Abstract
Background
To investigate the clinical characteristics of gestational diabetes mellitus (GDM) in Korea, using a nationwide database.
Methods
We analyzed 417,139 women who gave birth between 2011 and 2015 using the Korean National Health Information Database. They underwent the Korean National Health Screening Program within one year before pregnancy and were not prescribed drugs for diabetes nor diagnosed with diabetes mellitus before 280 days antepartum. Patients with GDM were defined as those who visited the outpatient clinic more than twice with GDM codes.
Results
The prevalence of GDM was 12.70% and increased with increasing maternal age, prepregnancy body mass index (BMI), waist circumference (WC), and fasting plasma glucose (FPG) (P for trend <0.05). As compared with those aged <25 years, the odds ratio for women with GDM aged ≥40 years were 4.804 (95% confidence interval [CI], 4.436 to 5.203) after adjustment for covariates. Women with prepregnancy BMI ≥30 kg/m2 were at 1.898 times (95% CI, 1.736 to 2.075) greater risk for GDM than those with prepregnancy BMI <18.5 kg/m2. Women with WC of ≥95 cm were at 1.158 times (95% CI, 1.029 to 1.191) greater risk for GDM than women with WC of less than 65 cm. High FPG, high income, smoking, and drinking were associated with an elevated risk of GDM.
Gestational diabetes mellitus (GDM) is defined as the existence of impaired glucose metabolism in pregnancy and is associated with high adverse health risks to both the mother and child [1–4]. In the Hyperglycemia and Adverse Pregnancy Outcomes study, the risk of adverse maternal, fetal, and neonatal outcomes increased with progressive hyperglycemia, even with no clear inflection points [5]. In addition, GDM has been related not only to adverse perinatal outcomes but also to long-term adverse outcomes, such as an increased risk of developing metabolic diseases later in life among both women and their offspring [6–9]. Nowadays, the prevalence of GDM has been increasing in the world [6,10]. Therefore, it is time to focus on women with GDM for the benefit of public health.
Until now, many risk factors have been identified, such as advanced maternal age, obesity, family history of type 2 diabetes mellitus, previous history of GDM, and ethnicity [11,12]. In the case of ethnicity, several community-based studies conducted in Western countries reported that Asian women are at higher risk of GDM than other ethnic groups despite having relatively low body mass index (BMI) values [13]. Even in Korea, of course, to prevent and manage the condition appropriately, it is necessary to know the characteristics of women with GDM. There are many studies that have analyzed the characteristics of women with GDM in small numbers [14–17]. However, limited studies have identified the characteristics of women with GDM using large-scale patient data. This study aimed to investigate the clinical characteristics of women with GDM in Korea using a large-scale population dataset from the National Health Information Database (NHID).
This analysis was conducted using data from the NHID, which is produced by the National Health Insurance Service with linkage to the National Death Registry and the National Health Screening Program. We identified pregnant women who gave birth between 2011 and 2015 in the NHID. Among them, we enrolled 426,602 women who were participated in the Korean National Health Screening Program within 1 year before the pregnancy assessed in this study. We excluded women who had been prescribed drugs for diabetes (n=3,305), who had fasting plasma glucose (FPG) levels of 126 mg/dL or greater (n=1,816) before pregnancy, or who were missing information (n=4,342). Finally, a total of 417,139 women were enrolled. All data used in this study were approved and provided in a de-identified form by the National Health Insurance Service (NHIS-2020-1-093). Approval for the present study protocol (2019-03-065) was obtained from the Institutional Review Board of CHA Bundang Medical Center. The requirement for informed consent was waived because we did not access personal identifying information.
Anthropometric and laboratory data were obtained via the National Health Screening Program before pregnancy. BMI, waist circumference (WC), and blood pressure (both systolic and diastolic values) were measured. Laboratory tests (e.g., plasma glucose, total cholesterol, triglyceride, high-density lipoprotein, and low-density lipoprotein) were performed using enzymatic methods (Hitachi 747 autoanalyzer, Hitachi Ltd., Tokyo, Japan) after an overnight fast of at least 8 hours. Information about lifestyle factors, including smoking status, alcohol status, regular exercise, and past medical history (family history of diabetes, history of hypertension, dyslipidemia, and prediabetes) was obtained using a standardized self-assessment questionnaire. A family history of diabetes was defined having first-degree relatives with diabetes. Smoking history was categorized based on the answers to “have you ever been a smoker?” and “if yes, do you smoke currently?” as follows: nonsmoker, ex-smoker, or current smoker. Heavy alcohol consumption was defined as drinking more than 30 g/day and drinking status was divided into three categories: nondrinker, mild drinker, and heavy drinker. Household income level was categorized as medical aid beneficiaries and by quartile (Q1–Q4). Regular exercise was defined as more than 30 minutes of moderate physical activity performed at least five times per week or more than 20 minutes of strenuous physical activity performed at least three times per week.
