Abstract
Purpose
With the emergence of next-generation sequencing (NGS) technology, profiling a wide range of genomic alterations has become a possibility resulting in improved implementation of targeted cancer therapy. In Asian populations, the prevalence and spectrum of clinically actionable genetic alterations has not yet been determined because of a lack of studies examining high-throughput cancer genomic data.
Materials and Methods
To address this issue, 1,071 tumor samples were collected from five major cancer institutes in Korea and analyzed using targeted NGS at a centralized laboratory. Samples were either fresh frozen or formalin-fixed, paraffin embedded (FFPE) and the quality and yield of extracted genomic DNA was assessed. In order to estimate the effect of sample condition on the quality of sequencing results, tissue preparation method, specimen type (resected or biopsied) and tissue storage time were compared.
Results
We detected 7,360 non-synonymous point mutations, 1,164 small insertions and deletions, 3,173 copy number alterations, and 462 structural variants. Fifty-four percent of tumors had one or more clinically relevant genetic mutation. The distribution of actionable variants was variable among different genes. Fresh frozen tissues, surgically resected specimens, and recently obtained specimens generated superior sequencing results over FFPE tissues, biopsied specimens, and tissues with long storage duration.
During the past decade, the emergence of next-generation sequencing (NGS) technologies has made comprehensive and highly sensitive analysis of cancer genomes feasible [1]. In addition to providing important insights into the molecular events involved in tumorigenesis, these technological advances offer great benefits to patients by improving diagnostic accuracy, identifying prognostic biomarkers and increasing utilization of targeted therapies [2,3]. Many NGSbased projects, including the Cancer Genome Atlas (TCGA) studies, have been able to identify a wide range of clinically relevant genomic alterations within multiple cancer types [4]. These molecular profiling efforts have been essential to the development of targeted therapies leading to more personalized cancer management. In addition, many clinical trials are now utilizing deep sequencing to randomize, cancer patients to new genomically-matched treatments [2,5]. While comprehensive molecular profiling of tumors has become increasingly important, there is a limited amount of highthroughput genomic data in East-Asian populations. Consequently, it has been difficult to estimate the frequency of clinically actionable mutations in Korea [2,6].
The National Health Insurance Service of Korea now includes NGS testing in its insurance coverage (S1 Table). While NGS tests have become more affordable, there are still challenges involved in bringing these tests into routine clinical practice in Korea. First, significant investments are required to build and maintain the computational infrastructure that enables genomic testing and research [7]. Second, bioinformatics specialists and server engineers are required to run the sophisticated software and manage scientific computing. These resource-intensive prerequisites surpass the capacity of smallest laboratories and impose significant challenges even for large cancer centers [8]. In light of such issues, we propose a new centralized laboratory model that could ensure accessibility and sustainability of NGS tests for providers. Local cancer centers would send their tumor tissue samples to a central sequencing and bioinformatics hub and have them analyzed within a reasonable turnaround time at a fraction of the cost (Fig. 1) [8].
In this study, we collected over 1,071 tumor samples, (598 lung cancers, 197 stomach cancers, 107 colorectal cancers [CRCs], 69 breast cancers, and 100 other cancer types including ovarian, head and neck, thymic, melanoma, and esophageal), from five major cancer centers in Korea and used a high-depth targeted sequencing panel to sequence genomic DNA samples. We comprehensively profiled genetic alterations in patients with advanced cancer and evaluated the clinical utility of NGS technology. Here, we present the genomic landscape of common cancers in Korea and defines potential driver mutations that may affect clinical decision making. Furthermore, we share our experience serving as a centralized laboratory for five major cancer centers in Korea. A thorough analysis of specimen types with respect to DNA yield and DNA fragment size helped optimize our NGS workflow. The ultimate goal is to advance the implementation and adoption of NGS in Korea as a critical component for improving patient outcomes through the practice of precision medicine.
We collected 1,071 tumor samples from 993 patients from five major Korean medical institutes. Specimens were prepared from fresh frozen (FF) tissue (n=323) or formalin-fixed and paraffin embedded (FFPE) tissue (n=748). Following microscopic examination of hematoxylin and eosin-stained slides, macrodissection was performed to enrich for tumor cells as needed. For DNA extraction, 10 tissue slides, 5 μm, were required for small biopsy samples whereas 2-5 slides, 5 μm, were needed for resected specimens. Genomic DNA from FFPE tissues was extracted using the Qiagen DNA FFPE Tissue kit (Qiagen, Valencia, CA), and DNA from FF tissues was extracted using the QIAamp DNA mini kit (Qiagen). DNA yield was evaluated using a Nanodrop 8000 UV-Vis spectrometer (NanoDrop Technologies Inc., Wilmington, DE) and Qubit 3.0 Fluorometer (Thermo Fisher Scientific, Waltham, MA). DNA size was examined using a 2200 TapeStation Instrument (Aglient Technologies, Santa Clara, CA). Specimens with a DNA yield over 100 ng and a median DNA fragment size of at least 350 bp were selected for targeted sequencing.
