exhibited the best performance (AUC, 0.801). In the test set with DXA, the predictive performance of DeepSurv was higher than that of FRAX, even when only images were used for the prediction (C-index, 0.614 for DeepSurv and 0.547 for FRAX) in women.
We showed relatively good performance with a small number of X-ray images. Cutting images to create patches for input in deep learning models has recently been attempted by other groups [28]. Early studies tried segmented images of vertebrae based on geometry and intensity to detect fractures, but reported unstable results [29,30]. However, with the recent employment of deep learning methods, researchers trained CNN models with patches from localized and segmented images of vertebrae, achieving an accuracy of 89% to 90% [28,31,32]. Therefore, in conjunction with deep learning models, multiple slices in the spine region provided better performance in detecting and localizing fractures than a single image slice. However, as this approach has never been tried for predicting fractures, we evaluated the performance of both single slices and multiple patches before we carried out the model analyses. Our results aligned with previous results showing that the patch images showed the highest performance in distinguishing between patients who developed fractures and those who did not.
In the present study, the performance of DeepSurv was acceptable in predicting fractures—in fact, its performance was better than that of FRAX. It was clinically notable that the image-only model without clinical risk factors also showed comparable performance in predicting fractures. There have been only a few studies on fracture prediction using deep learning [33-35]. Most studies used databases to build prediction models using ML. Su et al. [35] reported that the classification of a high-risk group for hip fractures using the ML method of classification and regression trees showed similar performance to that of FRAX (AUC, 0.72 vs. 0.70). In another study, a fracture prediction model using the CatBoost method slightly outperformed the FRAX score for fracture prediction (AUC, 0.69 vs. 0.66) [34]. Based on data from more than 280,000 individuals, a hip fracture prediction model using support vector machines and RUSBoost showed AUCs of 0.65 to 0.70 [35]. Although the C-index and AUC are not directly comparable, the performance of DeepSurv in the study was similar to or better than previously reported [33-35]. However, in most previous studies, performance was only demonstrated in terms of the AUC since fracture events were regarded as cross-sectional binary outcomes, without considering the time factor. Therefore, this study has clinical significance in that it introduces the concept of survival analysis to a deep learning-based fracture prediction model.
We demonstrated that the performance for fracture prediction of DeepSurv was acceptable compared to that of FRAX in other previous studies. The reported C-index values of FRAX range from 0.62 to 0.77 [35,36]. In a recent study, the performance of hip fracture prediction was reported using BMD, FRAX, and BMD with finite element analysis from DXA scans. The C-index values were 0.76 for total hip BMD, 0.73 for FRAX with BMD, and 0.77 for BMD with finite element analysis [37]. In our model using X-ray images, although C-index values differed depending on the degree of clinical information, they were between 0.76 and 0.79, similar to the previous studies. The results imply that with deep learning, similar performance to that of FRAX may be achieved only with a single X-ray image.
The study has some notable strengths. This was the first infield study to use X-ray images to build a fracture prediction model with a deep learning methodology. A previous small study built a fracture prediction model using CT images with deep learning [34], but no previous study has been tried with X-ray images. Another strength of this study is the use of DeepSurv, a survival deep learning model, the performance of which was analyzed in terms of the C-index. Most previous deep learning studies have been designed as cross-sectional studies that classify patients according to whether they experienced fractures or not [17,38]. However, for fracture events, the factor of time-to-event should be considered when constructing a prediction model. In addition, the process of selecting various forms of preprocessed images of spine X-rays was demonstrated, which may help in the design of future research using X-rays. Heatmaps of the deep learning process were also generated, enhancing the interpretability of the model. Moreover, the DeepSurv model in the study showed acceptable performances compared to FRAX, and it was clinically notable that X-ray image data analyzed using the DeepSurv model without clinical information showed better performance than FRAX.
The study has some limitations. The sample size was relatively small for ML, which may have led to overfitting of the training model. Furthermore, although we showed better performance of the DeepSurv model than FRAX, the model has room for improvement, as we had insufficient fracture cases. In practice, the model used by itself would not be sufficient to assess the risk of fracture or to start treatment based on its relatively low performance. Larger studies in the future could not only validate, but also improve upon the present findings. Since this was not a nationwide study, we could not identify fracture events that happened in other institutions. In addition, because most conventional fracture prediction models, including FRAX, are developed for the 10-year risk of fracture events, the comparison with FRAX in this study had a major inherent limitation. Therefore, the results should be interpreted with caution. With sufficient follow-up duration and more fracture cases, the model’s predictive performance may be improved. Although we used an intensive automated electronic medical record search, missing data related to the retrospective approach could have been present (e.g., age at menopause). The date of the subsequent fracture might not have been accurate since it was the date of X-ray acquisition, not the exact date of the fracture. Segmenting L5 was another challenge in the study due to lumbosacralization. Although the radiologists from our team reviewed all images, lumbosacralization may have affected the results of the study. We acknowledge potential issues of selection bias, since the participants were treated at a hospital and were more likely to have underlying diseases than the healthy population. In addition, as we selected patients with BMD data for the test set, the number of participants in the test set was relatively small. Therefore, selection bias might have affected the model’s performance.
In conclusion, we have shown that a deep learning-based model derived from spine X-rays may provide acceptable predictive performance for fracture based on a comparison with FRAX for presymptomatic prediction of future vertebral fractures. The incidental X-ray-based model could help find some unscreened individuals at increased risk for vertebral fracture; this issue of underrecognition is particularly relevant in the context of the coronavirus disease 2019 pandemic, which has made DXA screening difficult to access. This opportunistic approach may also add additional value to X-rays performed for other indications to find patients at high risk of fracture. Further studies conducted at various institutions with a longer duration of follow-up are needed before applying the algorithm.
ACKNOWLEDGMENTS
The study was funded by the National Research Foundation of Korea (grant number 2020R1A2C2011587).
Notes
Supplementary Information
Supplemental Fig. S1.
Preprocessed image types of L1-L5 (A) without or (B) with masks, L1-4 (C) without or (D) with masks, and L1-L5 patches (E) without or (F) with masks.
Supplemental Fig. S2.
Heatmap visualization of spine radiographs (A) without and (B) with osteoporotic fracture during follow-up.
REFERENCES
1. Tran O, Silverman S, Xu X, Bonafede M, Fox K, McDermott M, et al. Long-term direct and indirect economic burden associated with osteoporotic fracture in US postmenopausal women. Osteoporos Int. 2021; 32:1195–205.


