Abstract
Objectives
The outlook of artificial intelligence for healthcare (AI4H) is promising. However, no studies have yet discussed the issues from the perspective of stakeholders in Korea. This research aimed to identify stakeholders’ requirements for AI4H to accelerate the business and research of AI4H.
Methods
We identified research funding trends from the Korean National Science and Technology Knowledge Information Service (NTIS) from 2015 and 2019 using “healthcare AI” and related keywords. Furthermore, we conducted an online survey with members of the Korean Society of Artificial Intelligence in Medicine to identify experts’ opinions regarding the development of AI4H. Finally, expert interviews were conducted with 13 experts in three areas (hospitals, industry, and academia).
Results
We found 160 related projects from the NTIS. The major data type was radiology images (59.4%). Dermatology-related diseases received the most funding, followed by pulmonary diseases. Based on the survey responses, radiology images (23.9%) were the most demanding data type. Over half of the solutions were related to diagnosis (33.3%) or prognosis prediction (31%). In the expert interviews, all experts mentioned healthcare data for AI solutions as a major issue. Experts in the industrial field mainly mentioned regulations, practical efficacy evaluation, and data accessibility.
Conclusions
We identified technology, regulatory, and data issues for practical AI4H applications from the perspectives of stakeholders in hospitals, industry, and academia in Korea. We found issues and requirements, including regulations, data utilization, reimbursement, and human resource development, that should be addressed to promote further research in AI4H.
The outlook of artificial intelligence for healthcare (AI4H) is very promising [1]. The size of the AI4H market with software as a medical device (SaMD) is expected to reach about US $4.9 billion in 2020 and $45.2 billion in 2026, with an annual growth rate of 44.9%. In particular, growth from US $1.9 billion to $17.8 billion (an annual growth rate of 45.6%), $1.4 billion to $12.3 billion (44.5%), and $1.2 billion to $12.0 billion (46.3%) in the United States, Europe, and Asia-Pacific in this period [2,3]. AI4H is used for many healthcare applications, such as clinical decision support, virtual assistants, computer-aided diagnosis, and robots in hospitals [4]. As a result, the number of Food and Drug Administration (FDA)-approved AI-based algorithms in the United States has increased from 41 to 68, and in South Korea from 4 to 61, between January 2018 and December 2020 [5,6]. As described previously [7], there are 130 US FDA-approved medical AI devices.
Three fundamental factors have contributed to the recent achievements of AI4H: (1) advances in AI algorithms, (2) improvements in computing power such as general-purpose computing on graphics processing units, and (3) generation of healthcare big data such as Electronic Medical Records, genomic sequencing data, and computed tomography and magnetic resonance imaging (CT/MRI) scans. These factors converge to enable research on AI-based prognosis prediction, disease diagnosis, genomics, and radiomics. Some researchers have reported that AI outperformed physicians in disease classification, demonstrating its success in detecting diseases on medical images [7–9].
To address the success and trends of AI4H, several white papers and reports have been published, such as the AI index report from Stanford University [10] and the AI in healthcare report from the National Academy of Medicine [11]. Recently, a survey article identifying the expected impact and value of AI4H was published, based on an online survey with 151 experts from 15 countries in Europe. They divided challenges into technical and non-technical, for example data anonymization, sharing and regulatory issues, and standardization [12]. However, most of those reports have focused on showing current issues without proposing a practical discussion for real-world use. There have been a few previous studies discussing the practicality and challenges of AI4H in Korea [13,14], but no study has yet discussed the issues of AI4H from challenge identification to solutions in terms of stakeholders’ opinions, which will be very critical for practical applications.
The aim of this study is identifying stakeholders’ requirements for AI4H in Korea by reviewing governmental research and development funding and conducting an online survey and in-depth expert interviews. This study will help to promote future research and business of AI4H in Korea.
In order to identify the stakeholders’ requirements for AI4H in Korea, we analyzed research funding, an online survey, and in-depth interviews. First, the analysis of research funding identified resources and projects supported by the government over time, reflecting trends and demands in AI4H research. Second, the online survey investigated perspectives regarding topics, data, and solutions that should be studied in AI4H. Third, in-depth expert interviews identified the status, problems, and solutions of AI4H in Korea. We designed the interviews using the sample, phenomenon of interest, design, evaluation, and research (SPIDER) framework as a qualitative method [15]. A semi-structured interview form was also used to guarantee that the same topics were addressed in each interview and to obtain various opinions from experts. More details on the interview design are provided in Supplementary Figure S1.
