Abstract
Background
The advancement of information technology has immensely increased the quality and volume of health data. This has led to an increase in observational study, as well as to the threat of privacy invasion. Recently, a distributed research network based on the common data model (CDM) has emerged, enabling collaborative international medical research without sharing patient-level data. Although the CDM database for each institution is built inside a firewall, the risk of re-identification requires management. Hence, this study aims to elucidate the perceptions CDM users have towards CDM and risk management for re-identification.
Methods
The survey, targeted to answer specific in-depth questions on CDM, was conducted from October to November 2020. We targeted well-experienced researchers who actively use CDM. Basic statistics (total number and percent) were computed for all covariates.
Results
There were 33 valid respondents. Of these, 43.8% suggested additional anonymization was unnecessary beyond, “minimum cell count” policy, which obscures a cell with a value lower than certain number (usually 5) in shared results to minimize the liability of re-identification due to rare conditions. During extract-transform-load processes, 81.8% of respondents assumed structured data is under control from the risk of re-identification. However, respondents noted that date of birth and death were highly re-identifiable information. The majority of respondents (n = 22, 66.7%) conceded the possibility of identifier-contained unstructured data in the NOTE table.
Conclusion
Overall, CDM users generally attributed high reliability for privacy protection to the intrinsic nature of CDM. There was little demand for additional de-identification methods. However, unstructured data in the CDM were suspected to have risks. The necessity for a coordinating consortium to define and manage the re-identification risk of CDM was urged.
Graphical Abstract
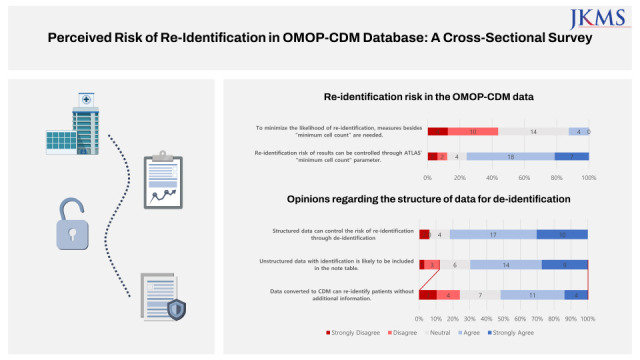
The advancement of information technology has immensely increased the volume, if not always the quality, of health data. This has led to an increase in the number of observational studies, as well as to the threat of privacy invasion.12 Recently, a distributed research network (DRN) based on the common data model (CDM) has emerged, enabling collaborative international medical research without sharing patient-level data.3 Researchers leverage the distributed research network based on the common data model to avoid limitations for international collaborative healthcare research. For network study based on CDM, an end-to-end study package for the entire analytic process should be built. This package can be executed locally inside a firewall for each CDM database. Then, tabular results excluding patient-level information can be shared for interpretation and database-level meta-analysis.4
Nonetheless, there are five limitations that induce disconnection and impede collaboration across networks or countries: 1) variance in governance policies and participation requirements between networks; 2) the lack of mechanism for broadcasting research capabilities or encouraging accessibility among participants; 3) a lack of security and reliability between networks for data requests and tracking response activity; 4) the absence of operational standards for describing data, which could allow for judgement of fitness-for-use of others’ data sources; and 5) the unavailability of reliable mechanisms for executing queries sent across networks.5
Although the CDM database for each institution is built inside a firewall, the risk of re-identification requires management because of risks of data leakage and misuse or overuse of data by inside researchers. Currently, CDM databases are accessible only to authorized researchers within the institution due to security concerns.6 Tools in CDM become more advanced as practical uses of CDM increases. The unspecified number of users accessing CDM increases alongside its total use. Although the distributed research network based on CDM shares only tabular data resulting from large-scale analytics outside the firewalls of participating institutions within the network, this does not strictly mean that privacy of patients is guaranteed, given the existence of the large-scale database within the institution. Hence, management of re-identification risks using CDM is essential for each institution. Therefore, this study will offer insight on which direction the governance of re-identification should follow.
The main objective of this survey was to investigate the risk of re-identification when using an Observational Medical Outcomes Partnership (OMOP)-CDM database, as well as the risk management demands of database users. We planned to recruit reliable and verified researchers familiar with OMOP-CDM with anonymity. To achieve this aim, the survey only targeted researchers working on Korean government-funded projects for OMOP-CDM. The survey collected responses by emailing researchers a link to the online survey. The survey was organized and distributed through Google Forms from October 30 to November 14, 2020, informing participants at the preface about both the objective and goals of the study. To avoid duplication, participants were asked for their phone number during introduction to the survey. These responses were submitted anonymously. Participation was voluntary since responders were provided sufficient knowledge of the survey’s intention, implying informed consent. Questionnaire materials did not present or cause any harm to participants.
