Abstract
Background/Aims
Colonoscopy is the gold standard diagnostic method for colorectal neoplasia, allowing detection and resection of adenomatous polyps; however, significant proportions of adenomas are missed. Computer-aided detection (CADe) systems in endoscopy are currently available to help identify lesions. Diminutive (≤5 mm) and nonpedunculated polyps are most commonly missed. This meta-analysis aimed to assess whether CADe systems can improve the real-time detection of these commonly missed lesions.
Methods
A comprehensive literature search was performed. Randomized controlled trials evaluating CADe systems categorized by morphology and lesion size were included. The mean number of polyps and adenomas per patient was derived. Independent proportions and their differences were calculated using DerSimonian and Laird random-effects modeling.
Results
Seven studies, including 2,595 CADe-assisted colonoscopies and 2,622 conventional colonoscopies, were analyzed. CADe-assisted colonoscopy demonstrated an 80% increase in the mean number of diminutive adenomas detected per patient compared with conventional colonoscopy (0.31 vs. 0.17; effect size, 0.13; 95% confidence interval [CI], 0.09–0.18); it also demonstrated a 91.7% increase in the mean number of nonpedunculated adenomas detected per patient (0.32 vs. 0.19; effect size, 0.05; 95% CI, 0.02–0.07).
Colorectal cancer is the third most common malignancy worldwide. Its prevalence continues to increase, with a projected 2.2 million cases and 1.1 million deaths in 2030.1 The adenoma-carcinoma sequence is well established with adenomas being the precursor lesion to 90% of colorectal cancer.2,3
Colonoscopy remains the gold standard diagnostic method for the detection and resection of neoplastic lesions. National colonoscopy-based screening programs have been proven to reduce the 10-year colorectal cancer risk.4,5 However, it is not possible to achieve 100% mucosal visualization with a standard colonoscope in a clean colon. This presents several limitations, including missed polyps. A meta-analysis including 15,000 tandem colonoscopies calculated a 26% adenoma miss rate.6
It has been shown that a 1% increase in the adenoma detection rate (ADR) decreases the cancer risk by 3%.7 A 2018 World Health Organization consensus defined postcolonoscopy colorectal cancer (PCCRC) as “cancer appearing after a colonoscopy in which no cancer is diagnosed.”8 A single-center retrospective study conducted over an 8-year period found that 85% of PCCRC was attributed to missed lesions during colonoscopy.9 A recent meta-analysis showed significantly higher adenoma miss rates in diminutive (≤5 mm) and nonpedunculated adenomas than in larger and pedunculated adenomas.6
Advances in technology and healthcare in recent years, including the introduction of high-definition endoscopes,10 strict quality performance indicators, and adjuncts, such as Endocuff (Arc Medical Design, Southend-on-Sea, UK), have improved the ADR. Progress in endoscopic therapeutic capabilities has also extended the types of lesions that are potentially resectable by endoscopic submucosal dissection.11
More recently, computer-aided detection (CADe) systems in endoscopy, which are based on convolutional neural networks, have been validated and made commercially available. This novel technology has been shown to detect polyps in real time with greater diagnostic accuracy than standard colonoscopy and yields a higher ADR.12
Previous studies have retrospectively assessed the application of CADe systems to endoscopic images and videos in a research setting. More recently, meta-analyses have assessed the impact of the use of CADe systems on the ADR in real time.12-14 However, unlike other studies, our meta-analysis aimed to provide an up-to-date analysis of the impact of the use of real-time CADe systems on the detection of most commonly missed polyps, including nonpedunculated and diminutive lesions.
This review was conducted and reported in accordance with the Preferred Reporting Items for Systematic Reviews and Meta-Analyses (PRISMA) statement.15
A comprehensive literature search of articles published until October 2020 was conducted. The databases used included Embase, Medline, and the Cochrane Library. The search terms used in Embase and Medline included “colon*,” “polyp,” “adenoma,” “artificial intelligence OR machine learning,” and “computer-aided or -assisted and diagnosis* OR detect*.” The search terms used in the Cochrane Library were “colonic polyp,” “artificial intelligence,” and “diagnosis, computer-assisted” (Appendix 1).
The primary outcomes of this study were the mean number of adenomas per patient (MAP) and mean number of polyps per patient (MPP) for diminutive (≤5 mm) and nonpedunculated (flat or sessile) lesions.
