Abstract
Purpose
We previously developed a model to more accurately predict life expectancy for stage IV cancer patients referred to radiation oncology. The goals of this study are to validate this model and to compare competing published models.
Materials and Methods
From May 2012 to March 2015, 280 consecutive patientswith stage IV cancerwere prospectively evaluated by a single radiation oncologist. Patients were separated into training, validation and combined sets. TheNEAT model evaluated number of active tumors (“N”), Eastern Cooperative Oncology Group performance status (“E”), albumin (“A”) and primary tumor site (“T”). The Odette Cancer Center model validated performance status, bone only metastases and primary tumor site. The Harvard TEACHH model investigated primary tumor type, performance status, age, prior chemotherapy courses, liver metastases, and hospitalization within 3 months. Cox multivariable analyses and logisticalregressionwere utilized to compare model performance.
Results
Number of active tumors, performance status, albumin, primary tumor site, prior hospitalizationwithin the last 3 months, and liver metastases predicted overall survival on uinvariate and multivariable analysis (p < 0.05 for all). The NEAT model separated patients into four prognostic groups with median survivals of 24.9, 14.8, 4.0, and 1.2 months, respectively (p < 0.001). The NEAT model had a C-index of 0.76 with a Nagelkerke’s R2 of 0.54 suggesting good discrimination, calibration and total performance compared to competing prognostic models.
Radiation therapy is a cost-effective and time efficient treatment to control pain, neurologic symptoms and obstructive symptoms from advanced cancer that can achieve local control of tumors in any organ system [1]. It is now well accepted that clinician intuition and experience alone is inadequate to accurately predict survival for stage IV cancer, with physicians typically overestimating survival [2-4]. There is significant interest in developing a more accurate model to predict survival for radiation oncology patients with stage IV cancer [5-7]. An accurate estimate of survival is important for patients to inform treatment decisions and to initiate endof-life planning and timely palliative care consultation [8]. Inaccurate survival prognostication can result in the patients receiving high intensity cancer care towards the end of life [9,10].
A model developed at Good Samaritan Hospital Medical Center separated patients with stage IV cancer into four prognostic groups using four prognostic factors that were found to be significant predictors of overall survival [5]. The acronym NEAT describes these factors: number of active tumors (“N”), Eastern Cooperative Oncology Group (ECOG) performance status (“E”), serum albumin (“A”), and primary tumor type (“T”). Four groups were identified with median survivals ranging from 1.2 months, 4.1 months, 14.5 months, and >31.4 months. The TEACHH model developed at Harvard Medical School divided incurable cancer patients into three life expectancy groups using type of cancer, performance status, age, prior palliative chemotherapy, prior hospitalizations, and hepatic metastases [7]. The three groups had median survivals of 1.7 months, 5 months, and 19.9 months, respectively. A third model developed at Odette Cancer Center in Toronto separated patients with advanced cancer into three groups using performance status, primary tumor type and site of metastases [6]. The three groups had survivals of 2.1 months, 6.0 months, and 13.8 months. Despite extensive research, there is no current consensus on the optimal approach to develop an accurate prognosis for patients with stage IV cancer.
The objectives of the current study were to (1) validate the results of the Good Samaritan model using a temporal validation set and (2) to evaluate the relative performance of the Good Samaritan model compared to the Odette Cancer Center and Harvard TEACHH models.
This study included 280 consecutive patients older than 18 years with metastatic stage IV solid tumor who were referred to a single physician in a large community hospital-based radiation oncology department between May 2012 and March 2015. Since 45 patients did not have a recent serum albumin available, the analysis was limited to 235 patients. The first 116 analyzed patients were evaluated between May 2012 and August 2013 and represented the training cohort. The subsequent 119 analyzed patients were evaluated between September 2013 and March 2015 and represented the validation cohort.
The charts of all patients were assessed for previously validated prognostic factors including performance status (ECOG 0 to 1 vs. ECOG 2 vs. ECOG 3-4), primary tumor type (breast, prostate, kidney, lung or other), number of tumors (1 to 5 vs. ≥ 6), serum albumin (≥ 3.4 g/dL vs. 2.4 to 3.3 g/dL vs. ≤ 2.4 g/dL), location of metastases (bone only vs. liver vs. other), age (> 60 vs. ≤ 60), hospitalization within the past 3 months (0 vs. ≥ 1), and prior palliative chemotherapy courses (≥ 2 vs. 0-1). The calculations of risk groups using the Good Samaritan NEAT, Odette Cancer Center, and Harvard TEACHH models are shown in Table 1.
