Abstract
Notes
AUTHOR CONTRIBUTIONS
Conceptualization: DHK, SWK, SL. Data curation: BAT, DHK, GK. Formal analysis: BAT, DHK, GK. Funding acquisition: DHK, SL. Methodology: BAT, DHK, GK. Project administration: DHK, SWK, SL. Visualization: BAT, DHK, GK. Writing–original draft: BAT, DHK. Writing–review & editing: all authors.
ACKNOWLEDGMENTS
REFERENCES











































































































Fig. 5.
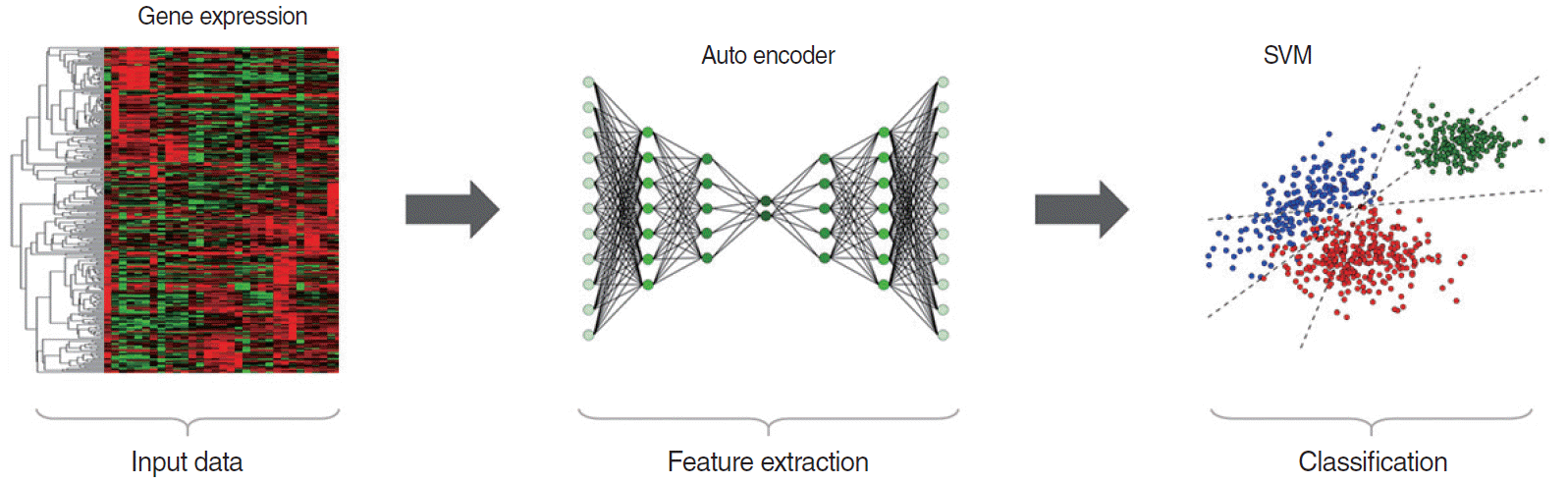
Fig. 6.
