Abstract
This study describes an objective approach to deriving the clinical performance of autoverification rules to inform laboratory practice when implementing them. Anonymized historical laboratory data for 12 biochemistry measurands were collected and Box-Cox-transformed to approximate a Gaussian distribution. The historical laboratory data were assumed to be error-free. Using the probability theory, the clinical specificity of a set of autoverification limits can be derived by calculating the percentile values of the overall distribution of a measurand. The 5th and 95th percentile values of the laboratory data were calculated to achieve a 90% clinical specificity. Next, a predefined tolerable total error adopted from the Royal College of Pathologists of Australasia Quality Assurance Program was applied to the extracted data before subjecting to Box-Cox transformation. Using a standard normal distribution, the clinical sensitivity can be derived from the probability of the Z-value to the right of the autoverification limit for a one-tailed probability and multiplied by two for a two-tailed probability. The clinical sensitivity showed an inverse relationship with between-subject biological variation. The laboratory can set and assess the clinical performance of its autoverification rules that conforms to its desired risk profile.
A laboratory quality system can be built upon a multilayer, interlinking electronic rule system (e.g. delta check, autoverification rules, linearity range check, serum indices check) to minimize the risk of errors and ensure patient safety. The quality system may include autoverification limits that check for gross errors at the individual result level [1-5]. Additionally, it may include delta checks, in which the current patient result is compared to the preceding one for significant differences, indicating an error [6–10]. Patient-based quality control can be applied for the detection of population-level shifts and/or drifts [11-14].
Laboratory practitioners must understand the clinical sensitivity and specificity of implemented autoverification rules to make an informed decision pertaining to the clinical risk. Currently, there exists a paucity of evidence-based approach on defining the clinical sensitivity and specificity of autoverification rules. We describe a simple approach to objectively define the clinical performance of laboratory-specific autoverification limits. This study was undertaken as part of an effort to optimize the autoverification limits and was exempted from ethics approval (Domain-Specific Review Board, Singapore; reference number: 2016/01115).
The definitions of autoverification limits and clinical performance are provided below:
Autoverification limits: Numerical threshold values within which the laboratory results are assumed to not contain significant/gross errors and are safe to be released without scientific or clinical review.
Clinical specificity: Probability of the chosen set of autoverification limits to correctly identify results without errors, calculated as: true negatives/(true negatives + false positives).
Clinical sensitivity: Probability of the chosen set of autoverification limits to correctly identify results with a predefined magnitude of bias, calculated as: true positives/(true positives + false negatives).
Anonymized historical data for 12 general biochemistry serum measurands from a laboratory serving community-ambulatory subjects (National University Hospital, Kent Ridge, Singapore) were collected as previously described [15]. The data were assumed to be error-free and were Box-Cox-transformed to approximate a Gaussian distribution using an automated online tool with default settings (http://www.wessa.net/rwasp_boxcoxnorm.wasp) [16].
As the laboratory data were assumed to be error-free, results falling within any selected autoverification limits were considered true negatives, whereas those falling outside the limits were considered false positives (Fig. 1A). When the percentile values of the overall data distribution are used as autoverification limits, this coincides with the clinical specificity. For example, if the 5th and 95th percentile values of the overall data distribution are taken as the autoverification limits, the clinical specificity of this set of autoverification limits will be 90%. If only one side of the autoverification limit is set, e.g., the 95th percentile value, the clinical specificity will be 95% [6, 7]. We selected a clinical specificity of 90% to maintain the false alarm rate at 10%.
Once the autoverification limits fulfilling the predefined clinical specificity have been determined and the magnitude of tolerable total error for the autoverification rules to be assessed against has been defined, the clinical sensitivity can be determined. The analytical performance specifications of the Royal College of Pathologists Australasia Quality Assurance Program were selected as the source of tolerable total error for 12 measurands [17]. The total error was applied to the extracted laboratory data before being Box-Cox transformed. The transformed laboratory data exceeding the autoverification limits were considered true positive, whereas those falling within the limits were considered false negative (Fig. 1B).