2. Williams SA, Daigle SG, Weiss R, Wang Y, Arora T, Curtis JR. Economic burden of osteoporosis-related fractures in the US Medicare population. Ann Pharmacother. 2021; 55:821–9.


3. National Bone Health Policy Institute. New report on burden of osteoporosis highlights huge and growing economic and human toll of the disease [Internet]. Arlington: BHOF;2019. [cited 2022 Jul 11]. Available from: https://www.bonehealthandosteoporosis.org/news/new-report-on-burden-of-osteoporosis-highlights-huge-and-growing-economic-and-human-toll-of-the-disease/.
4. Stone KL, Seeley DG, Lui LY, Cauley JA, Ensrud K, Browner WS, et al. BMD at multiple sites and risk of fracture of multiple types: long-term results from the Study of Osteoporotic Fractures. J Bone Miner Res. 2003; 18:1947–54.


5. Kanis JA, Harvey NC, Johansson H, Oden A, Leslie WD, McCloskey EV. FRAX update. J Clin Densitom. 2017; 20:360–7.


6. Dimai HP. Use of dual-energy X-ray absorptiometry (DXA) for diagnosis and fracture risk assessment: WHO-criteria, T- and Z-score, and reference databases. Bone. 2017; 104:39–43.


8. Hoiberg MP, Rubin KH, Hermann AP, Brixen K, Abrahamsen B. Diagnostic devices for osteoporosis in the general population: a systematic review. Bone. 2016; 92:58–69.


9. Marshall D, Johnell O, Wedel H. Meta-analysis of how well measures of bone mineral density predict occurrence of osteoporotic fractures. BMJ. 1996; 312:1254–9.