We focused on governmental research funding for AI4H in Korea to identify its trends. Research funding could be searched in the National Science and Technology Knowledge Information Service (NTIS) database platform. We searched for related AI4H projects from 2015 to 2019 using appropriate keywords [16]. More specifically, the following keywords related to AI4H were searched with synonyms and abbreviations: “healthcare AI,” “machine learning,” “standardization,” and “CDM.” We then extracted structured information, including project name, start date, subject, budget, implementation agency, and application status, from the NTIS before manually reviewing the information. Multi-year projects were counted once, in their starting year, in order to remove duplicates, and irrelevant projects were excluded. The data were categorized for analysis into pre-defined categories.
Between September 21 and 28, 2020, we conducted a survey to identify various expert opinions from members of the Korean Society of Artificial Intelligence in Medicine (KOSAIM) regarding the development of AI4H. The survey consisted of four questions regarding respondents’ affiliation, the target disease, the type of necessary data, and the target application (see Supplementary Figure S2). The affiliation question provided three possible answers: hospital, industry, or academia. Target diseases were submitted as free-text responses. The data types answers were divided into seven categories: laboratory test data, radiology images such as CT/MRI scans, genomic data, continuous vital signs, medical notes in free-text format including progress reports, radiology or pathology reports, and others. The target applications consisted of five items, including diagnostic prediction, prognosis prediction, treatment plan recommendation, monitoring, and others. A Google Form was used to implement the survey. We sent emails with a Google Form link to all participating members of the KOSAIM.
In-depth expert interviews were conducted with experts in three areas: hospitals, industry, and academia. We selected 13 experts among 30 candidates through internal meetings. Thereafter, to conduct the expert interviews, we implemented a semi-structured questionnaire consisting of five main points: introduction, initial discussion, questions about trends, essential questions, and closing. The questions identifying the status and problems of AI4H in experts’ practical environment were “What do you think of the current level of AI4H?”, “What are the limits of current AI4H?”, “What should be the directions of AI4H?”, and “What if healthcare AI expands enough, what challenges do we need to address?” All interviews were recorded with the interviewee’s agreement. We transcribed the interviews using the recorded files. According to the transcripts, the contents of the expert interviews were organized into six categories based on reviews by participant researchers: partnerships, training of human resources, technology for analysis, data, regulation, and others (see Supplementary Figure S1).
We used R version 4.0.2 for data analysis (https://www.rproject.org/). Categorical data were expressed as frequency and percentage. The chi-square test was performed to determine the relationships of categorical variables. We used two-sided tests with a statistical significance level of p < 0.05.
First, a total of 299 research projects were found in the NTIS database according to the inclusion criteria. After manually reviewing the candidate projects, 115 duplicated multi-year projects and 24 irrelevant projects were excluded. Finally, 160 projects were selected and analyzed.
The major data types used for AI4H during the past 5 years are shown in Table 1. As expected, among the 160 projects, the most common data type was radiology images (95 projects, 59.4%). Others (29 projects, 18.1%), including patient-reported outcome or digital phenotypes, ranked second. Laboratory data (6.9%), radiology/pathology reports (5.6%), and genomic data (5.0%) followed. The number of projects increased sharply in 2017.
Figure 1 depicts the average funding by target disease type per year. The unit of the funding scale is US $1,000. Dermatology-related diseases received the most funds (US $284,000), followed by pulmonary diseases (US $80,000), musculoskel et al system disorders (US $75,000), coronary artery diseases (US $60,000) and dementia (US $48,000).
A total of 101 KOSAIM members participated in the survey, 54 (53.5%) of whom worked in hospitals, 28 (27.7%) in academia, and 19 (18.8%) in industry.
Among the data demands in hospitals and academia, most of the responses indicated image types (27.6% and 20.5%) and laboratory data (22.1% and 16.7%). In contrast, researchers in industry expressed the highest demand for laboratory data (20.8%), followed by free-text reports (18.8%) and radiology/pathology reports (18.8%). The demand for free-text report analysis was higher than that for vital signs in hospitals and industry, but lower in academia (Table 2).