Patients with GDM were identified as follows: (1) delivered a baby between 2011 and 2015; (2) did not have a claim for diabetes mellitus based on International Classification of Diseases, 10th revision (ICD-10) codes (E10–E14) or oral antidiabetic drug or insulin use status before pregnancy; (3) did not have an FPG level of 126 mg/dL or greater before pregnancy; and (4) visited the outpatient clinic more than two times with GDM codes.
Data for categorical factors are reported as number (%) values and continuous variables are presented as mean±standard deviation or median (interquartile range) values. The significance of differences in measurements between women with GDM and women without GDM was assessed using the independent sample t test and chi-square test. The odds ratio (OR) and 95% confidence interval (CI) for the development of GDM were calculated by multivariate logistic regression analysis after adjustment for potential covariates. We also assessed participants for the risk of GDM according to location. A P value of less than 0.05 was considered statistically significant. Analyses were performed with SAS version 9.4 (SAS Institute, Cary, NC, USA).
Table 1 shows the baseline characteristics of study participants. The prevalence of GDM was 12.71% (n=53,011), while the mean age of women with GDM was 31.56 years and the mean prepregnancy BMI was 21.55 kg/m2. Age, BMI, WC, blood pressure (systolic and diastolic), FPG, total cholesterol, triglycerides, and low-density lipoprotein cholesterol were elevated, while high-density lipoprotein cholesterol was depressed in women with GDM. Among women with GDM, 18.53% had a family history of diabetes, 2.12% had hypertension, 4.16% had dyslipidemia, and 12.11% had prediabetes. Women who performed regular exercise and earned a high income more often had GDM relative to those who did not.
The prevalence of GDM was increased with increasing age (<25 years, 4.89%; 25–29 years, 8.36%; 30–34 years, 15.57%; 35–39 years, 19.42%; ≥40 years, 22.46%; P for trend <0.05) (Fig. 1A), prepregnancy BMI (<18.5 kg/m2, 10.57%; 18.5–22.9 kg/m2, 11.96%; 23.0–24.9 kg/m2, 15.31%; 25.0–29.9 kg/m2, 18.07%; ≥30.0 kg/m2, 24.53%; P for trend <0.05) (Fig. 1B), WC (<65 cm, 10.63%; 65–74 cm, 12.10%; 75–84 cm, 15.47%; 85–94 cm, 18.97%; ≥95 cm, 23.23%; P for trend <0.05) (Fig. 1C), and FPG (<80 mg/dL, 10.40%; 80–89 mg/dL, 11.50%; 90–99 mg/dL, 13.71%; 100–109 mg/dL, 18.29%; 110–125 mg/dL, 24.69%; P for trend <0.05) (Fig. 1D). Detailed characteristics according to age, prepregnancy BMI, WC, and FPG were presented as Supplemental Tables S1–S4. As compared with those aged younger than 25 years, the ORs for participants with GDM aged 25–29, 30–34, 35–39, and ≥40 years were 1.771 (95% CI, 1.658 to 1.892), 3.439 (95% CI, 3.222 to 3.671), 4.255 (95% CI, 3.971 to 4.559), and 4.804 (95% CI, 4.436 to 5.203) after adjustment for covariates (Table 2). Women with prepregnancy BMI values of 18.5–22.9, 23.0–24.9, 25.0–29.9, and ≥30 kg/m2 were at 1.043 (95% CI, 1.012 to 1.075), 1.226 (95% CI, 1.176 to 1.279), 1.383 (95% CI, 1.317 to 1.452), and 1.898 times (95% CI, 1.736 to 2.075) greater risk for GDM than those with prepregnancy BMI values of <18.5 kg/m2, respectively. Women with WC of 85 to 94 cm and ≥95 cm were at 1.142 (95% CI, 1.077 to 1.212) and 1.158 times (95% CI, 1.029 to 1.191) greater risk for GDM than women with WC of less than 65 cm. The ORs for GDM among participants with FPG levels of 100 to 109 mg/dL and 110 to 125 were 1.714 (95% CI, 1.649 to 1.780) and 2.483 (95% CI, 2.340 to 2.636) as compared with those with FPG levels of less than 80 mg/dL. The more detailed results by subdividing age, prepregnancy BMI, WC, and FPG were presented in Supplemental Table S5. The risk of GDM in women with the highest income (Q4) was 1.198 times (95% CI, 1.138 to 1.261) higher than in women who were medical aid beneficiaries.
As compared with women who were not drinkers, those with a mild drinking habit showed an elevated risk of GDM (OR, 1.024; 95% CI, 1.004 to 1.043), while those with a heavy drinking habit did not (Table 3). Meanwhile, ex- and current smokers were at 1.115 (95% CI, 1.063 to 1.169) and 1.087 times (95% CI, 1.035 to 1.143) greater risk, respectively, for developing GDM than nonsmokers. Regular exercise was not significantly associated with an elevated risk of GDM.