Targeted sequencing was performed using the Cancer-SCAN [9] panel which includes the whole exomes of 375 cancer-related genes and the intronic regions of 23 genes. Genomic DNA was sheared using a Covaris S220 (Covaris, Woburn, MA). Target capture was performed using the Sure-Select XT Reagent Kit, HSQ (Agilent Technologies) and a paired-end sequencing library was constructed with a barcode. Sequencing was performed on a HiSeq 2500 with 100-bp reads (Illumina, San Diego, CA). One hundred samples were able to be sequenced in a single experiment when using high throughput mode and 17 samples were able to be sequenced when using fast mode.
The paired-end reads were aligned to the human reference genome (hg19) using BWA-MEM v0.7.5. Samtools v0.1.18, GATK v3.1-1, and Picard v1.93 were used for bam file handling, local realignment, and removal of duplicate reads, respectively. Samples with a mean target coverage of less than 200× were excluded from further analysis. Single nucleotide variants (SNVs) with a variant allele fraction greater than 1% were detected using MuTect v1.1.4, and Lowfreq v0.6.1. Sequencing errors were filtered out by an in-house algorithm using data extracted from each bam file. Insertions and deletions (indels) that were less than 30 bp in size were detected using Pindel v0.2.5a4. Possible germline polymorphisms were also filtered out if the allele frequency was more than 0.1% in any of the normal population databases including the: 1000 Genomes Project database, The Exome Aggregation Consortium (ExAC) database, the National Heart, Lung and Blood Exome Sequencing Project (ESP) database, the Korean Reference Genome Database, or the Korean Variant Archive (KOVA) [10]. Structural variants (SVs) and large indels (> 30 bp) were detected using an in-house SV caller. Copy number alterations (CNAs) of each gene were also detected using an in-house copy number caller with copy numbers greater than 6 being marked as amplifications and a copy numbers less than 0.7 designated as deletions.
We defined actionable alterations as SNVs, indels, CNAs, or SVs with the potential to affect clinical decisions or impact the way patients are enrolled in clinical trials. Detected DNA alterations were annotated by an in-house database created for CancerSCAN [9]. SNVs were matched with the database for amino acid level change, indels were matched with the database at the exon level change, and CNAs and SVs were matched with changes at the gene level.
Statistical analysis was conducted using the R v3.3.2. A chisquare test was used to determine the association between two categorical variables, a T-test was used to examine the association between one categorical variable and one continuous variable, and a Pearson’s correlation test was used to evaluate the association between two continuous variables. Firth’s bias-reduced logistic regression was determined using the logistf R package v1.22 to assess the effect of each variable on the success of sequencing. Following this, an odds ratio (OR) was calculated for each variable. Significantly mutated genes were identified using MutSigCV v1.41. Genes with a significant CNA were identified using GISTIC v2.0.23. A p-value less than 0.05 was considered significant and genes with a false discovery rate (q-value) less than 0.1 were considered to be significantly mutated genes—either significantly amplified or significantly deleted.
The study was approved by the Institutional Review Board of Samsung Medical Center, Seoul, Korea (SMC-2016-03-094), Severance Hospital, Seoul, Korea (4-2016-0135), Seoul St. Mary’s Hospital, Seoul, Korea (2016939), Asan Medical Center, Seoul, Korea (S2016-0498-0007), and Seoul National University Hospital, Seoul Korea (H-1606-076-771). Some patients provided written informed consent for this study. Some patients provided written informed consent for other study and agreed secondary uses of data. Consent was waived for the other patients.
A total of 1,071 cancer samples of various types were collected from five different institutes: 598 lung cancers, 197 stomach cancers, 107 CRCs, 69 breast cancers, and 100 other cancer types including ovarian, head and neck, thymic, melanoma, and esophageal (S2 Table). Of the 598 lung cancer samples, 525 were non-small cell lung cancer (NSCLC) and 73 were small cell lung cancer (SCLC). NSCLC samples made up a high proportion (49%) of the samples in this study. Of the study samples, 493 were obtained by biopsy, 499 were obtained by surgical resection, and 79 were obtained from other methods. A total of 323 samples were FF tissue, and 748 samples were FFPE tissue. The median storage duration from tissue sampling to DNA extraction was 313 days (range, 1 to 6,018 days). In total, 224 samples (21%) were excluded due to low quality. This included 153 (14%) samples with a DNA yield less than or near 100 ng and 12 samples (1%) with a median DNA fragment size less than 350 bp that were excluded prior to sequencing (S3 Table). In addition, 59 samples (6%) with a mean target coverage less than 200× were excluded from the analysis. Some samples were obtained from different (primary or metastatic) sites of the same patient. The result from one sample is used per patients when analyzing mutation landscape.
Sequencing results from 803 patient specimens were included in the analysis. NGS detected 7,360 non-synonymous SNVs, 1,164 indels, 3,173 CNAs, and 462 SVs. Tumor mutational burden was calculated for each tumor type cohort. The median number of SNVs per megabase DNA within NSCLC, SCLC, stomach cancer, CRC, and breast cancer were 10.7, 14.2, 11.5, 12.3, and 11.1, respectively (S4 Fig.). The median number of small indels was 1 or 2 per megabase of DNA within each cancer types. More CNAs were present in SCLC and breast cancer than the other cancer types. For tested genes, the median number of genes with an altered copy number was 1.4% for SCLC and 1.1% for breast cancer.
