Abstract
Objectives
Thyroid cancer is the most common endocrine tumor, with rapidly increasing incidence worldwide. However, its transcriptomic characteristics associated with immunological signatures, driver fusions, and recurrence markers remain unclear. We aimed to investigate the transcriptomic characteristics of advanced papillary thyroid cancer.
Methods
This study included 282 papillary thyroid cancer tumor samples and 155 normal samples from Chungnam National University Hospital and Seoul National University Hospital. Transcriptomic quantification was determined by high-throughput RNA sequencing. We investigated the associations of clinical parameters and molecular signatures using RNA sequencing. We validated predictive biomarkers using the Cancer Genome Atlas database.
Results
Through a comparison of differentially expressed genes, gene sets, and pathways in papillary thyroid cancer compared to normal tumor-adjacent tissue, we found increased immune signaling associated with cytokines or T cells and decreased thyroid hormone synthetic pathways. In addition, patients with recurrence presented increased CD8+ T-cell and Th1-cell signatures. Interestingly, we found differentially overexpressed genes related to immune-escape signaling such as CTLA4, IDO1, LAG3, and PDCD1 in advanced papillary thyroid cancer with a low thyroid differentiation score. Fusion analysis showed that the PI3K and mitogen-activated protein kinase (MAPK) signaling pathways were regulated differently according to the RET fusion partner genes (CCDC6 or NCOA4). Finally, we identified HOXD9 as a novel molecular biomarker that predicts the recurrence of thyroid cancer in addition to known risk factors (tumor size, lymph node metastasis, and extrathyroidal extension).
Conclusion
We identified a high association with immune-escape signaling in the immune-hot group with aggressive clinical characteristics among Korean thyroid cancer patients. Moreover, RET fusion differentially regulated PI3K and MAPK signaling depending on the partner gene of RET, and HOXD9 was found to be a recurrence marker for advanced papillary thyroid cancer.
The incidence of thyroid cancer is increasing worldwide [1,2]. Distinguishing between benign and malignant thyroid nodules is a very challenging problem; thus, studies have investigated various clinical and experimental methods to increase the accuracy [3]. Approximately 90% of thyroid cancers are differentiated thyroid carcinomas (DTCs), including the papillary and follicular types. Although most DTCs have good prognoses, they often recur and in some cases become metastatic and aggressive, which makes treatment difficult. In addition, the dedifferentiation of DTC is known to be a mechanism related to the development of anaplastic thyroid carcinoma, which exhibits rapid disease progression and has an extremely poor survival rate [4]. However, the major mechanism related to thyroid dedifferentiation in advanced papillary thyroid cancer (PTC) is still unknown.
Decades after RNA sequencing was developed, attempts to use RNA sequencing continue to evolve clinically. In the past, RNA sequencing was used only to investigate gene expression, but it is now being used to analyze oncogenic fusions, the tumor microenvironment (by calculating the relative abundance of immune cells), and oncogenic splicing patterns [5-7]. While largescale comprehensive multi-omics studies of thyroid cancer have yielded insights on the molecular landscape of thyroid cancer, and a meta-analysis identified surgical factors related to the recurrence of thyroid cancer [8], the molecular markers that predict recurrence in advanced PTC remain incompletely understood [9-11].
RET rearrangement is a widespread gene fusion event observed in PTC that is crucial in thyroid tumorigenesis [12], and selpercatinib is a Food and Drug Administration-approved drug for RET fusion-positive thyroid cancer [13]. RET fusion in thyroid cancer occurs more frequently in children than in adults, and it is known that RET fusion causes transformations in thyroid cells that lead to hyperplasia or neoplasia [14]. However, since approximately 30% of patients are unresponsive to treatment, there is a need for biomarkers to distinguish different subtypes of RET fusion cases. In particular, few studies have investigated the molecular mechanisms of RET fusion in Korean thyroid cancer patients. Additionally, common driver mutations (e.g., BRAF and KRAS), identified either through targeted sequencing or through whole exome/genome sequencing, are insufficient to predict recurrence in Korean patients, who constitute a population with high iodine intake [15-17]. Thus, it is necessary to identify a molecular biomarker that predicts recurrence specifically in Korean patients with advanced PTC. Our study aimed to identify novel therapeutic targets and prognostic biomarkers through transcriptomic analyses of advanced PTC in Korean patients.
This study was approved by the Institutional Research and Ethics Committee at Chungnam National University Hospital (CNUH-2020-11-004-001), and Institutional Research and Ethics Committee at Seoul National University Hospital (H-1508-147-700). Forty PTC patients were enrolled from Seoul National University Hospital, and the other patients were enrolled at Chungnam National University Hospital. Informed consent for the study was obtained from the patients.
Fresh frozen tissues from 282 PTC patients who underwent thyroidectomy were analyzed using a massively parallel sequencing method. Normal thyroid tissues (n=155) were obtained from patients who underwent thyroidectomy, with confirmed cases of differentiated thyroid cancer. The clinicopathological characteristics of patients according to histology are shown in Supplementary Table 1.
In addition, a 2,100 Agilent Bioanalyzer (Agilent Technologies, Waldbronn, Germany) was used to estimate the RNA integrity number score. After stranded total RNA-seq library construction, we used the TruSeq Stranded Total RNA Sample Preparation Kit (Illumina, San Diego, CA, USA) based on the manufacturer’s instructions. We sequenced the samples via the HiSeq 4000 sequencing system, yielding 2×100-bp sequencing reads. The raw data were deposited in the Korean Nucleotide Archive (KoNA, https://kobic.re.kr/kona) with the accession ID PRJKA210106.
Sequencing reads were trimmed by trim galore (https://www.bioinformatics.babraham.ac.uk/projects/trim_galore/) with a threshold of average sequence quality over 30 and mapped to the human reference genome GRCh38 by STAR aligner (STAR-2.7.9a) [18]. Ensembl gencode (ver. 27) gene annotation was used to profile gene expression from raw read counts, normalization, and quantification by cufflinks at the gene level [19].
We performed single-sample gene set enrichment analysis (ssG-SEA) for functional characterization by samples. For the reference gene matrix transposed (GMT) file, we downloaded GMT files in MSigDB [20]. Then, the gene set variation analysis (GSVA) function in the GSVA package was used with the default option [21]. We used Enrichr for functional classification called GSEA by differentially expressed genes (DEGs) with a threshold P-value below 0.01 from Fisher’s exact test [22].