The mean number of lesions per patient was calculated rather than the detection rate in this study. This is attributed to the data available in the analyzed studies. However, there is good evidence that the mean percentage of lesions per patient correlates with the polyp detection rate (PDR) and ADR.16,17
The inclusion criteria were as follows: (1) randomized controlled studies reporting CADe of colorectal polyps during real-time colonoscopy; (2) studies reporting the MPP and MAP or studies with adequate information to calculate these data; (3) studies reporting the detection rate of polyps or adenomas according to morphology, size, and location; (4) studies reporting the ADR or PDR of CADe systems during real-time colonoscopy; and (5) studies published or translated into English.
The exclusion criteria were as follows: (1) studies with no original data present (e.g., review article or letter); (2) studies with no full-text available; (3) studies conducted in patients with inflammatory bowel disease; (4) studies published more than 20 years ago; (5) studies without adequate data to calculate the PDR, ADR, MPP, and MAP or studies not reporting these data; and (6) studies with overlapping data to the largest and latest datasets from centers publishing multiple studies.
Two authors (SN and AS) reviewed the articles. Among the retrieved articles, duplicates were excluded. Titles and abstracts were independently screened for relevance. Studies that were irrelevant to the research questions were excluded. The remaining articles were reviewed in full, and the reference lists were scrutinized for relevant studies. Disputed articles were settled via consensus between SN and AS after screening and full-text review. All included articles met the inclusion criteria.
Data were gathered from the studies using a standard spreadsheet template. For each study, we extracted the following data: study details (first author, year of publication, and journal), study design (type of study, method of artificial intelligence [AI], and exclusion criteria), and polyp characteristics (MPP, MAP, morphology, size, and location). Data were also gathered for the overall ADR and PDR in each study.
Study quality was independently assessed using the Jadad scale for randomized controlled trials (RCTs).18 Studies with a Jadad scale score of 3 or more were considered to be of “good” quality.
The MPP and MAP by morphology and size were calculated by dividing the total number of lesions by the number of patients in each group. The mean detection rates per patient are known to correlate well with the PDR and ADR.17
Independent proportions and their differences were calculated and pooled using DerSimonian and Laird random-effects modeling. This considered both between-study and within-study variances, which contributed to study weighting. Pooled values and 95% confidence intervals (CIs) were computed and presented in forest plots. Statistical heterogeneity was determined using I2 statistics, where an I2 value of <30% was considered low; 30%–60%, moderate; and >60%, high. Analyses were performed using Stata ver. 15 (StataCorp LP, College Station, TX, USA). The p-values of ≤0.05 were considered statistically significant.
A total of 899 articles were identified from the database searches. After removal of duplicates, 575 records were screened on the basis of their titles and abstracts. A total of 141 articles were identified as appropriate for full-text review. Further evaluation and application of the exclusion criteria yielded seven studies that were eligible for inclusion in this meta-analysis. The study screening and selection process are illustrated in Figure 1.
All seven studies used the CADe systems in real time.19-24 Sufficient data were available from six studies for the analysis of the mean number of diminutive polyps per patient and from five studies for the analysis of the mean number of diminutive adenomas per patient. Four studies assessed the mean number of nonpedunculated polyps per patient, while six studies reported the mean number of nonpedunculated adenomas per patient. One study did not report adenoma detection but only analyzed polyp detection.20
Sufficient data for calculating the MPP, MAP, mean number of diminutive polyps and adenomas per patient, and mean number of nonpedunculated polyps and adenomas per patient were available in all studies. A total of 3,052 polyps and 1,847 adenomas were reported in 5,028 patients. The study characteristics are listed in Table 1.19-25 A summary of the results is provided in Table 2.
Six studies identified 1,557 diminutive polyps in the CADe group (2,254 patients) and 849 diminutive polyps in the control group (2,278 patients). This equated to 0.691 diminutive polyps per patient in the CADe group compared with 0.373 in the control group, with a pooled effect size of 0.30 (95% CI, 0.19–0.42). This corresponded to an increase in the detection rate of diminutive polyps with CADe of 85.3%.
Five studies identified 680 diminutive adenomas in the CADe group (2,177 patients) and 381 diminutive adenomas in the control group (2,196 patients). There were 0.312 diminutive adenomas per patient in the CADe group and 0.173 in the conventional colonoscopy group, resulting in a pooled effect size of 0.13 (95% CI, 0.09–0.18) (Fig. 2). CADe-assisted colonoscopy detected an 80.0% higher number of diminutive adenomas than did conventional colonoscopy.
