Abstract
Background and Objectives
Bone marrow mesenchymal stem cells (BMSCs) show considerable promise in regenerative medicine. Many studies demonstrated that BMSCs cultured in vitro were highly heterogeneous and composed of diverse cell subpopulations, which may be the basis of their multiple biological characteristics. However, the exact cell subpopulations that make up BMSCs are still unknown.
Methods and Results
In this study, we used single-cell RNA sequencing (scRNA-Seq) to divide 6,514 BMSCs into three clusters. The number and corresponding proportion of cells in clusters 1 to 3 were 3,766 (57.81%), 1,720 (26.40%), and 1,028 (15.78%). The gene expression profile and function of the cells in the same cluster were similar. The vast majority of cells expressed the markers defining BMSCs by flow cytometry and gene expression analysis. Each cluster had at least 20 differentially expressed genes (DEGs). We conducted Gene Ontology enrichment analysis on the top 20 DEGs of each cluster and found that the three clusters had different functions, which were related to self-renewal, multilineage differentiation and cytokine secretion, respectively. In addition, the function of the top 20 DEGs of each cluster was checked by the National Center for Biotechnology Information gene database to further verify our hypothesis.
Bone marrow mesenchymal stem cells (BMSCs) show considerable promise for regenerative medicine, and tissue engineering because they have the competence of self-renewal and differentiation into various types of tissue-specific cells, including osteoblasts, chondrocytes and adipocytes (1, 2). Many studies indicated that BMSCs was a highly heterogeneous cell population composed of different cell subpopulations, which may be the basis of their multiple biological characteristics (3, 4). However, it is not clear which cell subpopulations with different properties or functions they consist of. In addition, most of the BMSCs in current studies came from younger donors, not elderly people.
By collectively sequencing tens of thousands of cell samples, bulk RNA sequencing only reveals the average effect of the cell population or information about the larger number of cells, masking the heterogeneity among cells. Therefore, the ideal method to analyze which cells the tissue consists of or to explore the heterogeneity among cells with the same type is to analyze individual cells in the sample. In 2009, Tang et al. (5) combined single-cell RNA amplification technology with second-generation sequencing technology to conduct single-cell RNA sequencing (scRNA-seq) experiments on mature mouse egg cells for the first time. With the development of single-cell isolation, gene amplification, sequencing, and other technologies, scRNA-seq has been gradually applied to study tumor cells, immune cells, etc., but few studies have used it to analyze human BMSCs (6, 7). It is thus of great interest for us to dissect the cellular heterogeneity of BMSCs by scRNA-seq profiling.
The experimental sample was taken from an 85-year-old female patient with intertrochanteric fracture admitted to our hospital. Informed consent was obtained from all participants. This study was approved by the ethics committee of the institutional review board at the Seventh Medical Center of Chinese PLA General Hospital (Number. 2017-87).
This patient was treated with intramedullary nailing. During the operation, after opening the apex of the trochiter with a drill, the aspirator (about 20 cm in length) was inserted into the medullary cavity. An injector was used to connect the tail of the aspirator, and about 10 ml of bone marrow was extracted from the fractured femur (Supplementary Fig. S1). Pay attention to avoid mixing with peripheral blood, and avoid violent oscillations during transportation (8).
This experiment adopted density gradient centrifuging to separate bone marrow mononuclear cells of the donor. The cells were cultured in dulbecco’s modified eagle medium (DMEM) (StemRD, USA) containing 10% fetal bovine serum (FBs) (StemRD, USA) and 1% penicillin and streptomycin (Corning, USA). The cells were maintained in a 5% CO2 humidified incubator at a constant temperature of 37℃. The medium was changed every 3 days. The cells were observed by inverted phase contrast microscope. When the adherent cells reached 80% confluence, they were digested with 0.25% trypsin (Corning, USA) and passaged. The BMSCs at passage 2 were harvested for the further experiments.
The BMSCs at passage 2 were digested with trypsin, washed with phosphate-buffered saline (PBS), and stained with CD29-APC (BD Biosciences, USA), CD44-FITC (BD Biosciences, USA), CD90-FITC (BD Biosciences, USA), CD105-APC (BD Biosciences, USA), CD34-PE (BD Biosciences, USA), CD45-PE (BD Biosciences, USA), and HLA-DR-PE (BD Biosciences, USA). The cells were washed and resuspended with PBS. Flow cytometry analysis was performed on the flow cytometer (BD Biosciences, USA).
The BMSCs at passage 2 were used to prepare a single-cell suspension. 10x Genomics Chromium was used to construct scRNA-seq response system, and all experiments were carried out according to the official operating manual (9). The single cells were separated by droplet micro-fluidics. A Gel Bead with Read1, cell label (Barcode), Unique Molecular Identifiers (UMI), and Poly (dT) was mixed with a single cell through oil phase to form the Gel Bead in Emulsions (GEMs). Barcode could distinguish different cells, UMI could distinguish different cDNA in the same cell, and Poly (dT) was used to enrich mRNA (10, 11). After lysing the cells, free mRNA underwent reverse transcriptional reaction and cDNA with Barcode and UMI information was generated. The Emulsions were then broken and the appropriate length of cDNA was selected as the template for PCR amplification. The Single Cell 30 Reagent Kit (v2 chemistry) was used to construct library. Agilent 2100 bioanalyzer was used to detect the peak type and fragment size of library. The resulting libraries were sequenced on the Illumina System.
The official 10x Genomics pipeline Cell Ranger was used to deal with raw data. The BCL format file generated by Illumina sequencers was converted to Fastq format file by using the Cell Ranger mkfastq pipeline. Next, the reads library was processed by using the Cell Ranger count pipeline to generate a gene-barcode matrix. Based on the STAR algorithm (12), the reads were aligned to a human reference genome. Cell barcodes and UMIs were calibrated and filtered. The expected number of recovered cells was set to 5,000, as a parameter related to cell barcode filtering.
The dimension of the data on these genes was reduced through principal component analysis (PCA). The cells were clustered into diverse clusters through the k-means clustering approach. Next, the clustered cells were visualized in two-dimensional projection of t-distributed stochastic neighbour embedding (t-SNE).
The Loupe Browser was used to analyze the expression of the International Society for Cellular Therapy (ICST)-proposed positive marker genes CD29 (ITGB1), CD44, CD90 (THY1), CD105 (ENG), and negative marker genes CD34, CD45 (PTPRC), HLA-DRA (1). Besides, the expression of markers for early passage, FGFR2, and late passage BMSCs, PLAT, were researched (13).
