Abstract
Background
Methods
Results
Conclusion
ACKNOWLEDGMENTS
Notes
DATA AVAILABILITY STATEMENT
The datasets generated during and/or analyzed during the current study are available from the corresponding author on reasonable request.
AUTHOR CONTRIBUTIONS
Conceptualization: Yuseon Cheong, Gyu-Sam Hwang. Data curation: Yuseon Cheong, Kyoung-Sun Kim. Formal analysis: Yuseon Cheong, Bo-Hyun Sang. Funding acquisition: Gyu-Sam Hwang. Methodology: Bo-Hyun Sang. Project administration: Gyu-Sam Hwang. Visualization: Sangho Lee. Writing - original draft: Yuseon Cheong, Sangho Lee, Do-Kyeong Lee. Writing - review & editing: Gyu-Sam Hwang. Investigation: Kyoung-Sun Kim Resources: Sangho Lee. Software: Do-Kyeong Lee. Supervision: Gyu-Sam Hwang. Validation: Kyoung-Sun Kim.
REFERENCES
Fig. 1.
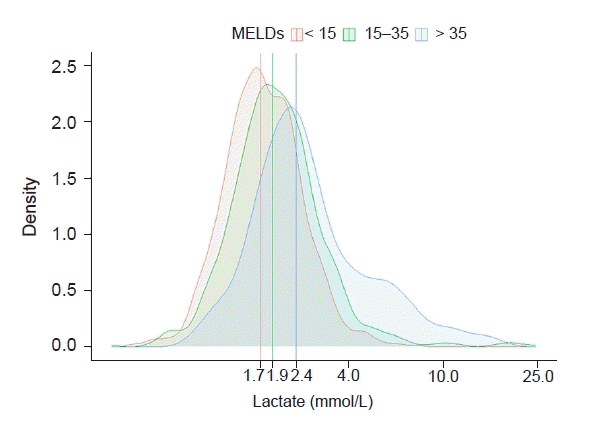
Fig. 2.
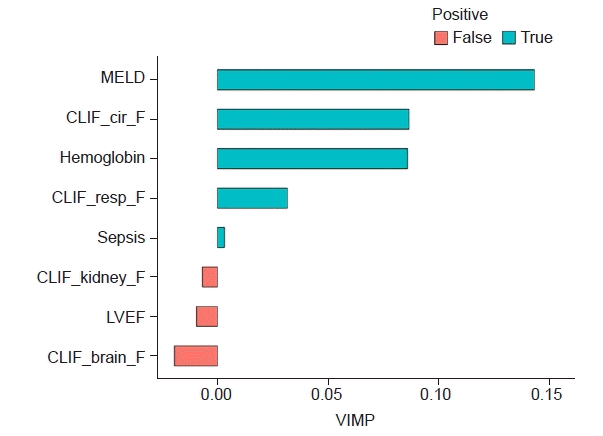
Fig. 3.
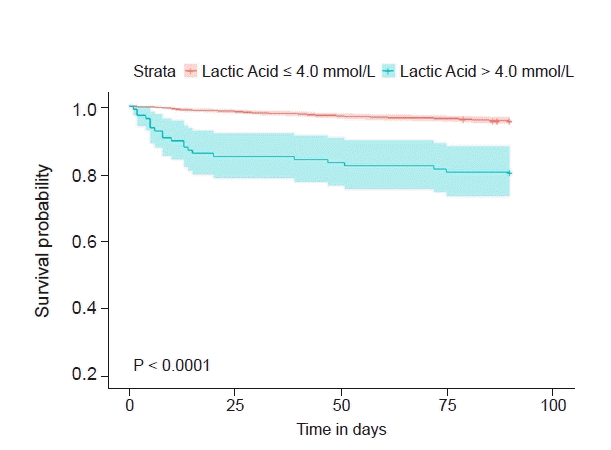
Table 1.