Sequenced reads were mapped to the human reference genome GRCh38 for fusion detection using STAR fusion with default options [5]. The result of fusion analysis was filtered out through the following three processes: (1) genes were eliminated, such as immunoglobulin genes, uncharacterized genes, and RNA genes; (2) fusions including the same gene or paralog gene were removed; and (3) fusions detected in normal samples were omitted. We performed outlier analysis for fusion candidates. The outlier means that the sample’s level at a given gene was greater than the 75th percentile +1.5 interquartile range. This trend should not be discovered in the normal group.
We used the thyroid differentiation score (TDS) score from the Thyroid Carcinoma cohort from the Cancer Genome Atlas (TCGA-THCA) study [9]. TDS scores is from ssGSEA performed with 16 genes (DIO1, DIO2, DUOX1, DUOX2, FOXE1, GLIS3, NKX2-1, PAX8, SLC26A4, SLA5A5, SLC5A8, TG, THRA, THRB, TPO, TSHR) associated with thyroid function and metabolism from the TCGA study. As a result of calculating the correlation of the TDS scores, correlation values were highly significant (R=0.859, P<2.2e-115).
We carried out immune-subtyping from xCell (https://xcell.ucsf.edu/) [6]. The abundances of 64 various cell types for PTC samples were computed using fragment per kilobase of transcript per million (FPKM). We divided the patients into three major clusters using unsupervised K-means clustering using pheatmap package from R 4.1.2 (https://cran.r-project.org/). These groups have a significant difference of ImmuneScore from xCell. Thus, we named them immune-hot, -intermediate, and -cold. ImmuneScore is a sum of 10 immune cell types such as B-cells, CD4+ T-cells, CD8+ T-cells, dendritic cells, eosinophils, macrophages, monocytes, mast cells, neutrophils, and NK cells. It is an experimental score and known to better optimize tumor microenvironment. In three subtypes, we compared various immune abundances and RNA expression (Fisher’s exact test, P<0.01). To discover sets of coexpressed and hub genes in the network, we utilized CEMiTool [23]. This tool integrates the identification and analysis of coexpression gene modules, evaluating which modules contain genes that are overrepresented by specific geneset terms with P-values below 0.01 from Fisher’s exact test.
We collected 282 PTC samples (Supplementary Table 1). The clinicopathological characteristics included capsular invasion, extrathyroidal extension (ETE), central and lateral lymph node metastasis (C_LNM and L_LNM), lymphovascular invasion (LVI), recurrence, age, and sex. The average age of the PTC patients was 49 years. The mean tumor size of the PTC samples was 1.83 cm.
We quantified FPKM values for 15,690 genes through a standard RNA-sequencing analysis pipeline. First, we identified DEGs in tumor samples compared to normal samples, with a fold-change >1.5 and P<0.01, and we selected a total of 2,307 genes as DEGs (Fig. 1A). There were 1,039 downregulated genes and 1,268 upregulated genes. The top five highly upregulated genes in PTC were ZCCHC12, FN1, CHI3L1, SLC34A2, and DCSTAMP.
We performed gene set analyses with DEGs using the Pathways-KEGG 2021 Human and MSigDB Hallmark gene sets in Enrichr (Fig. 1B). The upregulated gene set terms in PTC versus normal samples included cytokine receptor interactions, p53 signaling, and complement and coagulation in KEGG, and the epithelial-mesenchymal transition (EMT), the inflammatory response, and allograft rejection in Hallmark. The down-regulated gene set terms included thyroid hormone synthesis and amino acid metabolism in KEGG and the estrogen response, ultraviolet response, and hypoxia in Hallmark. Interestingly, many immuneassociated gene-sets were significantly enriched, such as the EMT (including ECM1, SPARC, and LOXL1) and the inflammatory response (including ICAM4, MMP14, and TLR2).
We also found that immune-related signaling was upregulated in PTC. When patients with and without LNM were compared, immune-related signaling (e.g., nuclear factor kappa-light-chain-enhancer of activated B cells [NF-κB], tumor necrosis factor-alpha [TNF-α], the epithelial cell score, Wnt signaling, CD4+ T-cells, and memory B-cells) was exclusively higher in patients with LNM. In addition, patients with recurrence had higher scores for CD8+ T-cells and Th1 cells than patients without recurrence (Fig. 1C).
We delineated pathway maps showing the expression of all genes with KEGG terms (Supplementary Fig. 1). In PTC tumors, we significant upregulation of many components of the cytokine receptor interaction pathway, including CCR1-2, CCR4-5, and CCR7 in the CC-subfamily and TGFBR1, BMPR2, and ACVR1 in the transforming growth factor-beta family.
Attempts to apply immunotherapy to thyroid cancer are continuing, and in addition to the discovery of many immune checkpoint inhibitors (ICIs) such as PD-L1, CTLA4, IDO1, and HAVCR2, various immune signaling pathways, such as TNF-α signaling and the IL-6/JAT/STAT3 pathway have been discovered [24,25]. To investigate the role of immune-escape signaling in advanced PTC, we investigated the expression of genes related to immunotherapy and immune subtyping using ImmuneScore. We performed an immune signature analysis using xCell from RNA sequencing data in PTC (Methods). We divided PTC into three groups using K-means clustering with 64 immune-related scores. Based on the immune scores, we classified patients into immune-hot (n=35), immune-intermediate (n=107) and immune-cold (n=140) groups. We identified significantly different immune-related scores and expression of the top 50 genes across the three immune groups (Fig. 2A). In the immune-hot group, B-cells, CD4+ or CD8+ T-cells, dendritic cells, and ImmuneScore values were higher. In the immune-intermediate group, astrocytes, fibroblasts, hepatocytes, and preadipocytes were enriched. In the immune-cold group, the TDS, common lymphoid progenitors, myocytes, osteoblasts were elevated (Fig. 2A, top panel). Analyses of the associations between clinical data and the immune-subtype showed significant differences in ETE status, C_LNM, and L_LNM (Fig. 2A, middle panel, Supplementary Table 2). Interestingly, the immune-hot group revealed poor prognostic indicators (tumor size, ETE, c_LNM, and L_LNM). We selected significantly different genes from each group and performed gene-set enrichment analysis. In the immune-hot group, genes related to interferon-gamma, such as ISG20, CASP8, and PTPN6, showed significant differences (P<0.001). In the immune-intermediate group, genes related to the EMT (e.g., LAMA2, MMP2, VEGFA, and BMP1; P<0.001) and in the immune-cold group, genes related to Myc (e.g., XRCC6, XPOT, NPM1, and CDK4; P<0.001) were significantly enriched (Fig. 2A, bottom panel). An analysis of the distribution of the TDS across the three immune groups showed that the TDS of the immune-cold group was significantly higher and the TDS of the immune-hot group was significantly lower than those of the other groups (Fig. 2B). In addition, we extracted the distribution of the TDS across immune-subtypes and genes that showed high correlations with the TDS (Fig. 2C). As the TDS decreased, the proportion of immune-hot tumors increased and the proportion of immune-cold tumors decreased. Conversely, as the TDS score increased, the proportion of immune-hot tumors decreased and the proportion of immune-cold tumors increased. Moreover, genes such as B3GNT9 (r=0.80), TSHR (r=0.77), MMP15 (r=0.77), LIPG (r=0.76), and CD24 (r=0.76) showed very high correlations with the TDS.