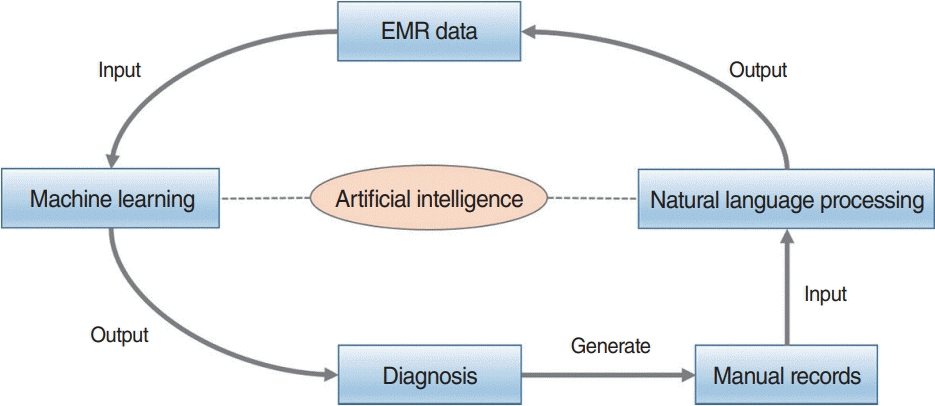
Table 1.
Study | Analysis modality | Objective | AI technique | Validation method | No. of samples in the training dataset | No. of samples in the testing dataset |
Best result |
|
---|---|---|---|---|---|---|---|---|
Accuracy (%)/AUC | Sensitivity (%)/specificity (%) | |||||||
[20] | CT | Anterior ethmoidal artery anatomy | CNN: Inception-V3 | Hold-out | 675 Images from 388 patients | 197 Images | 82.7/0.86 | - |
[21] | CT | Osteomeatal complex occlusion | CNN: Inception-V3 | - | 1.28 Million images from 239 patients | - | 85.0/0.87 | - |
[22] | CT | Chronic otitis media diagnosis | CNN: Inception-V3 | Hold-out | 975 Images | 172 Images | -/0.92 | 83.3/91.4 |
[23] | DECT | HNSCC lymph nodes | RF, GBM | Hold-out | Training and testing set are randomly chosen with a ratio 70:30 from a total of 412 lymph nodes from 50 patients. | 90.0/0.96 | 89.0/91.0 | |
[24] | microCT | Intratemporal facial nerve anatomy | PCA+SSM | - | 40 Cadaveric specimens from 21 donors | - | - | - |
[25] | CT | Extranodal extension of HNSCC | CNN | Hold out | 2,875 Lymph nodes | 200 Lymph nodes | 83.1/0.84 | 71.0/85.0 |
[26] | CT | Prediction of overall survival of head and neck cancer | NN, DT, boosting, Bayesian, bagging, RF, MARS, SVM, k-NN, GLM, PLSR | 10-CV | 101 Head and neck cancer patients, 440 radiomic features | -/0.67 | - | |
[27] | DECT | Benign parotid tumors classification | RF | Hold-out | 882 Images from 42 patients | Two-thirds of the samples | 92.0/0.97 | 86.0/100 |
[28] | fMRI | Predicting the language outcomes following cochlear implantation | SVM | LOOCV | 22 Training samples, including 15 labeled samples and 7 unlabeled samples | 81.3/0.97 | 77.8/85.7 | |
[29] | fMRI | Auditory perception | SVM | 10-CV | 42 Images from 6 participants | 47.0/- | - | |
[30] | MRI | Relationship between tinnitus and thicknesses of internal auditory canal and nerves | ELM | Repeated hold-out | 46 Images from 23 healthy subjects and 23 patients. Test was repeated 10 times for three training ratios, i.e., 50%, 60%, and 70%. | 94.0/- | - | |
[31] | MRI | Prediction of treatment outcomes of sinonasal squamous cell carcinomas | SVM | 9-CV | 36 Lesions from 36 patients | 92.0/- | 100/82.0 | |
[32] | Neuroimaging biomarkers | Tinnitus | SVM | 5-CV | 102 Images from 46 patients and 56 healthy subjects | 80.0/0.86 | - | |
[33] | MRI | Differentiate sinonasal squamous cell carcinoma from inverted papilloma | SVM | LOOCV | 22 Patients with inverted papilloma and 24 patients with SCC | 89.1/- | 91.7/86.4 | |
[34] | MRI | Speech improvement for CI candidates | SVM | LOOCV | 37 Images from 37 children with hearing loss and 40 images from 40 children with normal hearing | 84.0/0.84 | 80.0/88.0 | |
[35] | Endoscopic images | Laryngeal soft tissue | Weighted voting (UNet+ErfNet) | Hold-out | 200 Images | 100 Images | 84.7/- | - |
[36] | Laryngoscope images | laryngeal neoplasms | CNN | Hold-out | 14,340 Images from 5,250 patients | 5,093 Images from 2,271 patients | 96.24/- | 92.8/98.9 |
[37] | Laryngoscope images | Laryngeal cancer | CNN | Hold-out | 13,721 Images | 1,176 Images | 86.7/0.92 | 73.1/92.2 |
[38] | Laryngoscope images | Oropharyngeal cariconoma | Naive Bayes | Hold-out | 4 Patients with oropharyngeal cariconoma and 1 healthy subject | 16 Patients with oropharyngeal cariconoma and 9 healthy subjects | 65.9/- | 66.8/64.9 |