The demands examined were: 1) consistent requirement levels for additional de-identification 2) application of OMOP-CDM to individual de-identification processing and verification methods (International Organization for Standardization [ISO] 20889 standard)7; 3) requirement entities for establishment and management of de-identification processing criteria 4) opinions for mid- to long-term management plans, such as update cycles, development, and compliance with guidelines. To define contents of the survey, we reviewed OMOP-CDM data samples with two data de-identification and data security experts (GTK, SSK) to examine the data categories likely to contain identification information. A draft of the survey was first developed by a team of researchers and primary modifications were made after consulting experts. Following subsequent expert reviews, secondary modifications were made with survey content following ISO 25237.8 The survey was cross-sectional and structured into several sub-sections beginning with a statement of procedures and intentions. Additional nine expert advices were given for enhancing the quality of the survey. The nine experts involved were at a level, research associate for the CDM-related national projects, where they reviewed the content of the survey.
The questionnaire first asked participants about their experience with OMOP-CDM utilization before requesting general information about each participant. Next, questions focused on re-identification risk regarding extract, transform, and load (ETL) processes and how this risk could be controlled in OMOP-CDM usage environments through unique features that remove identification information. Subsequent items assessed the necessity for applying de-identification methods based on ISO. Lastly, items asked for verification of results from identification information processing and mid-to-long-term strategies for maintaining minimal risk of de-identification. A 5-point Likert scale or nominal scale was used where appropriate to qualify responses.
OMOP-CDM-based research usually employs tabular data to summarize research results across data partners without sharing patient-level microdata. Aggregated tabular data has a generally low risk of re-identification, but this may not be sufficient to protect privacy. If a cell value in a table is 1, it is possible to re-identify one individual with a specific value or medical history.9 Hence, the usual policy of OMOP-CDM study restricts the minimum cell count to 5, meaning if the value of a cell is below 5, the value is masked. For example, if a cell has a value of 4 describing a number of patients with certain medical condition, then the cell was masked as ‘< 5’. Further, we asked whether this function could be effective in controlling the risk of re-identification of a subject.
For de-identification process criteria, “date information (mean)” suggests averages for: 1) patient’s date of birth; 2) dates of hospitalization, discharge, or surgery; 3) the start and end of a visit; and 4) dates for tests, body measurements, dosing, etc. The criteria “personal information related to patients” includes family history and information about acquaintances. The term “test ID” refers to the unique identification number for a procedure or measurement (e.g., blood test or pathological diagnosis) in a medical narrative that is usually stored in the NOTE table. The definition of “rare disease” was assumed to vary since common rare diseases differ depending on the size of the medical institution.
We included the concept of systemic feedback given to the user according to degree of re-identification risk (e.g., “traffic light system”) in the questionnaire. For example, “Red (Danger)” indicates the “risk of subject re-identification in calculated results is high, denoting the need to prove that the request is not interrupted and/or that it is not an intentional re-identification attack.” “Yellow (Warning)” means “the risk of subject re-identification in calculated results is moderate, so a review of the security manager or the IRB may be necessary for the process to proceed.”
The survey recruited a sample of 34 individuals. Since one respondent did not meet selection criteria, 33 respondents with clinical data experience in OMOP-CDM were selected. Respondents comprised 24 employees from medical institutions and nine from non-medical institutions. The majority of the former (n = 22, 91.7%) worked at tertiary hospitals as physicians, while the remainder worked at general hospitals (n = 2, 8.3%) as physicians. The respondents worked in the following fields: data analysis (n = 25, 75.8%), database management (n = 15, 45.5%), clinical research (n = 13, 39.4%), and application/ tool development (n = 11, 33.3%). Twenty-seven (81.8%) respondents had experience using CDM for over one year, and 11 (33.3%) users had more than five years of experience.
About half (n = 14, 43.8%) of participants thought the “minimum cell count” policy would be enough to minimize liability of re-identification (Fig. 1). Furthermore, over 75% (n = 25, 75.6%) agreed that from ATLAS, a web interface with integrated features from numerous Observational Health Data Sciences and Informatics (OHDSI) applications with search and navigation capabilities,10 “minimum cell count” parameter could control the risk of re-identification in the results (Fig. 1). OHDSI is an international collaborative which grew out of OMOP, where it aims to establish open-source data analytic solutions to health databases network.11
CDM users noted that in the measurement table, value_source_value was mentioned in relation to the high risk of re-identification from inclusion of source data. The code value_source_value represents a measurement source value in the measurement table. Since value_source_value is an unstructured field with measurements, a data quality check was unavailable.12