Figure 2 shows the results of a comparison of demand for data types between NTIS and survey responses. The patterns of data demands were significantly different (p < 0.05), especially regarding medical image data (59.4% for NTIS and 23.9% for survey responses).
Over half of AI4H software was related to the diagnosis prediction (33.3%) or prognosis prediction (31%). Interestingly, researchers in the hospital field, who comprised one-fifth of the respondents, chose treatment plan recommendations (19.4%) (Table 3).
Thirteen experts in the field of medical AI participated in the expert interviews, of whom three (23.1%) worked in hospitals, four (30.8%) in academia, and six (46.1%) in industry.
We identified the major topics, organizing them into five items by interviewer affiliation, as shown in Table 4.
Half of the major topics for AI4H related to data among experts in hospitals. In industry, regulation was the most commonly identified problem (34.0%), followed by data (23.4%). In academia, data were often identified as a problem (36.0%), but respondents also frequently mentioned education (28.0%) and regulation (20.0%). Other topics included medical charges, marketability, practical applications, medicolegal issues, and organization of governance for AI4H.
Table 5 shows the specific keywords. In the hospital field, most experts mentioned needs related to data, such as standardization, a common data model, and data certification. They specifically mentioned that available domestic open datasets are insufficient for technology development and performance evaluation. Moreover, they pointed out that common data models and data standards such as Health Level Seven (HL7) are required. Furthermore, the necessity of an internal organization in charge of hospital data governance was proposed.
In the industrial field, experts mainly mentioned regulations, practical efficacy evaluation, and data accessibility. Although the Korean Privacy Information Protection Act has been amended, it is still difficult to use clinical data in practice. Because of this limitation, it is difficult to evaluate medical AI solutions. There is a need for proactive deregulation. The participants also proposed changing the insurance reimbursement plan to promote the adoption of AI4H.
Experts in academia argued for privacy protection and long-term funds. They mentioned the necessity of long-term collaboration between academia, hospitals, and industry. Moreover, some of them discussed the practical applications and responsibilities of AI4H.
Most interviewees, regardless of their position, emphasized the importance of training for AI4H experts. AI4H is a multidisciplinary field requiring knowledge of medical areas and AI technology. Specifically, academically oriented needs of education require more options in methodology of fundamental technology. In contrast, the market needs practical courses for employees who want to develop essential AI4H techniques.
According to the increasing demand and interest in AI4H, we aimed to identify the practical needs of AI4H stakeholders. Previous studies have examined the status of AI4H, and our study expanded the research further to address practical issues regarding the AI4H development. We identified demands and trends through an analysis of NTIS data, survey responses, and expert interviews.
The analysis of 5 years of NTIS data showed that many studies focused on disease diagnosis using radiology image data, as shown in Table 1. AI solutions using image data already have high accuracy and have been commercialized [5–6]. Research using radiology image data has tended to decrease from 2018, which could indicate that the research on radiology images has already reached a certain level. As the level of research and technology advances, research on genomic data, psychology, mental illness, and digital phenotypes, which are areas where demand was relatively low, is also increasing. This trend can be seen in the results of the survey; the data demands are diverse, as shown in Figure 2.
As presented in Table 2, the data demands of hospitals and academia have similar patterns, but those of industry are different in terms of a focus on non-image data. European researchers also reported the strongest demand for electronic health records and patient-generated data [12]. Through these results, it can be expected that the research demand and diversity of AI4H will increase further in the future, as exemplified by multi-modal research using radiology images with various data including laboratory results and free text.
The identified issues and requirements are presented in Table 6. In AI4H, medical data are an essential resource for the development and evaluation of AI models. Since developing new drugs and diagnostic technologies through healthcare big data will eventually lead to improvements in public health, research in this field should be accessible. To address this need, the government must clarify the related laws and regulations. It is also important to strengthen privacy protection using various recent technologies, such as federated learning, homomorphic encryption, synthetic data, differential privacy, and dynamic consent [17–21]. Other countries also have been arguing for regulations including data protection and data sovereignty which are very similar considerations [12].