[39] | Otoscopic images | Otologic diseases | CNN | Hold-out | 734 Images; 80% of the images were used for the training and 20% were used for validation. | 84.4/- | - | |
[40] | Otoscopic images | Otitis media | MJSR | Hold-out | 1,230 Images; 80% of and 20% were used the images were used for the training for validation. | 91.41/- | 89.48/93.33 | |
[41] | Otoscopic images | Otoscopic diagnosis | AutoML | Hold-out | 1,277 Images | 89 Images | 88.7/- | 86.1/- |
[42] | Digitized images | H&E-stained tissue of oral cavity squamous cell carcinoma | LDA, QDA, RF, SVM | Hold-out | 50 Images | 65 Images | 88.0/0.87 | 78.0/93.0 |
[43] | PESI-MS | Intraoperative specimens of HNSCC | LR | LOOCV | 114 Non-cancerous specimens and 141 cancerous specimens | 95.35/- | - | |
[44] | Biopsy specimen | Frozen section of oral cavity cancer | SVM | LOOCV | 176 Specimen pairs from 27 subjects | -/0.94 | 100/88.78 | |
[45] | HSI | Head and neck cancer classification | CNN | LOOCV | 88 Samples from 50 patients | 80.0/- | 81.0/78.0 | |
[46] | HSI | Head and neck cancer classification | CNN | LOOCV | 12 Tumor-bearing samples for 12 mice | 91.36/- | 86.05/93.36 | |
[47] | HSI | Oral cancer | SVM, LDA, QDA, RF, RUSBoost | 10-CV | 10 Images from 10 mice | 79.0/0.86 | 79.0/79.0 | |
[48] | HSI | Head and neck cancer classification | LDA, QDA, ensemble LDA, SVM, RF | Repeated hold-out | 20 Specimens from 20 patients | 16 Specimens from 16 patients | 94.0/0.97 | 95.0/90.0 |
[49] | HSI | Tissue surface shape reconstruction | SSRNet | 5-CV | 200 SL images | 96.81/- | 92.5/- | |
[50] | HSI | Tumor margin of HNSCC | CNN | 5-CV | 395 Surgical specimens | 98.0/0.99 | - | |
[51] | HSI | Tumor margin of HNSCC | LDA | 10-CV | 16 Surgical specimens | 90.0/- | 89.0/91.0 | |
[52] | HSI | Optical biopsy of head and neck cancer | CNN | LOOCV | 21 Surgical gross-tissue specimens | 81.0/0.82 | 81.0/80.0 | |
[53] | SRS | Frozen section of laryngeal squamous cell carcinoma | CNN | 5-CV | 18,750 Images from 45 patients | 100/- | - | |
[54] | HSI | Cancer margins of ex-vivo human surgical specimens | CNN | Hold-out | 11 Surgical specimens | 9 Surgical specimens | 81.0/0.86 | 84.0/77.0 |
[55] | USG | Genetic risk stratification of thyroid nodules | AutoML | Hold-out | 556 Images from 21 patients | 127 Images | 77.4/- | 45.0/97.0 |
[56] | CT | Concha bullosa on coronal sinus classification | CNN: Inception-V3 | Hold-out | 347 Images (163 concha bullosa images and 184 normal images) | 100 Images (50 concha bullosa images and 50 normal images) | 81.0/0.93 | - |
[57] | Panoramic radiography | Maxillary sinusitis diagnosis | AlexNet CNN | Hold-out | 400 Healthy images and 400 inflamed maxillary sinuses images | 60 Healthy and 60 inflamed maxillary sinuses images | 87.5/0.875 | 86.7/88.3 |
AI, artificial intelligence; AUC, area under the receiver operating characteristic curve; CT, computed tomography; CNN, convolutional neural network; DECT, dual-energy computed tomography; HNSCC, head and neck squamous cell carcinoma; RF, random forest; GBM, gradient boosting machine; PCA, principle component analysis; SSM, statistical shape model; NN, neural network; DT, decision tree; MARS, multi adaptive regression splines; SVM, support vector machine; k-NN, k-nearest neighbor; GLM, generalized linear model; PLSR, partial least squares and principal component regression; CV, cross-validation; fMRI, functional magnetic resonance imaging; LOOCV, leave-one-out cross-validation; ELM, extreme learning machine; CI, cochlear implant; MJSR, multitask joint sparse representation; LDA, linear discriminant analysis; QDA, quadratic discriminant analysis; PESI-MS, probe electrospray ionization mass spectrometry; LR, logistic regression; HSI, hyperspectral imaging; SSRNet, super-spectral-resolution network; SRS, stimulated Raman scattering; USG, ultrasonography.