In the immune-hot group with a low TDS score, the expression of genes related to immune-escape signaling (e.g., CTLA4, IDO1, LAG3, and PDCD1) was significantly higher than in the other groups (Fig. 2D). Finally, we performed module and co-expression analyses across the three immune groups (Fig. 2E). In the immune-hot group, genes such as ITGA4, UBC, NFKB1, and PRKCA belonging to the interferon-gamma module acted as hub genes with many interactions, in the immune-intermediate group, MMP2 was a hub gene, and in the immune-cold group, XPOT, TCEA1, and IARS were identified as hub genes. These data suggest that immune subtypes, including immune-hot, -intermediate, and -cold groups, were significantly associated with TDS, and prognostic clinical parameters were associated with the immune-hot signature.
We performed fusion analysis from RNA-sequencing data with STAR fusion (Methods), identified 3,160 fusions, filtered out low-significance fusions, and finally obtained 1,129 fusions. All significant fusions were found in PTC samples. Each fusion count is shown in order of frequency (Fig. 3A). FBXO25 fusion was found in 22 samples, followed by RET, CA13, NTRK1, and MET fusions. We performed fusion outlier analysis with three fusion genes (FBXO25, RET, and CA13) to determine driver fusions (Methods). No significant upregulation was observed for CA13 and FBXO25 fusion samples (Supplementary Fig. 2A). The expression of RET in all fusion-positive tumor samples was higher than that in fusion-negative samples, and CCDC6, NCOA4, and DLG5 were partner genes of RET fusion (Fig. 3B). We investigated how the mitogen-activated protein kinase (MAPK) and phosphatidylinositol 3-kinase (PI3K) pathways, which are known to be downstream signaling pathways impacted by RET fusion, are differentially regulated [26-28] by different fusion partners of RET. DLG5 was excluded from further analysis, as it was found in only one sample. We divided samples into RET-CCDC6, RET-NCOA4, and RET-WT and selected DEGs (Fisher exact test, P<0.05). In the RET-CCDC6 and RET-NCOA4 fusions, 213 and 1,260 genes were significant, respectively, and among them, genes belonging to the MAPK and PI3K signaling pathways were selected and described (Fig. 3C). In the RET-CCDC6 fusion group, CREB1 and PIK3R1 were upregulated, and PPP2R2D and MAP2K7 were downregulated. In the RET-NCOA4 group, more genes belonging to the PI3K and MAPK signaling pathways were differentially expressed than in the RET-CCDC6 fusion group. When the association with clinical factors according to the two partner genes was analyzed, ETE and L_LNM were significantly more frequent in RET-NCOA4 patients than in the RET-CCDC6 group (Supplementary Fig. 2B). We then selected marker genes that can distinguish RET-CCDC6 and RET-NCOA4, and discovered FGF13 in RET-CCDC6 fusion and AKT1 and CCND2 in RET-NCOA4 fusion (Supplementary Fig. 3, Fig. 3D). The three genes were validated in the TCGA-THCA cohort (Fig. 3E). Thus, we found that RET fusion differentially regulated the PI3K and MAPK signaling pathways according to the partner genes of RET (Fig. 3F) [29].
One of the unmet medical needs in treating thyroid cancer is the identification of biomarkers that predict recurrence or distant metastasis [30]. Clinical factors such as age, sex, tumor size, extracapsular invasion, ETE, C_LNM and L_LNM, and laterallymphovascular invasion are currently used to predict recurrence or distant metastasis [31,32]. Using the Fisher exact test for each clinical factor, we found that recurrence occurred more frequently in patients with large tumors, ETE, and L_LNM (Fig. 4A). For these three significant risk factors, we divided samples into recurrence and non-recurrence groups. Most recurrence samples had all three risk factors (n=24, 59%), and most nonrecurrence samples did not have any of the three risk factors (n=88, 36%) (Fig. 4B). However, interestingly, we found three no-risk-factor samples in the recurrence group and 42 all-risk-factor samples in the non-recurrence group. We named these two groups No_RF and All_RF to distinguish them from other patterns of recurrence. We then performed statistical tests to identify genes that could predict recurrence in the No_RF group and identified the homeobox d9 (HOXD9) gene, which was found to have a significant relationship with disease-free survival in the TCGA database using GEPIA (http://gepia.cancer-pku.cn/) (Fig. 4C and D).
To understand the relationship between HOXD9 expression and recurrence, we divided the patients into HOXD9-high and HOXD9-low groups by the median value of HOXD9 expression, compared the ratio of recurrence, and confirmed that the HOXD9-high group had significantly more frequent recurrence than the HOXD9-low group (P<0.001) (Fig. 4D). Furthermore, the HOXD9-high group had larger tumors and a higher frequency of ETE than the HOXD9-low group (Supplementary Fig. 4A and B). We found that HOXD9 was significantly related to recurrence, in addition to other factors known to cause recurrence (Fig. 4E). Additionally, gene-set enrichment analysis revealed that the NF-κB signaling pathway was the most significant gene set among the selected genes at a threshold of P<0.05 from the Fisher exact test and a fold change >1.5 (Fig. 4F and Supplementary Fig. 4C).
We then analyzed transcription factors regulating the expression of HOXD9 using a transcription factor database (Methods) and validated the genes in the TCGA-THCA cohort (P<0.001) (Supplementary Fig. 4D). Transcription factor 3 (TCF3) and enhancer of zeste 2 polycomb repressive complex 2 subunit (EZH2) are known to bind to the promoter region of HOXD9, and the correlation with HOXD9 was also significant (Fig. 4G). Thus, we suggest that TCF3 and EZH2 may promote overexpression of HOXD9 associated with recurrence. Therefore, we suggest that HOXD9 may be a novel prognostic marker of recurrent PTC that acts through the NF-κB signaling pathway (Fig. 4H).
In this study, we report the transcriptomic characterization of 282 Korean PTC samples. RNA sequencing was performed on samples from two centers, and we discovered a few novel transcriptomic characteristics. We also made efforts to increase the accuracy by validating the findings using TCGA-THCA data.