A total of 1,769 nonpedunculated lesions were identified in four studies, with 1,158 in the CADe group (1,337 patients) and 611 in the control group (1,393 patients). This equated to 0.841 nonpedunculated polyps per patient in the CADe group and 0.439 nonpedunculated polyps in the conventional colonoscopy group. An increase of 91.7% in the detection of nonpedunculated polyps was observed with the use of CADe in these studies. A pooled effect size of 0.39 (95% CI, 0.35–0.44) was generated in the analysis of nonpedunculated lesions between the CADe and conventional colonoscopy groups.
In six studies, 818 nonpedunculated adenomas were recorded in the CADe group (2,518 patients) and 477 in the control group (2,540 patients). This equated to 0.325 nonpedunculated adenomas per patient in the CADe group compared with 0.189 in the control group, with a pooled effect size of 0.05 (95% CI, 0.02–0.07) (Fig. 3). CADe-assisted colonoscopy detected a 71.5% higher number of nonpedunculated adenomas than did conventional colonoscopy.
Six studies assessed the ADR between CADe-assisted colonoscopy and standard colonoscopy, while six studies assessed the PDR between them (Table 3).19-25 All studies showed an improvement in the ADR and PDR with CADe-assisted colonoscopy in real time. The average ADR in CADe-assisted colonoscopy and standard colonoscopy was 33.65% and 22.85%, respectively, with a range of 16%–54.8% for CADe-assisted colonoscopy and 8%–40.4% for standard colonoscopy.
There was a high degree of variation among the studies. The heterogeneity across the studies was significant (p<0.05). The variation ranged from 95.6% to 98.7%.
The assessment of bias among the studies using the Jadad scale is shown in Appendix 2.19-25 Most of the RCTs scored 3 or more on the Jadad scale and were therefore considered to be of good quality; one study scored 2, suggesting poor quality.
This meta-analysis assessed the real-time performance of CADe systems in detecting lesions most commonly missed by endoscopists and provided additional data that these systems improve the ADR and PDR. The analysis demonstrated that CADe-assisted colonoscopy performed better than did standard colonoscopy in detecting diminutive and nonpedunculated polyps and adenomas. The CADe systems demonstrated a percentage increase in detection between 72% and 92%. The effect size was more pronounced in polyp detection than in adenoma detection.
The difference in the withdrawal times observed in most RCTs was not significant with the use of CADe systems. This suggests that the endoscopy workflow will not be substantially affected by the addition of a CADe system, nor will it take longer to detect “tricky” lesions during the procedure. However, three studies showed a significantly longer withdrawal time for CADe systems21-23; thus, it is difficult to gauge the realistic impact that CADe systems have on the withdrawal time given the variability in the type of systems used and relative infancy of such CADe systems in a real-time healthcare setting.
Increasing adenoma detection is known to reduce the risk of colorectal cancer. It is also recognized that missed lesions cause the majority of PCCRCs.9 Therefore, it is reasonable to assume that the significant increase in detection for most commonly missed adenomas would translate into reduced PCCRC rates.
An increase in polyp detection often includes benign lesions, such as hyperplastic polyps. AI-based technology aimed at the characterization of polyps during endoscopy is being optimized to improve the ADR. Once effective, this technology will allow a strategy of “resect and discard” or “diagnose and leave” for small polyps, which has thus far defied large-scale attempts at introduction into daily clinical practice.26,27
The studies included in this meta-analysis used CADe systems based on neural networks; however, the type of architecture and algorithm in each study differed, which likely contributed to the large heterogeneity among the studies. Although our findings may highlight the overall impact of the use of CADe systems in detecting this subset of lesions, it may dilute and therefore underestimate the effect of individual systems. Endoscopic experience and the withdrawal time were similarly not standardized across the studies, which may have also contributed to the heterogeneity in this meta-analysis.
Previous meta-analyses have excluded the study by Gong et al.,22 as the system used in their study focused on withdrawal quality rather than direct lesion detection. Our meta-analysis focused on the general application of CADe systems and its impact on lesion detection; therefore, we included their study in our analysis. This likely contributed to the large heterogeneity observed. More real-time studies will allow focused analyses of different CADe systems and their applications.