Based on the results of cell clustering, the expression difference of each gene between that cluster and the average of the rest of clusters were computed to identify the differentially expressed genes (DEGs) of each cluster. Meanwhile, the corresponding fold change (FC) and p-value of each gene were obtained (14, 15). DEGs must meet the following conditions: (1) mean UMI counts>1; (2) log2FC>1; (3) p<0.05. The top 20 DEGs were selected according to the log2FC sequence from large to small. Kobas 3.0 was used to conduct Gene Ontology (GO) enrichment analysis on the top 20 DEGs of each cluster, so as to determine their main functions. The function of the top 20 DEGs of each cluster was checked by the National Center for Biotechnology Information (NCBI) gene database (https://www.ncbi.nlm.nih.gov/gene/).
Almost all the cells expressed CD29 (99.95%), CD44 (96.18%), CD90 (99.32%), and CD105 (99.50%). Inversely, very few cells expressed CD34 (1.69%), CD45 (0.11%), or HLA-DR (0.63%) (Fig. 1a).
The expression of a variety of characteristic marker genes in BMSCs was examined. We found that the expression of the ISCT-proposed positive marker genes, such as CD29 (ITGB1), CD44, CD90 (THY1), and CD105 (ENG) was very high. On the contrary, the cells lacked expression of the negative marker genes, such as CD34, CD45 (PTPRC), and HLA-DRA (Fig. 1b). The proportion of cells expressing the marker genes is as follows: CD29 (ITGB1): 99.94%, CD44: 99.74%, CD90 (THY1): 99.75%, CD105 (ENG): 87.27%, CD34: 0.12%, CD45 (PTPRC): 0.23%, HLA-DRA: 0.03%. In addition, more than half of the cells expressed PLAT (52.26%), the marker for late passage BMSCs, but very few cells expressed FGFR2 (0.09%), the marker for early passage BMSCs (Fig. 1c).
The cells that have similar gene expression profile were clustered. The 6,514 cells were divided into three clusters through the k-means clustering approach. The axes correspond to the 2-dimensional embedding produced by the t-SNE algorithm (Fig. 3). The number and corresponding proportion of cells in clusters 1 to 3 were 3,766 (57.81%), 1,720 (26.40%), and 1,028 (15.78%), respectively. Meanwhile, the top 20 DEGs of each cluster were obtained (Table 2), and the clustering effect of them in each cluster was ideal (Fig. 4). The expression of the top 20 DEGs of three clusters in all cells was revealed in Supplementary Fig. S2∼4.
The top 20 DEGs corresponding to the three clusters were analyzed by GO analysis, and we found that the top 20 DEGs of different clusters were significantly enriched with different molecular functions, biological processes and cellular components (Supplementary Fig. S5∼7).
The top 20 DEGs of cluster 1 were significantly enriched in biological processes such as DNA conformation change and DNA packaging; cellular components such as chromosome and histone binding; molecular functions such as histone binding.
The top 20 DEGs of cluster 2 were significantly enriched in biological processes such as collagen fibril organization and endodermal cell differentiation; cellular components such as ferritin complex and fibrillar collagen trimer; molecular functions such as extracellular matrix structural constituent.
The top 20 DEGs of cluster 3 were significantly enriched in biological processes such as prostaglandin biosynthetic process, positive regulation of protein ubiquitination, innate immune response and response to cytokine; cellular components such as extracellular organelle and vesicle; molecular functions such as protein binding and enzyme binding.
BMSCs show considerable potential in clinical applications in regenerative medicine, especially because they are easily accessible and hold no ethical concerns. They possess the capability for tissue repair and immunomo-dulatory through cell-to-cell contact concomitant with paracrine secretion of large amounts of bioactive macromolecules (1). Various studies suggest that autologous BMSCs transplantation can be used to treat diseases such as myocardiopathy, osteoarthritis, and nerve injury (2, 16). In addition, the patients requiring this therapy are usually elderly. In this study, we successfully isolated and cultured BMSCs from an 85-year-old patient with hip fracture, which were consistent with the characteristics of BMSCs in terms of cell morphology, growth characteristics and phenotype.
Many studies demonstrated that invasive methods, such as bone marrow puncture, were used to collect bone marrow from the iliac crest, which not only increased the patients’ pain, but also increased the risk of complications such as infection (17). The volunteer in this study was an elderly patient with intertrochanteric fracture who was treated with intramedullary nailing. To avoid additional damage, we extracted bone marrow from the fractured femoral bone marrow cavity and successfully separated BMSCs. BMSCs from the elderly often show reduced proliferation and differentiation (18). In our study, when the cells were passed to the third generation, there was a clear decrease in proliferation. Therefore, we finally chose the BMSCs at passage 2 for the experiment.
By using flow cytometry, we found that nearly all the BMSCs expressed CD29, CD44, CD90, and CD105, but very few cells expressed CD34, CD45 or HLA-DR. We detected the expression of these markers by Loupe Browser and obtained similar results. On the one hand, these BMSCs fulfill the criteria suggested by the ICST (1); on the other hand, it shows that scRNA-Seq quantification of BMSCs populations corresponds with immunophenotyping by flow cytometry. At the same time, it demonstrates the reliability of the sequencing technology. Similarly, Oetjen et al. (19) used scRNA-Seq to evaluate over 76,000 cells from 20 healthy adult human donors, all the major subpopulations of bone marrow mononuclear cells were identified, and they found overall subpopulation frequencies were resemble to flow cytometry of the matched samples.
The BMSCs cultured in vitro may contain cell types at different stages of differentiation (20). Therefore, they are highly heterogeneous, with different morphology, phenotype, and differentiation ability (3, 4). In addition, they may be composed of different subpopulations of cells with different biological functions. However, studies on the biological characteristics of BMSCs subpopulations are very limited, possibly because there is no appropriate analysis method, or the cells cannot be classified into reasonable subpopulations. ScRNA-seq is a new method to study the heterogeneity among cells. It can amplify and sequence the transcriptome at the level of a single cell, and then divide the cell population into different clusters by gene expression difference among cells, so that cell type characteristics can be more fully defined. However, there are very few studies reporting this technology to analyze human BMSCs. Liu et al. (13) analyzed different passages of BMSCs from a healthy donor, and they described the changes that occurred in different passages of BMSCs. Their study demonstrated that FGFR2 was likely to be an important marker of early passage BMSCs. PLAT is a key gene associated with a subpopulation more prevalent in late passage BMSCs and that it is a potential marker for BMSC senescence. However, most of the early passage (passage 2) BMSCs probed in our study, expressed PLAT rather than FGFR2, which might mean that, unlike the BMSCs from young people, the BMSCs from elderly people show signs of aging at early passage. Passage 2 BMSCs may be considered early passage cells for the young people, but late passage cells for the elderly people.