To estimate the effect of sample condition on the test quality, statistical analyses were performed for the tissue preparation method, specimen type (resected or biopsied sample), cancer type, and tissue storage time. As sufficient DNA yield and fragment size were the primary prerequisites for the analysis, the association between sample condition and DNA yield and that between sample condition and DNA size were examined. DNA yield was found to be affected by the tissue preparation method, specimen type, and cancer type (S5 Table). Median DNA fragment size was found to be affected by the tissue preparation method and cancer type (S6 Table).
It is known that FFPE tissue experiences DNA degradation during storage [11]. Thus, we further examined the effect of sample condition on DNA fragment size (Fig. 2). When samples acquired from resection were treated with FFPE, DNA size was significantly shorter than FF samples (p < 0.001). Compared to the formalin fixation procedure for the biopsied samples, the formalin fixation procedure after resection was much more complex and time-consuming. For FF samples, no difference in DNA size was observed between biopsied samples and samples acquired from resection (p=0.141). Storage duration was correlated with DNA fragment size for FFPE tissues (p < 0.001) but not FF tissues (p=0.093).
After sequencing tumor samples and removing duplicate reads, the mean target coverage was 842× and was very important for mutation detection sensitivity. The mean target coverage was correlated with the amount of DNA input and the median DNA fragment size. When possible, 300 ng of DNA was used for sequencing and when the DNA amount was not sufficient, less than 300 ng of DNA was used. Mean target coverage was reduced when less than 300 ng of DNA (p < 0.001) or shorter median DNA fragment size (< 350 bp, p < 0.001) was used (S7 Table, S8 and S9 Figs.).
Tissue quality was found to vary among the five institutes (Table 1, S10 Fig.). Because different types of tissues were collected (e.g., FF vs. FFPE; biopsy vs. resection) among the institutes, it was difficult to do a direct comparison. However, when the percentages of samples that passed our inclusion criteria were compared, the test quality was remarkably worse in tissues from Institute B. Firth’s bias-reduced logistic regression model was carried out using sample conditions and overall test failure status to examine the effect of each variable (Fig. 3). The overall test failure rate was higher when FFPE tissue (OR, 0.19; p < 0.001), biopsied sample (OR, 0.17; p < 0.001), or samples with a long storage time (OR, 0.86/yr; p=0.005) were used (S11 Table). Although DNA length was longer in biopsied samples, the higher test failure rate observed was likely due to a lower DNA yield. The test failure rate was not significantly different among different tumor types, but a significant difference in the test failure rate of samples from different institutes was observed (institute C vs. E; OR, 0.07; p=0.014).
Quality of test influenced the detection of variants if the mean coverage is extremely low. The number of detected SNVs decreased when the mean target coverage was lower than 100× (p < 0.001). However, the number of detected CNAs increased when the mean target coverage was lower than 100× (p < 0.001) (S12 Fig.). The mean coverage did not affect the detection rate of clinically important variant, EGFR T790M.
We investigated the significantly mutated genes per tumor type and compared them to TCGA data. In our NSCLC samples (n=401), significantly mutated genes were EGFR (q < 0.001), PRKDC (q < 0.001), TP53 (q < 0.001), FGF23 (q < 0.001), KRAS (q < 0.001), EPHB6 (q=0.002), and RB1 (q=0.003) (S13 Fig.). Except for PRKDC, FGF23, and EPHB6 these genes were significantly mutated in TCGA data [4,12]. Other genes that were significantly mutated in NSCLC in the TCGA data (ARID1A, KEAP1, CDKN2A, PIK3CA, NKX2-1, SMAD4, and PTEN) were also frequently mutated in this study. Significantly amplified regions included the 7p11.2 region which contains the EGFR and HGF genes (q < 0.001), the 14q13.3 region which contains the FOXA1, NFKBIA, and NKX2-1 gene (q < 0.001), the 12q15 region which contains the BTG1 and MDM2 genes (q < 0.001), the 11q13.3 region which contains the CCND1, FGF4, MEN1, and FGF19 genes (q < 0.001), the 17q12 region which contains the ERBB2, RARA, CDK12, CCNE1, CEBPA, and MEF2B genes (q < 0.001), the 20q13.2 region which contains the AURKA, ZNF217 and NCOA3 genes (q < 0.001), and the 12q14.1 region which contains the CDK4, ERBB3, and MDM2 genes (q=0.004), and 3q27.1 region which contains the PIK3CA, SOX2, MAP3K13, and KLHL6 genes (q=0.035), and the 8q21.3 region which contains RUNX1T1 and MYC genes (q=0.092). Significantly deleted regions were the 9p21.3 region which contains the CDKN2A and CDKN2B genes (q < 0.001) (S14 Fig.).
In our SCLC samples (n=60), significantly mutated genes were TP53 (q < 0.001), RB1 (q < 0.001), FGF23 (q=0.005), and FANCM (q=0.060) (S15 Fig.). Prior studies have shown detection of TP53 and RB1 inactivation in nearly all SCLC samples when whole genome sequencing was carried out [13]. The mutation frequency of these genes observed in this study, 88% for TP53 and 75% for RB1, was similar to other exome sequencing data [14]. The 4q12 region that contains the KDR, KIT, and PDGFRA genes (q=0.024) and the 19q12 region that contains the CCNE1, CEBPA, and MEF2B genes (q=0.026) were also significantly amplified in our SCLC samples (q=0.023) (S16 Fig.).
In the stomach cancer samples (n=119), significantly mutated genes were TP53 (q < 0.001), CDH1 (q < 0.001), and ARID1A (q=0.041) (S17 Fig.). Other frequently altered genes that were significantly mutated in stomach cancer in the TCGA data [15] were KRAS, SMAD4, ERBB2, PIK3CA, CTNNB1 and APC. Significantly amplified regions in our samples were the 19q12 region which contains the CCNE1, CEBPA, and MEF2B genes (q < 0.001), the 10q26.13 region which contains the FGFR2 gene (q < 0.001), the 20q13.2 region which contains the AURKA, ZNF217 and NCOA3 genes (q < 0.001), the 7p11.2 region which contains the EGFR, HGF, and IKZF1 genes (q < 0.001), and the 17q12 containing the ERBB2 and RARA genes (q=0.001). Significantly deleted regions were the 19p13.2 region which contains the SMARCA4 gene (q=0.050) (S18 Fig.).