The majority of the studies included were conducted in Chinese institutions; therefore, the findings may not be completely applicable to healthcare systems in the Western world. The level of experience of the endoscopists participating in the studies varied from 1–3 years22 to 4–6 years of experience,21 with different overall numbers of procedures. Previous studies have demonstrated a significant correlation between the number of years of endoscopy experience and the ADR.28 Endoscopists with a higher level of skill and performance are more likely to detect diminutive or nonpedunculated polyps than their counterpart. It would be important to assess whether the impact of the use of CADe systems correlates with the level of experience to allow their application in a cost-effective manner.
The malignant potential of diminutive adenomas remains unclear. Vleugels et al.29 reported a low risk of diminutive lesions containing advanced pathology (5.6%) and cancer (0.07%). Kim et al.30 showed an increased risk of metachronous cancer in the presence of three or more nonadvanced diminutive adenomas and advanced pathology. The number of diminutive lesions plays a role in some screening programs internationally. Optical diagnosis using an AI-based system may improve the overall detection of colonic pathology and may lead to a resection and discard pathology with respect to diminutive lesions.
In conclusion, the use of CADe systems plays a significant role in the improvement of colonoscopy procedures and early detection of colorectal cancer. This review demonstrated that CADe systems can improve the detection of commonly missed adenomas, with the potential to further improve the efficacy of colonoscopy and reduce the incidence of PCCRC. However, the evidence for real-time CADe-assisted endoscopy is still relatively limited; further trials, including comparison of different systems and different levels of experience, are required before this method is incorporated more widely into standard clinical practice.
REFERENCES
1. Arnold M, Sierra MS, Laversanne M, et al. Global patterns and trends in colorectal cancer incidence and mortality. Gut. 2017; 66:683–691.
2. Centelles JJ. General aspects of colorectal cancer. ISRN Oncol. 2012; 2012:1–19.
3. Leslie A, Carey FA, Pratt NR, et al. The colorectal adenoma-carcinoma sequence. Br J Surg. 2002; 89:845–860.
4. Brenner H, Chang-Claude J, Jansen L, et al. Reduced risk of colorectal cancer up to 10 years after screening, surveillance, or diagnostic colonoscopy. Gastroenterology. 2014; 146:709–717.
5. Kahi CJ, Myers LJ, Slaven JE, et al. Lower endoscopy reduces colorectal cancer incidence in older individuals. Gastroenterology. 2014; 146:718–725.e3.
6. Zhao S, Wang S, Pan P, et al. Magnitude, risk factors, and factors associated with adenoma miss rate of tandem colonoscopy: a systematic review and meta-analysis. Gastroenterology. 2019; 156:1661–1674.e11.
7. Corley DA, Jensen CD, Marks AR, et al. Adenoma detection rate and risk of colorectal cancer. N Engl J Med. 2014; 370:1298–1306.
8. Rutter MD, Beintaris I, Valori R, et al. World Endoscopy Organization consensus statements on post-colonoscopy and post-imaging colorectal cancer. Gastroenterology. 2018; 155:909–925.e3.
9. Anderson R, Burr NE, Valori R. Causes of post-colonoscopy colorectal cancers based on World Endoscopy Organization system of analysis. Gastroenterology. 2020; 158:1287–1299.e2.
10. Sivananthan A, Glover B, Patel K, et al. The evolution of lower gastrointestinal endoscopy; where are we now. Ther Adv Gastrointest Endosc. 2020; 13:2631774520979591.
11. Harlow C, Sivananthan A, Ayaru L, et al. Endoscopic submucosal dissection: an update on tools and accessories. Ther Adv Gastrointest Endosc. 2020; 13:2631774520957220.
12. Hassan C, Spadaccini M, Iannone A, et al. Performance of artificial intelligence in colonoscopy for adenoma and polyp detection: a systematic review and meta-analysis. Gastrointest Endosc. 2021; 93:77–85.e6.
13. Barua I, Vinsard DG, Jodal HC, et al. Artificial intelligence for polyp detection during colonoscopy: a systematic review and meta-analysis. Endoscopy. 2021; 53:277–284.
14. Nazarian S, Glover B, Ashrafian H, et al. Diagnostic accuracy of artificial intelligence and computer-aided diagnosis for the detection and characterization of colorectal polyps: systematic review and meta-analysis. J Med Internet Res. 2021; 23:e27370.
15. Moher D, Liberati A, Tetzlaff J, et al. Preferred reporting items for systematic reviews and meta-analyses: the PRISMA statement. PLoS Med. 2009; 6:e1000097.
16. Ng S, Sreenivasan AK, Pecoriello J, et al. Polyp detection rate correlates strongly with adenoma detection rate in trainee endoscopists. Dig Dis Sci. 2020; 65:2229–2233.