Over 75% (n = 27, 81.8%) of respondents admitted that removing or processing direct identifiers in structured data during ETL processes could control the risk of re-identification (Fig. 2). Additionally, about 70% (n = 23, 69.7%) admitted unstructured data (free text in OMOP-CDM) with identifying information would be included in the NOTE table of OMOP-CDM (Fig. 2). Lastly, among those who chose “neutral,” “agree,” or “strongly agree” (n = 29) regarding the previous question, just over half (n = 15, 51.7%) believed data converted to CDM could be re-identified without additional information (Fig. 2). Respondents who answered “agree” or “strongly agree” when asked if data converted to OMOP-CDM could be used to re-identify patients without additional information were asked which information from OMOP-CDM’s domain had the highest likelihood of re-identification (Fig. 3). Both birth date and death date were cited as having the highest (n = 10, 31.3% each) risk of re-identifiable information.
Fig. 4 portrays the diverse values collected since determination of whether those values could lead to identification is essential. Supplementary Fig. 1 includes a bar chart showing the distribution of information for each date. Over half of participants believed standards for date information should be defined by the Korean OHDSI coordinating consortium (n = 17.5, 53.0%). For the criteria of “rare disease,” “measurements/ extreme values,” and “personal information related to patients,” just over half asserted this consortium should define consistent standards (n = 19, 57.6%; n = 20, 60.6%; and n = 18, 54.5%), while less than half (n = 15, 45.5%) under “test ID” agreed this consortium should define standards and a similar number (n = 14, 42.4%) asserted that standards should be defined by individual institutions. Supplementary Fig. 2 includes remaining criteria for the survey.
Various methods are available for strengthening the de-identification ability of data. Responses for all individual de-identification methods available from the survey are available in Supplementary Fig. 3. Fig. 5 depicts seven methods specifically chosen for generalizing responses about additional methods. Except for data reduction (n = 16, 50%), over half of respondents either disagreed or strongly disagreed with additional individual de-identification methods such as: aggregation (n = 22, 68.8%), cryptographic tools (n = 21, 65.6%), masking (n = 17, 53.1%), pseudonymization (n = 18, 56.3%), randomization (n = 25, 78.1%), and rounding (n = 21, 65.6%).
There are numerous ways to process measures and countermeasures when verifying identifying information (Fig. 6). For privacy protection models such as k-anonymity, I-diversity, and others (T-adjacent, M-invariant), over half of participants admitted that when identification removal procedures for ETL processes have no re-identification risk, further consideration is unnecessary (n = 16, 51.6%; n = 17, 53.1%; and n = 17, 53.1%, respectively). On the other hand, regarding the traffic light system, most people wanted the consortium to set consistent standards (n = 13, 40.6%; n = 15, 50%, respectively).
Discussions of mid-to long- term strategies are depicted in Fig. 7. The two periods of monitoring and updating anonymization guidelines were 6 months and 1-year (n = 23, 74.2% for both cases).
OMOP-CDM users overall perceived high credibility of OMOP-CDM’s de-identification ability. Moreover, additional de-identification methods were suspected to be unnecessary for OMOP-CDM. The “minimum cell count” parameter was believed to be an effective method for minimizing re-identification risk. During the ETL process, structured data were trusted to control re-identification risk; however, unstructured data in the NOTE table were suspected to present a risk for re-identification.
We identified perceived risk for re-identification when using OMOP-CDM databases from experts in medical informatics. These experts agreed that “minimum cell count” as a parameter in shared tabular data presents a powerful strategy for further protecting against re-identification risk. The level of de-identification for structured data in OMOP-CDM was perceived as strongly trustworthy given that the majority of respondents (81.8%) agreed there was no additional concern of re-identification. However, 69.7% of respondents showed concern that unstructured data may contain identifiable data, as presented in the NOTE table. Furthermore, together with OHDSI team, Pfaff et al. reported that personally identifiable information (PII) may exist even in the structured data as the values of certain SNOMED-CT or LOINC codes, such as in the values for 42077-8 of LOINC code (Patient home phone) or 394571004 of SNOMED (“Employer”).13 More research to evaluate the potential risk for leakage of PIIs and solutions to protect this in OMOP-CDM should be conducted and implemented.
Respondents assumed most criteria for de-identification were best defined by the coordinating consortium. However, 42.4% chose “test unique numbers,” indicating that standards should be individually chosen by institution, a considerably high proportion compared to other criteria. Also, most additional individual de-identification methods were deemed unnecessary. Aggregation, cryptographic tools, masking, pseudonymization, and rounding scored 50–70% negative opinions, suggesting a pessimistic view towards additional de-identification methods. However, randomization showed 78.1% of participants had negative opinions towards de-identification ability, a strong contrast to other tools.
Requests for anonymization or masking were made regarding the NOTE table and removal of specific identifiers. Some opined that since studies using OMOP-CDM data ordinarily have common subjects, rather than patients with rare disease, extreme values are improbable. For inclusion, however, the consortium must define standard processes. Hence, at the ETL stage, consistent criteria for the degree of filtration of patient identification is lacking.