In the CRC samples (n=23), significantly mutated genes were APC (q < 0.001), KRAS (q < 0.001), TP53 (q < 0.001), SMAD4 (q < 0.001), PIK3CA (q < 0.001), FBXW7 (q < 0.001), and SOX9 (q=0.033). These genes were also significantly mutated in TCGA data [16] (S19 Fig.). The 12p13 region that contains the CCND2, CHD4, FGF6, RAD52 and FGF23 genes was significantly amplified (q=0.013) (S20 Fig.).
In our breast cancer samples (n=25), the genes TP53 (q < 0.001), GATA3 (q < 0.001), and PIK3CA (q=0.014) were found to be significantly mutated (S21 Fig.). Other frequently altered genes that were significantly mutated in breast cancer in the TCGA data were CDH1, ERBB2, NF1, and MAP3K1. Significantly amplified regions in our samples were the 17q12 region which contains the ERBB2, RARA, and CDK12 genes (q < 0.001) and the 11q13.3 region which contains the CCND1, MEM1, and FGF19 genes (q=0.007) (S22 Fig.).
The TERT promoter region was included in the sequencing panel used in this study. We found that a TERT promoter mutation was present in 3% of NSCLCs, 5% of stomach cancers, 2% of CRCs, and 2% of breast cancers. Frequently altered sites were G>A substitutions at –124 bp (n=6) and –146 bp (n=3) upstream from the translation start site.
In this study, an actionable genetic alteration was defined as a genomic variation that was a known drug target, regardless of tumor type. Here, 54.2% of the tested samples had one or more actionable genetic alteration. The proportion of samples with actionable genetic variants was higher in NSCLC (68%), CRC (52%), and breast cancer samples (52%) than in stomach cancer (28%), SCLC (13%), and other cancer types (28%) (Fig. 4, S23 Fig.). There were 25 genes with actionable alterations; EGFR, KRAS and PIK3CA were the most frequently altered actionable genes containing 72% of actionable alterations. In NSCLC, 47% of samples had actionable alterations in the EGFR gene, and 27% of samples had actionable alterations in genes other than EGFR. Although actionable alterations of EGFR, KRAS, BRAF, ALK, RICTOR, RET, and ROS1 were mutually exclusive, some actionable alterations of PIK3CA, ERBB2, MDM2, CDK4, FGFR1, NRAS, and CTNNB1 coexisted with other actionable alterations (S24 Fig.). Actionable alterations of EGFR were only present in NSCLC, but actionable alterations of KRAS, PIK3CA, and ERBB2 were present across almost every cancer type in our study.
A 36-year-old never-smoker woman was diagnosed with lung adenocarcinoma and right upper lobectomy with adjuvant chemotherapy was done. No EGFR and KRA Smutation was detected at initial diagnosis. Two years after the surgery, adenocarcinoma recurred at supraclavicular lymph node and the patient received concurrent chemoradiotherapy. Two years later, tumor progressed to involve trachea. Tracheal lesion was biopsied to perform fluorescence in situ hybridization study for ALK, RET, ROS1 translocation. ALK translocation was detected and the patient is treated with crizotinib. Airway lesion and mediastinal lymphadenopathy improved after crizotinib treatment. Eighteen months after the therapy, size of mediastinal and intraabdominal lymph node increased. Mediastinal lymph nodes was biopsied to perform NGS study. ALK G1269A mutation was detected with KIF5B/ALK fusion. The patient received ceritinib which overcomes resistance to ALK G1269A mutation. Mediastinal and intraabdominal lymph nodes disappeared after two months of ceratinib therapy. After 16 months, the patient remains on treatment with no signs of tumor progression.
In this study, we report our experience as a central bioinformatics laboratory determining the frequency of actionable genomic alterations in over 1,000 tumor samples from five different institutes. We found that 54% of our tested samples had one or more actionable genetic alterations. A higher proportion of actionable genetic variants was observed in NSCLC (64%), CRC (52%), and breast cancer (52%) samples compared to the other cancer types. These findings underscore that numerous patients may benefit from clinical tumor sequencing. Several, but not all, actionable genes can be tested one at a time using conventional sequencing techniques such as Sanger sequencing. In contrast, a high-throughput panel sequencing can test all potential actionable genes simultaneously which ultimately helps clinicians find therapeutic options that best fit each patient. It takes a lot of time and specimens to test multiple genes sequentially. As a result, testing with multiplex platform has become commonplace in major cancer centers. We detected actionable alterations that provide therapeutic benefit in both the major tumor types in which genetic testing is frequently performed as well as in rare types of cancer, although such alterations appeared to be less frequent in the rare cancers [17]. Thus, high-throughput panel sequencing can also benefit patients with rare cancers. Novel, flexible clinical trial designs (i.e., umbrella trials and basket trials), in which patients are assigned to investigational therapies based on their mutational profile, have emerged and facilitated new targeted therapeutic strategies [18]. It is anticipated that patients with NGS-defined biomarkers will ultimately receive genomically matched therapies that could result in improved clinical outcomes [19]. Measuring the burden of nonsynonymous mutation has become important as it is the predictor of immunotherapy response in cancer patients [20]. NGS test will also be utilized in predicting immunotherapy response.
