This article has been
cited by other articles in ScienceCentral.
Abstract
Background
The Korea National Antimicrobial Use Analysis System (KONAS), a benchmarking system for antimicrobial use in hospitals, provides Korean Standardized Antimicrobial Administration Ratio (K-SAAR) for benchmarking. This article describes K-SAAR predictive models to enhance the understanding of K-SAAR, an important benchmarking strategy for antimicrobial usage in KONAS.
Methods
We obtained medical insurance claims data for all hospitalized patients aged ≥ 28 days in all secondary and tertiary care hospitals in South Korea (n = 347) from January 2019 to December 2019 from the Health Insurance Review & Assessment Service. Modeling was performed to derive a prediction value for antimicrobial use in each institution, which corresponded to the denominator value for calculating K-SAAR. The prediction values of antimicrobial use were modeled separately for each category, for all inpatients and adult patients (aged ≥ 15 years), using stepwise negative binomial regression.
Results
The final models for each antimicrobial category were adjusted for different significant risk factors. In the K-SAAR models of all aged patients as well as adult patients, most antimicrobial categories included the number of hospital beds and the number of operations as significant factors, while some antimicrobial categories included mean age for inpatients, hospital type, and the number of patients transferred from other hospitals as significant factors.
Conclusion
We developed a model to predict antimicrobial use rates in Korean hospitals, and the model was used as the denominator of the K-SAAR.
Keywords: SAAR, KONAS, Antimicrobial Stewardship, Benchmarking, Korea
INTRODUCTION
Measuring antimicrobial use and feedback is a core element of the antimicrobial stewardship program (ASP), a multidisciplinary activity for inducing proper antimicrobial use and reducing misuse or overuse of antimicrobials.
1 Therefore, it was included as a key component of the Korean National Action Plan on Antimicrobial Resistance from 2016 to 2020.
2
To properly evaluate antimicrobial use in hospitals and set goals for improvement, a benchmarking strategy is necessary. In addition to the direct comparison of antimicrobial use among hospitals, the Centers for Disease Control and Prevention in the US adopted a Standardized Antimicrobial Administration Ratio (SAAR) as a novel benchmarking strategy and provides it via National Healthcare Safety Network.
34 SAAR is calculated by dividing antimicrobial use by predicted antimicrobial use in a hospital; > 1 indicates that the amount of antimicrobial use is greater than the predicted value, and < 1 indicates that the antimicrobial use is less than the predicted value.
4 The value of predicted antimicrobial use reflects the characteristics of each hospital that might influence antimicrobial usage patterns; therefore, it differs among hospitals.
4
In 2021, the Korean Society of Infectious Diseases developed a benchmarking system for antimicrobial use in hospitals—the Korea National Antimicrobial Use Analysis System (KONAS)—in collaboration with the Korean Society for Antimicrobial Therapy, Health Insurance Review & Assessment Service (HIRA), and EvidNet. KONAS provides not only in-depth analysis results of antimicrobial use within each hospital, but also the antimicrobial use of other hospitals or Korean SAAR (K-SAAR) for benchmarking. Through this system, each medical institution can identify its antibiotic usage and patterns, which could help hospitals perform ASP. This article describes K-SAAR predictive models to enhance the understanding of K-SAAR, an important benchmarking strategy for antimicrobial usage in KONAS.
METHODS
Data source
We obtained medical insurance claims data for all hospitalized patients aged ≥ 28 days in all secondary and tertiary care hospitals in South Korea (n = 347) from January 2019 to December 2019 from the HIRA. The data included patients’ age and sex, hospital codes, hospitalization start date, length of hospitalization, diagnosis codes, medical departments in charge, medical costs, intensive care unit utilization during hospitalization, surgery and procedure codes, and prescription medication codes. The discharge diagnoses were coded according to the International Classification of Diseases, Tenth Revision.
A database was constructed to calculate the number of patient-days. We regarded different claims data as the same inpatient episode if all of the following three conditions were satisfied: 1) same recipient, 2) same hospital, and 3) the interval was less than 2 days between the final date of one claims data and the first date of another claims data.
Definitions
Antibiotics and antifungals were defined as medications with Anatomical Therapeutic Chemical (ATC) classes J01 and J02, respectively, and did not include antituberculous agents or antiviral agents. Systemic agents administered via oral or parenteral routes were included, whereas topical agents were excluded. We adopted the classification of antibiotics as previously developed and added some categories after discussion within the research group.
5 Accordingly, the antimicrobial categories for the K-SAAR model were determined as follows: 1) broad-spectrum antibacterial agents predominantly used for hospital-onset infections (including amikacin, cefepime, meropenem, and piperacillin/tazobactam), 2) broad-spectrum antibacterial agents predominantly used for community-acquired infections (including ceftriaxone, ertapenem, ciprofloxacin), 3) antibacterial agents predominantly used for resistant gram-positive bacterial infections (including linezolid, parenteral vancomycin), 4) narrow-spectrum beta-lactam agents (including amoxicillin/clavulanate, nafcillin, cefazolin, cefuroxime), 5) antifungal agents predominantly used for invasive candidiasis (including fluconazole, caspofungin), 6) antibacterial agents predominantly used for extensive antibiotic-resistant gram-negative bacterial infections (including colistin, tigecycline, ceftolozane/tazobactam), 7) carbapenem, 8) fluoroquinolone, 9) metronidazole, and 10) total antibacterial agents (
Supplementary Table 1). Some antimicrobials belong to two or more categories. The consumption of each category of antibiotics or antifungals was converted to a defined daily dose (DDD) following the ATC classification system of the World Health Organization
6 and then standardized per 1,000 patient-days.
Operations were defined as surgical procedures that were included in the ninth nationwide evaluation of the appropriateness of surgical prophylactic antibiotics in Korean hospitals in 2020, led by the HIRA.