Currently, due to the high cost of scRNA-seq, few large sample studies have been conducted. To overcome this shortcoming, we examined 6,514 cells and obtained a set of high-quality sequencing data (Table 1). Similarly, Zhang et al. (21) obtained valuable experimental results by sequencing 5,330 umbilical cord mesenchymal stem cells. The 6,514 BMSCs were divided into three clusters by scRNA-seq and bioinformatics analysis, and the gene expression profile and function of the cells in the same cluster were similar. By performing GO analysis to the top 20 DEGs in each cluster, we found that the biological functions of the three clusters were different, and preliminarily believed that cluster 1 was related to cell proliferation, cluster 2 was related to cell differentiation, and cluster 3 was related to various factors secreted by cells, which also corresponded to the characteristics of BMSCs. Actually, there were more than 20 DEGs in each cluster. In order to facilitate the study, we selected the top 20 DEGs for analysis, which was also the method commonly used in the previous studies (13). The function of the top 20 DEGs of each cluster was checked by NCBI gene database to further verify our hypothesis. And many genes’ function has been experimentally verified by scholars. Some functions of the top 20 DEGs in each cluster are as following:
Functional analysis of some genes in cluster 1: Cell division cycle associated 5 (CDCA5) is a vital regulator of sister chromatid cohesion and separation, and it is involved in the generation and maintenance of sister chromatid cohesion (22). FAM64A is a mitotic regulator which can foster cell metaphase-anaphase transition (23). MYBL2 is a physiological regulator of cell cycle progression, cell survival, and cell differentiation (24). The integrity of the human centromere DNA repeats is protected by CENP-A (25). PAQR4 contributes to modulation of cell proliferation and knockdown of PAQR4 leads to reduction of cell proliferation (26). During DNA replication, the histone chaperone Asf1b is important for Importin-histone recognition (27). HMGB2 as transcriptional activators through the modulation of chromatin structure (28). Chromatin assembly factor-1 (CAF-1) complex can adjust chromatin assembly in DNA replication and repair (29). It can be seen that many DEGs in cluster 1 are related to cell self-renewal.
Functional analysis of some genes in cluster 2: TGM2 is involved in the regulation of chondrogenesis (30). NEAT1 is thought to promote osteogenic differentiation in BMSCs by regulating miR-29b-3p/BMP1 axis (31). COL11A1 plays an essential role during the cartilage development process (32). Type V collagen might be considered as a stromal component that impairs osteogenesis (33). It can be seen that many top 20 DEGs in cluster 2 are related to multidirectional differentiation of BMSCs.
Functional analysis of some genes in cluster 3: Cyba gene encoding p22phox are essential for microbial killing and innate immunity (34). TIMP-1 is a key effector molecule responsible for the antiangiogenic effects of MSC, which is a mechanism to tune down immunity and inflammation (35). Annexin A1 (ANXA1) is an immune-modulating protein with various functions in the immune system (36). Lumican (LUM) affects cell migration and promotes wound repair (37). Dermatopontin (DPT) possesses significant pro-angiogenic properties and plays vital roles in cutaneous wound healing (38). In addition, some genes are involved in regulating cytokine secretion. ERp44 is a pH-regulated chaperone of the secretory pathway and an essential regulator of protein secretion at the ER-Golgi interface (39). The HSPA5 gene encodes the binding immunoglobulin protein is a necessary component of the translocation machinery for protein import into endoplasmic reticulum (40). It can be seen that proteins encoded by some top 20 DEGs in cluster 3 can be used as secretory factors to participate in immune regulation, damage repair, and other processes.
Liu et al. (13) analyzed 36 BMSCs from passage 3 by scRNA-seq and identified three BMSCs subpopulations, too. In their study, the BMSCs were separated into subpopulation A, B, and C. Subpopulation A was characterized by the strong expression of FGFR2. Since FGFR2 was involved in osteogenesis and more likely to be expressed by early passage cells, this subpopulation might include skeletal stem cells. Subpopulation B expressed higher levels of FGF5. FGF5 is a secreted heparin-binding growth factor, which could increase osteogenic differentiation of MSCs. This subpopulation may represent differentiating skeletal stem cell progenitors. Subpopulation C was characterized by strong expression of PLAT and VCAM1. Because VCAM1 promoted angiogenesis, this subpopulation may be involved with angiogenesis. Although both studies divided cells into three subpopulations, each subpopulation corresponds to different characteristic genes. The reasons are multifaceted. Firstly, we studied the elderly, while they studied relatively young individuals. Secondly, it was passage 2 BMSCs that we studied,which was different from the passage 3 BMSCs they studied. And their study proved that BMSC subpopulations change with passage. In addition, they grouped only 36 cells, perhaps because of different sequencing techniques.
This study was limited by the fact that the BMSCs from a single subject. Whether the top 20 DEGs of each subpopulation can be used as its characteristic gene is still up for discussion. With the further work, such as expanding sample size and functional verification, the characteristic genes of each subpopulation will be identified. The verification of DEGs and the sorting of cell subpopulations by these genes will be the next work. In the future, we may analyze each subpopulation in more detail, and further explore the relationship between DEGs and cell phenotypes.
In conclusion, this study demonstrated that BMSCs with high quality could be isolated and cultured from the bone marrow of the affected side femur of elderly hip fracture patients. The BMSCs could be divided into three subpopulations by using scRNA-seq, and the functions of subpopulations were related to self-renewal, multilineage differentiation, and cytokine secretion, respectively. The heterogeneity of BMSCs has been demonstrated. More studies including BMSCs from a wider range of subjects are needed.
Supplementary data including seven figures can be found with this article online at https://doi.org/10.15283/ijsc21042.
Acknowledgments
This work was supported by the special project for innovation of military medical research plan (16CXZ002) and the Beijing Nova Program (xx2018021).
References
1. Samsonraj RM, Raghunath M, Nurcombe V, Hui JH, van Wijnen AJ, Cool SM. 2017; Concise review: multifaceted characterization of human mesenchymal stem cells for use in regenerative medicine. Stem Cells Transl Med. 6:2173–2185. DOI: 10.1002/sctm.17-0129. PMID: 29076267. PMCID: PMC5702523.