The incidence rates of GDM in Seoul and Gyeonggi were 14.27% and 12.54% (Fig. 2). Participants in Sejong showed the highest incidence rate (27.86%) and those in Gwangju showed the lowest (4.59%). Participants from Daejeon, Ulsan, Sejong, and Gyeongbuk were at higher risk of GDM, while those of Busan, Daegu, Incheon, Gwangju, Gyeonggi, Gangwon, Jeonbuk, Jeonnam, and Gyeongnam were at lower risk relative to those in Seoul (Supplemental Table S6).
This study investigated the clinical characteristics of Korean women with GDM using information from a national database. Our study suggests the prevalence of GDM in Korean women was 12.70% overall in 2011 to 2015. The incidence rate of GDM was increased with advancing age, prepregnancy BMI, WC, and FPG. Meanwhile, risk factors for GDM included increased age, prepregnancy BMI, WC, FPG, high income, smoking, and drinking after adjustment for covariates.
Several studies have investigated the prevalence of GDM in Korea [18–20]. According to the ‘2013 Diabetes Fact Sheet in Korea,’ the prevalence of GDM was 4.1% in 2007 and steadily increased annually by 1% to 2% to 2011 [18]. In a retrospective cohort study conducted using the Health Insurance Review and Assessment (HIRA) database, the age-adjusted annual prevalence of GDM from 2009 to 2011 was 7.5% [19]. Before 2011 in Korea, universal screening of GDM was performed and GDM was diagnosed using a two-step approach involving a 50-g oral glucose tolerance test (OGTT) followed by a 100-g OGTT. The Korean Diabetes Association endorsed a one-step approach using a 75-g OGTT according to the International Association of the Diabetes and Pregnancy Study Groups criteria in 2011 [21] and pregnant women since then have been evaluated for GDM using one of both methods. The prevalence of GDM appeared to be increasing after the adoption of the one-step approach worldwide [6,10] and this trend would, in theory, be observable in Korea as well. In this study, although we could not know what proportion of participants was given the one-step versus two-step diagnostic approach, we found that the prevalence of GDM between 2011 and 2015 had increased to 12.70%.
Yoo et al. [20] investigated 219,043 women between January 2011 and December 2012 and showed that the prevalence of GDM was 23.81%. They used the same database (NHID) as this study and their definition of GDM also relied on ICD-10 codes (O24.4 and O24.9). However, the prevalence of GDM was higher in their investigation than in this study and worldwide. Besides the use of a one-step approach for GDM diagnosis, this difference may be because women with GDM were defined as those who visited the outpatient clinic more than just one time with an ICD-10 code for GDM. Because it is not possible to know the OGTT results of all women included in the nationwide database, defining GDM by ICD-10 code might be inevitable. Actually, in Korea, many clinicians input GDM codes to avoid pushback by the national insurance service when they prescribe an OGTT test, so the prevalence of GDM might have been overestimated in Yoo et al.’s study [20]. To overcome this bias, we defined patients with GDM as those who visited the outpatient clinic more than twice with GDM codes. In this study, the prevalence rates of GDM stratified by the number of outpatient clinic visits (i.e., >1 time, 2 times, or 3 times) were 36.53%, 12.71%, and 5.73%, respectively (Supplemental Table S7). The characteristics of women with GDM showed similar trends in all three scenarios. Considering the characteristics of NHID in Korea, we thought that defining GDM based on visiting the outpatient clinic more than two times with GDM codes was reasonable.
The global prevalence of GDM in 2005 to 2018 according to the literature ranged from 15.2% (Middle East and North Africa) to 6.1% (Europe) [6]. Meanwhile, the group of Western Pacific countries to which Korea belongs has exhibited a median prevalence rate of 10.3%. Because ethnicity is not homogenous in this geographic area, prevalence rates varied from 4.5% in Japan to 18.0% in Singapore. Although it is difficult to compare prevalence rates across countries directly, we identified that the prevalence of GDM in Korea was higher, above the median of that reported for Western Pacific countries. As compared with in other Western Pacific countries, the rapid increase in maternal age at delivery might have triggered a higher prevalence of GDM in Korea [22].