17. Delavari A, Salimzadeh H, Bishehsari F, et al. Mean polyp per patient is an accurate and readily obtainable surrogate for adenoma detection rate: results from an opportunistic screening colonoscopy program. Middle East J Dig Dis. 2015; 7:214–219.
18. Jadad AR, Moore RA, Carroll D, et al. Assessing the quality of reports of randomized clinical trials: is blinding necessary? Control Clin Trials. 1996; 17:1–12.
19. Wang P, Berzin TM, Glissen Brown JR, et al. Real-time automatic detection system increases colonoscopic polyp and adenoma detection rates: a prospective randomised controlled study. Gut. 2019; 68:1813–1819.
20. Luo Y, Zhang Y, Liu M, et al. Artificial intelligence-assisted colonoscopy for detection of colon polyps: a prospective, randomized cohort study. J Gastrointest Surg. 2021; 25:2011–2018.
21. Su JR, Li Z, Shao XJ, et al. Impact of a real-time automatic quality control system on colorectal polyp and adenoma detection: a prospective randomized controlled study (with videos). Gastrointest Endosc. 2020; 91:415–424.e4.
22. Gong D, Wu L, Zhang J, et al. Detection of colorectal adenomas with a real-time computer-aided system (ENDOANGEL): a randomised controlled study. Lancet Gastroenterol Hepatol. 2020; 5:352–361.
23. Liu WN, Zhang YY, Bian XQ, et al. Study on detection rate of polyps and adenomas in artificial-intelligence-aided colonoscopy. Saudi J Gastroenterol. 2020; 26:13–19.
24. Repici A, Badalamenti M, Maselli R, et al. Efficacy of real-time computer-aided detection of colorectal neoplasia in a randomized trial. Gastroenterology. 2020; 159:512–520.e7.
25. Wang P, Liu X, Berzin TM, et al. Effect of a deep-learning computer-aided detection system on adenoma detection during colonoscopy (CADe-DB trial): a double-blind randomised study. Lancet Gastroenterol Hepatol. 2020; 5:343–351.
26. Zachariah R, Samarasena J, Luba D, et al. Prediction of polyp pathology using convolutional neural networks achieves “resect and discard” thresholds. Am J Gastroenterol. 2020; 115:138–144.
27. Rodriguez-Diaz E, Baffy G, Singh SK. Development of computer-assisted real time histology of colorectal polyps based on the nice criteria using nearfocus narrow-band imaging. Gastroenterology. 2017; 152(5 Suppl 1):S81.
28. James P, Hegagi M, Hegagi M, et al. Variable endoscopist performance in proximal and distal adenoma detection during colonoscopy: a retrospective cohort study. BMC Gastroenterol. 2018; 18:73.
29. Vleugels JL, Hassan C, Senore C, et al. Diminutive polyps with advanced histologic features do not increase risk for metachronous advanced colon neoplasia. Gastroenterology. 2019; 156:623–634.e3.
30. Kim JY, Kim TJ, Baek SY, et al. Risk of metachronous advanced neoplasia in patients with multiple diminutive adenomas. Am J Gastroenterol. 2018; 113:1855–1861.
Fig. 1.
Preferred Reporting Items for Systematic Reviews and Meta-Analyses (PRISMA) flow diagram of study selection.
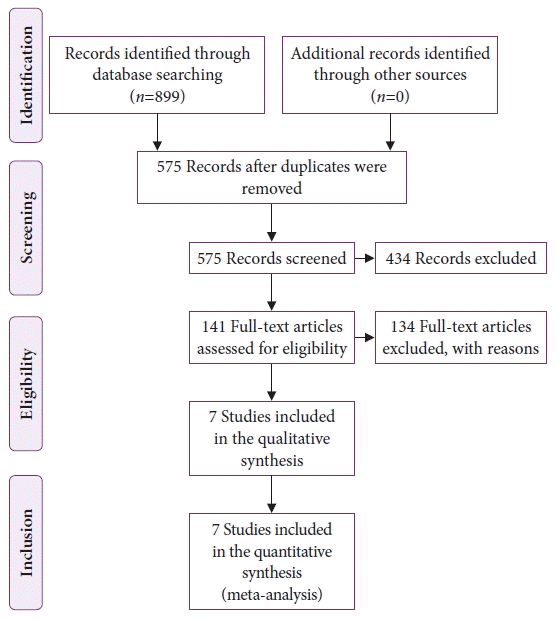
Fig. 2.