2. Hosseini S, Taghiyar L, Safari F, Baghaban Eslaminejad M. 2018; Regenerative medicine applications of mesenchymal stem cells. Adv Exp Med Biol. 1089:115–141. DOI: 10.1007/5584_2018_213. PMID: 29767289.


3. Han ZC, Du WJ, Han ZB, Liang L. 2017; New insights into the heterogeneity and functional diversity of human mesenchymal stem cells. Biomed Mater Eng. 28(s1):S29–S45. DOI: 10.3233/BME-171622. PMID: 28372276.
4. Rennerfeldt DA, Van Vliet KJ. 2016; Concise review: when colonies are not clones: evidence and implications of intracolony heterogeneity in mesenchymal stem cells. Stem Cells. 34:1135–1141. DOI: 10.1002/stem.2296. PMID: 26840390.


5. Tang F, Barbacioru C, Wang Y, Nordman E, Lee C, Xu N, Wang X, Bodeau J, Tuch BB, Siddiqui A, Lao K, Surani MA. 2009; mRNA-Seq whole-transcriptome analysis of a single cell. Nat Methods. 6:377–382. DOI: 10.1038/nmeth.1315. PMID: 19349980.


6. Yan KS, Janda CY, Chang J, Zheng GXY, Larkin KA, Luca VC, Chia LA, Mah AT, Han A, Terry JM, Ootani A, Roelf K, Lee M, Yuan J, Li X, Bolen CR, Wilhelmy J, Davies PS, Ueno H, von Furstenberg RJ, Belgrader P, Ziraldo SB, Ordonez H, Henning SJ, Wong MH, Snyder MP, Weissman IL, Hsueh AJ, Mikkelsen TS, Garcia KC, Kuo CJ. 2017; Non-equivalence of Wnt and R-spondin ligands during Lgr5+ intestinal stem-cell self-renewal. Nature. 545:238–242. DOI: 10.1038/nature22313. PMID: 28467820. PMCID: PMC5641471.


7. Wallrapp A, Riesenfeld SJ, Burkett PR, Abdulnour RE, Nyman J, Dionne D, Hofree M, Cuoco MS, Rodman C, Farouq D, Haas BJ, Tickle TL, Trombetta JJ, Baral P, Klose CSN, Mahlakõiv T, Artis D, Rozenblatt-Rosen O, Chiu IM, Levy BD, Kowalczyk MS, Regev A, Kuchroo VK. 2017; The neuropeptide NMU amplifies ILC2-driven allergic lung inflammation. Nature. 549:351–356. DOI: 10.1038/nature24029. PMID: 28902842. PMCID: PMC5746044.


8. Xiang S, Gao W, Peng H, Liu A, Ao Q, Yang M, Yu Y, Liu Y, Rong R. 2020; Standards of clinical-grade mesenchymal stromal cell preparation and quality control (2020 China Version). J Neurorestoratol. 8:197–216. DOI: 10.26599/JNR.2020.9040021.


9. De Simone M, Rossetti G, Pagani M. 2019; Chromium 10× single-cell 3' mRNA sequencing of tumor-infiltrating lympho-cytes. Methods Mol Biol. 1979:87–110. DOI: 10.1007/978-1-4939-9240-9_7. PMID: 31028634.


10. Tambe A, Pachter L. 2019; Barcode identification for single cell genomics. BMC Bioinformatics. 20:32. DOI: 10.1186/s12859-019-2612-0. PMID: 30654736. PMCID: PMC6337828. PMID: 451d82d819a24aa0ab7aa3244846e3f7.


11. Kivioja T, Vähärautio A, Karlsson K, Bonke M, Enge M, Linnarsson S, Taipale J. 2011; Counting absolute numbers of molecules using unique molecular identifiers. Nat Methods. 9:72–74. DOI: 10.1038/nmeth.1778. PMID: 22101854.


12. Dobin A, Davis CA, Schlesinger F, Drenkow J, Zaleski C, Jha S, Batut P, Chaisson M, Gingeras TR. 2013; STAR: ultrafast universal RNA-seq aligner. Bioinformatics. 29:15–21. DOI: 10.1093/bioinformatics/bts635. PMID: 23104886. PMCID: PMC3530905.


13. Liu S, Stroncek DF, Zhao Y, Chen V, Shi R, Chen J, Ren J, Liu H, Bae HJ, Highfill SL, Jin P. 2019; Single cell sequencing reveals gene expression signatures associated with bone marrow stromal cell subpopulations and time in culture. J Transl Med. 17:23. DOI: 10.1186/s12967-018-1766-2. PMID: 30635013. PMCID: PMC6330466. PMID: 69f3b7086d1a4211b1e73dd4f5f2e7db.


14. Yu D, Huber W, Vitek O. 2013; Shrinkage estimation of dispersion in Negative Binomial models for RNA-seq experiments with small sample size. Bioinformatics. 29:1275–1282. DOI: 10.1093/bioinformatics/btt143. PMID: 23589650. PMCID: PMC3654711.


15. Robinson MD, McCarthy DJ, Smyth GK. 2010; edgeR: a Biocon-ductor package for differential expression analysis of digital gene expression data. Bioinformatics. 26:139–140. DOI: 10.1093/bioinformatics/btp616. PMID: 19910308. PMCID: PMC2796818.


16. Huang H, Chen L, Mao G, Sharma HS. 2020; Clinical neurorestorative cell therapies: developmental process, current state and future prospective. J Neurorestoratol. 8:61–82. DOI: 10.26599/JNR.2020.9040009.