Standardization of healthcare data confers scalability on collaborative research.14 Through comparison of various OMOP-CDMs through criteria like completeness, integrity, flexibility, simplicity, integration, and implementation, OMOP-CDM had the overall highest rank accommodating large numbers of data elements and broadest terminology coverage in EHR.15 Furthermore, the ATLAS-based OMOP-CDM only provided users with macro data derived from queries as the environment cannot accommodate microdata. As a result, it is possible to argue that the OMOP-CDM usage environment can minimize re-identification risk, considering the data subject for OMOP-CDM use has been de-identified through statistical tools. However, OMOP-CDM faces a limit where, for intended attacks, the re-identification risk could be very high. Therefore, additional research on criteria is required to maintain a secure CDM environment in the face of intended attacks.
To increase the security of OMOP-CDM and maintain a safe environment, additional privacy protection models should be considered. Identified privacy protection models (including K-anonymity, I-diversity, T-adjacent, M-invariant, and others) were not assessed as causing re-identification problems, with just over 50% of participants feeling no need for additional consideration. For the implementation of privacy models, k-anonymity, l-diversity, and t-closeness were evaluated for each table, with the t-closeness model having the most effective anonymity. The previous study has demonstrated that privacy in the OMOP-CDM database can be easily enhanced by masking miscellaneous columns when assessing privacy risk assessed by K-anonymity, I-diversity, and T-closeness.6 For traffic light systems, “danger” and “warning” had 75% and 80%, respectively, requiring that standards be defined by the consortium or an authority. Since additional privacy models were not needed when traffic light systems were used, this suggests that people wanted a warning system for current status rather than an extra model for security.
There were suggestions that since IRB governs each institutions’ research, de-identification criteria should be defined independently by the institution, or, alternatively, that guidelines should be defined by government standards with a consortium noting problems for supplementation. Consequently, most respondents were negative about applying additional de-identification techniques or verification methodologies but demands for individual methodologies were high. Users demanded a dependable institution establish universal standards due to low self-reliability leading to the possibility of withholding judgment.
When monitoring OMOP-CDM data and managing anonymization guidelines, both were preferred to last either 6 months or one year. Since projects are usually scheduled annually, guidelines may change often, causing discomfort. If guidelines are monitored and managed yearly, fewer projects would need to frequently address their anonymization criteria. Therefore, among the various tasks respondents assigned to the consortium were providing communication channels for standards and guides, collecting opinions, and creating parameters to make multi-organizational study more efficient. Protocols for re-identification risk management and alerting users, such as the traffic light system, should be managed by collaboration between institution, IRB committee, and consortium. The application of anonymous processing levels is differentiated according to OMOP-CDM usage environment and the researcher's credibility and is believed to be presently sufficient.
To our knowledge, this is the first study investigating the current state of OMOP-CDM and its development as suggested by actual users. The most severe limitation faced by this research, the limited number of survey respondents, was due to the small subject pool. Hence, the survey may have been ambiguous and the responders may have heterogeneous experience in CDM. Although studies using OMOP-CDM are being actively conducted in Korea, it is impossible to determine the population of researchers having sufficient experience. Therefore, we targeted researchers working on Korean government-funded projects for OMOP-CDM to secure the expertise of our respondents. Another factor which could cause bias was the existence or absence of a unique data warehouse for each hospital. Hospitals with sufficient infrastructure to maintain a unique data warehouse may suggest less necessity for the use of OMOP-CDM, although the opposite would not be true. Even though this research identified a risk of re-identification of information and a time-series analysis, we were unable to present specified solutions for resolving related issues. For those who participated in this study through the OHDSI Korea website forum, we were unable to determine how expertise was related to inclusion criteria. A minor limitation was presented due to similarity between additional privacy protection models, as respondents had little understanding or ability to distinguish between them. However, this meaningful study can be extended to global survey study in the future among OMOP-CDM experts.
To conclude, OMOP-CDM users gave high reliability to OMOP-CDM’s de-identification ability, suggesting additional de-identification as unessential. In the course of the ETL process, users desired that a consortium define standards of criteria due to current re-identification risks. Consequently, to maintain a low re-identification risk in an unstructured text or time-series, which normally has a high risk of re-identification, regular management as determined by the consortium was suspected as vital. In normal CDM use environments, re-identification risk may be low; however, it is still vulnerable to intentional attacks. Thus, supplementary investigation is mandatory for preventing re-identification during identified attacks.
ACKNOWLEDGMENTS
We would like to express gratitude to all the respondents who participated in this study and MS. SeungHyun Chung for assisting in the process of publishing this paper.
Notes
References
1. Benson K, Hartz AJ. A comparison of observational studies and randomized, controlled trials. N Engl J Med. 2000; 342(25):1878–1886. PMID: 10861324.