Pooled analysis of the mean number of diminutive adenomas per patient between the computer-aided detection and conventional colonoscopy groups. Effect sizes (ES) are shown with 95% confidence intervals (CI). A random-effects model was used.
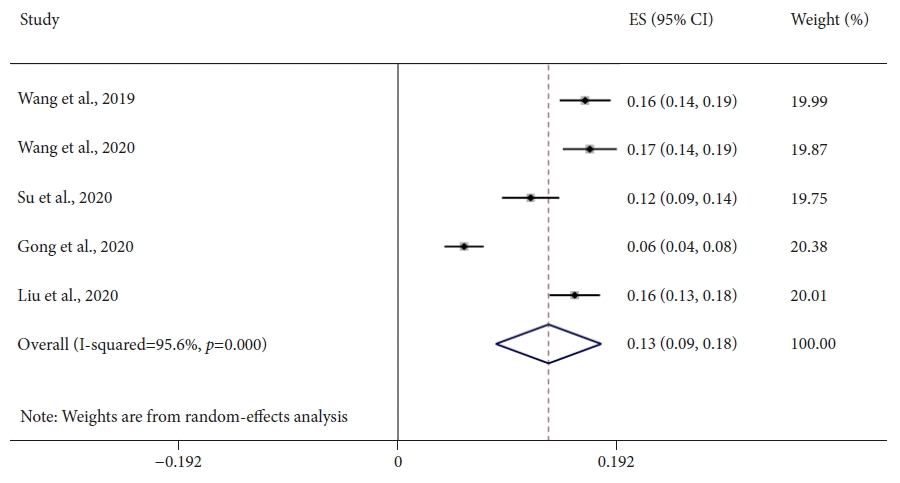
Fig. 3.
Pooled analysis of the mean number of nonpedunculated adenomas per patient between the computer-aided detection and conventional colonoscopy groups. Effect sizes (ES) are shown with 95% confidence intervals (CI). A random-effects model was used.

Table 1.
Characteristics of the included studies
Study | Machine learning approach |
No. of patients |
Reported polyps (total) | Reported adenomas (total) | Withdrawal timea) CADe vs. control (min) | ||
---|---|---|---|---|---|---|---|
Total | CADe | Control | |||||
Wang et al.,19 2019 | ANN: SegNet architecture | 1,058 | 522 | 536 | ✓ (767) | ✓ (424) | 6.18 vs. 6.07 (p=0.15) |
Wang et al.,25 2020 | ANN: SegNet architecture | 962 | 484 | 478 | ✓ (809) | ✓ (462) | 6.48 vs. 6.37 (p=0.14) |
Su et al.,21 2020 | DCNN | 623 | 308 | 315 | ✓ (273) | ✓ (169) | 7.03 vs. 5.68 (p<0.001) |
Gong et al.,22 2020 | DCNN | 704 | 355 | 349 | ✓ (284) | ✓ (85) | 6.38 vs. 4.76 (p<0.0001) |
Liu et al.,23 2020 | ANN | 1,026 | 508 | 518 | ✓ (734) | ✓ (394) | 6.82 vs. 6.74 (p<0.001) |
Luo et al.,20 2021 | CNN: YOLO | 150 | 77 | 82 | ✓ (185) | x | 6.22 vs. 6.17 (p=0.102) |
Repici et al.,24 2020 | CNN: GI Genius | 685 | 341 | 344 | x | ✓ (313) | 6.95 vs. 7.25 (p=0.1) |
Table 2.
Summary of the MPP and MAP, difference, and effect size between the CADe and control groups
Table 3.
Summary of polyp and adenoma detection between the CADe and control groups
Study | PDR–CADe (%) | PDR–control (%) | ADR–CADe (%) | ADR–control (%) |
---|---|---|---|---|
Wang et al.,19 2019 | 45.0 | 29.1 | 29.1 | 20.3 |
Wang et al.,25 2020 | 52 | 37 | 34 | 28 |
Su et al.,21 2020 | 38.3 | 25.4 | 28.9 | 16.5 |
Gong et al.,22 2020 | 47 | 34 | 16 | 8 |
Liu et al.,23 2020 | 43.7 | 27.8 | 39.1 | 23.9 |
Luo et al.,20 2021 | 38.7 | 34 | NK | NK |
Repici et al.,24 2020 | NK | NK | 54.8 | 40.4 |