RESULTS
Data of 8,958 patients (3,624 patients in Seoul St. Mary’s Hospital, 896 in Chungbuk National University Hospital, 3,809 in Seoul National University Hospital and 629 in Hallym University Dongtan Sacred Heart Hospital) administered with SGLT2i between January 1, 2012, and May 31, 2019 were collected (
Fig. 1). Of the patients, 5% (449/8,958) had ABGA after the index date. Among them, 0.35% (31/8,958) had pH < 7.3 and bicarbonate < 18 mmHg; however, 15 tests were performed after discontinuing SGLT2i for more than three months. Therefore, SGLT2i-related DKA was suspected in 0.18% (16/8,958) patients.
Approximately 95% (8,509/8,958) of patients had no ABGA after the index date. Among the patients who were suspected of not having SGLT2i-related DKA, we found 15 patients with the “DKA” diagnosis. Eight of these were not newly diagnosed after the index date. After three or more months of discontinuing SGLT2i, five of the remaining seven patients with the “DKA” diagnosis were confirmed with general DKA not related to SGLT2i use. Therefore, only two patients were suspected of having SGLT2i-related DKA. The 94.8% (8,494/8,958) of patients who did not have ABGAs and did not have a “DKA” diagnosis were excluded from consideration for SGLT2i-related DKA. Finally, 99.1% (8880/8958) showed “less likely DKA.”
The results of 18 patients who were identified as having SGLT2i-related DKA by ABGA are listed in
Table 1. In addition, details of the two patients who were identified as having SGLT2i-related DKA by the named diagnosis are summarized in
Table 2. Only one case out of the 18 cases (Patient 1, hereafter abbreviated as Pt 1) was confirmed to have SGLT2i-related DKA in chart reviews. The temporal and sequential relationship between SGLT2i prescription and DKA was clear, and the blood glucose and ketone levels indicated SGLT2i-related DKA. Nine cases were confirmed or suspected as general DKA. One of these cases (Pt 2), where the patient was unable to receive insulin while traveling and the blood glucose level at the emergency department was 635 mg/dL, was confirmed as a general DKA case. In the remaining eight cases (Pt 3–Pt 10), it was difficult to find an association with SGLT2i, and a likelihood of high anion gap metabolic acidosis due to factors other than DKA existed. However, because a “DKA” ICD-10 classification was included in the EMR, these eight cases were classified as suspected general DKA to reflect the opinion of the medical staff at the time. In one of the cases (Pt 10), there was a prescription for SGLT2i in the EMR; however, the chart review confirmed that the patient did not take SGLT2i, and general DKA was suspected. Furthermore, the medical records showed a “DKA” diagnosis for four patients (Pt 11–Pt 14) for whom DKA was excluded from the actual chart review. The ABGA values of two patients (Pt 11, Pt 12) were measured immediately after liver transplantation and that of one (Pt 13) was measured during surgery. In other words, the findings suggested a high anion gap metabolic acidosis due to other causes. The other patient (Pt 14) showed a normal anion gap; thus, DKA could be excluded. In addition, for two cases (Pt 15, Pt 16), DKA could neither be suspected nor excluded through chart review alone. A patient who was prescribed SGLT2i at another hospital visited the emergency department (Pt 17), and it was difficult to judge DKA through ABGA alone. Even when there was no record of DKA and only the diagnosis name existed (Pt 18), DKA could not be confirmed (
Table 2).