7
Predictive modeling for K-SAAR
Modeling was performed to derive a prediction value for antimicrobial use in each institution, which corresponded to the denominator value for calculating K-SAAR. The prediction values of antimicrobial use were modeled separately for each category, for all inpatients and adult patients (aged ≥ 15 years), using stepwise negative binomial regression. The association between annual antimicrobial consumption in each category and factors that were thought to potentially explain the differences in antimicrobial consumption among hospitals was assessed. The independent factors that can influence antimicrobial consumption in the hospital were selected through discussion with the research group. Because an objective of providing K-SAAR is to improve antimicrobial use in each hospital, the factors that could be associated with the characteristics of patients or hospitals were included and those about workforces (e.g., number of infectious diseases specialists) and/or policies (e.g., ASP) were excluded. To obtain enough sample size with standardized data, variables that were available from the medical insurance claims data were finally included; information such as antimicrobial resistance pathogens and the existence of a resident training system was not included. The selected independent factors were as follows: 1) hospital type (tertiary care hospital vs. secondary care hospital), 2) location type (general ward vs. intensive care unit), 3) mean age of inpatients, 4) number of hospital beds, 5) number of operations per year, 6) number of general anesthesia procedures per year, 7) number of patients with malignancy per year, and 8) number of patients transferred from other hospitals per year. Continuous variables were categorized according to a previously reported method.
8
All analyses were conducted using the SAS software (version 9.4; SAS Institute Inc., Cary, NC, USA).
Ethics statement
The study protocol was approved by the Institutional Review Board (IRB) of Yonsei University Health System Clinical Trial Center (IRB No. 4-2019-1297). The requirement for written informed consent from patients was waived.
RESULTS
K-SAAR models were constructed using medical insurance claims data in 2019 from all secondary and tertiary care hospitals with 347 general wards and 298 intensive care units. The variables for the K-SAAR modeling are summarized in
Table 1 and
Supplementary Table 2.
Table 1
Characteristics of hospitals and their inpatients included as data sources for Korean Standardized Antimicrobial Administration Ratio predictive modeling
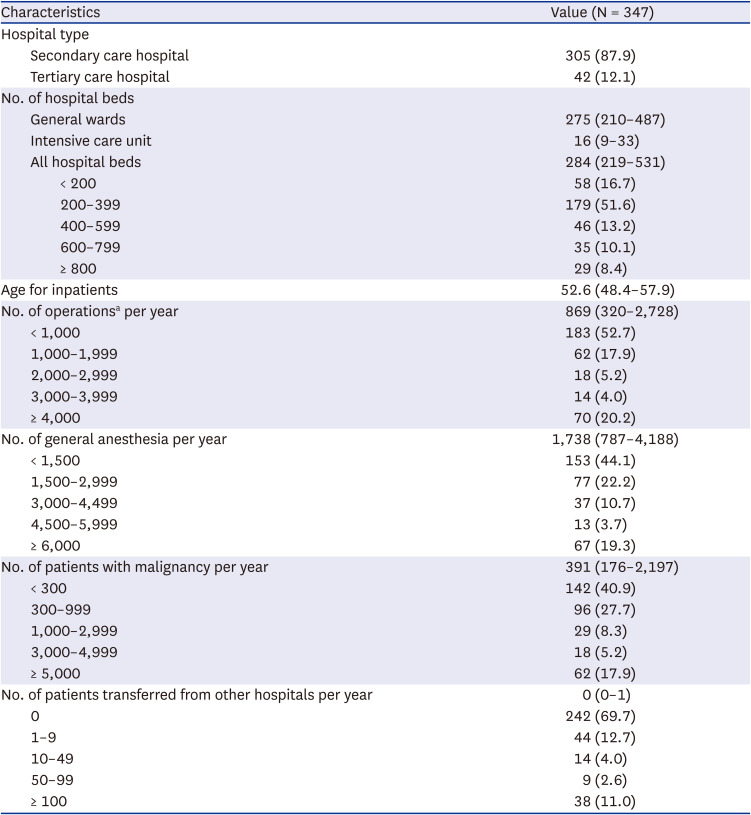
Characteristics |
Value (N = 347) |
Hospital type |
|
|
Secondary care hospital |
305 (87.9) |
|
Tertiary care hospital |
42 (12.1) |
No. of hospital beds |
|
|
General wards |
275 (210–487) |
|
Intensive care unit |
16 (9–33) |
|
All hospital beds |
284 (219–531) |
|
|
< 200 |
58 (16.7) |
|
|
200–399 |
179 (51.6) |
|
|
400–599 |
46 (13.2) |
|
|
600–799 |
35 (10.1) |
|
|
≥ 800 |
29 (8.4) |
Age for inpatients |
52.6 (48.4–57.9) |
No. of operationsa per year |
869 (320–2,728) |
|
< 1,000 |
183 (52.7) |
|
1,000–1,999 |
62 (17.9) |
|
2,000–2,999 |
18 (5.2) |
|
3,000–3,999 |
14 (4.0) |
|
≥ 4,000 |
70 (20.2) |
No. of general anesthesia per year |
1,738 (787–4,188) |
|
< 1,500 |
153 (44.1) |
|
1,500–2,999 |
77 (22.2) |
|
3,000–4,499 |
37 (10.7) |
|
4,500–5,999 |
13 (3.7) |
|
≥ 6,000 |
67 (19.3) |
No. of patients with malignancy per year |
391 (176–2,197) |
|
< 300 |
142 (40.9) |
|
300–999 |
96 (27.7) |
|
1,000–2,999 |
29 (8.3) |
|
3,000–4,999 |
18 (5.2) |
|
≥ 5,000 |
62 (17.9) |
No. of patients transferred from other hospitals per year |
0 (0–1) |
|
0 |
242 (69.7) |
|
1–9 |
44 (12.7) |
|
10–49 |
14 (4.0) |
|
50–99 |
9 (2.6) |
|
≥ 100 |
38 (11.0) |
The models estimated the predicted antimicrobial consumption for a given location (total vs. general ward vs. intensive care unit) and antimicrobial categories. Non-significant factors were not included in the final models, and the final models for each antimicrobial category were adjusted for different significant risk factors. In the K-SAAR models of all aged patients as well as adult patients, most antimicrobial categories included the number of hospital beds and the number of operations as significant factors, while some antimicrobial categories included mean age for inpatients, hospital type, and the number of patients transferred from other hospitals as significant factors. For instance, the statistically significant factors for the model about ‘total antibacterial agents’ for all hospitalized patients (aged ≥ 28 days) were intensive care unit (parameter estimate, 0.306; 95% confidence interval [CI], 0.256, 0.357), mean age of inpatients (parameter estimate, −0.005; 95% CI, −0.008, −0.002), 366–612 hospital beds (parameter estimate, −0.100; 95% CI, −0.166, −0.035), and ≥ 613 hospital beds (parameter estimate, 0.082; 95% CI, 0.016, 0.147). The statistically significant factors for the model about ‘broad-spectrum antibacterial agents predominantly used for hospital-onset infection’ for adult patients (≥ 15 years) were intensive care unit (parameter estimate, 1.289; 95% CI, 1.179, 1.399), mean age of inpatients (parameter estimate, 0.025; 95% CI, 0.014, 0.035), and 945–3,463 operations per year (parameter estimate, −0.276; 95% CI, −0.429, −0.124). Detailed risk model parameter estimates are presented in
Tables 2 and
3. The K-SAAR values for each hospital are shown in
Supplementary Table 2.