10. Siris ES, Chen YT, Abbott TA, Barrett-Connor E, Miller PD, Wehren LE, et al. Bone mineral density thresholds for pharmacological intervention to prevent fractures. Arch Intern Med. 2004; 164:1108–12.


11. Keel S, Wu J, Lee PY, Scheetz J, He M. Visualizing deep learning models for the detection of referable diabetic retinopathy and glaucoma. JAMA Ophthalmol. 2019; 137:288–92.


12. Sung J, Park S, Lee SM, Bae W, Park B, Jung E, et al. Added value of deep learning-based detection system for multiple major findings on chest radiographs: a randomized crossover study. Radiology. 2021; 299:450–9.


13. Yasaka K, Akai H, Abe O, Kiryu S. Deep learning with convolutional neural network for differentiation of liver masses at dynamic contrast-enhanced CT: a preliminary study. Radiology. 2018; 286:887–96.


14. Yamashita R, Nishio M, Do R, Togashi K. Convolutional neural networks: an overview and application in radiology. Insights Imaging. 2018; 9:611–29.


15. Derkatch S, Kirby C, Kimelman D, Jozani MJ, Davidson JM, Leslie WD. Identification of vertebral fractures by convolutional neural networks to predict nonvertebral and hip fractures: a registry-based cohort study of dual X-ray absorptiometry. Radiology. 2019; 293:405–11.


16. Bluthgen C, Becker AS, Vittoria de Martini I, Meier A, Martini K, Frauenfelder T. Detection and localization of distal radius fractures: deep learning system versus radiologists. Eur J Radiol. 2020; 126:108925.
17. Olczak J, Fahlberg N, Maki A, Razavian AS, Jilert A, Stark A, et al. Artificial intelligence for analyzing orthopedic trauma radiographs. Acta Orthop. 2017; 88:581–6.


18. Yasaka K, Akai H, Kunimatsu A, Kiryu S, Abe O. Prediction of bone mineral density from computed tomography: application of deep learning with a convolutional neural network. Eur Radiol. 2020; 30:3549–57.


19. Loffler MT, Jacob A, Scharr A, Sollmann N, Burian E, El Husseini M, et al. Automatic opportunistic osteoporosis screening in routine CT: improved prediction of patients with prevalent vertebral fractures compared to DXA. Eur Radiol. 2021; 31:6069–77.


20. Shin CS, Kim MJ, Shim SM, Kim JT, Yu SH, Koo BK, et al. The prevalence and risk factors of vertebral fractures in Korea. J Bone Miner Metab. 2012; 30:183–92.


21. Sun K, Xiao B, Liu D, Wang J. Deep high-resolution representation learning for human pose estimation. In : Proceedings of the IEEE/CVF Conference on Computer Vision and Pattern Recognition; 2019 Jun 16-20; Long Beach, CA. Available from: https://openaccess.thecvf.com/content_CVPR_2019/papers/Sun_Deep_High-Resolution_Representation_Learning_for_Human_Pose_Estimation_CVPR_2019_paper.pdf.


22. Katzman JL, Shaham U, Cloninger A, Bates J, Jiang T, Kluger Y. DeepSurv: personalized treatment recommender system using a Cox proportional hazards deep neural network. BMC Med Res Methodol. 2018; 18:24.


23. Iyer S, Sowmya A, Blair A, White C, Dawes L, Moses D. A novel approach to vertebral compression fracture detection using imitation learning and patch based convolutional neural network. In : Proceedings of the 2020 IEEE 17th International Symposium on Biomedical Imaging (ISBI); 2020 Apr 3-7; Iowa City, IA. Piscataway, NJ: IEEE;2020. p. 726–30.


24. de Vries B, Hegeman JH, Nijmeijer W, Geerdink J, Seifert C, Groothuis-Oudshoorn C. Comparing three machine learning approaches to design a risk assessment tool for future fractures: predicting a subsequent major osteoporotic fracture in fracture patients with osteopenia and osteoporosis. Osteoporos Int. 2021; 32:437–49.


25. Xiao X, Wu Q. The utility of genetic risk score to improve performance of FRAX for fracture prediction in US postmenopausal women. Calcif Tissue Int. 2021; 108:746–56.