Statistical analysis was performed with Stata ver. 13 (StataCorp., College Station, TX). The primary outcome was overall survival, defined as time from initial radiation oncology consultation to date of death. Patients who were lost to follow-up were censored at the date of last follow-up. Survival data was analyzed using the Kaplan-Meier method and summarized by median and 6-month survival. Chi-square tests were performed to determine differences in categorical variables between cohorts. Differences in survival were assessed through the log-rank method. The multivariable analyses were performed by Cox proportional hazards models and verified by tests of correlations over time with examination of residual plots. The ability of various models to predict 6-month survival was analyzed using logistical regression. Patients who were lost to follow-up with less than 6-month follow-up were excluded from the logistical regression. Statistical tests were two sided and p-values of < 0.05 were considered statistically significant.
The three prognostic models were assessed using the performance measures discrimination, calibration and overall performance [11]. Predictive discrimination is the probability of concordance between predicted and observed survival. Predictive discrimination is measured by the Harrell’s C index with 0.5-0.59 indicating poor, 0.6-0.69 indicating moderate, 0.7-0.79 indicating good, 0.8-0.89 indicating very good, and > 0.9 indicating excellent discrimination [12,13]. The C indices of various models were compared using the techniques described by Newson [14]. Calibration quantifies whether the observed outcomes and predicted probabilities agree. For calibration < 10% point difference between predicted and observed mortality is considered a well calibrated model [13]. Overall performance of various prediction models is the distance between predicted outcome and actual outcome and is measured by Nagelkerke’s R2 from a logistical regression [11].
The characteristics of the 116 patients in the training cohort and 119 in the validation are summarized in Table 2. There were differences between the populations with respect to primary tumor site, performance status, age and frequency of bone only metastases.
Median survival was 4.1 months in the training cohort (95% confidence interval [CI], 3.0 to 5.8) and 6.7 months in the validation cohort (95% CI, 4.4 to 10.1) (p=0.07). The median overall survival for the entire cohort was 5.4 months (95% CI, 4.1 to 6.6) with a median follow-up for surviving patients of 20.7 months (95% CI, 8.5 to 26.1) (Fig. 1).
All four components of the NEAT model, including number of tumors, ECOG performance status, serum albumin and primary tumor site predicted survival on both univariate and Cox multivariable analysis (Table 3). For the Odette Cancer Center model, primary tumor site and Karnofsky performance status were predictive on univariate and Cox multivariable analysis but bone only metastasis was not predictive (Table 3). With respect to the TEACHH model, primary tumor site, ECOG performance status, prior hospitalization within the last 3 months and liver metastases predicted survival on both univariate and Cox multivariable analysis (Table 3). However, age and extent of prior palliative chemotherapy were not predictive.
In the training group, Good Samaritan NEAT model divided the patients into four distinct groups with median survivals of 37.5, 14.8, 4.0, and 1.2 months, respectively (p < 0.01). The Odette Cancer Center model separated the patients into three groups with median survivals of 37.5, 12.7, and 2.2 months (p < 0.01). The Harvard TEACHH model divided the patients into three groups with median survivals of 25.0, 4.8, and 1.2 months (p < 0.01). In all cases, there was good visual agreement between the estimated survival from the Cox model compared to actual outcome estimated by the Kaplan-Meier method (Fig. 2A-C).
In the temporal validation cohort, the Good Samaritan NEAT model identified four distinct groups of patients with median survivals of 19.7, 14.9, 4.3, and 0.9 months (p < 0.01). The Odette Cancer Center model separated patients into groups with median survivals of 10.4, 9.2, and 3.0 months (p < 0.01). The Harvard TEACHH model divided patients into three groups with median survivals of 16.5, 6.8, and 1.8 months (p < 0.01). There remained good visual agreement between predicted and observed survival in all three models (Fig. 2D-F).
In the combined dataset, the NEAT model separated patients into four prognostic groups with median survivals of 24.9, 14.8, 4.0, and 1.2 months, respectively (Fig. 3A). The Odette Cancer Center model divided patients into three prognostic groups with median survivals of 16.4, 12.4, and 2.6 months, respectively (Fig. 3B). The Harvard TEACHH model identified cohorts with median survivals of 25.0, 5.7, and 1.6 months, respectively (Fig. 3C).
The C indices for the Good Samaritan NEAT model were 0.76, 0.75, and 0.76 for the training, validation and combined sets (Table 4). The corresponding C indices for the Odette Cancer Center model were 0.67, 0.64, and 0.66, respectively for the training, validation and combined groups. Finally, the C indices for the Harvard TEACHH model were 0.59, 0.60, and 0.60, respectively for the training, validation and combined groups. In all groups, the C indices were significantly higher for the Good Samaritan NEAT model than the Odette Cancer Center or Harvard TEACHH models (p < 0.01 for all).