17. Cox CS Jr, Hetz RA, Liao GP, Aertker BM, Ewing-Cobbs L, Juranek J, Savitz SI, Jackson ML, Romanowska-Pawliczek AM, Triolo F, Dash PK, Pedroza C, Lee DA, Worth L, Aisiku IP, Choi HA, Holcomb JB, Kitagawa RS. 2017; Treatment of severe adult traumatic brain injury using bone marrow mononuclear cells. Stem Cells. 35:1065–1079. DOI: 10.1002/stem.2538. PMID: 27800660. PMCID: PMC5367945.


18. Khan H, Mafi P, Mafi R, Khan W. 2018; The effects of ageing on differentiation and characterisation of human mesenchymal stem cells. Curr Stem Cell Res Ther. 13:378–383. DOI: 10.2174/1574888X11666160429122527. PMID: 27133083.


19. Oetjen KA, Lindblad KE, Goswami M, Gui G, Dagur PK, Lai C, Dillon LW, McCoy JP, Hourigan CS. 2018; Human bone marrow assessment by single-cell RNA sequencing, mass cytometry, and flow cytometry. JCI Insight. 3:e124928. DOI: 10.1172/jci.insight.124928. PMID: 30518681. PMCID: PMC6328018.


20. Kim JA, Im KO, Park SN, Kwon JS, Kim SY, Oh K, Lee DS, Kim MK, Kim SW, Jang M, Lee G, Oh YM, Lee SD, Lee DS. 2015; Cytogenetic heterogeneity and their serial dynamic changes during acquisition of cytogenetic aberrations in cultured mesenchymal stem cells. Mutat Res. 777:60–68. DOI: 10.1016/j.mrfmmm.2015.04.003. PMID: 25974687.


21. Zhang S, Wang JY, Li B, Yin F, Liu H. 2021; Single-cell transcriptome analysis of uncultured human umbilical cord mesenchymal stem cells. Stem Cell Res Ther. 12:25. DOI: 10.1186/s13287-020-02055-1. PMID: 33413643. PMCID: PMC7791785. PMID: bf5b4c1f56d0453b955bdaa39992b1f4.


22. Wang J, Xia C, Pu M, Dai B, Yang X, Shang R, Yang Z, Zhang R, Tao K, Dou K. 2018; Silencing of CDCA5 inhibits cancer progression and serves as a prognostic biomarker for hepatocellular carcinoma. Oncol Rep. 40:1875–1884. DOI: 10.3892/or.2018.6579. PMID: 30015982. PMCID: PMC6111608.


23. Yao Z, Zheng X, Lu S, He Z, Miao Y, Huang H, Chu X, Cai C, Zou F. 2019; Knockdown of FAM64A suppresses proliferation and migration of breast cancer cells. Breast Cancer. 26:835–845. DOI: 10.1007/s12282-019-00991-2. PMID: 31264076.


24. Musa J, Aynaud MM, Mirabeau O, Delattre O, Grünewald TG. 2017; MYBL2 (B-Myb): a central regulator of cell proliferation, cell survival and differentiation involved in tumorigenesis. Cell Death Dis. 8:e2895. DOI: 10.1038/cddis.2017.244. PMID: 28640249. PMCID: PMC5520903.


25. Giunta S, Funabiki H. 2017; Integrity of the human centromere DNA repeats is protected by CENP-A, CENP-C, and CENP-T. Proc Natl Acad Sci U S A. 114:1928–1933. DOI: 10.1073/pnas.1615133114. PMID: 28167779. PMCID: PMC5338446.


26. Wang L, Zhang R, You X, Zhang H, Wei S, Cheng T, Cao Q, Wang Z, Chen Y. 2017; The steady-state level of CDK4 protein is regulated by antagonistic actions between PAQR4 and SKP2 and involved in tumorigenesis. J Mol Cell Biol. 9:409–421. DOI: 10.1093/jmcb/mjx028. PMID: 28992327.


27. Soniat M, Cağatay T, Chook YM. 2016; Recognition elements in the histone H3 and H4 tails for seven different importins. J Biol Chem. 291:21171–21183. DOI: 10.1074/jbc.M116.730218. PMID: 27528606. PMCID: PMC5076525.


28. Kimura A, Matsuda T, Sakai A, Murao N, Nakashima K. 2018; HMGB2 expression is associated with transition from a quiescent to an activated state of adult neural stem cells. Dev Dyn. 247:229–238. DOI: 10.1002/dvdy.24559. PMID: 28771884.


29. Cheloufi S, Elling U, Hopfgartner B, Jung YL, Murn J, Ninova M, Hubmann M, Badeaux AI, Euong Ang C, Tenen D, Wesche DJ, Abazova N, Hogue M, Tasdemir N, Brumbaugh J, Rathert P, Jude J, Ferrari F, Blanco A, Fellner M, Wenzel D, Zinner M, Vidal SE, Bell O, Stadtfeld M, Chang HY, Almouzni G, Lowe SW, Rinn J, Wernig M, Aravin A, Shi Y, Park PJ, Penninger JM, Zuber J, Hochedlinger K. 2015; The histone chaperone CAF-1 safeguards somatic cell identity. Nature. 528:218–224. DOI: 10.1038/nature15749. PMID: 26659182. PMCID: PMC4866648.


30. Kanawa M, Igarashi A, Fujimoto K, Higashi Y, Kurihara H, Sugiyama M, Saskianti T, Kato Y, Kawamoto T. 2018; Genetic markers can predict chondrogenic differentiation potential in bone marrow-derived mesenchymal stromal cells. Stem Cells Int. 2018:9530932. DOI: 10.1155/2018/9530932. PMID: 30405725. PMCID: PMC6199884.


31. Zhang Y, Chen B, Li D, Zhou X, Chen Z. 2019; LncRNA NEAT1/miR-29b-3p/BMP1 axis promotes osteogenic differentiation in human bone marrow-derived mesenchymal stem cells. Pathol Res Pract. 215:525–531. DOI: 10.1016/j.prp.2018.12.034. PMID: 30638953.