Our data revealed that ZCHHC12, an oncogene in thyroid cancer, is significantly overexpressed in PTC tumors. Previous research identified ZCCHC12 as a novel oncogene in PTC and found that it was particularly associated with lymph node metastasis [33]. We also investigated the expression patterns of genes belonging to a particular gene set by analyzing genes, gene sets, and pathways. We found that THRA and PAX8 were repressed, as has previously been reported in PTC patients [11]. We subtyped 282 samples into immune-hot, immune-intermediate, and immune-cold groups using xCell. Based on this, we found immune subtype-specific genes in each subtype, such as PTPN22, PODN, and CASC4. In particular, PTPN22, a physiological regulator of the T cell receptor known as a target for immunotherapy, is upregulated in many human cancers, and we discovered an association between PTPN22 and the immune score in the immune-hot group [34,35]. PODN, which encodes a small proteoglycan family protein that is a type of ECM protein, was upregulated in gastric tumors, was associated with cell proliferation, and was one of six genes that predicted the prognosis of gastric cancer [36]. CASC4, for which anomalous splicing events were identified, was upregulated in human breast cancer and ovarian cancer. Its overexpression is associated with a poor prognosis in breast cancer patients with accumulating aberrantly spliced forms [37].
The overexpression of the well-known immune inhibitor genes in the immune-hot subtype was also confirmed in our dataset and the TCGA-THCA dataset. The overexpression of both CTLA4 and PD1 suggests that combination therapy is expected to be effective for the treatment of aggressive immune-hot thyroid cancer [38]. In addition, LAG3 is expressed in exhausted T cells and is known to be an ICI [39]. Furthermore, since IDO1 was associated with immune escape and inflammatory neovascularization in a previous report, we speculated that IDO1 might be a key player in the immune-escape mechanism in thyroid cancer cells [40]. It is also generally known that thyroid cancer is not immunogenic, but thyroid cancer has immunogenic properties during dedifferentiation [24]. Based on this, we found that IDO1 may be a gene that induces immune escape, yielding an improved understanding of thyroid cancer and immunogenicity. In addition, therapeutic targets in immune-intermediate and immune-cold groups were identified through a module analysis. XPOT, IARS, TRIB3, and MMP2 were candidate molecular targets. The function of IARS in cancer remains unclear, and XPOT, a member of the RAN GTPase exportin family for exporting tRNA, has been found to be associated with tumor development [41]. The elevated expression of MMP2 is involved in angiogenesis and development in cancer cells [42].
We also found RET-CCDC6 and RET-NCOA4 to be driver fusions in thyroid cancer. In particular, we revealed that these two fusions regulate the MAPK and PI3K signaling pathways differently depending on the partner gene. We discovered FGF13 as a marker gene in RET-CCDC6 and AKT1 and CCND2 as marker genes in RET-NCOA4. FGF13, AKT1, and CCND2 promote the survival of cancer cells, are involved in angiogenesis as cancer hallmark genes, or are associated with tumorigenesis [43-45]. We first found that the various RET fusions have different oncogenic impacts depending on the partner genes in thyroid cancer. This finding will be important in developing therapeutic targets for tumors with RET fusions.
Finally, HOXD9 was identified as a novel prognostic biomarker of recurrence in PTC samples. HOXD9, a well-known oncogene, is upregulated in various cancers, including gastric cancer, hepatocellular carcinoma, and thyroid cancer [46,47]. Our data also revealed that the NF-κB signaling pathway was the most significant gene set among the pathways enriched in the HOXD9-high group. NF-κB signaling is known to cause oncogenesis and disease recurrence in malignant cells, as well as therapy resistance [48]. NF-κB signaling induces the MAPK signaling pathway downstream of RET fusion [49]. In addition, we showed that HOXD9 regulation by TCF3 and EZH2 impacted immune signaling pathways. Overexpression of HOXD9 shortened disease-free survival in the TCGA-THCA data.
There are inherent limitations to this study. The experiment was performed only with RNA sequencing, and samples from various races and centers could not be collected. However, we tried to overcome this limitation by analyzing the TCGA-THCA data. Further functional studies are necessary to validate our findings. In conclusion, our study showed a high association with immune-escape signaling in the immune-hot group of thyroid cancer patients with aggressive clinical factors. Moreover, RET fusion differentially regulated PI3K and MAPK signaling depending on the partner gene of RET, and HOXD9 was found to be a recurrence marker in advanced PTC patients.
▪ Presentation of genes associated with tumorigenesis in papillary thyroid cancer (PTC) and anaplastic thyroid cancer patients.
▪ Patients were divided into three immune groups such as immune-hot, immune-intermediate, immune-cold.
▪ Suggestion of immunotherapy for immune-hot group with immune-escape signaling and low thyroid differentiation score.
▪ RET fusion differentially regulated PI3K and MAPK signaling depending on the partner genes such as CCDC6 and NCOA4.
▪ HOXD9 was found to be a recurrence marker for advanced PTC patients.
Notes
AUTHOR CONTRIBUTIONS
Conceptualization: SYK, BSK, SJP, YEK. Data curation: JHK, JLP, SKK, SY, SEL, YJP, EJC, JMK, HMK, JRK, SNJ, HRW, JWC. Formal analysis: SJP, YEK, SWB, ISC. Funding acquisition: SYK, BSK. Methodology: SYK, BSK, SJP, YEK. Project administration: SYK, BSK. Visualization: SJP, YEK. Writing–original draft: All authors. Writing–review & editing: SYK, BSK, SJP, YEK.
ACKNOWLEDGMENTS
This work was supported by Systemic Industrial Infrastructure Projects through the Ministry of Trade, Industry, and Energy (P0009796, 2019 to SYK and YEK).
Supplementary Materials
Supplementary materials can be found via https://doi.org/10.21053/ceo.2021.02215.
Supplementary Table 1.
Clinical characteristics of Korean papillary thyroid cancer patients
Supplementary Table 2.
Associations between clinical characteristics and immune subtypes
Supplementary Fig. 1.
The most altered pathways of papillary thyroid cancer.
Supplementary Fig. 2.
(A) Expression patterns of non-driver fusion. Boxplots showing non-driver oncogenic fusions, such as CA13 and FBXO25. (B) The ratio of extrathyroidal extension (ETE) and lateral lymph node metastasis (L_LNM) according to the partner gene of RET fusion compared to normal samples. WT, wildtype.
Supplementary Fig. 3.
Differently regulated genes in PI3K and mitogen-activated protein kinase (MAPK) signaling according to the partner genes in the fusion analysis. We found RET-CCDC6-specific expression of FGF13 (A) and RET-NCOA4-specific expression of AKT1 and CCND2 (B). WT, wildtype.
Supplementary Fig. 4.
Characteristics of the HOXD9 high group. (A, B) Associations between HOXD9 and clinical factors, including tumor size and extrathyroidal extension (ETE). (C) Nuclear factor kappa-light-chain-enhancer of activated B cells (NF-κB) signaling from gene set enrichment analysis in the HOXD9-high group. (D) Transcription factors such as transcription factor 3 (TCF3) and enhancer of zeste 2 polycomb repressive complex 2 subunit (EZH2) regulate the expression of HOXD9 as binding promoter regions of HOXD9. KTC, Korean Thyroid Cancer; TCGA-THCA, the Thyroid Carcinoma cohort from the Cancer Genome Atlas.
REFERENCES
1. Ahn HS, Kim HJ, Welch HG. Korea’s thyroid-cancer “epidemic”: screening and overdiagnosis. N Engl J Med. 2014; Nov. 371(19):1765–7.