2. Keshta I, Odeh A. Security and privacy of electronic health records: concerns and challenges. Egypt Inform J. 2021; 22(2):177–183.


3. Overhage JM, Ryan PB, Reich CG, Hartzema AG, Stang PE. Validation of a common data model for active safety surveillance research. J Am Med Inform Assoc. 2012; 19(1):54–60. PMID: 22037893.


4. You SC, Rho Y, Bikdeli B, Kim J, Siapos A, Weaver J, et al. Association of ticagrelor vs clopidogrel with net adverse clinical events in patients with acute coronary syndrome undergoing percutaneous coronary intervention. JAMA. 2020; 324(16):1640–1650. PMID: 33107944.


5. Malenfant JM, Hochstadt J, Nolan B, Barrett K, Corriveau D, Dee D, et al. Cross-Network Directory Service: Infrastructure to enable collaborations across distributed research networks. Learn Health Syst. 2019; 3(2):e10187. PMID: 31245605.


6. Jeon S, Seo J, Kim S, Lee J, Kim JH, Sohn JW, et al. Proposal and assessment of a de-identification strategy to enhance anonymity of the observational medical outcomes partnership common data model (OMOP-CDM) in a public cloud-computing environment: anonymization of medical data using privacy models. J Med Internet Res. 2020; 22(11):e19597. PMID: 33177037.


7. International Organization for Standardization. Privacy Enhancing Data De-identification Terminology and Classification of Techniques. Geneva, Switzerland: International Organization for Standardization;2018.
8. International Organization for Standardization. Health Informatics — Pseudonymization. Geneva, Switzerland: International Organization for Standardization;2017.
9. O’Keefe CM, Rubin DB. Individual privacy versus public good: protecting confidentiality in health research. Stat Med. 2015; 34(23):3081–3103. PMID: 26045214.


10. Observational Health Data Sciences and Informatics. ATLAS – a unified interface for the OHDSI tools. Accessed May 2, 2022.
https://www.ohdsi.org/atlas-a-unified-interface-for-the-ohdsi-tools/
.
11. Hripcsak G, Duke JD, Shah NH, Reich CG, Huser V, Schuemie MJ, et al. Observational Health Data Sciences and Informatics (OHDSI): opportunities for observational researchers. Stud Health Technol Inform. 2015; 216:574–578. PMID: 26262116.
12. Khare R, Utidjian LH, Razzaghi H, Soucek V, Burrows E, Eckrich D, et al. Design and refinement of a data quality assessment workflow for a large pediatric research network. EGEMS (Wash DC). 2019; 7(1):36. PMID: 31531382.


13. Pfaff ER, Haendel MA, Kostka K, Lee A, Niehaus E, Palchuk MB, et al. Ensuring a safe(r) harbor: excising personally identifiable information from structured electronic health record data. J Clin Transl Sci. 2021; 6(1):e10. PMID: 35211336.


14. Schneeweiss S, Brown JS, Bate A, Trifirò G, Bartels DB. Choosing among common data models for real-world data analyses fit for making decisions about the effectiveness of medical products. Clin Pharmacol Ther. 2020; 107(4):827–833. PMID: 31330042.


15. Garza M, Del Fiol G, Tenenbaum J, Walden A, Zozus MN. Evaluating common data models for use with a longitudinal community registry. J Biomed Inform. 2016; 64:333–341. PMID: 27989817.


SUPPLEMENTARY MATERIALS
Supplementary Fig. 1
Barchart showing the distribution for each date information.
Supplementary Fig. 3
Distribution of all individual de-identification methods from the survey.