26. El-Hajj Fuleihan G, Chakhtoura M, Cauley JA, Chamoun N. Worldwide fracture prediction. J Clin Densitom. 2017; 20:397–424.


27. Han X, Zhang Y, Shao Y. On comparing 2 correlated C indices with censored survival data. Stat Med. 2017; 36:4041–9.


28. Ghosh S, Raja’S A, Chaudhary V, Dhillon G. Automatic lumbar vertebra segmentation from clinical CT for wedge compression fracture diagnosis. In : SPIE Medical Imaging 2011; Computer-Aided Diagnosis. 2011 Feb 15; Orlando, FL: https://doi.org/10.1117/12.878055.


29. Wang Y, Yao J, Burns JE, Summers R. Osteoporotic and neoplastic compression fracture classification on longitudinal CT. In : Proceedings of the 2016 IEEE 13th International Symposium on Biomedical Imaging (ISBI); 2016 Apr 13-16; Prague, CZ. Piscataway, NJ: IEEE;2016. p. 1181–4.


30. Bar A, Wolf L, Amitai OB, Toledano E, Elnekave E. Compression fractures detection on CT. In : SPIE Medical Imaging 2017: Computer-Aided Diagnosis; 2017 Feb 13-16; Orlando, FL. https://doi.org/10.1117/12.2249635.


31. Tomita N, Cheung YY, Hassanpour S. Deep neural networks for automatic detection of osteoporotic vertebral fractures on CT scans. Comput Biol Med. 2018; 98:8–15.


32. Muehlematter UJ, Mannil M, Becker AS, Vokinger KN, Finkenstaedt T, Osterhoff G, et al. Vertebral body insufficiency fractures: detection of vertebrae at risk on standard CT images using texture analysis and machine learning. Eur Radiol. 2019; 29:2207–17.


33. Tecle N, Teitel J, Morris MR, Sani N, Mitten D, Hammert WC. Convolutional neural network for second metacarpal radiographic osteoporosis screening. J Hand Surg Am. 2020; 45:175–81.


34. Yamamoto N, Sukegawa S, Kitamura A, Goto R, Noda T, Nakano K, et al. Deep learning for osteoporosis classification using hip radiographs and patient clinical covariates. Biomolecules. 2020; 10:1534.


35. Su Y, Kwok T, Cummings SR, Yip B, Cawthon PM. Can classification and regression tree analysis help identify clinically meaningful risk groups for hip fracture prediction in older American men (the MrOS cohort study)? JBMR Plus. 2019; 3:e10207.


36. Kong SH, Ahn D, Kim BR, Srinivasan K, Ram S, Kim H, et al. A novel fracture prediction model using machine learning in a community-based cohort. JBMR Plus. 2020; 4:e10337.