2. Morris LG, Sikora AG, Tosteson TD, Davies L. The increasing incidence of thyroid cancer: the influence of access to care. Thyroid. 2013; Jul. 23(7):885–91.


3. Yeon EK, Sohn YM, Seo M, Kim EJ, Eun YG, Park WS, et al. Diagnostic performance of a combination of shear wave elastography and B-mode ultrasonography in differentiating benign from malignant thyroid nodules. Clin Exp Otorhinolaryngol. 2020; May. 13(2):186–93.


4. Giani F, Vella V, Tumino D, Malandrino P, Frasca F. The possible role of cancer stem cells in the resistance to kinase inhibitors of advanced thyroid cancer. Cancers (Basel). 2020; Aug. 12(8):2249.


5. Haas BJ, Dobin A, Li B, Stransky N, Pochet N, Regev A. Accuracy assessment of fusion transcript detection via read-mapping and de novo fusion transcript assembly-based methods. Genome Biol. 2019; Oct. 20(1):213.


6. Aran D, Hu Z, Butte AJ. xCell: digitally portraying the tissue cellular heterogeneity landscape. Genome Biol. 2017; Nov. 18(1):220.


8. Ahn SH, Kim WS. The effect of prophylactic central neck dissection during hemithyroidectomy on locoregional recurrence in patients with papillary thyroid carcinoma: a meta-analysis. Clin Exp Otorhinolaryngol. 2020; May. 13(2):194–202.


9. Cancer Genome Atlas Research Network. Integrated genomic characterization of papillary thyroid carcinoma. Cell. 2014; Oct. 159(3):676–90.
10. Yoo SK, Lee S, Kim SJ, Jee HG, Kim BA, Cho H, et al. Comprehensive analysis of the transcriptional and mutational landscape of follicular and papillary thyroid cancers. PLoS Genet. 2016; Aug. 12(8):e1006239.


11. Yoo SK, Song YS, Lee EK, Hwang J, Kim HH, Jung G, et al. Integrative analysis of genomic and transcriptomic characteristics associated with progression of aggressive thyroid cancer. Nat Commun. 2019; Jun. 10(1):2764.


12. Salvatore D, Santoro M, Schlumberger M. The importance of the RET gene in thyroid cancer and therapeutic implications. Nat Rev Endocrinol. 2021; May. 17(5):296–306.


13. Wirth LJ, Sherman E, Robinson B, Solomon B, Kang H, Lorch J, et al. Efficacy of selpercatinib in RET-altered thyroid cancers. N Engl J Med. 2020; Aug. 383(9):825–35.


14. Santoro M, Carlomagno F. Central role of RET in thyroid cancer. Cold Spring Harb Perspect Biol. 2013; Dec. 5(12):a009233.


15. Kim HJ, Park HK, Byun DW, Suh K, Yoo MH, Min YK, et al. Iodine intake as a risk factor for BRAF mutations in papillary thyroid cancer patients from an iodine-replete area. Eur J Nutr. 2018; Mar. 57(2):809–15.


16. Kim WB, Jeon MJ, Kim WG, Kim TY, Shong YK. Unmet clinical needs in the treatment of patients with thyroid cancer. Endocrinol Metab (Seoul). 2020; Mar. 35(1):14–25.


17. Kim HJ. Correlation of BRAF mutation of papillary thyroid carcinoma and urine iodine status in Korean population. Seoul: Yonsei University Health System;2021.
18. Dobin A, Davis CA, Schlesinger F, Drenkow J, Zaleski C, Jha S, et al. STAR: ultrafast universal RNA-seq aligner. Bioinformatics. 2013; Jan. 29(1):15–21.


19. Trapnell C, Roberts A, Goff L, Pertea G, Kim D, Kelley DR, et al. Differential gene and transcript expression analysis of RNA-seq experiments with TopHat and Cufflinks. Nat Protoc. 2012; Mar. 7(3):562–78.


20. Liberzon A, Birger C, Thorvaldsdottir H, Ghandi M, Mesirov JP, Tamayo P. The Molecular Signatures Database (MSigDB) hallmark gene set collection. Cell Syst. 2015; Dec. 1(6):417–25.
21. Hanzelmann S, Castelo R, Guinney J. GSVA: gene set variation analysis for microarray and RNA-seq data. BMC Bioinformatics. 2013; Jan. 14:7.


22. Kuleshov MV, Jones MR, Rouillard AD, Fernandez NF, Duan Q, Wang Z, et al. Enrichr: a comprehensive gene set enrichment analysis web server 2016 update. Nucleic Acids Res. 2016; Jul. 44(W1):W90–7.


23. Russo PS, Ferreira GR, Cardozo LE, Burger MC, Arias-Carrasco R, Maruyama SR, et al. CEMiTool: a Bioconductor package for performing comprehensive modular co-expression analyses. BMC Bioinformatics. 2018; Feb. 19(1):56.


24. Wang X, Peng W, Li C, Qin R, Zhong Z, Sun C. Identification of an immune-related signature indicating the dedifferentiation of thyroid cells. Cancer Cell Int. 2021; Apr. 21(1):231.


25. Cunha LL, Domingues GA, Morari EC, Soares FA, Vassallo J, Ward LS. The immune landscape of the microenvironment of thyroid cancer is closely related to differentiation status. Cancer Cell Int. 2021; Jul. 21(1):387.


26. de Groot JW, Links TP, Plukker JT, Lips CJ, Hofstra RM. RET as a diagnostic and therapeutic target in sporadic and hereditary endocrine tumors. Endocr Rev. 2006; Aug. 27(5):535–60.
27. Li AY, McCusker MG, Russo A, Scilla KA, Gittens A, Arensmeyer K, et al. RET fusions in solid tumors. Cancer Treat Rev. 2019; Dec. 81:101911.


28. Paratala BS, Chung JH, Williams CB, Yilmazel B, Petrosky W, Williams K, et al. RET rearrangements are actionable alterations in breast cancer. Nat Commun. 2018; Nov. 9(1):4821.


29. Santoro M, Moccia M, Federico G, Carlomagno F. RET gene fusions in malignancies of the thyroid and other tissues. Genes (Basel). 2020; Apr. 11(4):424.


30. Park JL, Kim SK, Jeon S, Jung CK, Kim YS. MicroRNA profile for diagnostic and prognostic biomarkers in thyroid cancer. Cancers (Basel). 2021; Feb. 13(4):632.


31. Jo K, Lim DJ. Clinical implications of anti-thyroglobulin antibody measurement before surgery in thyroid cancer. Korean J Intern Med. 2018; Nov. 33(6):1050–7.


32. Randolph GW, Duh QY, Heller KS, LiVolsi VA, Mandel SJ, Steward DL, et al. The prognostic significance of nodal metastases from papillary thyroid carcinoma can be stratified based on the size and number of metastatic lymph nodes, as well as the presence of extranodal extension. Thyroid. 2012; Nov. 22(11):1144–52.