In this study, many well-known risk factors for GDM were identified in Korean pregnant women similarly to as in other studies. Advanced maternal age is a representative risk factor [6, 23]. In a prospective cohort study (n=14,613), American women older than 40 years of age had a greater than two-fold higher risk of GDM as compared with those younger than 30 years of age (prevalence: 9.8% vs. 4.1%) [23]. In our study, we noted that the prevalence rate of GDM in Korean pregnant women was 15.57% among those aged 30 to 34 years and 22.46% among those older than 40 years. In addition, women in our study who were 30 years and 40 years or older were at 3.178 and 4.827 times greater risk, respectively, of GDM than those who were younger than 25 years, following adjustment for confounding factors. Obesity before pregnancy is also an important risk factor [6,23]. The relative risk value for GDM in a population of American women was 2.90 (95% CI, 2.15 to 3.91) for those with BMI values of at least 30 kg/m2 [23]. Korean women with prepregnancy BMI values of at least 30 kg/m2 were at 1.935 times greater risk for GDM and those with WC of 80 to 85 cm were at 1.107 times greater risk for the same after adjustment for covariates. The reason for why the risk of obesity triggering GDM was lower among Korean women relative to American women is not clear, although it may be because Korean women tend to be less obese and have lower visceral adiposity than Western women. Impaired fasting glucose was associated with an elevated risk of GDM by 2.4 times (FPG level: 110 to 125 mg/dL). High income, drinking, and smoking were associated with GDM as well. Finally, the regional prevalence of GDM in Korea ranged from 4.59% (Gwangju) to 27.86% (Sejong). We only elucidated the GDM prevalence rate of each region in this study, so further investigations are needed to clarify the causes of such regional differences. Overall, the prevalence rate and OR for GDM in Korean women were higher than those for women of other ethnic groups and are compatible with the findings of previous studies suggesting Asian women are at higher risk for GDM [6].
We do not yet know exactly why Korean women are at high risk for GDM. One possible explanation is that Korean women have relatively low insulin secretory function [24–26]. In general, GDM is developed when insulin secretion does not adequately compensate for increased insulin resistance during pregnancy [1,6]. In a 10-year follow-up study of 4,106 Korean participants with normal glucose tolerance, impaired β-cell compensation for a progressive decline in insulin sensitivity was a crucial factor in the deterioration of glucose tolerance [26]. Many Korean pregnant women may not have sufficient compensatory insulin secretory function to overcome the increased insulin resistance inherent during pregnancy.
Several limitations should be considered when interpreting the results of this study. First, the definition of GDM was not based on the OGTT results but instead ICD-10 codes and the number of outpatient clinic visits. It might influence that the prevalence of GDM seems to be higher than expected. Furthermore, we could not have evaluated the association between various glucose indices and the risk of GDM. Second, we could not discern how many women were diagnosed using a one-step or two-step approach. Because the prevalence of GDM increased in other countries following adoption of the one-step approach, we could have evaluated the effect of the adoption of the one-step approach if we have known the proportion of women diagnosed with each approach. Third, because we excluded women with pregestational diabetes based on only FPG criteria, we could not figure out pregestational diabetes diagnosed by HbA1c or 75-g OGTT criteria. Therefore, some women with pregestational diabetes might have been included. Lastly, we were not able to obtain information regarding previous history of GDM, pregnancy outcomes, neonatal outcomes, and postpartum follow-up due to the characteristics of the database we used. This study, however, may be valuable because it evaluated the prevalence of GDM and the association between the risk factors and GDM using Korean national-level data.
In conclusion, the prevalence of GDM in Korean women increased up to 12.70% in the period of 2011 to 2015. Advanced maternal age, prepregnancy BMI, WC, FPG, high income, smoking, and drinking were associated with an increased risk for GDM. These data suggest the importance of GDM screening and prevention in high-risk groups in Korea.
Baseline Characteristics of Study Participants According to Age
Baseline Characteristics of Study Participants According to Prepregnancy Body Mass Index
Baseline Characteristics of Study Participants According to Waist Circumference
Baseline Characteristics of Study Participants According to Fasting Plasma Glucose
Incidence Rate and Odds Ratios for Gestational Diabetes Mellitus According to Age, Prepregnancy Body Mass Index, Waist Circumference, and Fasting Plasma Glucose
Odds Ratios for Gestational Diabetes Mellitus Based on Location
Baseline Characteristics of Study Participants According to the Number of Outpatient Clinic Visits
ACKNOWLEDGMENTS
This work was supported by the Korean Endocrine Society of EnM Research Award 2019. This study used the National Health Insurance Service (NHIS) Database (NHIS-2020-1-093).
REFERENCES
1. American Diabetes Association. 2. Classification and diagnosis of diabetes: standards of medical care in diabetes-2020. Diabetes Care. 2020; 43:S14–31.
2. Kim KS, Park SW, Cho YW, Kim SK. Vitamin D deficiency at mid-pregnancy is associated with a higher risk of postpartum glucose intolerance in women with gestational diabetes mellitus. Endocrinol Metab (Seoul). 2020; 35:97–105.