We identified needs for cohort and open dataset development supported by the government and the expansion of data quality certification for hospitals. To promote access to patient data in hospitals, it is necessary to establish a national healthcare big data system, such as the “All of Us” project in the United States [22]. The data should be FAIR (findable, accessible, interoperable, and reusable) [23]. With the FAIR guideline, data quality should also be guaranteed. Efforts for data quality management through standardization are also needed. To accelerate data quality management, a data certification program might be considered. The Korea Data Agency already runs a data certification center that screens and certifies data quality, data management, and data security to ensure the quality of the information systems used at institutions or companies [24]. Furthermore, a governance organization is required to manage and supervise the data evaluation methods and standardization of medical data [25].
Reimbursement for AI SaMD is the biggest issue in Korea, where there were 61 approved AI SaMDs in 2020, but none of them were listed in the National Health Insurance register [26]. Without reimbursement, it is difficult for hospitals in Korea to use these AI SaMDs. Therefore, a forward-looking policy is necessary. The Digital Health Applications of Germany [27], US Centers for Medicare & Medicaid Service New Technology Add-on Payment [28], and US Medicare Coverage of Innovative Technology [29] could be considered as benchmarks for insurance reimbursement. In terms of real-world applications and regulations, it is also necessary to develop data de-identification technology and ethical guidelines on AI4H.
Well-known institutions offer various AI courses. However, most courses are conducted in the short term and have limited options regarding methodologies of fundamental technology. Furthermore, there are few courses designed for the medical field with accompanying medical domain education. In order to lead and sustain AI4H in the future, long-term human resources development programs should be implemented.
There are several limitations of this study. First, there is the issue of selection bias with participants who presented opinions supporting AI4H. However, we selected KOSAIM members, who are experts in the field of medical AI, in order to obtain reliable results. In addition, the sample sizes of the survey and the expert interviews were relatively small. Second, as we examined research funding only in the NTIS database using the keyword “healthcare AI,” some research projects might have been excluded. Finally, meaningful opinions might have been excluded because the frequency of the terms in the interviews was used for analysis; however, the interview content was sufficiently discussed.
In conclusion, we identified technology, regulatory, and data issues for practical AI4H applications from the perspectives of experts in the hospital, industry, and academia fields in Korea. We found four main issues—laws and regulations, data utilization, reimbursement, and human resource development—and identified requirements for further research in AI4H.
Supplementary Materials
Supplementary materials can be found via https://doi.org/10.4258/hir.2022.28.2.143.
Figure S1. Semi-structured format of the expert interviews.
Figure S2. Survey format for the members of the Korean Society of Artificial Intelligence in Medicine (KOSAIM).
hir-2022-28-2-143-suppl.pdf
Acknowledgments
This research was supported by a grant from the Korea Health Information Service (2020).
References
1. BIS Research. Global artificial intelligence market in healthcare sector: analysis & forecasts, 2017–2025 [Internet]. Fremont (CA): BIS Research;2017. [cited at 2022 Jan 10]. Available from: https://bisresearch.com/industry-report/global-artificial-intelligence-market-2025.html
.
2. Markets and Markets. Artificial Intelligence in healthcare market by offering (hardware, software, services), technology (machine learning, NLP, context-aware computing, computer vision), application, end user and geography - global forecast to 2027 [Internet]. Northbrook (IL): Markets and Markets;2020. [cited at 2022 Jan 10]. Available from: https://www.marketsandmarkets.com/Market-Reports/artificial-intelligence-healthcare-market-54679303.html
.
3. Research and Markets. Global clinical decision support system market is forecast to reach EUR 277,79 billion by 2025 growing at a CAGR of 11% [Internet]. New York (NY): Markets Insider;2020. [cited at 2022 Jan 10]. Available from: https://markets.businessinsider.com/news/stocks/global-clinical-decision-support-system-market-is-forecast-to-reach-usd-317-billion-by-2025-growing-at-a-cagr-of-11-1029817665
.
4. Mahler M. Precision medicine and artificial intelligence: the perfect fit for autoimmunity. Cambridge (MA): Academic Press;2021.
5. The Medical Futurist. FDA-approved A.I.-based algorithms [Internet]. Budapest, Hungary: The Medical Futurist;2021. [cited at 2022 Jan 10]. Available from: https://medicalfuturist.com/fda-approved-ai-basedalgorithms/
.
6. Ministry of Food and Drug Safety. Development of medical device international standard guideline for artificial intelligence [Internet]. Cheongju, Korea: Ministry of Food and Drug Safety;2020. [cited at 2022 Jan 10]. Available from: https://www.mfds.go.kr/brd/m_99/view.do?seq=44588&srchFr=&srchTo=&srchWord=&srchTp=&itm_seq_1=0&itm_seq_2=0&multi_itm_seq=0&company_cd=&company_nm=&page=1
.