Table 1
Chart review by endocrinologists of patients diagnosed with “Confirmed DKA” in our algorithm
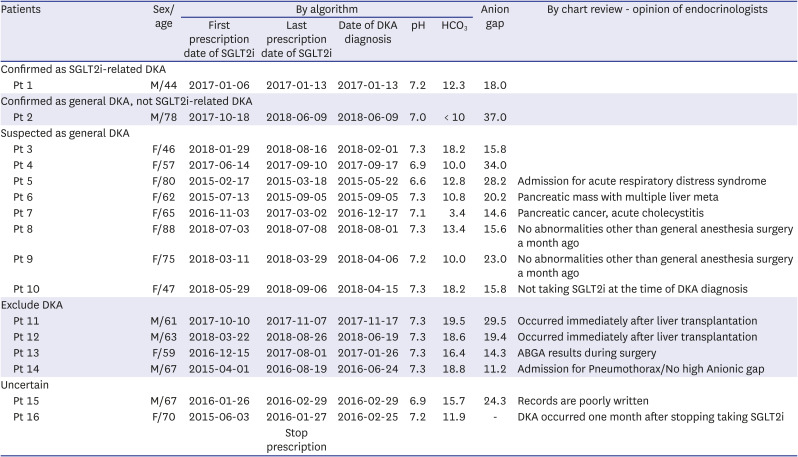
Patients |
Sex/age |
By algorithm |
Anion gap |
By chart review - opinion of endocrinologists |
First prescription date of SGLT2i |
Last prescription date of SGLT2i |
Date of DKA diagnosis |
pH |
HCO3
|
Confirmed as SGLT2i-related DKA |
|
|
|
|
|
|
|
|
|
Pt 1 |
M/44 |
2017-01-06 |
2017-01-13 |
2017-01-13 |
7.2 |
12.3 |
18.0 |
|
Confirmed as general DKA, not SGLT2i-related DKA |
|
|
|
|
|
|
|
|
|
Pt 2 |
M/78 |
2017-10-18 |
2018-06-09 |
2018-06-09 |
7.0 |
< 10 |
37.0 |
|
Suspected as general DKA |
|
|
|
|
|
|
|
|
|
Pt 3 |
F/46 |
2018-01-29 |
2018-08-16 |
2018-02-01 |
7.3 |
18.2 |
15.8 |
|
|
Pt 4 |
F/57 |
2017-06-14 |
2017-09-10 |
2017-09-17 |
6.9 |
10.0 |
34.0 |
|
|
Pt 5 |
F/80 |
2015-02-17 |
2015-03-18 |
2015-05-22 |
6.6 |
12.8 |
28.2 |
Admission for acute respiratory distress syndrome |
|
Pt 6 |
F/62 |
2015-07-13 |
2015-09-05 |
2015-09-05 |
7.3 |
10.8 |
20.2 |
Pancreatic mass with multiple liver meta |
|
Pt 7 |
F/65 |
2016-11-03 |
2017-03-02 |
2016-12-17 |
7.1 |
3.4 |
14.6 |
Pancreatic cancer, acute cholecystitis |
|
Pt 8 |
F/88 |
2018-07-03 |
2018-07-08 |
2018-08-01 |
7.3 |
13.4 |
15.6 |
No abnormalities other than general anesthesia surgery a month ago |
|
Pt 9 |
F/75 |
2018-03-11 |
2018-03-29 |
2018-04-06 |
7.2 |
10.0 |
23.0 |
No abnormalities other than general anesthesia surgery a month ago |
|
Pt 10 |
F/47 |
2018-05-29 |
2018-09-06 |
2018-04-15 |
7.3 |
18.2 |
15.8 |
Not taking SGLT2i at the time of DKA diagnosis |
Exclude DKA |
|
|
|
|
|
|
|
|
|
Pt 11 |
M/61 |
2017-10-10 |
2017-11-07 |
2017-11-17 |
7.3 |
19.5 |
29.5 |
Occurred immediately after liver transplantation |
|
Pt 12 |
M/63 |
2018-03-22 |
2018-08-26 |
2018-06-19 |
7.3 |
18.6 |
19.4 |
Occurred immediately after liver transplantation |
|
Pt 13 |
F/59 |
2016-12-15 |
2017-08-01 |
2017-01-26 |
7.3 |
16.4 |
14.3 |
ABGA results during surgery |
|
Pt 14 |
M/67 |
2015-04-01 |
2016-08-19 |
2016-06-24 |
7.3 |
18.8 |
11.2 |
Admission for Pneumothorax/No high Anionic gap |
Uncertain |
|
|
|
|
|
|
|
|
|
Pt 15 |
M/67 |
2016-01-26 |
2016-02-29 |
2016-02-29 |
6.9 |
15.7 |
24.3 |
Records are poorly written |
|
Pt 16 |
F/70 |
2015-06-03 |
2016-01-27 |
2016-02-25 |
7.2 |
11.9 |
- |
DKA occurred one month after stopping taking SGLT2i |
Stop prescription |

Table 2
Chart review by endocrinologists of patients diagnosed with “DKA possibility” in our algorithm

Patients |
Sex/age |
By algorithm |
By chart review |
First prescription date of SGLT2i |
Last prescription date of SGLT2i |
Date of DKA diagnosis |
pH |
HCO3
|
Anion gap |
Opinion of endocrinologists |
Pt 17 |
M/63 |
Prescribing at another hospital |
2018-04-03 |
6.9 |
9.8 |
19.2 |
Exclude DKA |
Results at the time of death due to hepatic failure |
Pt 18 |
M/20 |
2016-11-28 |
2018-05-30 |
2016-11-28 |
- |
- |
- |
Uncertain |
No record of DKA, only presence of ICD-10 codes |

As a result, the positive predictive value (PPV) of our algorithm based on “confirmed DKA” was only 6.3% (1/16 patients) (
Supplementary Table 2). Even if the algorithm test date criteria cutoff was changed to within 1 month (6.7%, 1/15), within 15 d (7.7%, 1/13), and within 7 d (10.0%, 1/10) after stopping SGLT2i, the PPV was considerably low. There was no statistically significant difference in the interval after stopping SGLT2i (
P > 0.999) (
Supplementary Table 2).