For a standard normal distribution with a positive bias, the clinical sensitivity for a one-tailed detection can be obtained by examining the probability of observing a Z-value that is higher than the Z-value corresponding to the autoverification limit for the error-free distribution. This is mathematically represented as:
clinical sensitivity (one-tailed)=probability
[Z>critical Z-value at upper autoverification limits].
For a two-tailed detection, the probability is multiplied by two as the positive and negative bias can be detected by the upper and lower autoverification limits, respectively. A step-by-step protocol for alanine aminotransferase as an example is provided in Fig. 2. The other 11 measurands were analyzed in the same manner. The data for the 12 general biochemistry measurands required variable degrees of Box-Cox transformation, with optimal lambdas ranging from –0.66 to 2.00 (Table 1) [16]. The 5th and 95th percentile values (corresponding to a clinical specificity of 90%) of the transformed distribution were determined (Table 1). The clinical sensitivities at the autoverification limits derived from the laboratory data after the application of the tolerable total error are summarized in Table 1.
In general, the clinical sensitivities were inversely correlated with between-subject biological variation (Supplemental Data Fig. S1). Serum chloride, with the smallest between-subject biological variation (1.3%), had the highest (two-tailed) clinical sensitivity (100%), whereas γ-glutamyltransferase, with the largest between-subject biological variation (44.5%), had the lowest clinical sensitivity (6.1%). A relatively high clinical sensitivity is required for the autoverification limits (e.g., 80%–95%) to maintain a low false-negative (missed-detection) rate [18].
The combination of an increasingly sophisticated laboratory information technology, the ability to generate large volumes of laboratory data using automation, and the increasing clinical demands for ever shorter sample-to-result times, necessitating a means to release patient results in a timely manner, while ensuring patient safety. In response, autoverification limits have been conceived as an electronic means to release patient results efficiently, without prior scientific or clinical review. The application of autoverification limits has gained significant traction in laboratory practice and is one of the most commonly implemented electronic laboratory rules [1–5].
Several approaches have been described to determine autoverification limits, with the most simplistic approach being setting the reference interval as the autoverification limits [1–5]. The assumption here is that any laboratory results within this interval should not elicit a significant clinical reaction, and they are considered sufficiently benign to be released without manual review. These limits are used primarily to rule out results that may be associated with significant errors. There are also approaches that extend the autoverification limits beyond reference intervals into what could be regarded physiologically extreme ranges, e.g., critical values or physiologically improbable ranges [19]. The latter includes values that are generally not compatible with life (e.g., potassium >8 mmol/L). In this case, the autoverification is geared toward identifying results that likely are associated with gross significant errors. Lastly, autoverification limits can be set based on professional opinion.
The above approaches do not directly inform laboratory practitioners of the clinical performance of the applied autoverification rules, particularly, the clinical sensitivity and its associated false-negative rate. This may lead to the setting of autoverification rules that do not match the acceptable clinical risk profile of the laboratory. The approach described here leverages on the simple concept of probability theory and Z-values, which we have previously applied in the context of delta checks [7]. Clinical specificity and sensitivity have an inverse relationship, and careful tradeoffs must be considered when deciding upon the limits. The need for operational efficiency to return results in a timely manner has often led to laboratories adopting wider autoverification limits (i.e., favoring higher specificity). This study clearly demonstrated the impact of between-subject biological variation on clinical sensitivity. For measurands that have a wide between-subject biological variation, the implementation of wider autoverification limits (i.e., higher specificity) may translate into poorer clinical sensitivity and the inability to direct results with potential errors for manual review.
Our simple approach should help laboratory practitioners consider the risk profile and clinical impacts in the selection and implementation of autoverification limits. A Microsoft Excel tool for determining clinical specificity and clinical sensitivity is included in the Supplemental Data.
Notes
REFERENCES
1. Shih MC, Chang HM, Tien N, Hsiao CT, Peng CT. 2011; Building and validating an autoverification system in the clinical chemistry laboratory. Lab Med. 42:668–73. DOI: 10.1309/LM5AM4IIXC4OIETD.