The overall actionable mutation frequency in our study (54%) was significantly higher than that observed in the Memorial Sloan Kettering Cancer Center (MSKCC)-study using MSK-IMPACT (37%) [2]. There are three factors that may have contributed to this difference. First, the proportion of samples from NSCLC patients in our cohort was higher than that in the MSKCC-study [2]. Second, EGFR mutation in NSCLC patients has been reported to be more prevalent in the Asian population and in the female populations [4,21]. Indeed, compared to the EGFR mutation frequency in NSCLC patients in the MSKCC cohort (24%), the frequency in our cohort was much higher (47%), similar to previous reports [2,21]. Third, MSK-IMPACT excluded KRAS mutations from the actionable category, while we included them in our analysis [2]. The combination of these three factors likely increased the actionable mutation frequency in our study. The mutation burdens in this study were also somewhat higher than the previously reported mutation rates [22,23]. As our target genes were enriched for cancer-related genes and the samples sequenced at high coverage (> 800×), the mutation burden of our data is likely higher than that of whole genome sequencing or whole exome sequencing data.
In comparison with the conventional sequencing methods, one big hurdle in the implementation of NGS tests into routine practice can be the cost efficiency on the provider end. Substantial setup costs are required for the computational infrastructure, such as a laboratory space for the cooling system, electricity, fault recovery, a backup system, along with IT support [7]. To improve the affordability and sustainability of this technology, an alternative approach to current practice needs to be considered. Souilmi et al. [24] suggested that this bioinformatic bottlenecks could be overcome by cloud-based computing. However, the possibility of exposing sensitive personal genomic data while transferring and sharing data raises privacy and ethical concerns. Even if these issues are resolved by de-identification techniques, another challenge is the integration of multiple data sources and the minimization of inter-laboratory data discrepancies that can arise from differences in instruments, experimental methods, sequencing instruments and analysis platforms. Furthermore, the target regions differ substantially between laboratories, which leads to challenges in the normalization of the systemic biases, especially in copy number and RNA expression data [25]. The use of different data analysis tools by each laboratory results in a different output format. Merging these different outputs into one format is another obstacle.
To overcome these challenges, we performed pan-cancer panel sequencing using a centralized laboratory system model [8]. This system allowed us to consistently sequence tumor samples from different institutes and combine data sets without adjusting their format. Within a centralized system we needed to set appropriate tissue requirements for optimizing an NGS workflow [26]. Recently, several studies have discussed the appropriate amount of DNA required for successful sequencing [5,26-28]. Cho et al. [26] proposed that more than five unstained slides (5 μm in thickness) for FFPE biopsies and more than one unstained slide for FFPE resection specimens were adequate for a NGS. However, the minimum amount of DNA required for different NGS workflows varies, and the amount of DNA extracted from one slide also varies depending on the area and volume of the tumor tissue [26,27]. Thus, it is difficult to predict the minimum number of unstained slides and the exact DNA yield in clinical practice. To minimize test failures resulting from an insufficient amount of DNA, we established our criterion: 10 unstained slides for FFPE biopsies and two unstained slides for FFPE resection specimens. Additionally, we found that for FFPE samples, inadequate DNA quality was correlated with the storage duration. As DNA in FFPE tissue blocks is likely to be degraded during storage, we suggest that the storage duration should be considered and, if possible, controlled when sequencing FFPE samples. It would be better to use FFPE tissue blocks with storage duration less than 3 years to ensure test quality. To use NGS study in clinics, it is important to check if the sequencing covered all the important position. We used test results with mean target coverage more than 200× only. Theoretically 200× coverage is required to detect variants of allele fraction 10% with 99% sensitivity [28].
To use NGS test in clinical practice, it should not take too long for the test results to be produced. The duration of DNA extraction to NGS reporting was 2-3 weeks with fast mode [29]. We believe that this turn-around time is adequate for patients to make timely therapeutic decisions. For longitudinal monitoring of a patient’s actionable tumor mutations, it is not always possible to collect recurring tumor specimens by invasive methods. In those cases, sequencing circulating tumor DNA (ctDNA) from plasma may be a viable alternative [30]. Several attempts have been made to apply this technique in clinical trials [31]. Once the accuracy of testing ctDNA improves, genome-matched therapy is likely to be more widely practiced.
In conclusion, drawing on our experiences, we support the use of an NGS workflow in a centralized laboratory system model. We hope that our insight could help in the integration of genomic data from different institutes and the understanding of disease-gene relationships.
Electronic Supplementary Material
Supplementary materials are available at Cancer Research and Treatment website (https://www.e-crt.org).
ACKNOWLEDGMENTS
We thank Kirsten Curnow, Kristine Jinnett, and Mueller Amy, an employee of Illumina Inc., for providing medical writing support. This research was supported by a grant of the Korean Health Technology R&D Project through the Korea Health Industry Development Institute, funded by the Ministry of Health & Welfare, Republic of Korea (HI13C2096 to W.Y.P., HI14C0072 to H.L.K.), and grant from Ministry of Food and Drug Safety, Republic of Korea (16173-MFDS004 to W.Y.P.).
References
1. Meyerson M, Gabriel S, Getz G. Advances in understanding cancer genomes through second-generation sequencing. Nat Rev Genet. 2010; 11:685–96.