DISCUSSION
Recently, real-world evidence (RWE), which creates large amounts of data in a short time, has become a major subject in the field of medicine.
8 However, there are many concerns about various uncontrolled biases in RWE that have not been clearly resolved,
9 and various OPs have been proposed to address them.
12 Because CDMs for multicenter research are considered RWE, they are also of concerns. In this research, we aimed to create a detection algorithm for SGLT2i-related DKA based on CDM data. Further, we attempted to judge the reliability of this method using direct chart reviews. The results of the developed algorithm were highly unsatisfactory.
DKA has a clear diagnostic standard based on high anion gap metabolic acidosis,
11 which is suitable for retrospective cohort research. The existence of a definite test value for diagnosing DKA using EMR data means that it is possible to obtain a clear diagnosis using an algorithm. Moreover, because DKA is a life-threatening side effect, diagnosis via the ICD-10 classification itself must be relatively accurate. Therefore, we initially believed that SGLT2i-related DKA could be accessed with only two items: ABGA and ICD-10 classification. In addition, SGLT2i-related DKA is a very rare side effect.
345 To measure the incidence of such rare side effects, a cohort study with many samples is more useful than a randomized controlled trial (RCT)
8 because it is difficult to generalize a DKA incidence from a small-sample-sized RCT to the entire real-world population. Therefore, we performed a cohort study. We believe it is possible to objectively estimate the incidence of SGLT2i-related DKA occurring in real clinical settings. However, the most problematic consideration in creating the proposed detection algorithm was that SGLT2i-related DKA has very different clinical features from general DKA.
4131415 Considering the various causes of DKA, the goal was to clearly distinguish between them and select only SGLT2i-related DKA. Thus, we repeatedly upgraded our algorithm by consulting with seven endocrinologists.
In this study, laboratory findings of glucose, sodium, and chloride levels were not considered in the diagnosis of SGLT2i-related DKA; only pH and bicarbonate levels were considered in the algorithm. Therefore, including all the diagnostic criteria of general DKA in the diagnosis algorithm would help in diagnosing SGLT2i-related DKA accurately. Although the sensitivity of the diagnosis of SGLT2i-related DKA could be increased in this case, a significant underestimation was likely as well because it is sometimes unnecessary to confirm the DKA diagnosis criteria to confirm SGLT2i-related DKA in an actual clinical setting. Nevertheless, only some tests included in the actual DKA diagnostic criteria are considered in the final diagnosis. In other words, there is a possibility of missing the diagnosis of real SGLT2i-related DKA. Conversely, if only a part of the diagnostic criteria is included, the likelihood of missing the real SGLT2i-related DKA decreases; however, the likelihood of overestimation will increase. Therefore, we planned to confirm SGLT2i-related DKA through direct chart reviews in early stages of algorithm development. Consequently, only pH and bicarbonate values—the core diagnostic criteria of DKA—were used to induce the overestimation of SGLT2i-related DKA diagnosis. In addition, a direct chart review was conducted on patients with suspected SGLT2i-related DKA.
According to various RCT results, SGLT2i-related DKA incidence is reported to be 0.02–0.1%.
141617 However, as mentioned earlier, large-scale cohort studies may be more useful in studying side effects with a low incidence, such as SGLT2i-related DKA. Furthermore, the occurrence of SGLT2i-related DKA not recognized by medical staff at the actual treatment site is expected to be more frequent. It is also reported that the actual diagnosis rate is lower than the suspected occurrence rate of SGLT2i-related DKA.