32. Narakornsak S, Aungsuchawan S, Pothacharoen P, Markmee R, Tancharoen W, Laowanitwattana T, Thaojamnong C, Peerapapong L, Boonma N, Tasuya W, Keawdee J, Poovachiranon N. 2017; Sesamin encouraging effects on chondrogenic differentiation of human amniotic fluid-derived mesenchymal stem cells. Acta Histochem. 119:451–461. DOI: 10.1016/j.acthis.2017.04.006. PMID: 28499502.


33. Longo A, Tobiasch E, Luparello C. 2014; Type V collagen counteracts osteo-differentiation of human mesenchymal stem cells. Biologicals. 42:294–297. DOI: 10.1016/j.biologicals.2014.07.002. PMID: 25132375.


34. Belambri SA, Rolas L, Raad H, Hurtado-Nedelec M, Dang PM, El-Benna J. 2018; NADPH oxidase activation in neutrophils: Role of the phosphorylation of its subunits. Eur J Clin Invest. 48 Suppl 2:e12951. DOI: 10.1111/eci.12951. PMID: 29757466.


35. Maffioli E, Nonnis S, Angioni R, Santagata F, Calì B, Zanotti L, Negri A, Viola A, Tedeschi G. 2017; Proteomic analysis of the secretome of human bone marrow-derived mesenchymal stem cells primed by pro-inflammatory cytokines. J Proteomics. 166:115–126. DOI: 10.1016/j.jprot.2017.07.012. PMID: 28739509.


36. Moraes LA, Ampomah PB, Lim LHK. 2018; Annexin A1 in inflammation and breast cancer: a new axis in the tumor microenvironment. Cell Adh Migr. 12:417–423. DOI: 10.1080/19336918.2018.1486143. PMID: 30122097. PMCID: PMC6363057.


37. Akram KM, Samad S, Spiteri MA, Forsyth NR. 2013; Mesenchymal stem cells promote alveolar epithelial cell wound repair in vitro through distinct migratory and paracrine mechanisms. Respir Res. 14:9. DOI: 10.1186/1465-9921-14-9. PMID: 23350749. PMCID: PMC3598763.


38. Krishnaswamy VR, Balaguru UM, Chatterjee S, Korrapati PS. 2017; Dermatopontin augments angiogenesis and modulates the expression of transforming growth factor beta 1 and integrin alpha 3 beta 1 in endothelial cells. Eur J Cell Biol. 96:266–275. DOI: 10.1016/j.ejcb.2017.02.007. PMID: 28336087.


39. Watanabe S, Harayama M, Kanemura S, Sitia R, Inaba K. 2017; Structural basis of pH-dependent client binding by ERp44, a key regulator of protein secretion at the ER-Golgi interface. Proc Natl Acad Sci U S A. 114:E3224–E3232. DOI: 10.1073/pnas.1621426114. PMID: 28373561. PMCID: PMC5402435.


40. Wang J, Lee J, Liem D, Ping P. 2017; HSPA5 Gene encoding Hsp70 chaperone BiP in the endoplasmic reticulum. Gene. 618:14–23. DOI: 10.1016/j.gene.2017.03.005. PMID: 28286085. PMCID: PMC5632570.