3. Falcone V, Kotzaeridi G, Breil MH, Rosicky I, Stopp T, Yerlikaya-Schatten G, et al. Early assessment of the risk for gestational diabetes mellitus: can fasting parameters of glucose metabolism contribute to risk prediction? Diabetes Metab J. 2019; 43:785–93.


4. Morettini M, Castriota C, Gobl C, Kautzky-Willer A, Pacini G, Burattini L, et al. Glucose effectiveness from short insulin-modified IVGTT and its application to the study of women with previous gestational diabetes mellitus. Diabetes Metab J. 2020; 44:286–94.


5. HAPO Study Cooperative Research Group. Metzger BE, Lowe LP, Dyer AR, Trimble ER, Chaovarindr U, et al. Hyperglycemia and adverse pregnancy outcomes. N Engl J Med. 2008; 358:1991–2002.


6. Zhu Y, Zhang C. Prevalence of gestational diabetes and risk of progression to type 2 diabetes: a global perspective. Curr Diab Rep. 2016; 16:7.


7. Cho GJ, Park JH, Lee H, Yoo S, Shin SA, Oh MJ. Prepregnancy factors as determinants of the development of diabetes mellitus after first pregnancy. J Clin Endocrinol Metab. 2016; 101:2923–30.


8. Kramer CK, Campbell S, Retnakaran R. Gestational diabetes and the risk of cardiovascular disease in women: a systematic review and meta-analysis. Diabetologia. 2019; 62:905–14.


9. Tobias DK, Stuart JJ, Li S, Chavarro J, Rimm EB, Rich-Edwards J, et al. Association of history of gestational diabetes with long-term cardiovascular disease risk in a large prospective cohort of US women. JAMA Intern Med. 2017; 177:1735–42.


10. McIntyre HD, Catalano P, Zhang C, Desoye G, Mathiesen ER, Damm P. Gestational diabetes mellitus. Nat Rev Dis Primers. 2019; 5:47.