2. Zehir A, Benayed R, Shah RH, Syed A, Middha S, Kim HR, et al. Mutational landscape of metastatic cancer revealed from prospective clinical sequencing of 10,000 patients. Nat Med. 2017; 23:703–13.
3. Katsnelson A. Momentum grows to make 'personalized' medicine more 'precise'. Nat Med. 2013; 19:249.


4. Cancer Genome Atlas Research Network. Comprehensive molecular profiling of lung adenocarcinoma. Nature. 2014; 511:543–50.
5. Frampton GM, Fichtenholtz A, Otto GA, Wang K, Downing SR, He J, et al. Development and validation of a clinical cancer genomic profiling test based on massively parallel DNA sequencing. Nat Biotechnol. 2013; 31:1023–31.


6. Cho YS, Kim H, Kim HM, Jho S, Jun J, Lee YJ, et al. An ethnically relevant consensus Korean reference genome is a step towards personal reference genomes. Nat Commun. 2016; 7:13637.


7. Schadt EE, Linderman MD, Sorenson J, Lee L, Nolan GP. Computational solutions to large-scale data management and analysis. Nat Rev Genet. 2010; 11:647–57.


8. Hynes SO, Pang B, James JA, Maxwell P, Salto-Tellez M. Tissue-based next generation sequencing: application in a universal healthcare system. Br J Cancer. 2017; 116:553–60.


9. Shin HT, Choi YL, Yun JW, Kim NK, Kim SY, Jeon HJ, et al. Prevalence and detection of low-allele-fraction variants in clinical cancer samples. Nat Commun. 2017; 8:1377.


10. Lee S, Seo J, Park J, Nam JY, Choi A, Ignatius JS, et al. Korean Variant Archive (KOVA): a reference database of genetic variations in the Korean population. Sci Rep. 2017; 7:4287.


11. Guyard A, Boyez A, Pujals A, Robe C, Tran Van Nhieu J, Allory Y, et al. DNA degrades during storage in formalin-fixed and paraffin-embedded tissue blocks. Virchows Arch. 2017; 471:491–500.


12. Cancer Genome Atlas Research Network. Comprehensive genomic characterization of squamous cell lung cancers. Nature. 2012; 489:519–25.
13. George J, Lim JS, Jang SJ, Cun Y, Ozretic L, Kong G, et al. Comprehensive genomic profiles of small cell lung cancer. Nature. 2015; 524:47–53.
14. Peifer M, Fernandez-Cuesta L, Sos ML, George J, Seidel D, Kasper LH, et al. Integrative genome analyses identify key somatic driver mutations of small-cell lung cancer. Nat Genet. 2012; 44:1104–10.
15. Cancer Genome Atlas Research Network. Comprehensive molecular characterization of gastric adenocarcinoma. Nature. 2014; 513:202–9.
16. Cancer Genome Atlas Network. Comprehensive molecular characterization of human colon and rectal cancer. Nature. 2012; 487:330–7.
17. Lim SM, Yoo JE, Lim KH, Meng Tai DW, Cho BC, Park YN. Rare incidence of ROS1 rearrangement in cholangiocarcinoma. Cancer Res Treat. 2017; 49:185–92.
18. Menis J, Hasan B, Besse B. New clinical research strategies in thoracic oncology: clinical trial design, adaptive, basket and umbrella trials, new end-points and new evaluations of response. Eur Respir Rev. 2014; 23:367–78.


19. Jordan EJ, Kim HR, Arcila ME, Barron D, Chakravarty D, Gao J, et al. Prospective comprehensive molecular characterization of lung adenocarcinomas for efficient patient matching to approved and emerging therapies. Cancer Discov. 2017; 7:596–609.


20. Rizvi NA, Hellmann MD, Snyder A, Kvistborg P, Makarov V, Havel JJ, et al. Cancer immunology: mutational landscape determines sensitivity to PD-1 blockade in non-small cell lung cancer. Science. 2015; 348:124–8.
21. Lee B, Lee T, Lee SH, Choi YL, Han J. Clinicopathologic characteristics of EGFR, KRAS, and ALK alterations in 6,595 lung cancers. Oncotarget. 2016; 7:23874–84.


22. Alexandrov LB, Nik-Zainal S, Wedge DC, Aparicio SA, Behjati S, Biankin AV, et al. Signatures of mutational processes in human cancer. Nature. 2013; 500:415–21.
23. Kandoth C, McLellan MD, Vandin F, Ye K, Niu B, Lu C, et al. Mutational landscape and significance across 12 major cancer types. Nature. 2013; 502:333–9.


24. Souilmi Y, Lancaster AK, Jung JY, Rizzo E, Hawkins JB, Powles R, et al. Scalable and cost-effective NGS genotyping in the cloud. BMC Med Genomics. 2015; 8:64.


25. Pfundt R, Del Rosario M, Vissers LE, Kwint MP, Janssen IM, de Leeuw N, et al. Detection of clinically relevant copy-number variants by exome sequencing in a large cohort of genetic disorders. Genet Med. 2017; 19:667–75.


26. Cho M, Ahn S, Hong M, Bang H, Van Vrancken M, Kim S, et al. Tissue recommendations for precision cancer therapy using next generation sequencing: a comprehensive single cancer center's experiences. Oncotarget. 2017; 8:42478–86.


27. Austin MC, Smith C, Pritchard CC, Tait JF. DNA yield from tissue samples in surgical pathology and minimum tissue requirements for molecular testing. Arch Pathol Lab Med. 2016; 140:130–3.


28. Lee C, Bae JS, Ryu GH, Kim NK, Park D, Chung J, et al. A method to evaluate the quality of clinical gene-panel sequencing data for single-nucleotide variant detection. J Mol Diagn. 2017; 19:651–8.


29. Hagemann IS, Devarakonda S, Lockwood CM, Spencer DH, Guebert K, Bredemeyer AJ, et al. Clinical next-generation sequencing in patients with non-small cell lung cancer. Cancer. 2015; 121:631–9.