Fig. 1
Expression of BMSCs markers by flow cytometry analysis and scRNA-seq. (a) Expression of surface marker antigens of the BMSCs. (b) Expression patterns of characteristic marker genes were projected on the t-SNE plot. (c) Expression patterns of marker gene for early passage BMSCs, FGFR2, and late passage BMSCs, PLAT, were projected on the t-SNE plot. Each cell is coloured based on the expression level of the indicated marker gene.
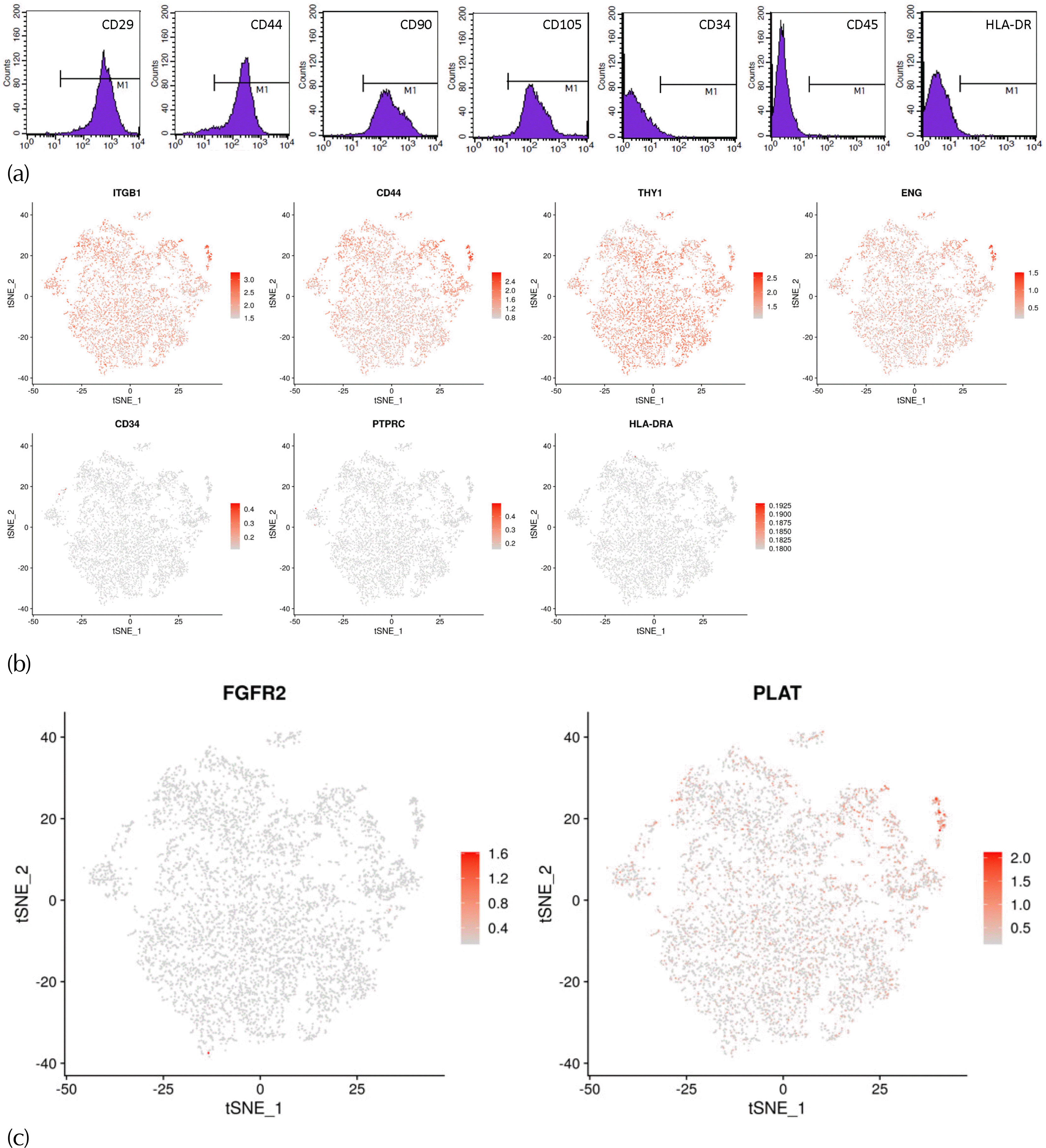
Fig. 2
The barcode on the left of the green marker line is the valid cell, and the Barcode on the right is the background. The figure on the right of the marker line has a steep downward trend, indicating that the effective cells are well differentiated from the background. The intersection point between the marker line and the abscissa is the effective cell number.
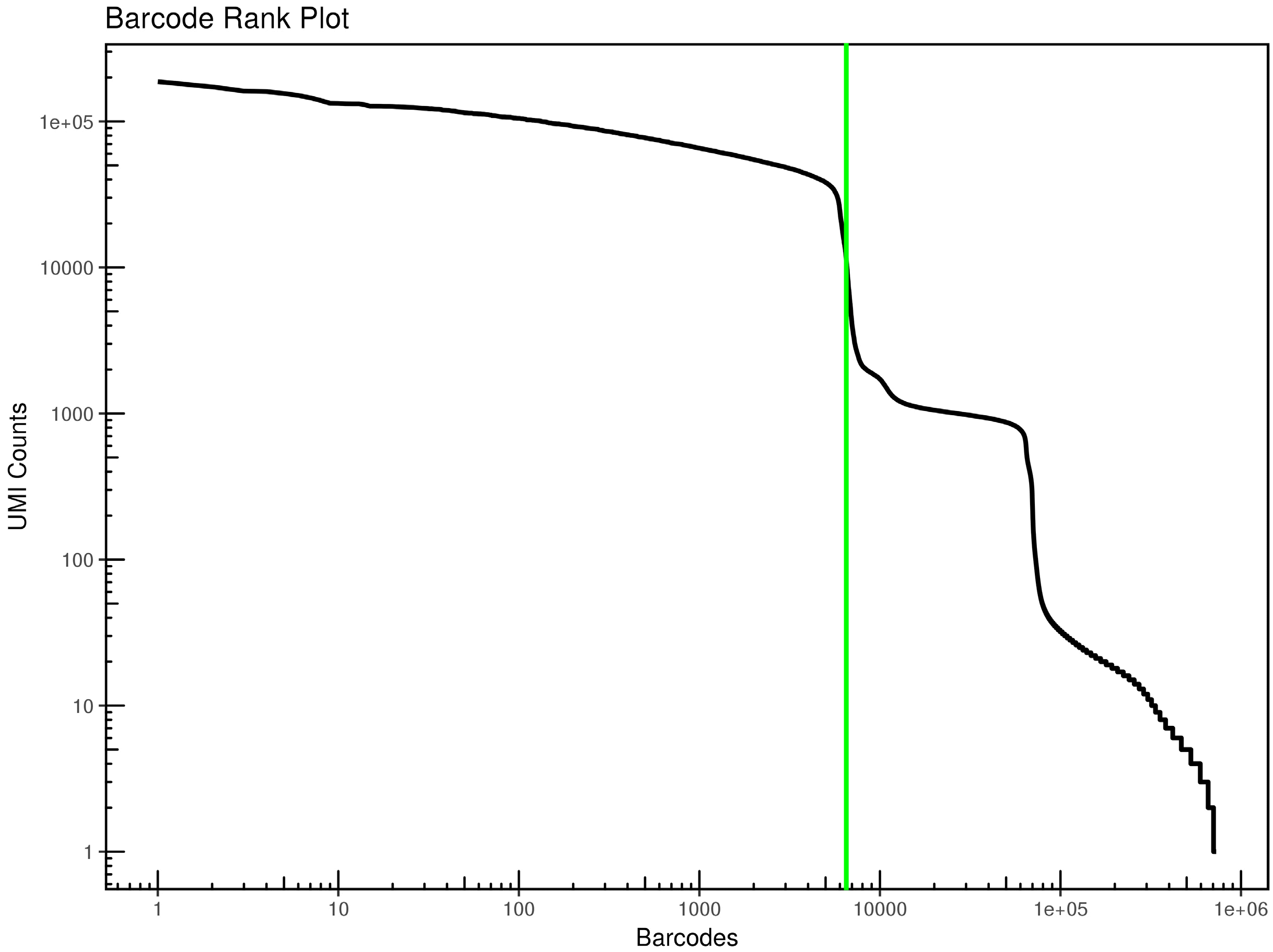