Table 2.
Study | Analysis modality | Objective | AI technique | Validation method | No. of samples in the training dataset | No. of samples in the testing dataset | Best result |
---|---|---|---|---|---|---|---|
[58] | CI | Noise reduction | NC+DDAE | Hold-out | 120 Utterances | 200 Utterances | Accuracy: 99.5% |
[59] | CI | Segregated speech from background noise | DNN | Hold-out | 560×50 Mixtures for each noise type and SNR | 160 Noise segments from original unperturbed noise | Hit ratio: 84%; false alarm: 7% |
[60] | CI | Improved pitch perception | ANN | Hold-out | 1,500 Pitch pairs | 10% of the training material | Accuracy: 95% |
[61] | CI | Predicted speech recognition and QoL outcomes | k-NN, DT | 10-CV | A total of 29 patients, including 48% unilateral CI users and 51% bimodal CI users | Accuracy: 81% | |
[62] | CI | Noise reduction | DDAE | Hold-out | 12,600 Utterances | 900 Noisy utterances | Accuracy: 36.2% |
[63] | CI | Improved speech intelligibility in unknown noisy environments | DNN | Hold-out | 640,000 Mixtures of sentences and noises | - | Accuracy: 90.4% |
[64] | CI | Modeling electrode-to-nerve interface | ANN | Hold-out | 360 Sets of fiber activation patterns per electrode | 40 Sets of fiber activation patterns per electrode | - |
[65] | CI | Provided digital signal processing plug-in for CI | WNN | Hold-out | 120 Consonants and vowels, sampled at 16 kHz; half of data was used as training set and the rest was used as testing set. | SNR: 2.496; MSE: 0.086; LLR: 2.323 | |
[66] | CI | Assessed disyllabic speech test performance in CI | k-NN | - | 60 Patients | - | Accuracy: 90.83% |
[67] | Acoustic signals | Voice disorders detection | CNN | 10-CV | 451 Images from 10 health adults and 70 adults with voice disorders | Accuracy: 90% | |
[68] | Dysphonic symptoms | Voice disorders detection | ANN | Repeated hold-out | 100 Cases of neoplasm, 508 cases of benign phonotraumatic, 153 cases of vocal palsy | Accuracy: 83% | |
[69] | Pathological voice | Voice disorders detection | DNN, SVM, GMM | 5-CV | 60 Normal voice samples and 402 pathological voice samples | Accuracy: 94.26% | |
[70] | Acoustic signal | Hot potato voice detection | SVM | Hold-out | 2,200 Synthetic voice samples | 12 HPV samples from real patients | Accuracy: 88.3% |
[71] | SEMG signals | Voice restoration for laryngectomy patients | XGBoost | Hold-out | 75 Utterances using 7 SEMG sensors | - | Accuracy: 86.4% |
AI, artificial intelligence; CI, cochlear implant; NC, noise classifier; DDAE, deep denoising autoencoder; DNN, deep neural network; SNR, signal-to-noise ratio; ANN, artificial neural network; QoL, quality of life; k-NN, k-nearest neighbors; DT, decision tree; CV, cross-validation; WNN, wavelet neural network; MSE, mean square error; LLR, log-likelihood ratio; CNN, convolutional neural network; GMM, Gaussian mixture model; SVM, support vector machine; HPV, human papillomavirus; SEMG, surface electromyographic.