Fig. 1.
Diagram of central lab model. Samples are collected to a central lab and processed using the same platform so the resulting data can be easily integrated. At a central laboratory, sample preparation procedures, including quality control, sequencing, and data analysis, were performed. In this project, five tertiary hospitals were participating. FF, fresh frozen; FFPE, formalin-fixed, paraffin embedded.
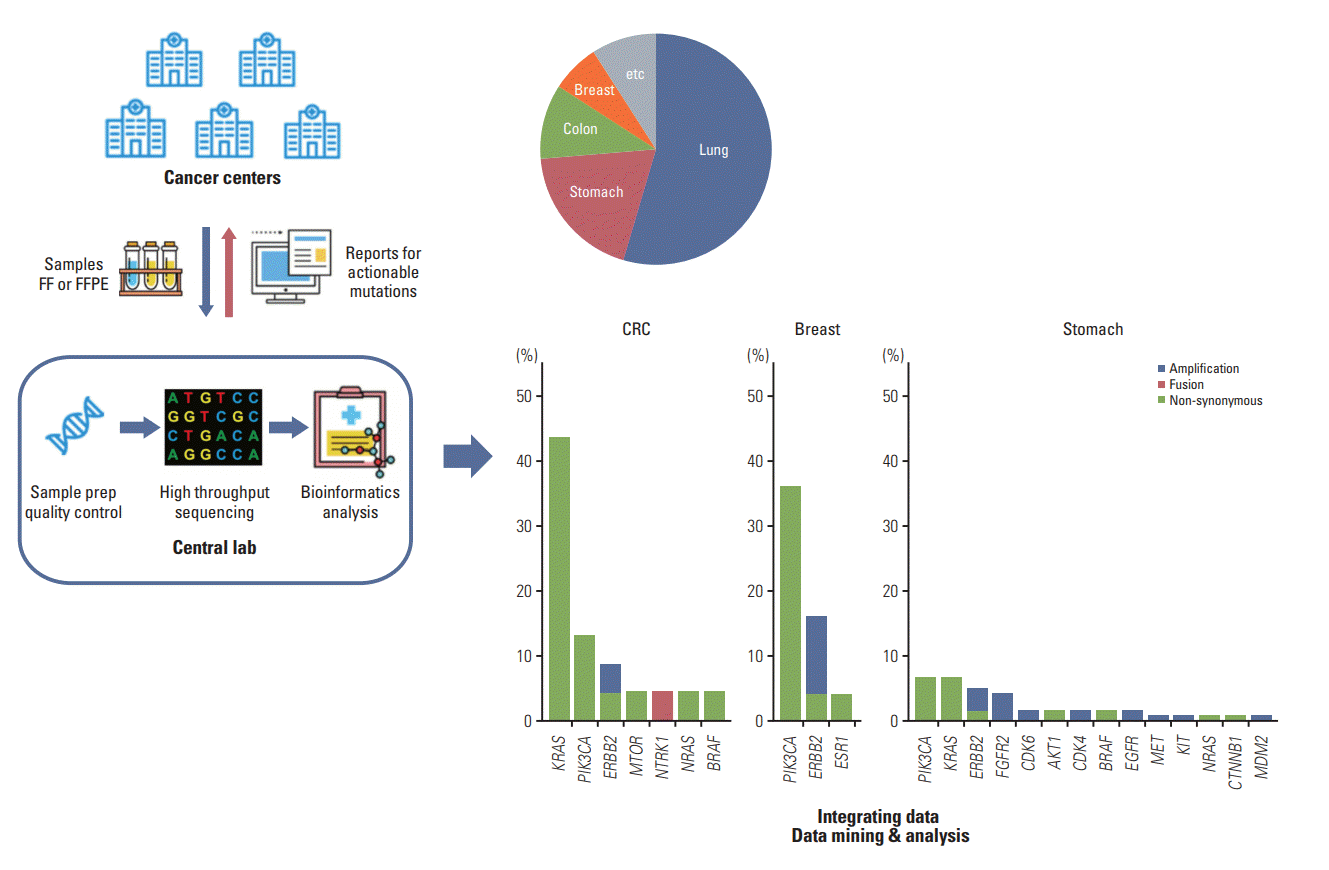
Fig. 2.
The effect of sample condition on extracted DNA size. (A) In formalin-fixed, paraffin embedded (FFPE) samples, the size of DNA was shorter with degradation. This phenomenon was more prominent in resected tissues than in biopsied tissues. The horizontal line at 350 bp represented the minimum required size of extracted DNA to be included in the sequencing step. (B) The size of DNA tended to be shorter as storage duration prolonged. The horizontal line at 350 bp represented the minimum required size of extracted DNA to be included in the sequencing step. FF, fresh frozen; NSCLC, non-small cell lung cancer; SCLC, small cell lung cancer.