Table 2
Korean standardized antimicrobial administration ratio risk model parameter estimates for all patients
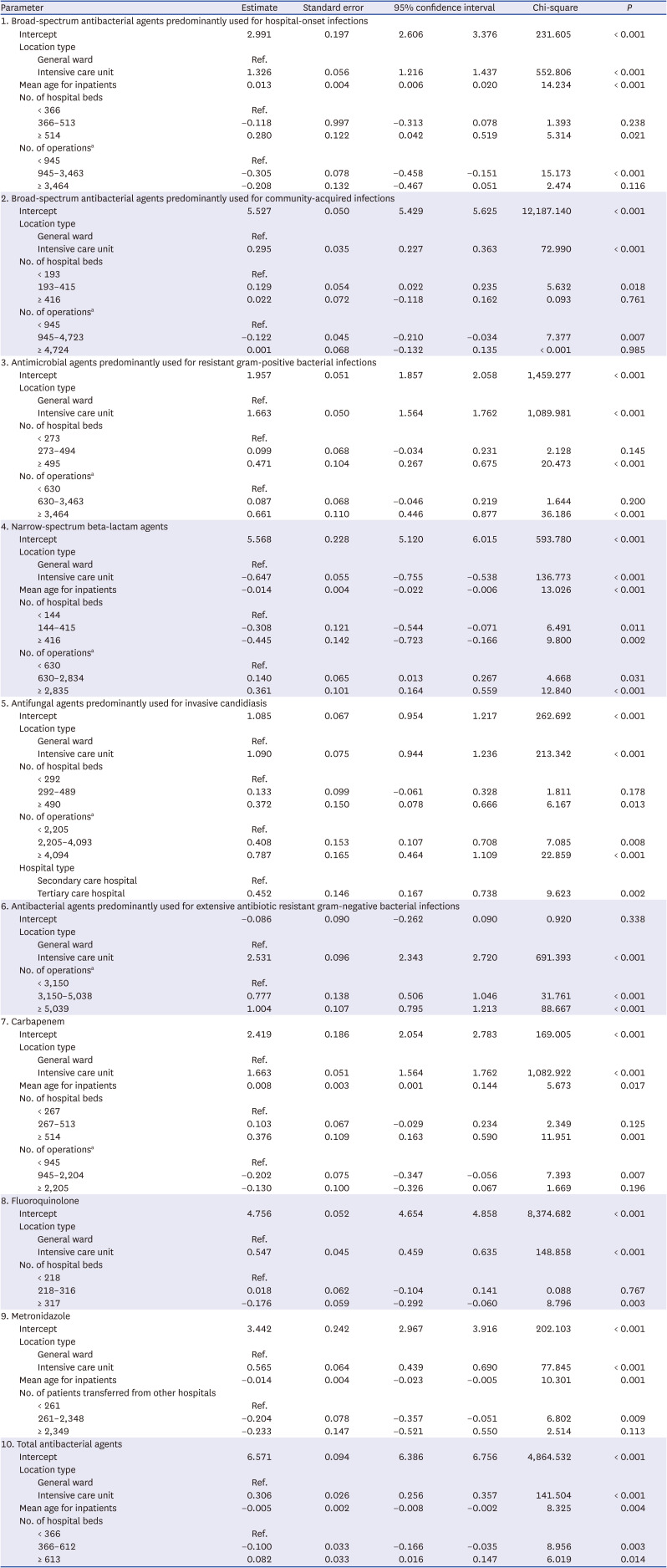
Parameter |
Estimate |
Standard error |
95% confidence interval |
Chi-square |
P
|
1. Broad-spectrum antibacterial agents predominantly used for hospital-onset infections |
|
Intercept |
2.991 |
0.197 |
2.606 |
3.376 |
231.605 |
< 0.001 |
|
Location type |
|
|
|
|
|
|
|
|
General ward |
Ref. |
|
|
|
|
|
|
|
Intensive care unit |
1.326 |
0.056 |
1.216 |
1.437 |
552.806 |
< 0.001 |
|
Mean age for inpatients |
0.013 |
0.004 |
0.006 |
0.020 |
14.234 |
< 0.001 |
|
No. of hospital beds |
|
|
|
|
|
|
|
|
< 366 |
Ref. |
|
|
|
|
|
|
|
366–513 |
−0.118 |
0.997 |
−0.313 |
0.078 |
1.393 |
0.238 |
|
|
≥ 514 |
0.280 |
0.122 |
0.042 |
0.519 |
5.314 |
0.021 |
|
No. of operationsa
|
|
|
|
|
|
|
|
|
< 945 |
Ref. |
|
|
|
|
|
|
|
945–3,463 |
−0.305 |
0.078 |
−0.458 |
−0.151 |
15.173 |
< 0.001 |
|
|
≥ 3,464 |
−0.208 |
0.132 |
−0.467 |
0.051 |
2.474 |
0.116 |
2. Broad-spectrum antibacterial agents predominantly used for community-acquired infections |
|
Intercept |
5.527 |
0.050 |
5.429 |
5.625 |
12,187.140 |
< 0.001 |
|
Location type |
|
|
|
|
|
|
|
|
General ward |
Ref. |
|
|
|
|
|
|
|
Intensive care unit |
0.295 |
0.035 |
0.227 |
0.363 |
72.990 |
< 0.001 |
|
No. of hospital beds |
|
|
|
|
|
|
|
|
< 193 |
Ref. |
|
|
|
|
|
|
|
193–415 |
0.129 |
0.054 |
0.022 |
0.235 |
5.632 |
0.018 |
|
|
≥ 416 |
0.022 |
0.072 |
−0.118 |
0.162 |
0.093 |
0.761 |
|
No. of operationsa
|
|
|
|
|
|
|
|
|
< 945 |
Ref. |
|
|
|
|
|
|
|
945–4,723 |
−0.122 |
0.045 |
−0.210 |
−0.034 |
7.377 |
0.007 |
|
|
≥ 4,724 |
0.001 |
0.068 |
−0.132 |
0.135 |
< 0.001 |
0.985 |
3. Antimicrobial agents predominantly used for resistant gram-positive bacterial infections |
|
Intercept |
1.957 |
0.051 |
1.857 |
2.058 |
1,459.277 |
< 0.001 |
|
Location type |
|
|
|
|
|
|
|
|
General ward |
Ref. |
|
|
|
|
|
|
|
Intensive care unit |
1.663 |
0.050 |
1.564 |
1.762 |
1,089.981 |
< 0.001 |
|
No. of hospital beds |
|
|
|
|
|
|
|
|
< 273 |
Ref. |
|
|
|
|
|
|
|
273–494 |
0.099 |
0.068 |
−0.034 |
0.231 |
2.128 |
0.145 |
|
|
≥ 495 |
0.471 |
0.104 |
0.267 |
0.675 |
20.473 |
< 0.001 |
|
No. of operationsa
|
|
|
|
|
|
|
|
|
< 630 |
Ref. |
|
|
|
|
|
|
|
630–3,463 |
0.087 |
0.068 |
−0.046 |
0.219 |
1.644 |
0.200 |
|
|
≥ 3,464 |
0.661 |
0.110 |
0.446 |
0.877 |
36.186 |
< 0.001 |
4. Narrow-spectrum beta-lactam agents |
|
Intercept |
5.568 |
0.228 |
5.120 |
6.015 |
593.780 |
< 0.001 |
|
Location type |
|
|
|
|
|
|
|
|
General ward |
Ref. |
|
|
|
|
|
|
|
Intensive care unit |
−0.647 |
0.055 |
−0.755 |
−0.538 |
136.773 |
< 0.001 |
|
Mean age for inpatients |
−0.014 |
0.004 |
−0.022 |
−0.006 |
13.026 |
< 0.001 |
|
No. of hospital beds |
|
|
|
|
|
|
|
|
< 144 |
Ref. |
|
|
|
|
|
|
|
144–415 |
−0.308 |
0.121 |
−0.544 |
−0.071 |
6.491 |
0.011 |
|
|
≥ 416 |
−0.445 |
0.142 |
−0.723 |
−0.166 |
9.800 |
0.002 |
|
No. of operationsa
|
|
|
|
|
|
|
|
|
< 630 |
Ref. |
|
|
|
|
|
|
|
630–2,834 |
0.140 |
0.065 |
0.013 |
0.267 |
4.668 |