37. Engels A, Reber KC, Lindlbauer I, Rapp K, Buchele G, Klenk J, et al. Osteoporotic hip fracture prediction from risk factors available in administrative claims data: a machine learning approach. PLoS One. 2020; 15:e0232969.
Fig. 1.
Flow chart of patient selection. SNUH, Seoul National University Hospital; AP, anteroposterior.
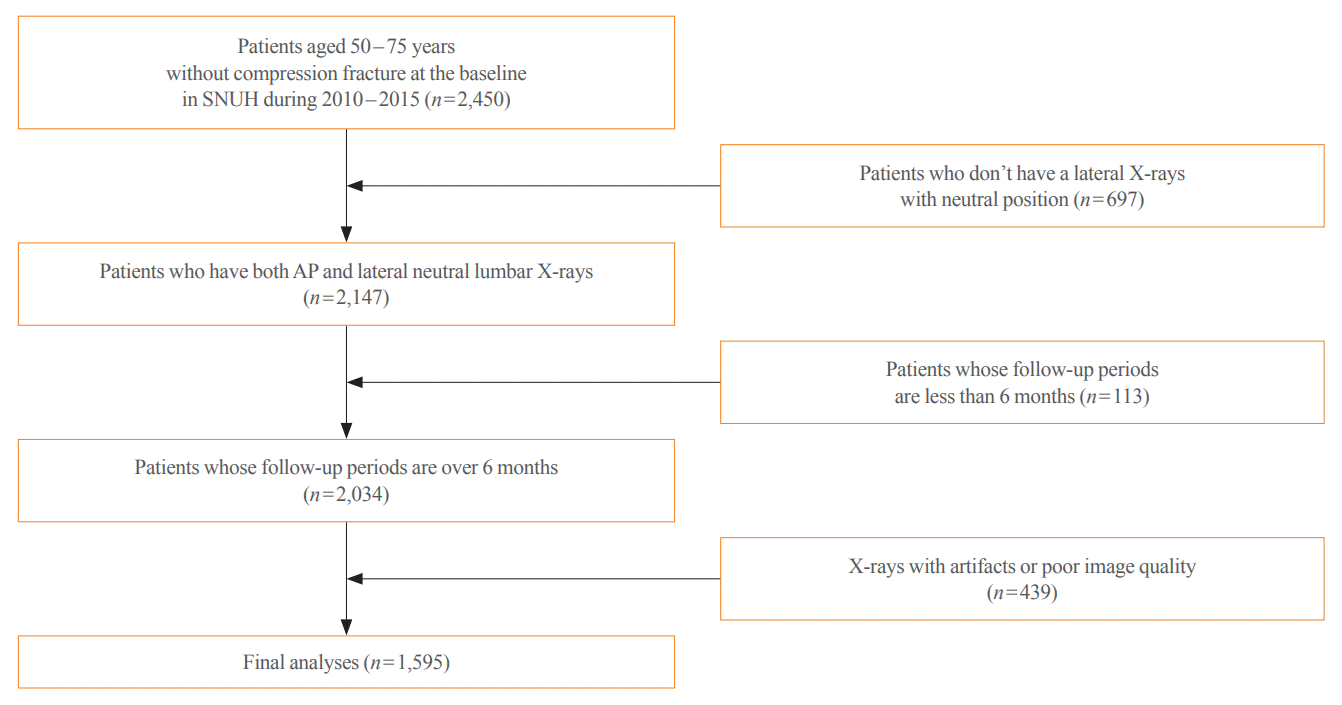
Fig. 2.
The architecture of the deep learning-based survival prediction model. HRNet, high-resolution net; ResNet, residual network; BMI, body mass index; FC, fold change.
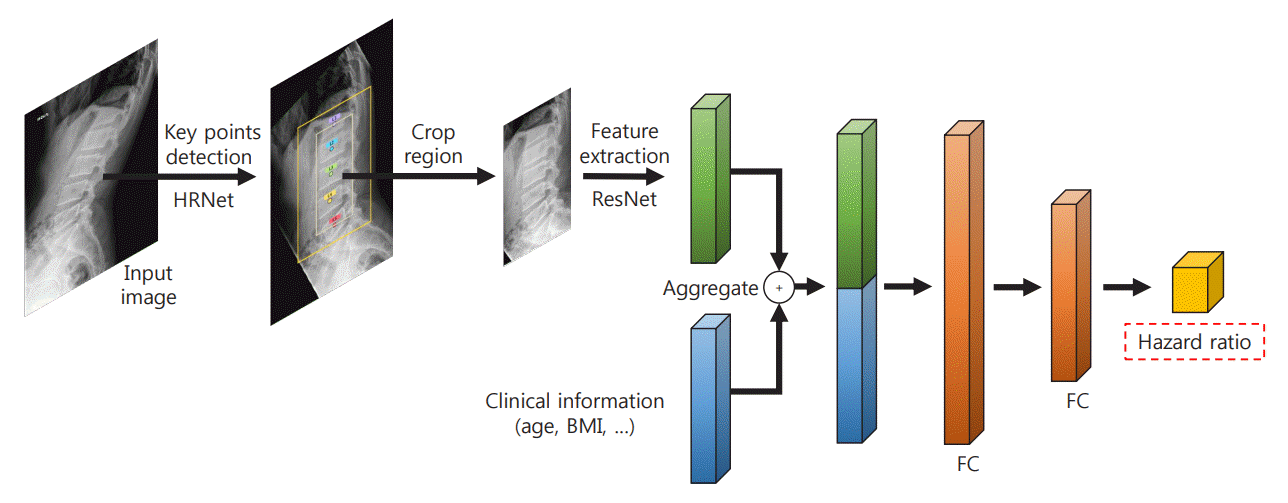
Fig. 3.
Performance of the fracture prediction model in the training set using Cox proportional hazard and DeepSurv methods (A) according to clinical models and (B) analytic methods. Model 1 adjusted for age and sex, model 2 additionally adjusted for body mass index, and model 3 additionally adjusted for the use of