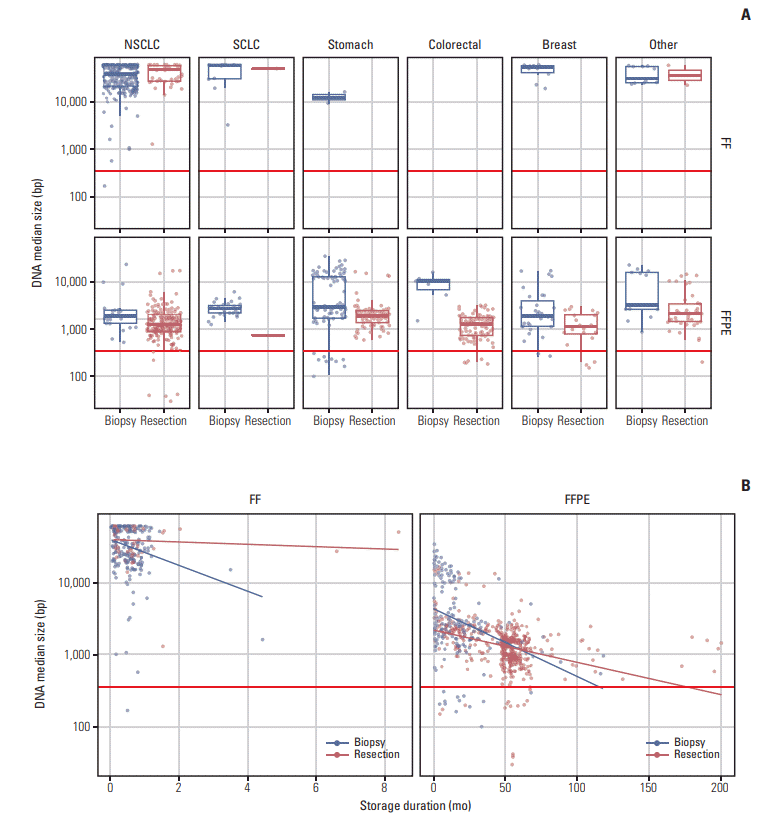
Fig. 3.
Multivariate analysis of the effect of sample condition on QC pass. QC fails were more frequently observed particularly in biopsied specimens or specimens with long storage time. In the forest plot, odds ratios (ORs) were described in log scale. When the confidence interval does not contain 1.00, the p-value is less than 0.05. FF, fresh frozen; FFPE, formalin-fixed, paraffin embedded.
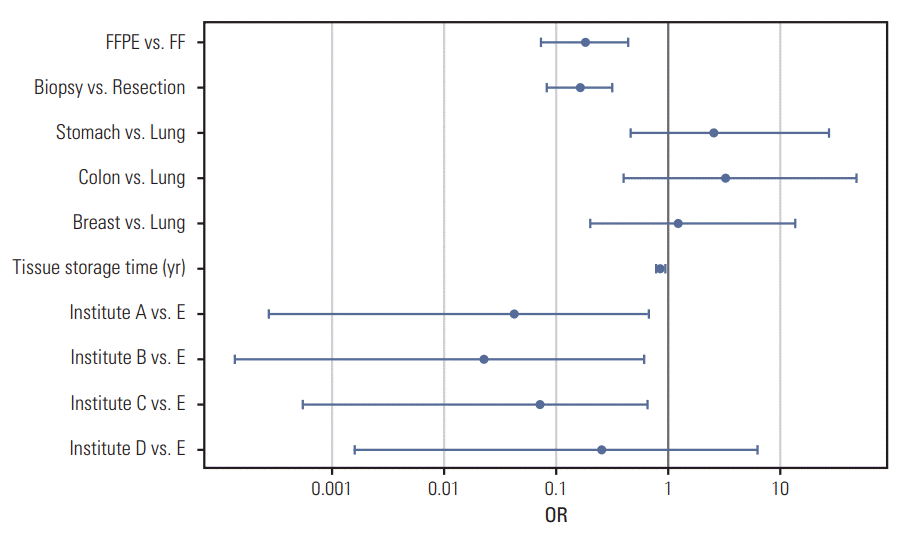
Fig. 4.
Proportion of patients having actionable variants of certain genes in this cohort. Most frequently observed mutations in each cancer types were EGFR mutation in non-small cell lung cancer (NSCLC), RICTOR amplification in small cell lung cancer (SCLC), KRAS mutation in colorectal cancer (CRC), and PIK3CA mutation in breast cancer and stomach cancer. Significant proportion of actionable mutations were found in low frequency, showing long tail.
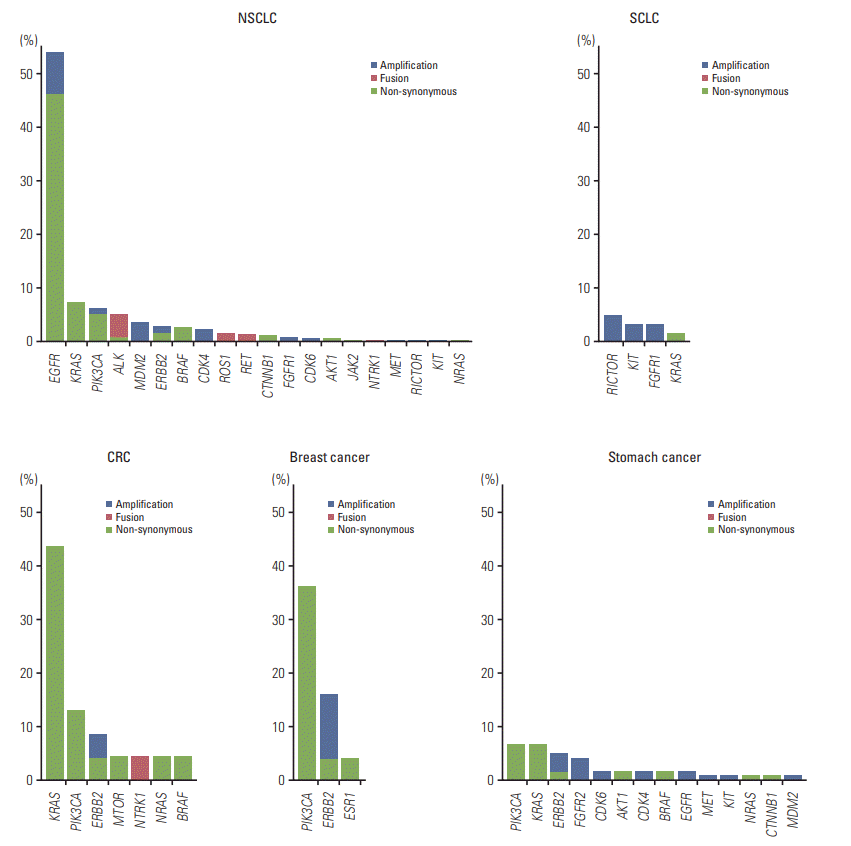
Table 1.
Quality measures and pass rate among different institutes