0.031 |
|
|
≥ 2,835 |
0.361 |
0.101 |
0.164 |
0.559 |
12.840 |
< 0.001 |
5. Antifungal agents predominantly used for invasive candidiasis |
|
Intercept |
1.085 |
0.067 |
0.954 |
1.217 |
262.692 |
< 0.001 |
|
Location type |
|
|
|
|
|
|
|
|
General ward |
Ref. |
|
|
|
|
|
|
|
Intensive care unit |
1.090 |
0.075 |
0.944 |
1.236 |
213.342 |
< 0.001 |
|
No. of hospital beds |
|
|
|
|
|
|
|
|
< 292 |
Ref. |
|
|
|
|
|
|
|
292–489 |
0.133 |
0.099 |
−0.061 |
0.328 |
1.811 |
0.178 |
|
|
≥ 490 |
0.372 |
0.150 |
0.078 |
0.666 |
6.167 |
0.013 |
|
No. of operationsa
|
|
|
|
|
|
|
|
|
< 2,205 |
Ref. |
|
|
|
|
|
|
|
2,205–4,093 |
0.408 |
0.153 |
0.107 |
0.708 |
7.085 |
0.008 |
|
|
≥ 4,094 |
0.787 |
0.165 |
0.464 |
1.109 |
22.859 |
< 0.001 |
|
Hospital type |
|
|
|
|
|
|
|
|
Secondary care hospital |
Ref. |
|
|
|
|
|
|
|
Tertiary care hospital |
0.452 |
0.146 |
0.167 |
0.738 |
9.623 |
0.002 |
6. Antibacterial agents predominantly used for extensive antibiotic resistant gram-negative bacterial infections |
|
Intercept |
−0.086 |
0.090 |
−0.262 |
0.090 |
0.920 |
0.338 |
|
Location type |
|
|
|
|
|
|
|
|
General ward |
Ref. |
|
|
|
|
|
|
|
Intensive care unit |
2.531 |
0.096 |
2.343 |
2.720 |
691.393 |
< 0.001 |
|
No. of operationsa
|
|
|
|
|
|
|
|
|
< 3,150 |
Ref. |
|
|
|
|
|
|
|
3,150–5,038 |
0.777 |
0.138 |
0.506 |
1.046 |
31.761 |
< 0.001 |
|
|
≥ 5,039 |
1.004 |
0.107 |
0.795 |
1.213 |
88.667 |
< 0.001 |
7. Carbapenem |
|
Intercept |
2.419 |
0.186 |
2.054 |
2.783 |
169.005 |
< 0.001 |
|
Location type |
|
|
|
|
|
|
|
|
General ward |
Ref. |
|
|
|
|
|
|
|
Intensive care unit |
1.663 |
0.051 |
1.564 |
1.762 |
1,082.922 |
< 0.001 |
|
Mean age for inpatients |
0.008 |
0.003 |
0.001 |
0.144 |
5.673 |
0.017 |
|
No. of hospital beds |
|
|
|
|
|
|
|
|
< 267 |
Ref. |
|
|
|
|
|
|
|
267–513 |
0.103 |
0.067 |
−0.029 |
0.234 |
2.349 |
0.125 |
|
|
≥ 514 |
0.376 |
0.109 |
0.163 |
0.590 |
11.951 |
0.001 |
|
No. of operationsa
|
|
|
|
|
|
|
|
|
< 945 |
Ref. |
|
|
|
|
|
|
|
945–2,204 |
−0.202 |
0.075 |
−0.347 |
−0.056 |
7.393 |
0.007 |
|
|
≥ 2,205 |
−0.130 |
0.100 |
−0.326 |
0.067 |
1.669 |
0.196 |
8. Fluoroquinolone |
|
Intercept |
4.756 |
0.052 |
4.654 |
4.858 |
8,374.682 |
< 0.001 |
|
Location type |
|
|
|
|
|
|
|
|
General ward |
Ref. |
|
|
|
|
|
|
|
Intensive care unit |
0.547 |
0.045 |
0.459 |
0.635 |
148.858 |
< 0.001 |
|
No. of hospital beds |
|
|
|
|
|
|
|
|
< 218 |
Ref. |
|
|
|
|
|
|
|
218–316 |
0.018 |
0.062 |
−0.104 |
0.141 |
0.088 |
0.767 |
|
|
≥ 317 |
−0.176 |
0.059 |
−0.292 |
−0.060 |
8.796 |
0.003 |
9. Metronidazole |
|
Intercept |
3.442 |
0.242 |
2.967 |
3.916 |
202.103 |
< 0.001 |
|
Location type |
|
|
|
|
|
|
|
|
General ward |
Ref. |
|
|
|
|
|
|
|
Intensive care unit |
0.565 |
0.064 |
0.439 |
0.690 |
77.845 |
< 0.001 |
|
Mean age for inpatients |
−0.014 |
0.004 |
−0.023 |
−0.005 |
10.301 |
0.001 |
|
No. of patients transferred from other hospitals |
|
|
|
|
|
|
|
|
< 261 |
Ref. |
|
|
|
|
|
|
|
261–2,348 |
−0.204 |
0.078 |
−0.357 |
−0.051 |
6.802 |
0.009 |
|
|
≥ 2,349 |
−0.233 |
0.147 |
−0.521 |