glucocorticoids and secondary osteoporosis. The C-index values were as follows: Model 1 (Cox proportional hazard [CoxPH], 0.712; 95% confidence interval [CI], 0.652 to 0.773; DeepSurv without images, 0.765; 95% CI, 0.693 to 0.837; DeepSurv with images, 0.794; 95% CI, 0.760 to 0.828); Model 2 (CoxPH, 0.709; 95% CI, 0.648 to 0.771; DeepSurv without images, 0.737; 95% CI, 0.683 to 0.791; DeepSurv with images, 0.782; 95% CI, 0.755 to 0.810); Model 3 (CoxPH, 0.712; 95% CI, 0.654 to 0.770; DeepSurv without images, 0.740; 95% CI, 0.686 to 0.795; DeepSurv with images, 0.764; 95% CI, 0.739 to 0.789). aP<0.05 between groups.
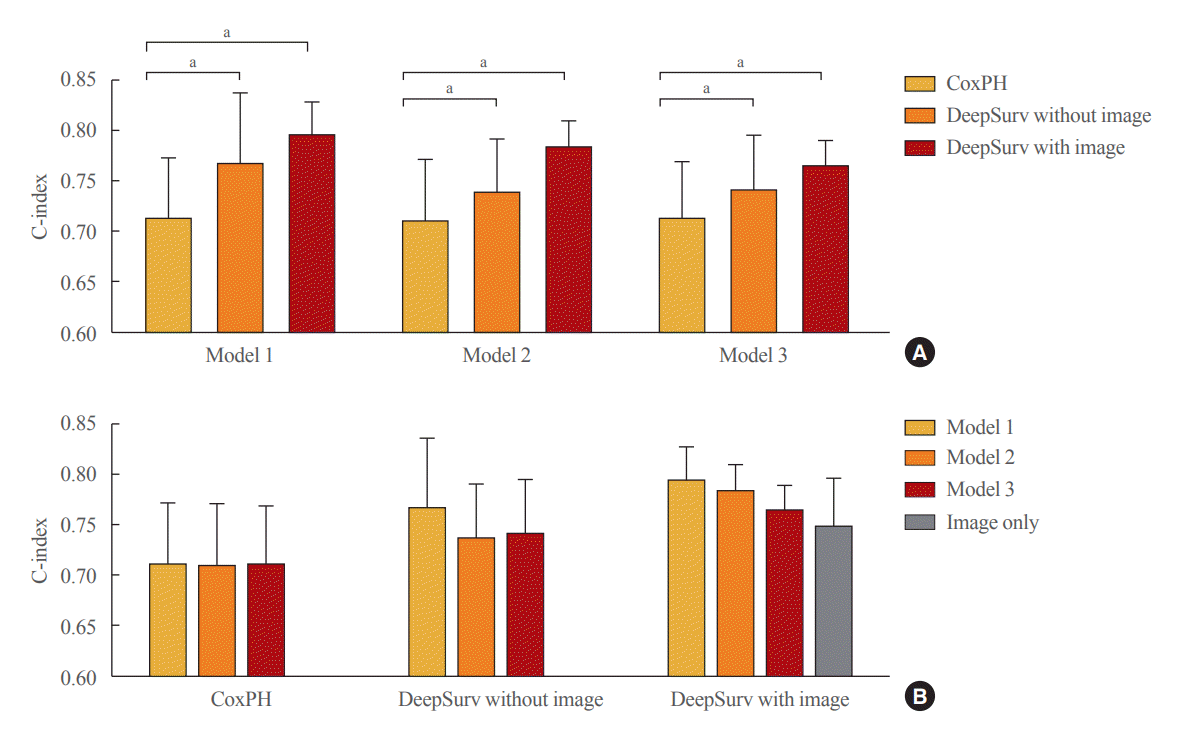
Fig. 4.
Performance of fracture prediction model in the test set using Cox proportional hazard and DeepSurv methods (A) according to clinical models and (B) analytic methods. Model 1 adjusted for age, model 2 additionally adjusted for body