Table 3.
Study | Analysis modality | Objective | AI technique | Validation method | No. of samples in the training dataset | No. of samples in the testing dataset | Best result |
---|---|---|---|---|---|---|---|
[73] | EEG signal of PSG | Sleep stage scoring | CNN | 5-CV | 294 Sleep studies; 122 composed the training set, 20 composed the validation set, and 152 were used in the testing set. | Accuracy: 81.81%; F1 score: 81.50%; Cohen’s Kappa: 72.76% | |
[72] | EEG, EMG, EOG signals of PSG | Sleep stage scoring | CNN | Hold-out | 42,560 Hours of PSG data from 5,213 patients | 580 PSGs | Accuracy: 86%; F1 score: 81.0%; Cohen’s Kappa: 82.0% |
[74] | Sleep heart rate variability in PSG | Long-term cardiovascular outcome prediction | XGBoost | 5-CV | 1,252 Patients with cardio vascular disease and 859 patients with non-cardio vascular disease | Accuracy: 75.3% | |
[87] | Sleep breathing sound using an air-conduction microphone | AHI prediction | Gaussian process, SVM, RF, LiR | 10-CV | 116 Patients with OSA | CC: 0.83; LMAE: 9.54 events/hr; RMSE: 13.72 events/hr | |
[88] | Gene signature | Thyroid cancer lymph node metastasis and recurrence rediction | LDA | 6-CV | 363 Samples | 72 Samples | AUC: 0.86; sensitivity: 86%; specificity: 62%; PPV: 93%; NPV: 42% |
[89] | Gene expression profile | Response prediction to chemotherapy in patient with HNSCC | SVM | LOOCV | 16 TPF-sensitive patients and 13 non-TPF-sensitive patients | Sensitivity: 88.3%; specificity: 88.9% | |
[90] | Mucus cytokines | SNOT-22 scores prediction of CRS patients | RF, LiR | - | 147 Patients with 65 patients with postoperative follow-up | R2: 0.398 | |
[91] | Cellular cartography | Single-cell resolution mapping of the organ of Corti | Gentle boost, RF, CNN | Hold-out | 20,416 Samples | 19,594 Samples | Recall: 99.3%; precision: 99.3%; F1: 93.3% |
[92] | RNA sequencing, miRNA sequencing, methylation data | HNSCC progress prediction | Autoencoder and SVM | 2×5-CV | 360 Samples from TCGA | C-index: 0.73; Brier score: 0.22 | |
[93] | DNA repair defect | HNSCC progress prediction | CART | 10×5-CV | 180 HPV-negative HNSCC patients | AUC: 1.0 | |
[94] | PESI-MS | Identified TGF-β signaling in HNSCC | LDA | LOOCV | A total of 240 and 90 mass spectra from TGF-β-unstimulated and stimulated HNSCC cells, respectively | Accuracy: 98.79% | |
[95] | Next generation sequencing of RNA | Classified the risk of malignancy in cytologically indeterminate thyroid nodules | Ensemble of elastic net GLM and SVM | 40×5-CV | A total of 10,196 genes, among which are 1,115 core genes | Sensitivity: 91%; specificity: 68% | |
[96] | Gene expression profile | HPV-positive oropharyngeal squamous cell carcinoma detection | LR | 500-CV | 146 Genes from patients with node-negative disease and node-positive disease | AUC: 0.93 | |
[97] | miRNA expression profile | Sensorineural hearing loss prediction | DF, DJ, LR, NN | LOOCV | 16 Patients were included. | Accuracy: 100% |