0.550 |
2.514 |
0.113 |
10. Total antibacterial agents |
|
Intercept |
6.571 |
0.094 |
6.386 |
6.756 |
4,864.532 |
< 0.001 |
|
Location type |
|
|
|
|
|
|
|
|
General ward |
Ref. |
|
|
|
|
|
|
|
Intensive care unit |
0.306 |
0.026 |
0.256 |
0.357 |
141.504 |
< 0.001 |
|
Mean age for inpatients |
−0.005 |
0.002 |
−0.008 |
−0.002 |
8.325 |
0.004 |
|
No. of hospital beds |
|
|
|
|
|
|
|
|
< 366 |
Ref. |
|
|
|
|
|
|
|
366–612 |
−0.100 |
0.033 |
−0.166 |
−0.035 |
8.956 |
0.003 |
|
|
≥ 613 |
0.082 |
0.033 |
0.016 |
0.147 |
6.019 |
0.014 |
Table 3
Korean standardized antimicrobial administration ratio risk model parameter estimates for adult patients
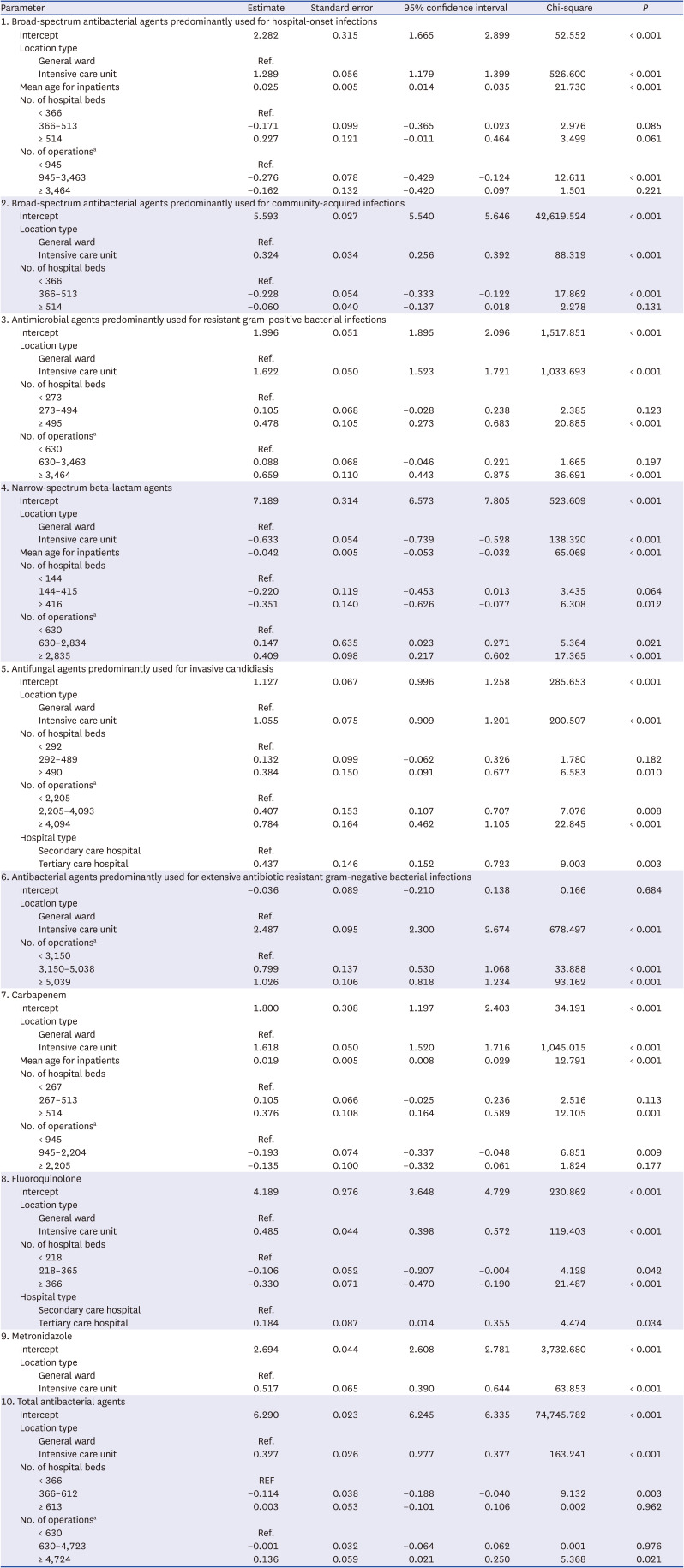
Parameter |
Estimate |
Standard error |
95% confidence interval |
Chi-square |
P
|
1. Broad-spectrum antibacterial agents predominantly used for hospital-onset infections |
|
Intercept |
2.282 |
0.315 |
1.665 |
2.899 |
52.552 |
< 0.001 |
|
Location type |
|
|
|
|
|
|
|
|
General ward |
Ref. |
|
|
|
|
|
|
|
Intensive care unit |
1.289 |
0.056 |
1.179 |
1.399 |
526.600 |
< 0.001 |
|
Mean age for inpatients |
0.025 |
0.005 |
0.014 |
0.035 |
21.730 |
< 0.001 |
|
No. of hospital beds |
|
|
|
|
|
|
|
|
< 366 |
Ref. |
|
|
|
|
|
|
|
366–513 |
−0.171 |
0.099 |
−0.365 |
0.023 |
2.976 |
0.085 |
|
|
≥ 514 |
0.227 |
0.121 |
−0.011 |
0.464 |
3.499 |
0.061 |
|
No. of operationsa
|
|
|
|
|
|
|
|
|
< 945 |
Ref. |
|
|
|
|
|
|
|
945–3,463 |
−0.276 |
0.078 |
−0.429 |
−0.124 |
12.611 |
< 0.001 |
|
|
≥ 3,464 |
−0.162 |
0.132 |
−0.420 |
0.097 |
1.501 |
0.221 |
2. Broad-spectrum antibacterial agents predominantly used for community-acquired infections |
|
Intercept |
5.593 |
0.027 |
5.540 |
5.646 |
42,619.524 |
< 0.001 |
|
Location type |
|
|
|
|
|
|
|
|
General ward |
Ref. |
|
|
|
|
|
|
|
Intensive care unit |
0.324 |
0.034 |
0.256 |
0.392 |
88.319 |
< 0.001 |
|
No. of hospital beds |
|
|
|
|
|
|
|
|
< 366 |
Ref. |
|
|
|
|
|
|
|
366–513 |
−0.228 |
0.054 |
−0.333 |
−0.122 |
17.862 |
< 0.001 |
|
|
≥ 514 |
−0.060 |
0.040 |
−0.137 |
0.018 |
2.278 |
0.131 |
3. Antimicrobial agents predominantly used for resistant gram-positive bacterial infections |
|
Intercept |
1.996 |
0.051 |
1.895 |
2.096 |
1,517.851 |
< 0.001 |
|
Location type |
|
|
|
|
|
|
|
|
General ward |
Ref. |
|
|
|
|
|
|
|
Intensive care unit |
1.622 |
0.050 |
1.523 |
1.721 |
1,033.693 |
< 0.001 |
|
No. of hospital beds |
|
|
|
|
|
|
|
|
< 273 |
Ref. |
|
|
|
|
|
|
|
273–494 |
0.105 |
0.068 |
−0.028 |
0.238 |
2.385 |
0.123 |
|
|
≥ 495 |
0.478 |
0.105 |
0.273 |
0.683 |
20.885 |
< 0.001 |
|
No. of operationsa
|
|
|
|
|
|
|
|
|
< 630 |
Ref. |
|
|
|
|
|
|
|
630–3,463 |
0.088 |
0.068 |
−0.046 |
0.221 |
1.665 |
0.197 |
|
|
≥ 3,464 |
0.659 |
0.110 |
0.443 |
0.875 |
36.691 |
< 0.001 |
4. Narrow-spectrum beta-lactam agents |
|
Intercept |
7.189 |
0.314 |
6.573 |
7.805 |
523.609 |
< 0.001 |
|
Location type |
|
|
|
|
|
|
|
|
General ward |
Ref. |
|
|
|
|
|
|
|
Intensive care unit |
−0.633 |
0.054 |
−0.739 |
−0.528 |
138.320 |
< 0.001 |
|
Mean age for inpatients |
−0.042 |
0.005 |