7. Wu E, Wu K, Daneshjou R, Ouyang D, Ho DE, Zou J. How medical AI devices are evaluated: limitations and recommendations from an analysis of FDA approvals. Nat Med. 2021; 27(4):582–4.


8. Shen J, Zhang CJ, Jiang B, Chen J, Song J, Liu Z, et al. Artificial intelligence versus clinicians in disease diagnosis: systematic review. JMIR Med Inform. 2019; 7(3):e10010.


9. Liu X, Faes L, Kale AU, Wagner SK, Fu DJ, Bruynseels A, et al. A comparison of deep learning performance against health-care professionals in detecting diseases from medical imaging: a systematic review and meta-analysis. Lancet Digit Health. 2019; 1(6):e271–e297.


10. Perrault R, Shoham Y, Brynjolfsson E, Clark J, Etchemendy J, Grosz B, et al. The artificial intelligence index 2019 annual report [Internet]. Stanford (CA): Stanford University;2019. [cited at 2022 Jan 10]. Available from: https://hai.stanford.edu/sites/default/files/ai_index_2019_report.pdf
.
11. Matheny M, Israni ST, Auerbach A, Beam A, Bleicher P, Chapman W, et al. Artificial intelligence in health care: the hope, the hype, the promise, the peril [Internet]. Washington (DC): National Academy of Medicine;2019. [cited at 2022 Jan 10]. Available from: https://nam.edu/artificial-intelligence-special-publication/
.
12. Big Data Value Association. AI in healthcare whitepaper [Internet]. Brussel, Belgium: Big Data Value Association;2020. [cited at 2022 Jan 10]. Available from: https://www.bdva.eu/sites/default/files/AI%20in%20Healthcare%20Whitepaper_November%202020_0.pdf
.
13. Park CW, Seo SW, Kang N, Ko B, Choi BW, Park CM, et al. Artificial Intelligence in health care: current applications and issues. J Korean Med Sci. 2020; 35(42):e379.


14. Lee D, Yoon SN. Application of artificial intelligence-based technologies in the healthcare industry: opportunities and challenges. Int J Environ Res Public Health. 2021; 18(1):271.


15. Cooke A, Smith D, Booth A. Beyond PICO: the SPIDER tool for qualitative evidence synthesis. Qual Health Res. 2012; 22(10):1435–43.
16. National Science & Technology Information Service [Internet]. Daejeon, Korea: National Science & Technology Information Service;2022. [cited at 2022 Jan 10]. Available from: https://www.ntis.go.kr/en/GpIndex.do
.
17. Konecny J, McMahan HB, Yu FX, Richtarik P, Suresh AT, Bacon D. Federated learning: strategies for improving communication efficiency [Internet]. Ithaca (NY): arXiv.org;2017. [cited at 2022 Jan 10]. Available from: https://arxiv.org/abs/1610.05492
.
18. Gentry C. Fully homomorphic encryption using ideal lattices. In : Proceedings of the 41st Annual ACM Symposium on Theory of Computing (STOC); ; 2009 May 31–Jun 2; Bethesda, MA. p. 169–78.


19. Bellovin SM, Dutta PK, Reitinger N. Privacy and synthetic datasets. Stanf Technol Law Rev. 2019; 22(1):1–52.


20. Dwork C, Roth A. The algorithmic foundations of differential privacy. Found Trends Theor Comput Sci. 2014; 9(3–4):211–407.


21. Kaye J, Whitley EA, Lund D, Morrison M, Teare H, Melham K. Dynamic consent: a patient interface for twenty-first century research networks. Eur J Hum Genet. 2015; 23(2):141–6.


22. All of Us Research Program Investigators. The “All of Us” Research Program. N Engl J Med. 2019; 381(7):668–76.
23. Wilkinson MD, Dumontier M, Aalbersberg IJ, Appleton G, Axton M, Baak A, et al. The FAIR Guiding Principles for scientific data management and stewardship. Sci Data. 2016; 3:160018.