11. American Diabetes Association. 14. Management of diabetes in pregnancy: standards of medical care in diabetes-2020. Diabetes Care. 2020; 43:S183–92.
12. Hedderson MM, Darbinian JA, Quesenberry CP, Ferrara A. Pregravid cardiometabolic risk profile and risk for gestational diabetes mellitus. Am J Obstet Gynecol. 2011; 205:55.


13. Hedderson M, Ehrlich S, Sridhar S, Darbinian J, Moore S, Ferrara A. Racial/ethnic disparities in the prevalence of gestational diabetes mellitus by BMI. Diabetes Care. 2012; 35:1492–8.


14. Oh TJ, Kim YG, Kang S, Moon JH, Kwak SH, Choi SH, et al. Oral glucose tolerance testing allows better prediction of diabetes in women with a history of gestational diabetes mellitus. Diabetes Metab J. 2019; 43:342–9.


15. Kang S, Kim MH, Kim MY, Hong JS, Kwak SH, Choi SH, et al. Progression to gestational diabetes mellitus in pregnant women with one abnormal value in repeated oral glucose tolerance tests. Diabetes Metab J. 2019; 43:607–14.


16. Kim MH, Kwak SH, Kim SH, Hong JS, Chung HR, Choi SH, et al. Pregnancy outcomes of women additionally diagnosed as gestational diabetes by the International Association of the Diabetes and Pregnancy Study Groups Criteria. Diabetes Metab J. 2019; 43:766–75.


17. Hong S, Lee SM, Kwak SH, Kim BJ, Koo JN, Oh IH, et al. A comparison of predictive performances between old versus new criteria in a risk-based screening strategy for gestational diabetes mellitus. Diabetes Metab J. 2020; 44:726–36.


18. Korean Diabetes Association. Diabetes fact sheet in Korea 2013 [Internet]. Seoul: Korean Diabetes Association;2013. [cited 2021 May 4]. Available from: https://www.diabetes.or.kr/pro/news/admin.php?category=A&code=admin&mode=view&number=1426
.
19. Koo BK, Lee JH, Kim J, Jang EJ, Lee CH. Prevalence of gestational diabetes mellitus in Korea: a national health insurance database study. PLoS One. 2016; 11:e0153107.


20. Yoo HJ, Choi KM, Baik SH, Park JH, Shin SA, Hong SC, et al. Influences of body size phenotype on the incidence of gestational diabetes needing prescription; analysis by Korea National Health Insurance (KNHI) claims and the National Health Screening Examination (NHSE) database. Metabolism. 2016; 65:1259–66.


21. International Association of Diabetes and Pregnancy Study Groups Consensus Panel. Metzger BE, Gabbe SG, Persson B, Buchanan TA, Catalano PA, et al. International Association of Diabetes and Pregnancy Study Groups recommendations on the diagnosis and classification of hyperglycemia in pregnancy. Diabetes Care. 2010; 33:676–82.


22. Statistics Korea. Birth statistics in 2019 [Internet]. Daejeon: Statistics Korea;2020. [cited 2021 May 4]. Available from: http://kostat.go.kr/portal/eng/pressReleases/8/10/index.board?bmode=read&bSeq=&aSeq=385158&pageNo=1&rowNum=10&navCount=10&currPg=&searchInfo=&sTarget=title&sTxt=
.
23. Solomon CG, Willett WC, Carey VJ, Rich-Edwards J, Hunter DJ, Colditz GA, et al. A prospective study of pregravid determinants of gestational diabetes mellitus. JAMA. 1997; 278:1078–83.


24. Moon J, Kim JY, Yoo S, Koh G. Fasting and postprandial hyperglycemia: their predictors and contributions to overall hyperglycemia in Korean patients with type 2 diabetes. Endocrinol Metab (Seoul). 2020; 35:290–7.