18 Here, “confirmed DKA” was found for 0.25% (22/8,958) patients using the algorithm, which achieved our initial goal of overestimating the diagnosis of SGLT2i-related DKA. In this study, only one case of SGLT2i-related DKA (0.01%, 1/8,958) was reported using chart review, which aligned with the results of other studies. Because our study planned direct chart review by experts from the beginning, we minimized the criteria of OP and secured many overestimated cases. Therefore, it was expected that SGLT2i-related DKA would be higher than that in the RCT; however, the results were opposite. There was also a likelihood that the physicians did not recognize SGLT2i-related DKA nor perform active laboratory tests.
The anion gap is an essential diagnostic criterion of DKA; nevertheless, it was excluded from the proposed algorithm. Serum sodium and serum chloride values are required to calculate the anion gap.
1119 However, an endocrinologist checked the anion gap during the actual chart review and found that, in a few cases, sodium and chloride were measured at the same time as ABGA. In other words, the anion gap values in the EMR data may have been unreliable. Therefore, we were concerned that too many patients would be excluded if the anion gap was included in the diagnostic criteria. After reviewing the chart, in some cases, general DKA could be confirmed because of a high anion gap; however, in some cases, confirming SGLT2i-related DKA was difficult. This is considered an intrinsic limitation of EMR-based retrospective cohort research in which only correlations can be measured without considering causalities between SGLT2i and DKA.
912 Despite the chart reviews, the main reason for the difficulty in distinguishing between general DKA and SGLT2i-related DKA is that EMR data are not sufficiently reliable.
12 Unless medical staff clearly suspect SGLT2i-related DKA, data may be insufficient, and the contents of the test results or charts would not reflect the patient’s situation.
20 The anion gap, for example, helps diagnose DKA and can help in tracking whether ketosis improves in these situations.
11 However, through direct chart review, we determined that normal anion gap acidosis may occur if the medical staff do not anticipate DKA in advance and perform intravenous hydration for other treatment purposes. Furthermore, high anion gap metabolic acidosis does not only occur in DKA; it may be caused by renal failure, rhabdomyolysis, lactic acidosis, and other drug- and alcohol-related intoxication, including ketoacidosis.
2122 If a detailed medical history is not recorded in the EMR, identifying the cause of DKA will be difficult. Thus, there is not only less confidence in the test results but also no clear diagnostic standard that satisfies the diagnostic criteria of SGLT2i-related DKA in the clinical setting.
The diagnosis of SGLT2i-related DKA may have been delayed because SGLT2i-related DKA, unlike general DKA, can occur at normal blood glucose levels.
131623 Moreover, glucose levels could not be included in the diagnostic criteria because relevant data were not recorded at the treatment site. Thus, we believe that SGLT2i-related DKA diagnosis may have been overestimated owing to the lack of glucose level and anion gap data in the algorithm.
Because DKA is a serious complication and direct chart review by medical staff is necessary for accurate diagnosis, overestimation and not underestimation was more appropriate for this study. When analyzing SGLT2i-related DKA, the relationship between the period when SGLT2i is used and that when DKA occurs must be understood. In this study, DKA occurring within three months of discontinuing SGLT2i administration was assumed to be SGLT2i-related DKA. This also contributed toward overestimation of the detection of SGLT2i-related DKA. The period of three months was arbitrarily defined to induce overestimation and because chart reviews were planned. However, according to actual chart reviews, we found that this period did not correlate with the occurrence of SGLT2i-related DKA. Furthermore, information on disturbance variables that are likely to affect the occurrence of SGLT2i-related DKA should be collected. Although it is mentioned that the data must be acquired continuously, selecting SGLT2i-related DKA accurately is difficult because EMR data do not include the various causes of DKA. The goal was to upgrade the algorithm through continuous feedback based on chart reviews. Thus, algorithms and not manual decision-making should be used for decision support at this point.
After the suspension of SGLT2i, a significant aspect was noted at the time of diagnosing DKA via chart review. Even when DKA occurrence was suspected in the past, SGLT2i was sometimes still prescribed (Pt 3, Pt 7, Pt 10, Pt 12, Pt 13, and Pt 14). In addition, SGLT2i was continuously prescribed for elderly patients for whom SGLT2i was not recommended (Pt 2, Pt 5, Pt 8, and Pt 9). Although it is questionable to conclude that DKA was caused by such prescriptions, it is an important point indicating that more careful prescriptions are needed.