24. Korea Data Agency. K-DATA [Internet]. Seoul, Korea: Korea Data Agency;2021. [cited at 2022 Jan 10]. Available from: http://global.kdata.or.kr/en/kdata/
.
25. Jeon JH, Lee KC. Top 10 key standardization trends and perspectives on artificial intelligence in medicine. Electron Telecommun Trends. 2020; 35(2):1–16.
26. National Evidence-based Healthcare Collaborating Agency. Case report of new medical technology evaluation [Internet]. Seoul, Korea: National Evidence-based Healthcare Collaborating Agency;2020. [cited at 2022 Jan 10]. Available from: https://nhta.neca.re.kr/nhta/publication/nhtaU0605V.ecg?seq=8970
.
27. Federal Institute for Drugs and Medical Devices. The fast-track process for Digital Health Applications (DiGA) according to Section 139e SGB V [Internet]. Bonn, Germany: Federal Institute for Drugs and Medical Devices;2020. [cited at 2022 Jan 10]. Available from: https://www.bfarm.de/SharedDocs/Downloads/EN/MedicalDevices/DiGA_Guide.pdf?__blob=publicationFile&v=2
.
28. Centers for Medicare & Medicaid Services. New medical services and new technologies [Internet]. Baltimore (MD): Centers for Medicare & Medicaid Services;2021. [cited at 2022 Jan 10]. Available from: https://www.cms.gov/Medicare/Medicare-Fee-for-Service-Payment/AcuteInpatientPPS/newtech/
.
29. Federal Register. Medicare Program: Medicare coverage of innovative technology (MCIT) and definition of “Reasonable and Necessary”: delay of effective date [Internet]. Washington (DC): Federal Register;2021. [cited at 2022 Jan 10]. Available form: https://www.federalregister.gov/documents/2021/05/18/2021-10466/medicareprogram-medicare-coverage-of-innovative-technologymcit-and-definition-of-reasonable-and
.
Figure 2
Comparison of data types between NTIS (from 2015 to 2019) and the KOSAIM members’ survey in 2020.
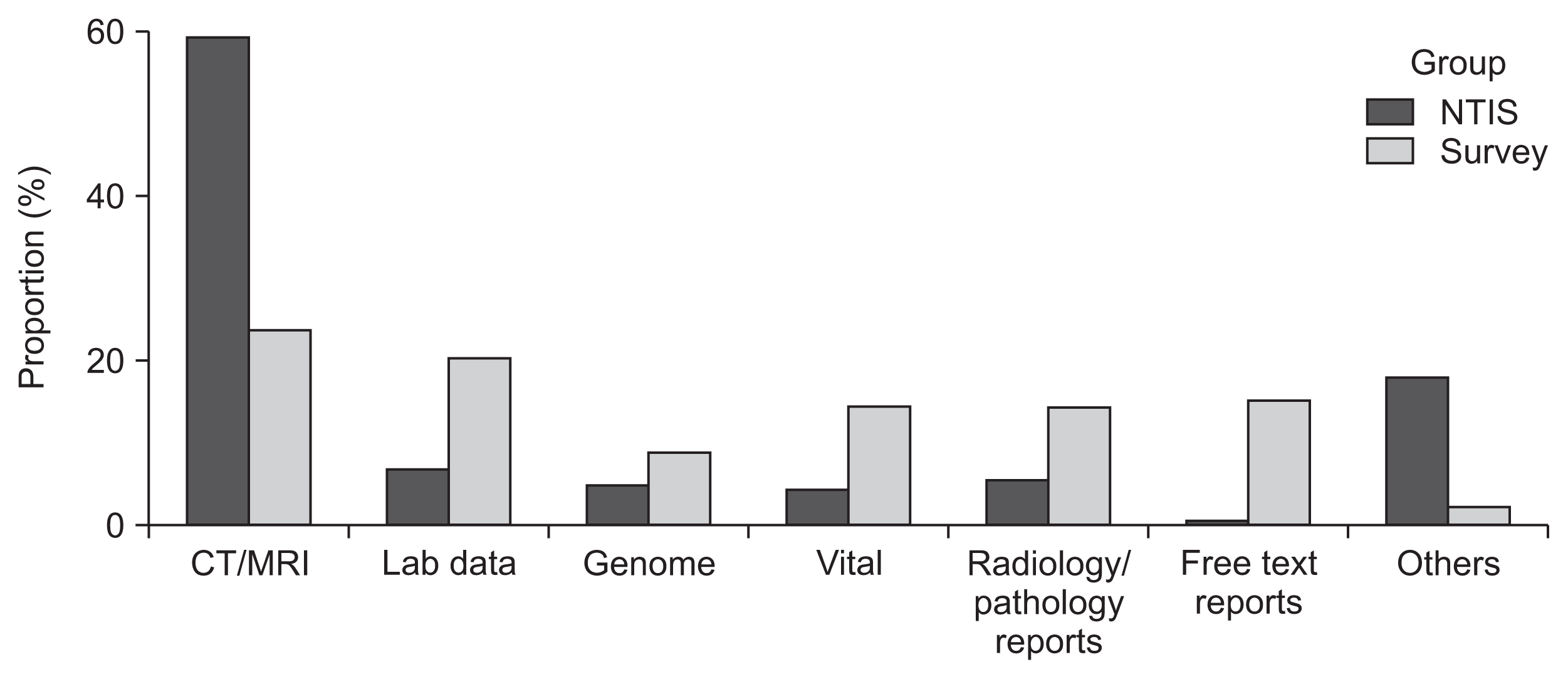
Table 1
Major data types during the past 5 years