−0.053 |
−0.032 |
65.069 |
< 0.001 |
|
No. of hospital beds |
|
|
|
|
|
|
|
|
< 144 |
Ref. |
|
|
|
|
|
|
|
144–415 |
−0.220 |
0.119 |
−0.453 |
0.013 |
3.435 |
0.064 |
|
|
≥ 416 |
−0.351 |
0.140 |
−0.626 |
−0.077 |
6.308 |
0.012 |
|
No. of operationsa
|
|
|
|
|
|
|
|
|
< 630 |
Ref. |
|
|
|
|
|
|
|
630–2,834 |
0.147 |
0.635 |
0.023 |
0.271 |
5.364 |
0.021 |
|
|
≥ 2,835 |
0.409 |
0.098 |
0.217 |
0.602 |
17.365 |
< 0.001 |
5. Antifungal agents predominantly used for invasive candidiasis |
|
Intercept |
1.127 |
0.067 |
0.996 |
1.258 |
285.653 |
< 0.001 |
|
Location type |
|
|
|
|
|
|
|
|
General ward |
Ref. |
|
|
|
|
|
|
|
Intensive care unit |
1.055 |
0.075 |
0.909 |
1.201 |
200.507 |
< 0.001 |
|
No. of hospital beds |
|
|
|
|
|
|
|
|
< 292 |
Ref. |
|
|
|
|
|
|
|
292–489 |
0.132 |
0.099 |
−0.062 |
0.326 |
1.780 |
0.182 |
|
|
≥ 490 |
0.384 |
0.150 |
0.091 |
0.677 |
6.583 |
0.010 |
|
No. of operationsa
|
|
|
|
|
|
|
|
|
< 2,205 |
Ref. |
|
|
|
|
|
|
|
2,205–4,093 |
0.407 |
0.153 |
0.107 |
0.707 |
7.076 |
0.008 |
|
|
≥ 4,094 |
0.784 |
0.164 |
0.462 |
1.105 |
22.845 |
< 0.001 |
|
Hospital type |
|
|
|
|
|
|
|
|
Secondary care hospital |
Ref. |
|
|
|
|
|
|
|
Tertiary care hospital |
0.437 |
0.146 |
0.152 |
0.723 |
9.003 |
0.003 |
6. Antibacterial agents predominantly used for extensive antibiotic resistant gram-negative bacterial infections |
|
Intercept |
−0.036 |
0.089 |
−0.210 |
0.138 |
0.166 |
0.684 |
|
Location type |
|
|
|
|
|
|
|
|
General ward |
Ref. |
|
|
|
|
|
|
|
Intensive care unit |
2.487 |
0.095 |
2.300 |
2.674 |
678.497 |
< 0.001 |
|
No. of operationsa
|
|
|
|
|
|
|
|
|
< 3,150 |
Ref. |
|
|
|
|
|
|
|
3,150–5,038 |
0.799 |
0.137 |
0.530 |
1.068 |
33.888 |
< 0.001 |
|
|
≥ 5,039 |
1.026 |
0.106 |
0.818 |
1.234 |
93.162 |
< 0.001 |
7. Carbapenem |
|
Intercept |
1.800 |
0.308 |
1.197 |
2.403 |
34.191 |
< 0.001 |
|
Location type |
|
|
|
|
|
|
|
|
General ward |
Ref. |
|
|
|
|
|
|
|
Intensive care unit |
1.618 |
0.050 |
1.520 |
1.716 |
1,045.015 |
< 0.001 |
|
Mean age for inpatients |
0.019 |
0.005 |
0.008 |
0.029 |
12.791 |
< 0.001 |
|
No. of hospital beds |
|
|
|
|
|
|
|
|
< 267 |
Ref. |
|
|
|
|
|
|
|
267–513 |
0.105 |
0.066 |
−0.025 |
0.236 |
2.516 |
0.113 |
|
|
≥ 514 |
0.376 |
0.108 |
0.164 |
0.589 |
12.105 |
0.001 |
|
No. of operationsa
|
|
|
|
|
|
|
|
|
< 945 |
Ref. |
|
|
|
|
|
|
|
945–2,204 |
−0.193 |
0.074 |
−0.337 |
−0.048 |
6.851 |
0.009 |
|
|
≥ 2,205 |
−0.135 |
0.100 |
−0.332 |
0.061 |
1.824 |
0.177 |
8. Fluoroquinolone |
|
Intercept |
4.189 |
0.276 |
3.648 |
4.729 |
230.862 |
< 0.001 |
|
Location type |
|
|
|
|
|
|
|
|
General ward |
Ref. |
|
|
|
|
|
|
|
Intensive care unit |
0.485 |
0.044 |
0.398 |
0.572 |
119.403 |
< 0.001 |
|
No. of hospital beds |
|
|
|
|
|
|
|
|
< 218 |
Ref. |
|
|
|
|
|
|
|
218–365 |
−0.106 |
0.052 |
−0.207 |
−0.004 |
4.129 |
0.042 |
|
|
≥ 366 |
−0.330 |
0.071 |
−0.470 |
−0.190 |
21.487 |
< 0.001 |
|
Hospital type |
|
|
|
|
|
|
|
|
Secondary care hospital |
Ref. |
|
|
|
|
|
|
|
Tertiary care hospital |
0.184 |
0.087 |
0.014 |
0.355 |
4.474 |
0.034 |