Fig. 1
Prevalence of gestational diabetes mellitus according to age (A), prepregnancy body mass index (B), waist circumference (C), and fasting plasma glucose (D) category.
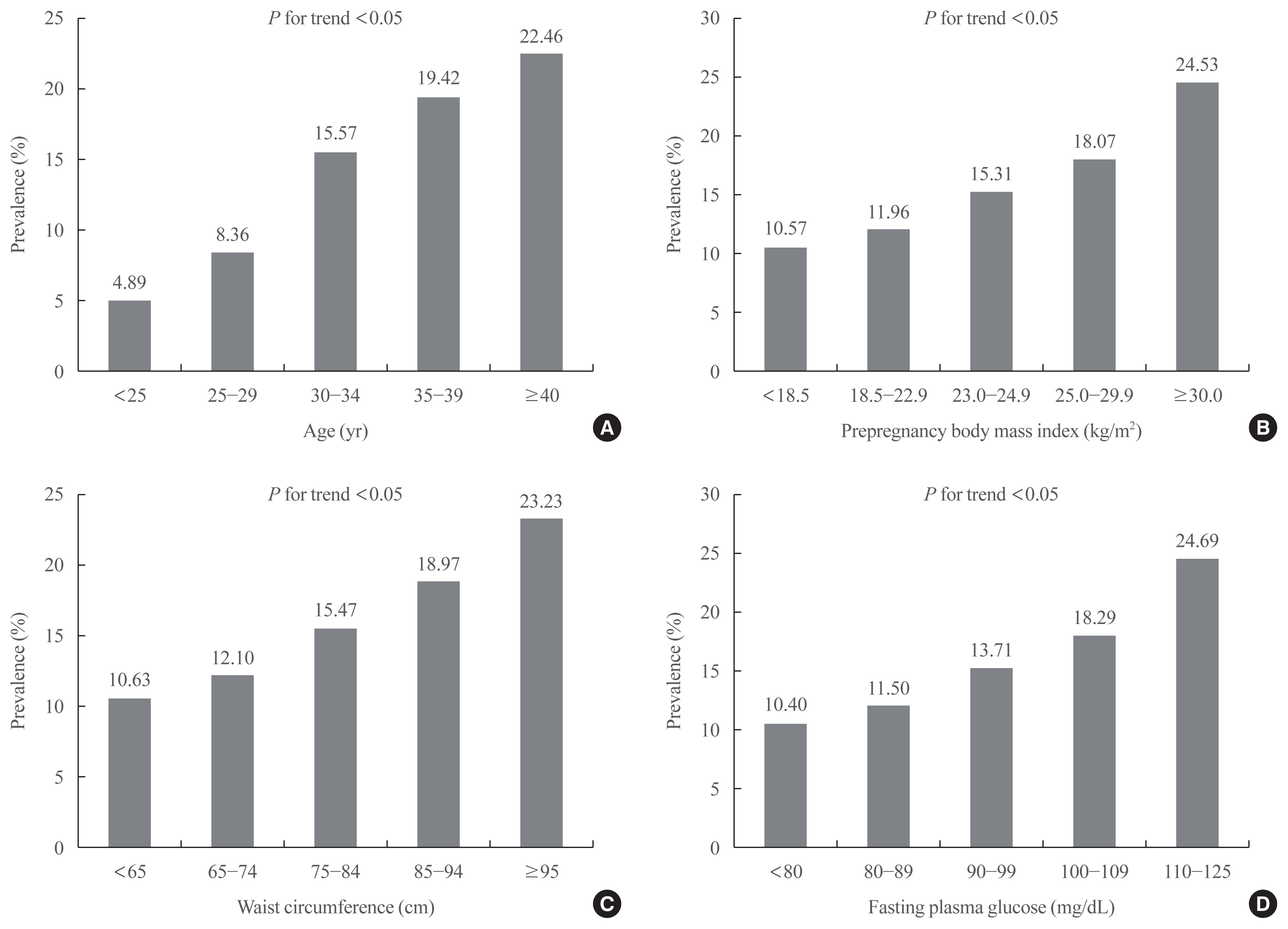
Table 1
Baseline Characteristics of Study Participants
Table 2
Odds Ratios for Gestational Diabetes Mellitus According to Age, Prepregnancy Body Mass Index, Waist Circumference, Fasting Plasma Glucose, and Income
Variable | No. of patients | No. of event | Odds ratio (95% confidence interval) | |
---|---|---|---|---|
|
||||
Univariate | Multivariatea | |||
Age, yr | ||||
<25 | 20,630 | 1,009 | 1 (Reference) | 1 (Reference) |
25–29 | 165,534 | 13,841 | 1.773 (1.660–1.893) | 1.771 (1.658–1.892) |
30–34 | 182,149 | 28,368 | 3.584 (3.360–3.823) | 3.439 (3.222–3.671) |
35–39 | 38,547 | 7,484 | 4.681 (4.373–5.011) | 4.255 (3.971–4.559) |
≥40 | 10,279 | 2,309 | 5.629 (5.204–6.088) | 4.804 (4.436–5.203) |
|
||||
Prepregnancy body mass index, kg/m2 | ||||
<18.5 | 64,604 | 6,829 | 1 (Reference) | 1 (Reference) |
18.5–22.9 | 272,760 | 32,618 | 1.149 (1.118–1.181) | 1.043 (1.012–1.075) |
23.0–24.9 | 43,525 | 6,663 | 1.529 (1.475–1.586) | 1.226 (1.176–1.279) |
25.0–29.9 | 30,837 | 5,573 | 1.866 (1.796–1.939) | 1.383 (1.317–1.452) |
≥30.0 | 5,413 | 1,328 | 2.751 (2.573–2.941) | 1.898 (1.736–2.075) |
|
||||
Waist circumference, cm | ||||
<65 | 92,878 | 9,871 | 1 (Reference) | 1 (Reference) |
65–74 | 231,120 | 27,969 | 1.158 (1.130–1.186) | 1.032 (1.005–1.060) |
75–84 | 75,188 | 11,630 | 1.539 (1.495–1.584) | 1.117 (1.078–1.157) |
85–94 | 14,772 | 2,802 | 1.968 (1.880–2.061) | 1.142 (1.077–1.212) |