33. Wang O, Zheng Z, Wang Q, Jin Y, Jin W, Wang Y, et al. ZCCHC12, a novel oncogene in papillary thyroid cancer. J Cancer Res Clin Oncol. 2017; Sep. 143(9):1679–86.


34. Ho WJ, Croessmann S, Lin J, Phyo ZH, Charmsaz S, Danilova L, et al. Systemic inhibition of PTPN22 augments anticancer immunity. J Clin Invest. 2021; Jul. 131(17):e146950.


35. Cubas R, Khan Z, Gong Q, Moskalenko M, Xiong H, Ou Q, et al. Autoimmunity linked protein phosphatase PTPN22 as a target for cancer immunotherapy. J Immunother Cancer. 2020; Oct. 8(2):e001439.


36. Ross MD, Bruggeman LA, Hanss B, Sunamoto M, Marras D, Klotman ME, et al. Podocan, a novel small leucine-rich repeat protein expressed in the sclerotic glomerular lesion of experimental HIV-associated nephropathy. J Biol Chem. 2003; Aug. 278(35):33248–55.


37. Duval S, Abu-Thuraia A, Elkholi IE, Chen R, Seebun D, Mayne J, et al. Shedding of cancer susceptibility candidate 4 by the convertases PC7/furin unravels a novel secretory protein implicated in cancer progression. Cell Death Dis. 2020; Aug. 11(8):665.


38. Rotte A. Combination of CTLA-4 and PD-1 blockers for treatment of cancer. J Exp Clin Cancer Res. 2019; Jun. 38(1):255.


39. Nguyen LT, Ohashi PS. Clinical blockade of PD1 and LAG3: potential mechanisms of action. Nat Rev Immunol. 2015; Jan. 15(1):45–56.


40. Prendergast GC, Mondal A, Dey S, Laury-Kleintop LD, Muller AJ. Inflammatory reprogramming with IDO1 inhibitors: turning immunologically unresponsive ‘cold’ tumors ‘hot’. Trends Cancer. 2018; Jan. 4(1):38–58.


41. Lin J, Hou Y, Huang S, Wang Z, Sun C, Wang Z, et al. Exportin-T promotes tumor proliferation and invasion in hepatocellular carcinoma. Mol Carcinog. 2019; Feb. 58(2):293–304.


42. Hojilla CV, Mohammed FF, Khokha R. Matrix metalloproteinases and their tissue inhibitors direct cell fate during cancer development. Br J Cancer. 2003; Nov. 89(10):1817–21.


43. Bublik DR, Bursac S, Sheffer M, Orsolic I, Shalit T, Tarcic O, et al. Regulatory module involving FGF13, miR-504, and p53 regulates ribosomal biogenesis and supports cancer cell survival. Proc Natl Acad Sci U S A. 2017; Jan. 114(4):E496–505.


44. Somanath PR, Razorenova OV, Chen J, Byzova TV. Akt1 in endothelial cell and angiogenesis. Cell Cycle. 2006; Mar. 5(5):512–8.


45. Ding ZY, Li R, Zhang QJ, Wang Y, Jiang Y, Meng QY, et al. Prognostic role of cyclin D2/D3 in multiple human malignant neoplasms: a systematic review and meta-analysis. Cancer Med. 2019; Jun. 8(6):2717–29.


46. Lv X, Li L, Lv L, Qu X, Jin S, Li K, et al. HOXD9 promotes epithelial-mesenchymal transition and cancer metastasis by ZEB1 regulation in hepatocellular carcinoma. J Exp Clin Cancer Res. 2015; Oct. 34:133.


47. Zhu H, Dai W, Li J, Xiang L, Wu X, Tang W, et al. HOXD9 promotes the growth, invasion and metastasis of gastric cancer cells by transcriptional activation of RUFY3. J Exp Clin Cancer Res. 2019; Sep. 38(1):412.


48. Verzella D, Pescatore A, Capece D, Vecchiotti D, Ursini MV, Franzoso G, et al. Life, death, and autophagy in cancer: NF-κB turns up everywhere. Cell Death Dis. 2020; Mar. 11(3):210.