AI, artificial intelligence; EEG, electroencephalogram; PSG, polysomnography; CNN, convolutional neural network; CV, cross-validation; EMG, electromyography; EOG, electrooculogram; AHI, apnea-hypopnea index; SVM, support vector machine; RF, random forest; LiR, linear regression; OSA, obstructive sleep apnea; CC, correlation coefficient; LMAE, least mean absolute error; RMSE, root mean squared error; LDA, linear discriminant analysis; AUC, area under the receiver operating characteristic curve; PPV, positive predictive value; NPV, negative predictive value; HNSCC, head and neck squamous cell carcinoma; LOOCV, leave-one-out cross validation; TPF, docetaxel, cisplatin, and 5-fluorouracil; SNOT-22, 22-item sinonasal outcome test; CRS, chronic rhinosinusitis; miRNA, microRNA; TCGA, the cancer genome atlas; CART, classification and regression trees; HPV, human papillomavirus; PESI-MS, probe electrospray ionization mass spectrometry; TGF-β, transforming growth factor beta; GLM, generalized linear model; LR, logistic regression; DF, decision forest; DJ, decision jungle; NN, neural network.
Table 4.
Study | Analysis modality | Objective | AI technique | Validation method | No. of samples in the training dataset | No. of samples in the testing dataset | Best result |
---|---|---|---|---|---|---|---|
[98] | Hearing aids | Hearing gain prediction | CRDN | Hold-out | 2,182 Patients that were diagnosed with hearing loss; the percentages of randomly sampled training, validation, and test sets were 40%, 30%, and 30%, respectively. | MAPE: 9.2% | |
[99] | Hearing aids | Predicted CI outcomes | RF | LOOCV | 121 Postlingually deaf adults with CI | MAE: 6.1; Pearson’s correlation coefficient: 0.96 | |
[100] | Clinical data | SSHL prediction | DBN, LR, SVM, MLP | 4-CV | 1,220 Unilateral SSHL patients | Accuracy: 77.58%; AUC: 0.84 | |
[101] | Clinical data including demographics and risk factors | Determined the risk of head and neck cancer | LR | Hold-out | 1,005 Patients, containing 932 patients with no cancer outcome and 73 patients with cancer outcome | 235 Patients, containing 212 patients with no cancer outcome and 23 patients with cancer outcome | AUC: 0.79 |
[102] | Clinical data including symptom | Peritonsillar abscess diagnosis prediction | NN | Hold-out | 641 Patients | 275 Patients | Accuracy: 72.3%; sensitivity: 6.0%; specificity: 50% |
[103] | Vestibular test batteries | Vestibular function assessment | DT, RF, LR, AdaBoost, SVM | Hold-out | 5,774 Individuals | 100 Individuals | Accuracy: 93.4% |
[104] | Speakers and microphones within existing smartphones | Middle ear fluid detection | LR | LOOCV | 98 Patient ears | AUC: 0.9; sensitivity: 84.6%; specificity: 81.9% | |
[105] | Cancer data survival | 5-Year survival patients with oral cavity squamous cell carcinoma | DF, DJ, LR, NN | Hold-out | 26,452 Patients | 6,613 Patients | AUC: 0.8; accuracy: 71%; precision: 71%; recall: 68% |
[106] | Histological data | Occult lymph node metastases identification in clinically oral cavity squamous cell | RF, SVM, LR, C5.0 | Hold-out | 56 Patients | 112 Patients | AUC: 0.89; accuracy: 88.0%; NPV: >95% |
[107] | Clinicopathologic data | Head and neck free tissue transfer surgical complications prediction | GBDT | Hold-out | 291 Patients | 73 Patients | Specificity: 62.0%; sensitivity: 60.0%; F1: 60.0% |