Variable | Lactate ≤ 4 mmol/L (n = 1,895) | Lactate > 4 mmol/L (n = 107) | Total (n = 2,002) | P value |
---|---|---|---|---|
Sex, male | 1,408 (74.3) | 81 (75.7) | 1,489 (74.4) | 0.834 |
Age (yr) | 53.0 (48.0, 58.0) | 53.0 (46.5, 57.0) | 53.0 (48.0, 58.0) | 0.563 |
BMI (kg/m2) | 24.0 (21.9, 26.5) | 23.7 (21.0, 26.3) | 24.0 (21.8, 26.4) | 0.058 |
Re-transplantation | 77 (4.1) | 14 (13.1) | 91 (4.5) | < 0.001† |
OLT | 326 (17.2) | 58 (54.2) | 384 (19.2) | < 0.001† |
CAD | 79 (6.3) | 8 (12.1) | 87 (6.6) | 0.112 |
CVA | 15 (0.9) | 1 (1.2) | 16 (0.9) | 1.000 |
Diabetes mellitus | 459 (24.2) | 29 (27.1) | 488 (24.4) | 0.576 |
Hypertension | 273 (14.4) | 17 (15.9) | 290 (14.5) | 0.778 |
MELD | 15.0 (10.0, 24.0) | 34.0 (21.0, 41.0) | 16.0 (10.0, 26.0) | < 0.001† |
Causes of liver disease | ||||
Hepatitis B virus | 1192 (62.9) | 54 (50.5) | 1246 (62.2) | 0.013* |
Hepatitis C virus | 135 (7.1) | 6 (5.6) | 141 (7.0) | 0.687 |
Alcohol | 366 (19.3) | 31 (29.0) | 397 (19.8) | 0.021* |
Others | 202 (10.7) | 16 (14.9) | 218 (11.0) | 0.853 |
Laboratory values | ||||
Hemoglobin (g/dl) | 10.3 (8.8, 12.2) | 9.0 (8.0, 10.2) | 10.3 (8.7, 12.2) | < 0.001† |
WBC (x103/μl) | 3.6 (2.4, 5.2) | 7.1 (4.2, 11.8) | 3.7 (2.5, 5.5) | < 0.001† |
Natrium (mmol/L) | 138.0 (135.0, 141.0) | 137.0 (134.0, 141.5) | 138.0 (135.0, 141.0) | 0.954 |
CRP (mg/dl) | 0.4 (0.1, 1.3) | 1.7 (0.7, 3.6) | 0.4 (0.1, 1.4) | < 0.001† |
INR | 1.4 (1.2, 1.8) | 2.1 (1.5, 2.6) | 1.5 (1.2, 1.9) | < 0.001† |
Total bilirubin (mg/dl) | 2.4 (1.2, 9.2) | 15.4 (4.8, 30.2) | 2.6 (1.3, 11.1) | < 0.001† |
Creatinine (mg/dl) | 0.8 (0.6, 1.0) | 1.2 (0.7, 2.1) | 0.8 (0.7, 1.0) | < 0.001† |
Lactate (mmol/L) | 1.8 (1.4, 2.3) | 5.5 (4.6, 7.0) | 1.9 (1.4, 2.4) | < 0.001† |
Values are presented as median (1Q, 3Q) or number (%). BMI: body mass index, OLT: orthotopic liver transplantation, CAD: coronary artery disease, CVA: cerebrovascular accident, MELD: model for end-stage liver disease, WBC: white blood cell, CRP: C-reactive protein, INR: international normalized ratio.
Table 2.
Variable | Lactate ≤ 4 mmol/L (n = 1,895) | Lactate > 4 mmol/L (n = 107) | Total (n = 2,002) | P value |
---|---|---|---|---|
CLIF_liver | 413 (21.8) | 67 (62.6) | 480 (24.0) | < 0.001† |
CLIF_respiratory | 162 (8.6) | 55 (51.4) | 217 (10.8) | < 0.001† |
CLIF_kidney | 138 (7.3) | 28 (26.2) | 166 (8.3) | < 0.001† |
CLIF_coagulation | 157 (8.3) | 29 (27.1) | 186 (9.3) | < 0.001† |
CLIF_circulatory | 77 (4.1) | 36 (33.6) | 113 (5.6) | < 0.001† |
CLIF_brain | 41 (2.2) | 11 (10.3) | 52 (2.6) | < 0.001† |