49. Dhawan P, Richmond A. A novel NF-kappa B-inducing kinase-MAPK signaling pathway up-regulates NF-kappa B activity in melanoma cells. J Biol Chem. 2002; Mar. 277(10):7920–8.
Fig. 1.
Transcriptomic overview and immuno-clinical associations in Korean papillary thyroid cancer patients. (A) Volcano plots showing differentially expressed genes across groups. Red and gray dots represent significance (Fisher exact test; P<0.01 and fold change >1.5) and nonsignificance, respectively. (B) Results of gene-set enrichment analysis using the KEGG and Hallmark database from Enrichr. Light red and light blue indicate upregulated and downregulated terms in tumors compared to normal tissues. (C) Increased immune-related signaling was exclusively observed when lymph node metastasis (LNM) and recurrence occurred. Nuclear factor kappa-light-chain-enhancer of activated B cells (NF-κB) and tumor necrosis factor-alpha (TNF-α) were only enriched when LNM occurred and CD8+ T cells and Th1 cells were only enriched when recurrence occurred. EMT, epithelial-mesenchymal transition; UV, ultraviolet.
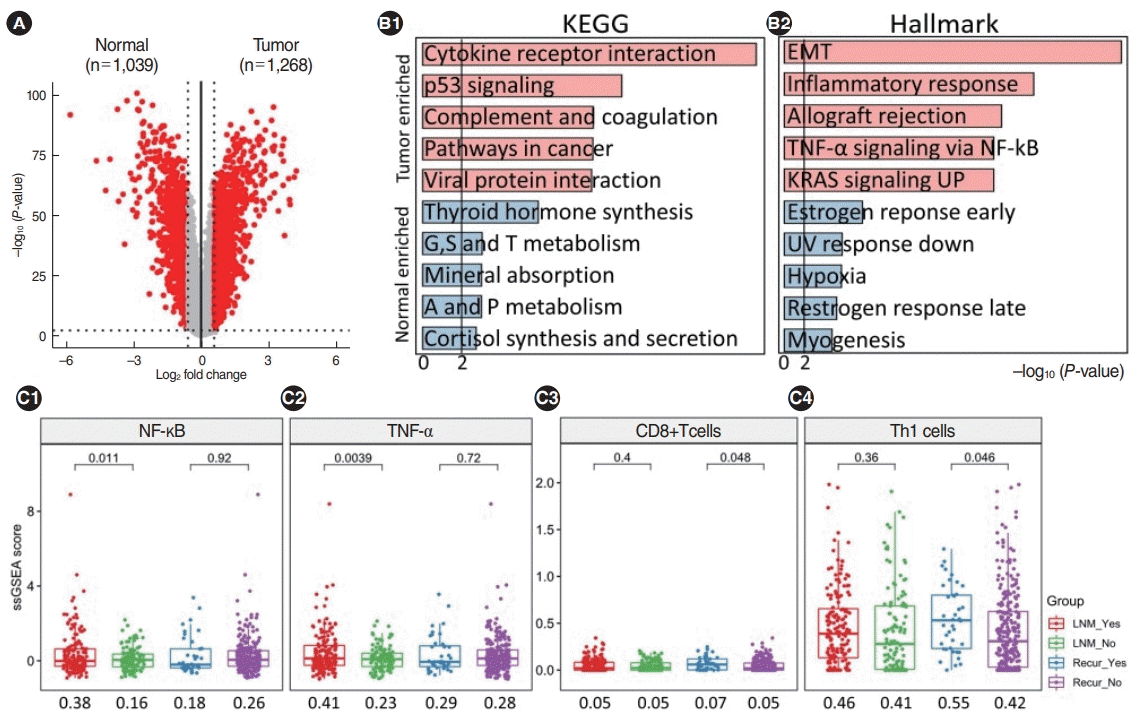
Fig. 2.
Association of immune signatures with the thyroid differentiation score (TDS) in advanced papillary thyroid cancer (PTC). (A) The immune landscape of PTC using the immune signature of xCell. The immune subtype based on the immune score was represented as immunehot (n=35), immune-intermediate (n=107), and immune-cold (n=140). (B) The TDS across three immune subtypes. (C) Heatmap sorted by TDS and expression of the top 20 genes that showed high correlations with the TDS. (D) Boxplots showing the major immune-checkpoint inhibitors, including CTLA4, IDO1, LAG3, and PDCD1. (E) Hub gene discovery through network module analysis for each immune subtype. ECI, extracapsular invasion; ETE, extrathyroidal extension; C_LNM, central lymph node metastasis; L_LNM, lateral lymph node metastasis; LVI, lymphovascular invasion; EMT, epithelial-mesenchymal transition.
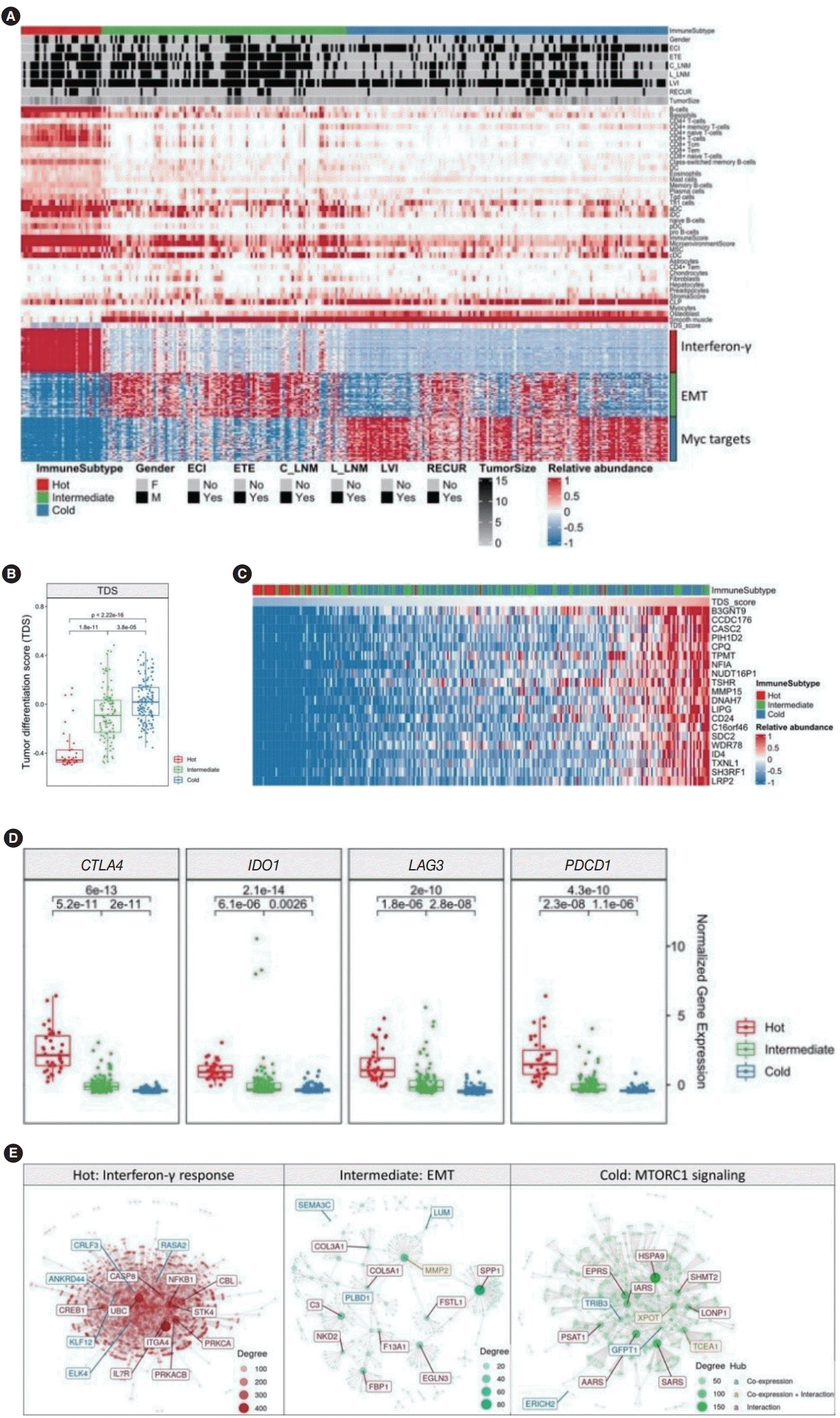
Fig. 3.