[108] | Clinicopathologic data | Delayed adjuvant radiation prediction | RF | Hold-out | 61,258 Patients | 15,315 Patients | Accuracy: 64.4%; precision: 58.5% |
[109] | Clinicopathologic data | Occult nodal metastasis prediction in oral cavity squamous cell carcinoma | LR, RF, SVM, GBM | Hold-out | 1,570 Patients | 391 Patients | AUC: 0.71; sensitivity: 75.3%; specificity: 49.2% |
[110] | Dataset of the center of pressure sway during foam posturography | Peripheral vestibular dysfunction prediction | GBDT, bagging, LR | CV | 75 Patients with vestibular dysfunction and 163 healthy controls | AUC: 0.9; recall: 0.84 | |
[111] | TEOAE signals | Meniere’s disease hearing outcome prediction | SVM | 5-CV | 30 Unilateral patients | Accuracy: 82.7% | |
[112] | Semantic and syntactic patterns in clinical documentation | Vestibular diagnoses | NLP+Naïve Bayes | 10-CV | 866 Physician-generated histories from vestibular patients | Sensitivity: 93.4%; specificity: 98.2%; AUC: 1.0 | |
[113] | Endoscopic imaging | Nasal polyps diagnosis | ResNet50, Xception, and Inception V3 | Hold-out | 23,048 Patches (167 patients) as training set, 1,577 patches (12 patients) as internal validation set, and 1,964 patches (16 patients) as external test set | Inception V3: AUC: 0.974 | |
[114] | Intradermal skin tests | Allergic rhinitis diagnosis | Associative classifier | 10-CV | 872 Patients with allergic symptoms | Accuracy: 88.31% | |
[115] | Clinical data | Identified phenotype and mucosal eosinophilia endotype subgroups of patients with medical refractory CRS | Cluster analysis | - | 46 Patients with CRS without nasal polyps and 67 patients with nasal polyps | - | |
[116] | Clinical data | Prognostic information of patient with CRS | Discriminant analysis | - | 690 Patients | - | |
[117] | Clinical data | Identified phenotypic subgroups of CRS patients | Discriminant analysis | - | 382 Patients | - | |
[118] | Clinical data | Characterization of distinguishing clinical features between subgroups of patients with CRS | Cluster analysis | - | 97 Surgical patients with CRS | - | |
[119] | Clinical data | Identified features of CRS without nasal polyposis | Cluster analysis | - | 145 Patients of CRS without nasal polyposis | - | |
[120] | Clinical data | Identified inflammatory endotypes of CRS | Cluster analysis | - | 682 Cases (65% with CRS without nasal polyps) | - | |
[121] | Clinical data | Identified features of CRS with nasal polyps | Cluster analysis | - | 375 Patients | - |
AI, artificial intelligence; CRDN, cascade recurring deep network; MAPE, mean absolute percentage error; RF, random forest; LOOCV, leave-one-out cross validation; CI, cochlear implant; MAE, mean absolute error; SSHL, sudden sensorineural hearing loss; DBN, deep belief network; LR, logistic regression; SVM, support vector machine; MLP, multilayer perceptron; CV, cross-validation; AUC, area under the receiver operating characteristic curve; NN, neural network; DT, decision tree; DF, decision forest; DJ, decision jungle; NPV, negative predictive value; GBDT, gradient boosted decision trees; GBM, gradient boosting machine; TEOAE, transient-evoked otoacoustic emission; NLP, natural language processing; CRS, chronic rhinosinusitis.