2. Randell EW, Yenice S, Khine Wamono AA, Orth M. 2019; Autoverification of test results in the core clinical laboratory. Clin Biochem. 73:11–25. DOI: 10.1016/j.clinbiochem.2019.08.002. PMID: 31386832.


3. Feitosa MS, Bücker DH, Santos SME, Vasconcellos LS. 2016; Implementation of criteria for automatic release of clinical chemistry test results in a laboratory at an academic public hospital. J Bras Patol Med Lab. 52:149–56. DOI: 10.5935/1676-2444.20160026.


4. van Rossum HH. 2020; An approach to selecting auto-verification limits and validating their error detection performance independently for pre-analytical and analytical errors. Clin Chim Acta. 508:130–6. DOI: 10.1016/j.cca.2020.05.026. PMID: 32416173.


5. Fraser CG, Stevenson HP, Kennedy IMG. 2002; Biological variation data are necessary prerequisites for objective autoverification of clinical laboratory data. Accredit Qual Assur. 7:455–60. DOI: 10.1007/s00769-002-0526-3.


6. Tan RZ, Markus C, Choy KW, Doery JCG, Loh TP. 2020; Optimized delta check rules for detecting misidentified specimens in children. Am J Clin Pathol. 153:605–12. DOI: 10.1093/ajcp/aqz201. PMID: 31889173.


7. Markus C, Tan RZ, Loh TP. 2021; Evidence-based approach to setting delta check rules. Crit Rev Clin Lab Sci. 58:49–59. DOI: 10.1080/10408363.2020.1800585. PMID: 32795201.


8. Tan RZ, Markus C, Loh TP. 2020; Impact of delta check time intervals on error detection capability. Clin Chem Lab Med. 58:384–9. DOI: 10.1515/cclm-2019-1004. PMID: 31734649.


9. Tan RZ, Markus C, Loh TP. 2020; Relationship between biological variation and delta check rules performance. Clin Biochem. 80:42–7. DOI: 10.1016/j.clinbiochem.2020.03.017. PMID: 32247779.


10. Tan RZ, Markus C, Loh TP. 2020; An approach to optimize delta checks in test panels-the effect of the number of rules included. Ann Clin Biochem. 57:215–22. DOI: 10.1177/0004563220904749. PMID: 31955587.


11. Badrick T, Bietenbeck A, Cervinski MA, Katayev A, van Rossum HH, Loh TP, et al. 2019; Patient-based real-time quality control: review and recommendations. Clin Chem. 65:962–71. DOI: 10.1373/clinchem.2019.305482. PMID: 31263035.


12. Badrick T, Bietenbeck A, Katayev A, van Rossum HH, Loh TP, Cervinski MA, et al. 2020; Implementation of patient-based real-time quality control. Crit Rev Clin Lab Sci. 57:532–47. DOI: 10.1080/10408363.2020.1765731. PMID: 32486872.
13. Badrick T, Cervinski M, Loh TP. 2019; A primer on patient-based quality control techniques. Clin Biochem. 64:1–5. DOI: 10.1016/j.clinbiochem.2018.12.004. PMID: 30550876.


14. Loh TP, Bietenbeck A, Cervinski MA, van Rossum HH, Katayev A, Badrick T, et al. 2020; Recommendation for performance verification of patient-based real-time quality control. Clin Chem Lab Med. 58:1205–13. DOI: 10.1515/cclm-2019-1024. PMID: 32049646.


15. Liu J, Tan CH, Loh TP, Badrick T. 2016; Verification of out-of-control situations detected by "average of normal" approach. Clin Biochem. 49:1248–53. DOI: 10.1016/j.clinbiochem.2016.07.012. PMID: 27452179.