Different regulation patterns of the PI3K and mitogen-activated protein kinase (MAPK) signaling pathways according to the partner genes of RET fusion. (A) Bar plot showing the fusion count across papillary thyroid cancer (PTC) tumors. (B) Outlier analysis of RET fusion. Blue and red dots indicate the expression of the RET gene in samples without and with fusions, respectively. (C) Impacts of the two partner genes of RET fusion on the MAPK and PI3K pathways. “Common” indicates overlapping genes in both pathways. Circles and squares indicate cis- and trans-acting genes, but there is no cis-interaction, and their sizes indicate the significance of the P-value. (D, E) The genes regulated by RET fusion of CCDC6 and NCOA4 may bridge the MAPK signaling pathway. (D) PTC in the Korean Thyroid Cancer (KTC) cohort. (E) The Thyroid Carcinoma cohort from the Cancer Genome Atlas (TCGA-THCA). (F) Two ways that RET fusion regulates MAPK signaling with different partner genes. WT, wildtype.
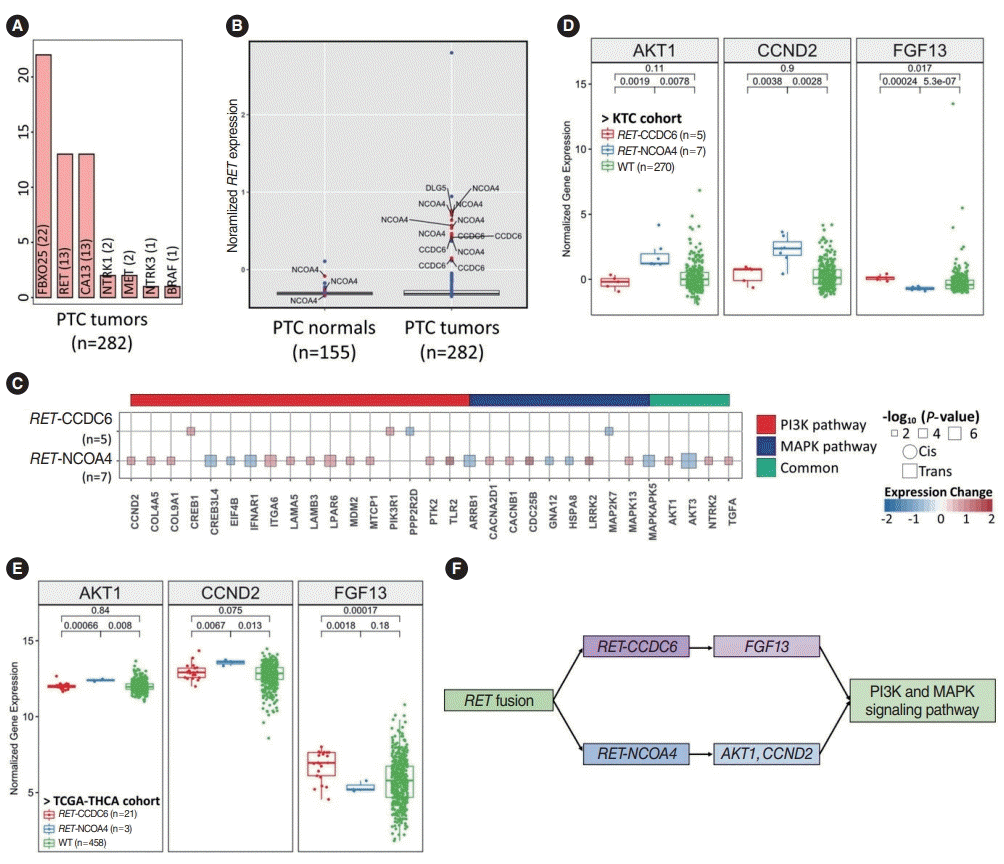
Fig. 4.
HOXD9 is a candidate gene associated with the recurrence of papillary thyroid cancer (PTC). (A) Discovery of recurrence-related factors in PTC samples. The Fisher exact test was performed with a threshold of P<0.01. (B) Barplots showing the spectrum of samples in the PTC group for well-known risk factors such as tumor size, extrathyroidal extension (ETE), and lateral lymph node metastasis (L_LNM). (C) Workflow for selecting HOXD9. (D) Kaplan-Meier plot of thyroid cancer patients based on the expression of HODX9 (high or low based on the median value from the GEPIA database. (E) Barplots showing the recurrence rate based on the expression of HOXD9. (F) Enriched signaling pathways in the HOXD9-high group. (G) Correlation of gene expression between HOXD9 and transcription factors such as transcription factor 3 (TCF3) and enhancer of zeste 2 polycomb repressive complex 2 subunit (EZH2) that regulate HOXD9 in the Korean Thyroid Cancer (KTC) cohort and the Thyroid Carcinoma cohort from the Cancer Genome Atlas (TCGA-THCA cohort). (H) Summary of the two routes to recurrence from Korean PTC samples. ECI, extracapsular invasion; C_LNM, central lymph node metastasis; L_LVI, lateral-lymphovascular invasion; NS, not significant; RF, risk factor; DFS, disease free survival; NF-κB, nuclear factor kappa-light-chain-enhancer of activated B cells; TNF-α, tumor necrosis factoralpha; TF, transcription factor.
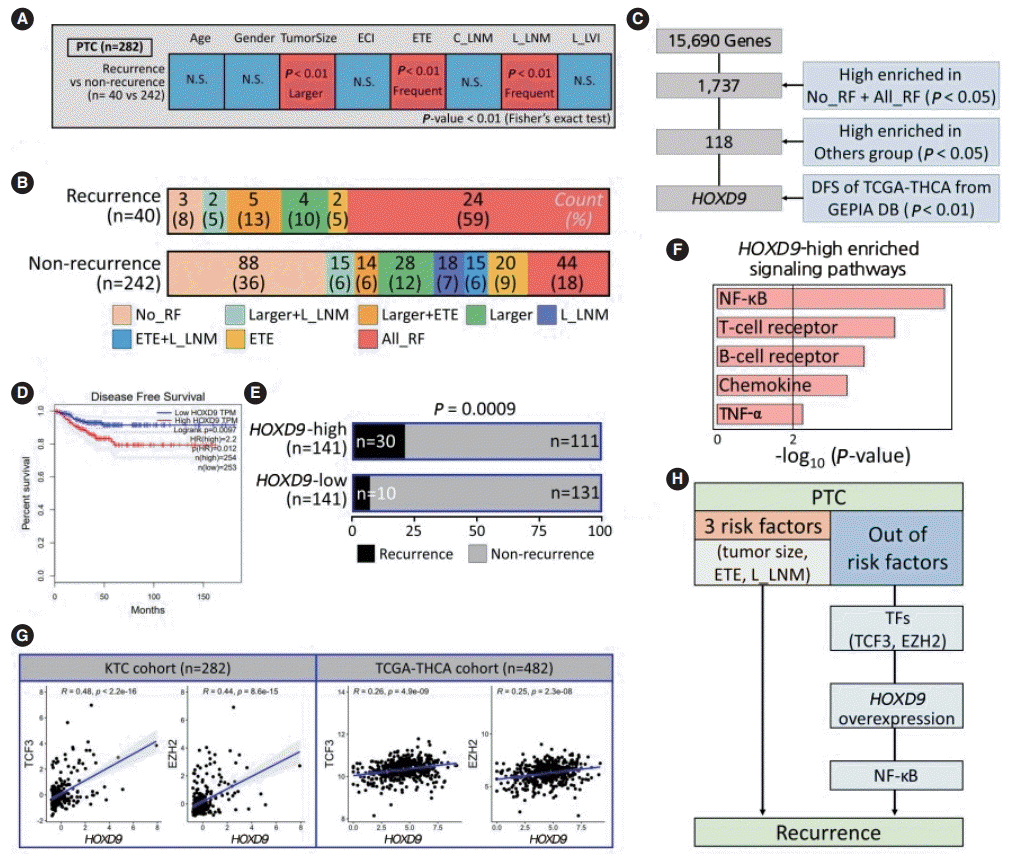