≥95 | 3,181 | 739 | 2.545 (2.338–2.770) | 1.158 (1.039–1.290) |
|
||||
Fasting plasma glucose, mg/dL | ||||
<80 | 76,790 | 7,987 | 1 (Reference) | 1 (Reference) |
80–89 | 174,346 | 20,051 | 1.119 (1.089–1.151) | 1.078 (1.048–1.108) |
90–99 | 128,183 | 17,578 | 1.369 (1.331–1.408) | 1.269 (1.233–1.306) |
100–109 | 30,359 | 5,553 | 1.928 (1.858–2.001) | 1.714 (1.649–1.780) |
110–125 | 7,461 | 1,842 | 2.824 (2.666–2.991) | 2.483 (2.340–2.636) |
|
||||
Income | ||||
Medical aid beneficiaries | 17,809 | 2,351 | 1 (Reference) | 1 (Reference) |
Q1 | 68,009 | 7,967 | 0.935 (0.890–0.982) | 1.036 (0.985–1.090) |
Q2 | 139,934 | 16,380 | 0.934 (0.892–0.978) | 1.095 (1.044–1.147) |
Q3 | 144,292 | 19,139 | 1.078 (1.029–1.128) | 1.150 (1.098–1.205) |
Q4 | 45,987 | 7,174 | 1.303 (1.239–1.369) | 1.198 (1.138–1.261) |
Table 3
Odds Ratios for Gestational Diabetes Mellitus According to Drinking, Smoking, and Regular Exercise Status
Variable | No. of patients | No. of event | Incidence rate, % | Odds ratioa (95% CI) |
---|---|---|---|---|
Drinking | ||||
None | 223,340 | 28,954 | 12.96 | 1 (Reference) |
Mild (≤30 g/day) | 185,868 | 23,098 | 12.43 | 1.024 (1.004–1.043) |
Heavy (>30 g/day) | 7,931 | 959 | 12.09 | 1.043 (0.972–1.120) |
|
||||
Smoking status | ||||
Nonsmoker | 387,087 | 48,805 | 12.61 | 1 (Reference) |
Ex-smoker | 15,097 | 2,197 | 14.55 | 1.115 (1.063–1.169) |
Current smoker | 14,955 | 2,009 | 13.43 | 1.087 (1.035–1.143) |
|
||||
Regular exercise | ||||
No | 376,823 | 47,658 | 12.65 | 1 (Reference) |
Yes | 40,316 | 5,353 | 13.28 | 1.030 (0.999–1.062) |