Our results make it difficult to clearly determine a specific disease using only OPs. This is especially true when various causes of DKA exist, although test results may indicate DKA. Using an endocrinologist’s chart review, it was often possible to suspect high anion gap metabolic acidosis owing to factors other than DKA. However, because the ICD-10 DKA classification existed in the medical records, we decided to rely on that DKA diagnosis. In other words, it was assumed that the data extracted from this study corresponded to DKA; subsequently, we focused on determining whether the DKA was due to SGLT2i or other causes. The primary focus was to distinguish SGLT2i-related DKA from general DKA to a substantial extent; however, this was difficult without direct chart reviews. Moreover, as shown in our study, the detection of SGLT2i-related DKA through manual chart reviews was difficult. Consequently, achieving such detection with an algorithm would also be difficult. Our results show that SGLT2i-related DKA could be suspected due to DKA occurrence while SGLT2i was administered. Nonetheless, it was very difficult to evaluate this aspect using an algorithm alone. Moreover, when detecting diagnoses based on the ICD-10 classification, we extracted only DKA data. However, we should have also identified general metabolic acidosis because the medical staff might have failed to recognized the association with SGLT2i. The definitive OP with medical knowledge must be studied and analyzed in the future. Approaches through various fields, such as drug dynamics and continuous algorithm updates according to expert chart reviews, will be required because the results for SGLT2i-related DKA depend on the OP.
12 In this study, although algorithm updates were performed seven times, the algorithm failed to accurately determine cases of specific diseases. To detect a rare disease, the specificity and negative predictive values (NPVs) of the algorithm must be increased; however, this may vary depending on specific purposes. Thus, the algorithm should be allowed to perform overestimations and consider all situations wherein DKA is likely to occur. Moreover, a direct chart review is indispensable for updating the algorithm via continuous chart reviews.
In addition to the aforementioned limitation, our study has the inherent limitations common to retrospective cohort studies. First, SGLT2i medication compliance, the most important factor in adverse drug reactions, is unclear. Second, DKA is a serious endocrinological emergency. If an actual DKA occurs, the patients get treated at a nearby hospital and not where the SGLT2i was prescribed. In such cases, medical staff should prioritize checking the charts, if available. Finally, to determine the accuracy of the algorithm, the sensitivity, specificity, PPV, and NPV must be evaluated. However, not all patients taking SGLT2i were chart-reviewed in this study—only PPVs were possible. However, because PPV was low (6.3–10.0%), calculating the remaining sensitivity, specificity, and NPV was not relevant. A high PPV in diseases with very low prevalence is not expected.
We used a CDM in this study. Similar to the retrospective cohort studies, CDMs require a unified OP because different results are obtained depending on the design of OP.
12 Therefore, actual patient data should be checked to verify if the OP was well crafted. Although CDM is a multicenter trial, it has the advantage of protecting the privacy of the study subjects because personal information is not collected. However, verification is often impossible owing to this protective measure. Some studies have highlighted that medical product safety surveillance is a strength of retrospective cohort studies, including CDMs.
824 However, it is uncertain if side effects can be appropriately detected in the retrospective cohort studies that identify only correlations and not causalities.
912 Owing to the nature of such retrospective cohort research, the OP accuracy must be verified by random direct chart reviews. Protecting patients’ personal information is important; however, in retrospective cohort studies, data revalidation is also crucial. Studies that can be performed with CDMs must be distinguished from those that cannot. A CDM emphasizing privacy has limited practical reliability because the OP of a specific disease cannot be verified.
In this study, directly checking the SGLT2i-related DKA based on the actual chart review and upgrading the algorithm accordingly had limitations. Owing to the limitations in EMR data, an algorithm that clearly differentiates SGLT2i-related DKA is not possible. However, the novelty of our study lies in the limitations of each step in the OP algorithm being clinically and realistically presented. We suggested the implications of the research results and methods of upgrading the algorithm. In addition, we analyzed physicians’ responses to DKA at each stage. Before proceeding with clinical studies, the purpose and results of the study should be carefully considered; therefore, active participation by clinicians is required. Therefore, it a sufficient validation for OP of a specific disease is required before CDM research. Otherwise, an open multicenter trial that can understand the patient’s condition may be much better than CDM. Notably, the field of medicine emphasizes not only data abundance but also data quality.