16. Wessa P. Box-Cox Normality Plot (v.1.1.12) in Free Statistics Software, Office for Research Development and Education. http://www.wessa.net/rwasp_boxcoxnorm.wasp. 2016. Updated on Aug 2021.
17. The Royal College of Pathologists of Australasia quality assurance programs. Chemical pathology analytical performance specifications. https://rcpaqap.com.au/resources/chemical-pathology-analytical-performance-specifications/. Updated on Aug 2021.
18. Loh TP, Lord SJ, Bell K, Bohn MK, Lim CY, Markus C, et al. 2021; Setting minimum clinical performance specifications for tests based on disease prevalence and minimum acceptable positive and negative predictive values: practical considerations applied to COVID-19 testing. Clin Biochem. 88:18–22. DOI: 10.1016/j.clinbiochem.2020.11.003. PMID: 33227233. PMCID: PMC7678449.


19. Krasowski MD, Davis SR, Drees D, Morris C, Kulhavy J, Crone C, et al. 2014; Autoverification in a core clinical chemistry laboratory at an academic medical center. J Pathol Inform. 5:13. DOI: 10.4103/2153-3539.129450. PMID: 24843824. PMCID: PMC4023033.


Fig. 1
Diagram showing the determination of overall (A) clinical specificity and (B) sensitivity from laboratory data that have been transformed to approximate a Gaussian distribution. The dotted line in panel (B) represents the original distribution before addition of the positive error.
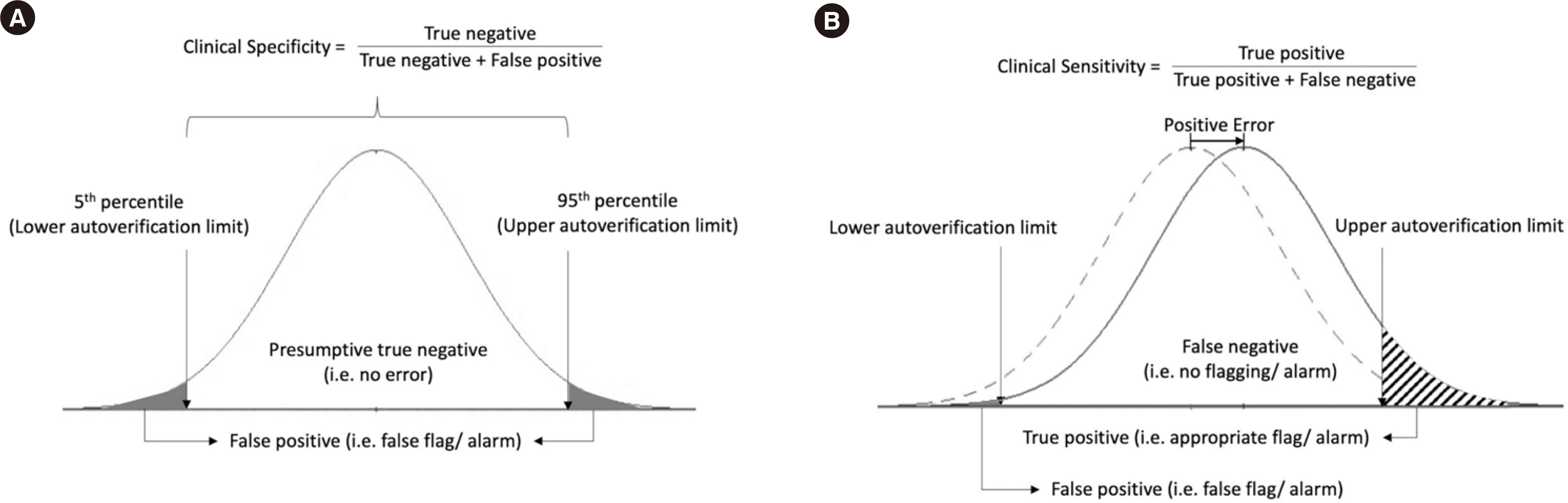
Fig. 2
Step-by-step protocol for determining clinical specificity and sensitivity and autoverification limits for serum alanine aminotransferase as an example.
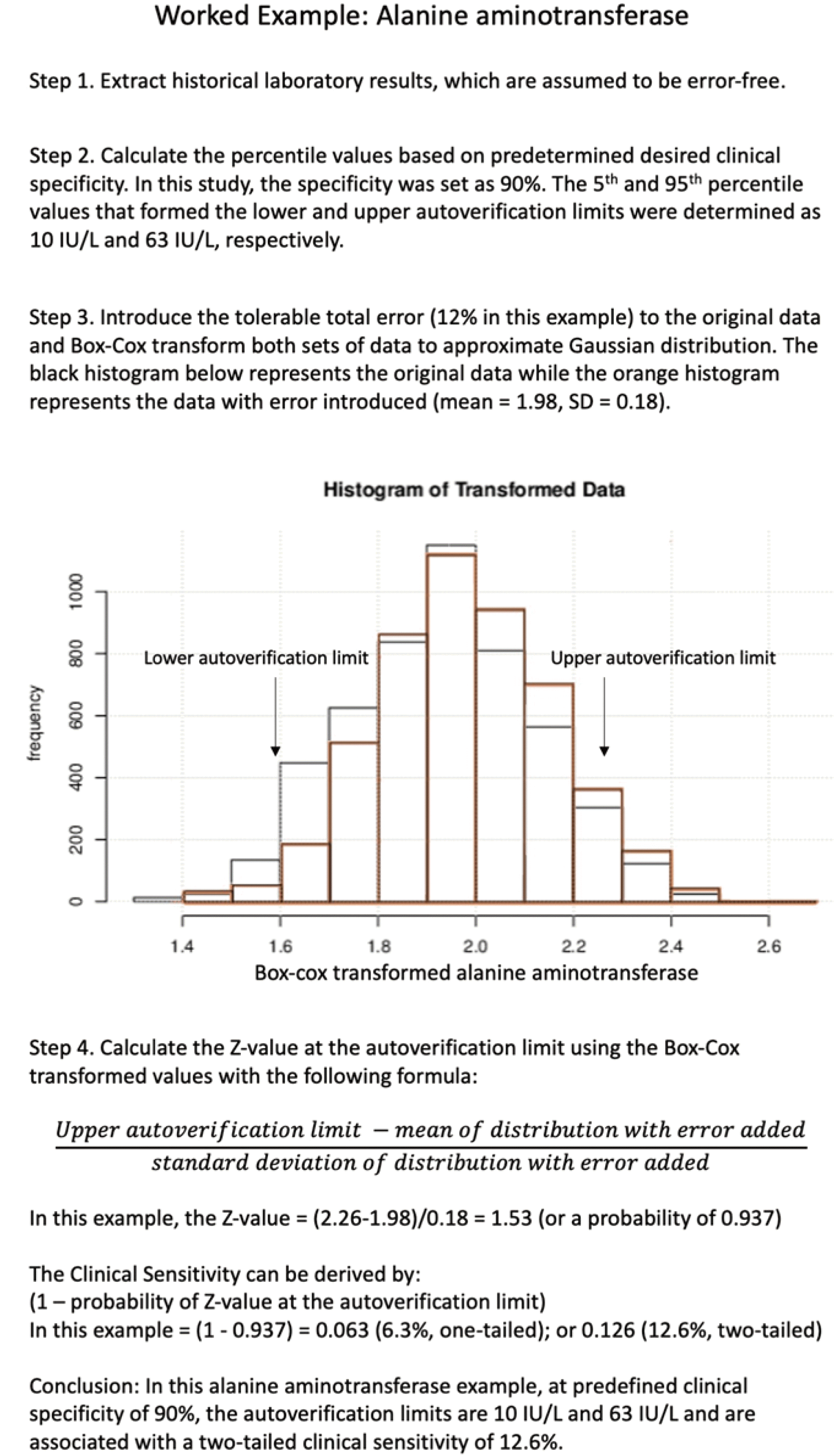
Table 1
Summary of optimal lambdas for Box-Cox transformation, lower and upper autoverification limits at 90% clinical specificity, and Z-values and corresponding probabilities (the one-tailed clinical sensitivity was calculated as one-probability) after application of the tolerable total error
Abbreviations: RCPAQAP APS, Royal College of Pathologists Australasia Quality Assurance Programs, analytical performance specifications; CVg, between-subject biological variation (obtained from https://biologicalvariation.eu/).