mass index, and model 3 additionally adjusted for the use of glucocorticoids and secondary osteoporosis. The C-index values were as follows: Model 1 (Cox proportional hazard [CoxPH], 0.552; 95% confidence interval [CI], 0.550 to 0.554; DeepSurv without images, 0.568; 95% CI, 0.521 to 0.585; DeepSurv with images, 0.544; 95% CI, 0.481 to 0.607); Model 2 (CoxPH, 0.553; 95% CI, 0.552 to 0.555; DeepSurv without images, 0.558; 95% CI, 0.521 to 0.595; DeepSurv with images, 0.610; 95% CI, 0.576 to 0.644); Model 3 (CoxPH, 0.594; 95% CI, 0.584 to 0.604; DeepSurv without images, 0.433; 95% CI, 0.510 to 0.579; DeepSurv with images, 0.612; 95% CI, 0.571 to 0.653). FRAX, Fracture Risk Assessment Tool. aP<0.05 between groups.
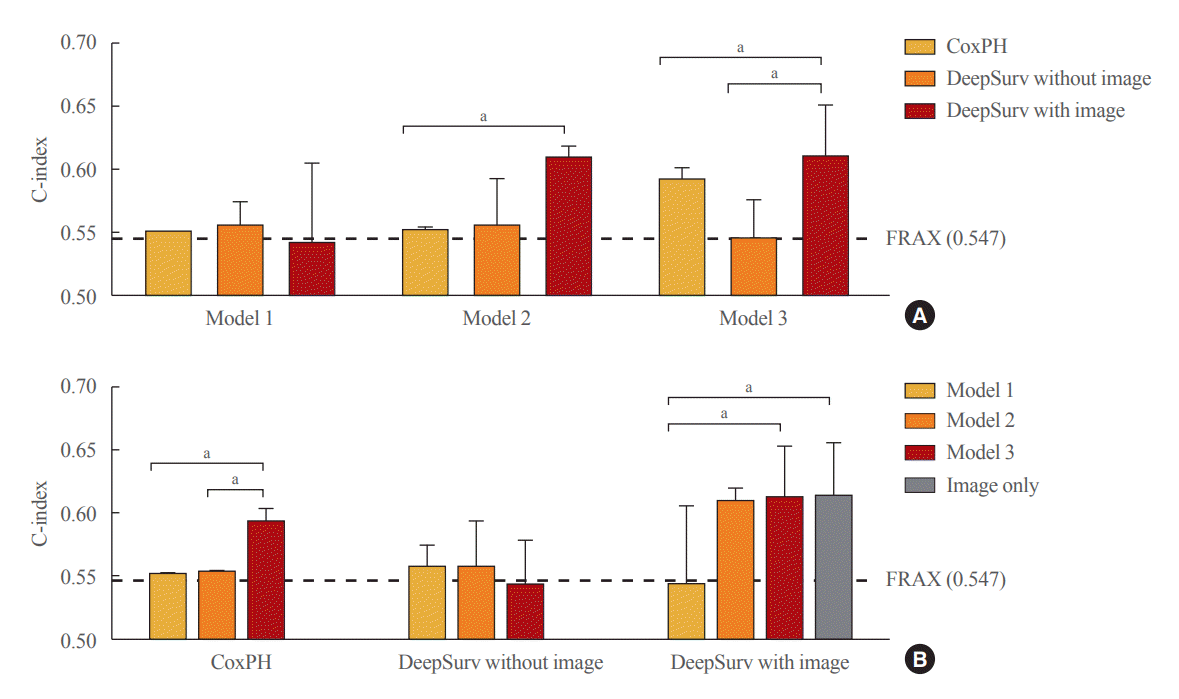
Table 1.
Baseline Clinical Characteristics
Table 2.
Performance in Discriminating Patients Likely to Develop a Fracture according to Image Types