2015 | 2016 | 2017 | 2018 | 2019 | All | |
---|---|---|---|---|---|---|
CT/MRI | 2 (66.7) | 2 (40.0) | 22 (57.9) | 37 (62.7) | 32 (58.2) | 95 (59.4) |
Lab data | 0 (0) | 0 (0) | 4 (10.5) | 4 (6.8) | 3 (5.5) | 11 (6.9) |
Genome | 0 (0) | 1 (20.0) | 1 (2.6) | 2 (3.4) | 4 (7.3) | 8 (5.0) |
Vital | 0 (0) | 0 (0) | 2 (5.3) | 2 (3.4) | 3 (5.5) | 7 (4.4) |
Radiology/pathology reports | 1 (33.3) | 0 (0) | 2 (5.3) | 2 (3.4) | 4 (7.3) | 9 (5.6) |
Free text reports | 0 (0) | 1 (20.0) | 0 (0) | 0 (0) | 0 (0) | 1 (0.6) |
Othersa | 0 (0) | 1 (20.0) | 7 (18.4) | 12 (20.3) | 9 (16.4) | 29 (18.1) |
All | 3 (100) | 5 (100) | 38 (100) | 59 (100) | 55 (100) | 160 (100) |
Table 2
Data demands for AI development
Hospital | Industry | Academia | All | |
---|---|---|---|---|
CT/MRI | 45 (27.6) | 8 (16.7) | 16 (20.5) | 69 (23.9) |
Lab data | 36 (22.1) | 10 (20.8) | 13 (16.7) | 59 (20.4) |
Genome | 10 (6.1) | 4 (8.3) | 12 (15.4) | 26 (9.0) |
Vital | 22 (13.5) | 7 (14.6) | 13 (16.7) | 42 (14.5) |
Radiology/pathology reports | 23 (14.1) | 9 (18.8) | 10 (12.8) | 42 (14.5) |
Free text reports | 25 (15.3) | 9 (18.8) | 10 (12.8) | 44 (15.2) |
Othersa | 2 (1.2) | 1 (2.1) | 4 (5.1) | 7 (2.4) |
All | 163 (100) | 48 (100) | 78 (100) | 289 (100) |
Table 3
Solution demands for AI development
Hospital | Industry | Academia | All | |
---|---|---|---|---|
Diagnosis prediction | 35 (32.4) | 8 (32.0) | 14 (36.8) | 57 (33.3) |
Prognosis prediction | 32 (29.6) | 8 (32.0) | 13 (34.2) | 53 (31.0) |
Monitoring | 16 (14.8) | 5 (20.0) | 6 (15.8) | 27 (15.8) |
Treatment plan recommendation | 21 (19.4) | 4 (16.0) | 4 (10.5) | 29 (17.0) |
Othersa | 4 (3.7) | 0 (0) | 1 (2.6) | 5 (2.9) |
All | 108 (100) | 25 (100) | 38 (100) | 171 (100) |
Table 4
Comparison of major topics by affiliation in the expert interviews
Table 5
Keywords of expert interviews
Table 6
Identified issues and requirements of stakeholders