9. Metronidazole |
|
Intercept |
2.694 |
0.044 |
2.608 |
2.781 |
3,732.680 |
< 0.001 |
|
Location type |
|
|
|
|
|
|
|
|
General ward |
Ref. |
|
|
|
|
|
|
|
Intensive care unit |
0.517 |
0.065 |
0.390 |
0.644 |
63.853 |
< 0.001 |
10. Total antibacterial agents |
|
Intercept |
6.290 |
0.023 |
6.245 |
6.335 |
74,745.782 |
< 0.001 |
|
Location type |
|
|
|
|
|
|
|
|
General ward |
Ref. |
|
|
|
|
|
|
|
Intensive care unit |
0.327 |
0.026 |
0.277 |
0.377 |
163.241 |
< 0.001 |
|
No. of hospital beds |
|
|
|
|
|
|
|
|
< 366 |
REF |
|
|
|
|
|
|
|
366–612 |
−0.114 |
0.038 |
−0.188 |
−0.040 |
9.132 |
0.003 |
|
|
≥ 613 |
0.003 |
0.053 |
−0.101 |
0.106 |
0.002 |
0.962 |
|
No. of operationsa
|
|
|
|
|
|
|
|
|
< 630 |
Ref. |
|
|
|
|
|
|
|
630–4,723 |
−0.001 |
0.032 |
−0.064 |
0.062 |
0.001 |
0.976 |
|
|
≥ 4,724 |
0.136 |
0.059 |
0.021 |
0.250 |
5.368 |
0.021 |
DISCUSSION
KONAS is the first benchmarking system for antimicrobial use in Korean hospitals, providing not only the crude amount of antimicrobial use in each hospital for direct comparison, but also K-SAAR for indirect comparison. With K-SAAR, medical personnel can easily measure and monitor antimicrobial use in their hospitals and grasp the degree of antimicrobial use, using benchmarking data. In particular, when K-SAAR is above 1, further evaluation and possible hospital-specific interventions can be initiated.
There are several advantages of K-SAAR compared to a direct comparison of antimicrobial use among hospitals. First, the novel metric is a customized indicator that reflects the characteristics of each hospital. The predictive value of antimicrobial use in a hospital is derived from meticulous modeling using variables that are associated with hospital characteristics and might influence the pattern of antimicrobial use in a hospital. Therefore, medical personnel can detect the possible overuse or misuse of antimicrobials in their hospital more easily and precisely. Second, the overall pattern of antimicrobial use within hospitals can be identified through various K-SAAR values according to patient age group, hospital location, and antimicrobial category. For instance, if the K-SAARs for broad-spectrum antibacterial agents predominantly used for hospital-onset infections and antibacterial agents predominantly used for resistant gram-positive infections are observed above 1, and those for broad-spectrum antibacterial agents predominantly used for community-acquired infections and narrow-spectrum beta-lactam agents are observed below 1, medical personnel can suspect that certain classes of antimicrobials such as anti-pseudomonal β-lactam antibiotics or glycopeptides might be excessively prescribed within the hospital. Third, there is little risk of unintentional exposure to individual hospital information. For a direct comparison, even if the hospital names are anonymized, the source of hospital data presented for comparison can be inferred from the remaining information.