Fig. 3
T-SNE projection of cells colored by clustering. Each dot in the figure represents a cell. The legend on the right is the name of the cluster. Different colors represent different clusters.
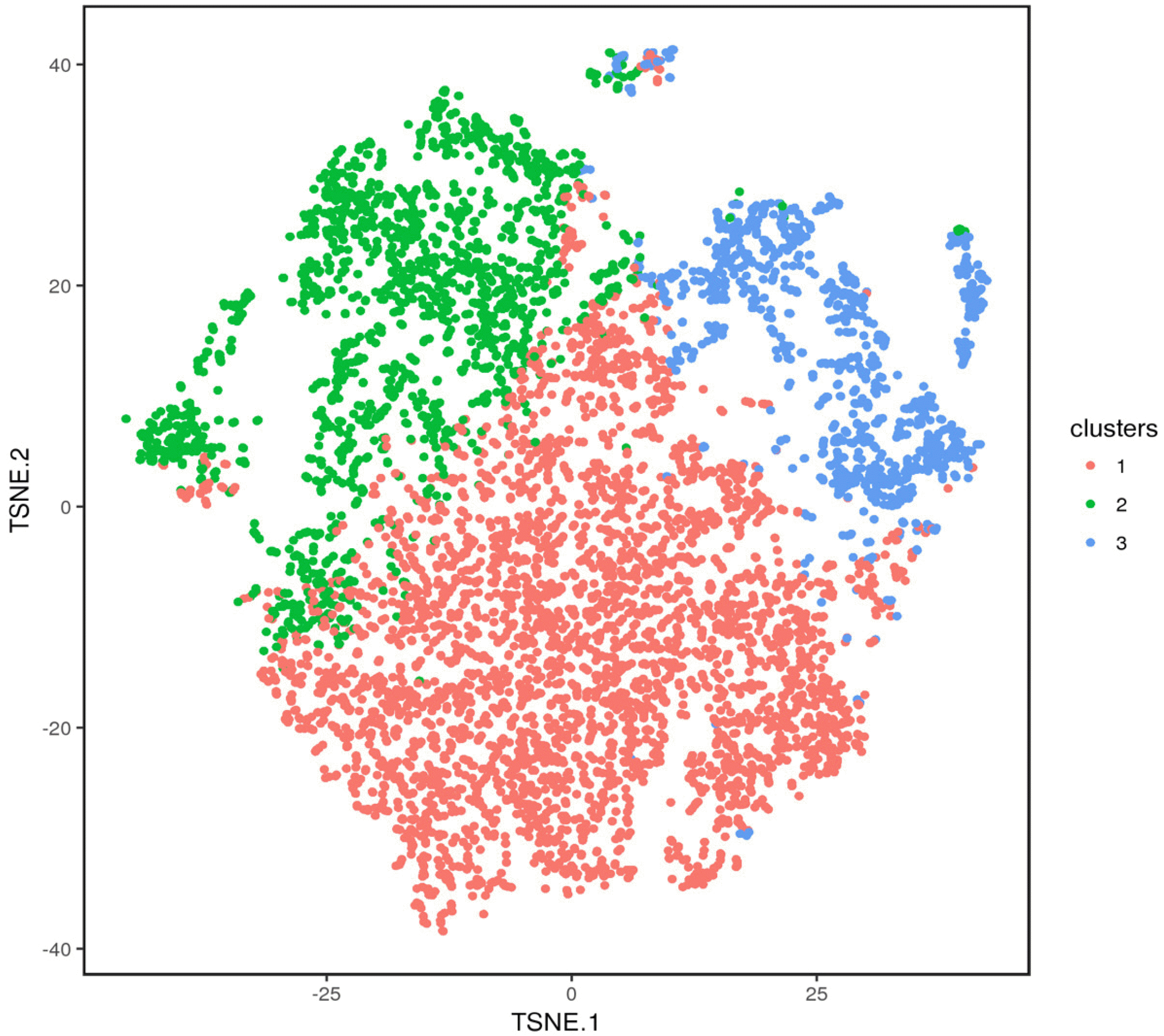
Fig. 4
Clustering heat map of top 20 DEGs corresponding to the three clusters. Each column in the figure represents a gene and each row represents a cluster. Each small area color in the heat map represents the average expression level of the corresponding gene in the cluster. As shown in the right legend, the color from red to blue represents the gene expression from high to low. Different clusters are distinguished by different colors in the first line.
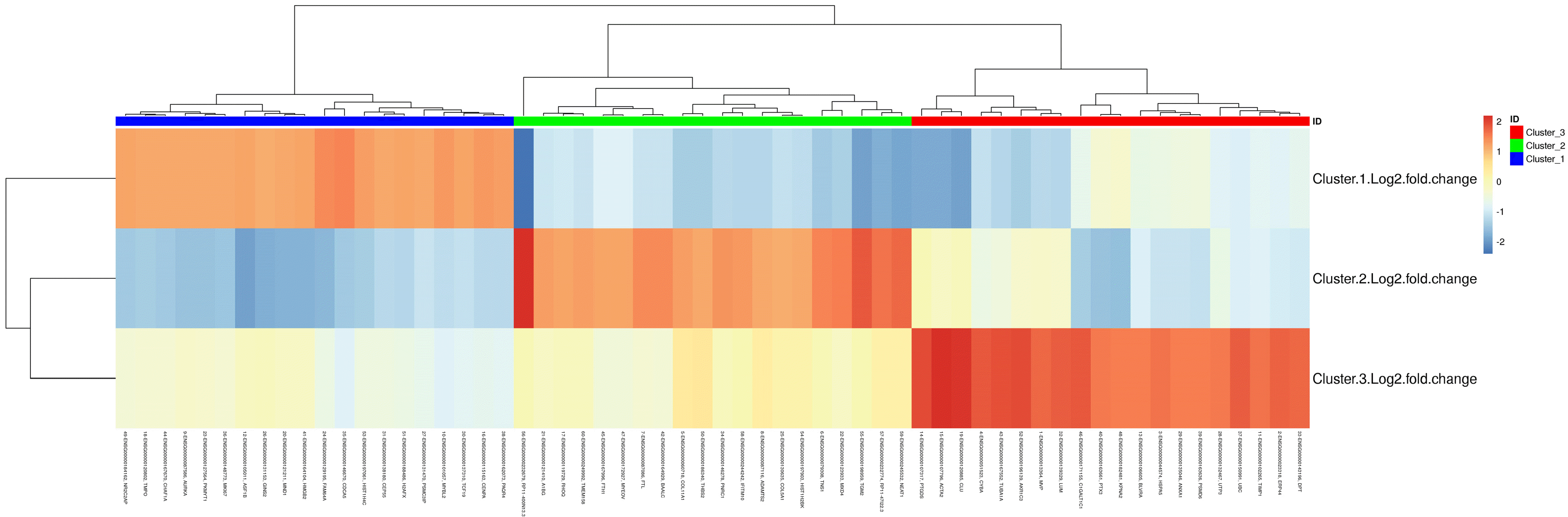
Table 1
Summary statistics for the sequencing data of the BMSCs
Table 2
Log2FC and p-value corresponding to the top 20 DEGs of the three clusters