Despite these merits, medical personnel should remember that the K-SAAR metric provides a quantitative evaluation of antimicrobial use, but does not provide a definitive measure of the appropriateness or judiciousness of antimicrobial use. Therefore, K-SAAR can be used for monitoring antimicrobial use or follow-up of ASP intervention and should not be used for public reporting or other external accountability purposes.
4
There are some potential limitations to the present study. First, the amount of antimicrobial consumption was measured using DDD instead of directly observed therapy (DOT). In fact, according to a recent guideline for ASP, DOT is the preferred method for the measurement of antimicrobial consumption, because DDD has the possibility of underestimation among patients with decreased renal function and pediatric patients.
9 However, the DOT of antimicrobials provided by the medical insurance claims data has yet to be validated properly. If the DOT of medical insurance claims data reflects the actual data well, an update of the K-SAAR predictive model using antimicrobial consumption data measured by the DOT should be performed. Second, the current K-SAAR does not apply to pediatric patients. Given that the antimicrobial consumption data were measured by DDD, we could not develop a predictive model for pediatric patients. This problem will be solved if the DOT of medical insurance claim data is validated. Even though limitations of data regarding pediatric patients exist, the research group performed the modeling for all inpatients (aged ≥ 28 days) to enhance the understanding of overall antimicrobial consumption within the hospital at the current phase. Third, the current K-SAAR applies only to annual antimicrobial use in secondary or tertiary care hospitals. It is necessary to develop a K-SAAR predictive model that can be applied to not only antimicrobial use in small- and medium-sized hospitals but also to quarterly antimicrobial use. Fourth, factors reflecting the characteristics and severity of patients within each hospital (e.g., number of transplant recipients, number of patients on hemodialysis, number of septic shock cases, number of neutropenic patients, etc.) were not sufficiently included in the model variables. Because some variables are not available from the medical insurance claim data, the establishment of a system that can collect standardized data from each hospital is necessary in the near future. With such a system, an updated model that reflects patient characteristics and severity more precisely might be able to be developed.
In conclusion, we developed a model to predict antimicrobial use rates in Korean hospitals, and the model was used as the denominator of the K-SAAR. The K-SAAR helps medical personnel easily grasp the status of antimicrobial use in their hospital by providing the ratio between observed antimicrobial use and predicted antimicrobial use. Through K-SAAR, each hospital can effectively investigate the potential overuse or misuse of antimicrobials so that they can establish appropriate ASP interventions.