The calibration of the Good Samaritan NEAT model was good, with close agreement between the observed 6-month mortality rates in the low risk, intermediate risk, and high risk groups. The Odette Cancer Center model had comparable mortality rates for risk groups 2 and 3. The TEACHH model showed similar mortality rates for risk group A.
Finally, in the combined group the Nagelkerke’s R2 for predicting 6-month survival was 0.54 for the Good Samaritan NEAT model compared to 0.30 for the Odette Cancer Center model and 0.16 for the Harvard TEACHH model.
Approximately 20% to 40% of patients treated in radiation oncology departments have metastatic cancer requiring radiotherapy [7]. Patients with stage IV cancer referred to radiation oncology are heterogeneous ranging from the actively dying with survival measured in days to patients with asymptomatic oligometastases who may achieve longterm remission [15-17]. We previously developed a robust model that separated patients into four distinct groups with markedly different life expectancies [5]. In the training, validation and combined cohorts, the Good Samaritan NEAT model performed well compared to previously published models evaluating radiation oncology patients with metastatic cancer.
There is significant focus in the literature on preventing overtreatment of poor prognosis patients [18]. Conversely, accurate identification of better prognosis patients may prompt the treating physician to consider treatment intensification to improve disease-free survival and even overall survival [19-21]. Broader use of predictive models may allow for personalized treatment consistent with patient’s needs, values and preferences [1].
There is a robust literature on estimating life expectancy for terminally ill cancer patients seen in inpatient medical oncology, palliative care and hospice units who were no longer candidates for treatment [7,22]. Site-specific prognostic factors for brain metastases, spinal cord compression and bone metastases are particularly useful for subspecialists with practice focused on a specific organ site [23-25]. For the practicing general radiation oncologist, the ideal prognostic model would account for both poor and favorable prognosis patients in multiple clinical contexts over diverse cancer types [1]. The Good Samaritan NEAT model accounts for tumor histology and tumor burden to accurately predict survival for patients with longer life expectancy while strongly weighting both performance status and albumin for patients with short life expectancy.
While performance status and primary tumor are also included in other radiation oncology predictive models, there is significant divergence in other prognostic factors. After analyzing 29 prognostic factors, the Good Samaritan NEAT model identified serum albumin and number of active tumors as novel predictors of survival in radiation oncology patients [5]. For oncology patients, albumin is an objective measure of anorexia-cachexia syndrome and malnutrition that is widely available on comprehensive metabolic panels [26]. Additionally, hypoalbuminemia can also reflect physiologic causes of third spacing, inflammation and sepsis that may be associated with poor prognosis. Oligometastases are defined as one to five distant metastases that are potentially amenable to involved site radiation to all areas of known disease [16,17,27]. Although the exact number of dominant masses and pathologic lymph nodes is not always specified on radiology reports, radiation oncologists have extensive experience with reviewing recent computed tomography, magnetic resonance imaging, positron emission tomography, and bone scans and can readily quantify number of tumors through electronic medical records and picture archiving and communication systems. Interestingly, performance status, extent of active disease and intake are key elements of the Palliative Performance Scale, which is widely used in palliative medicine to predict survival [28]. The NEAT model further refines and extends the Palliative Performance Scale by quantifying the relative contribution of performance status, extent of active disease, intake and primary tumor site on prognosis.
In community practice, the Good Samaritan NEAT model appeared to have a higher C-index and Nagelkerke’s R2 than the Odette Cancer Center and Harvard TEACHH models. In this study, differences in model performance were driven by variables other than performance status and primary tumor site (Table 3). The NEAT model included number of tumors and albumin as variables that strongly predicted survival on multivariable analysis. The TEACHH model included age and extent of prior palliative chemotherapy and the Odette Cancer Center included bone only metastases that were not statistically significant on multivariable analysis.
The Good Samaritan NEAT model was developed in the community hospital setting and may not be generalizable to major academic medical centers where the Odette Cancer Center and Harvard TEACCH models were developed. The Odette Cancer Center study included 33% of patients with bone only metastases compared to 14% in the current dataset. While these clearly reflect differences in practice patterns the causes is unclear. One might speculate that 64% of the patients in the Odette Cancer Center study were patients from a novel Rapid Response Radiotherapy Program treated in 1999 to 2000 that affected referral patterns to increase utilization of palliative radiotherapy for bone metastases that would otherwise be treated with systemic therapy alone in other settings. The Harvard TEACHH dataset included 45% of patients who were 60 years of age or younger compared to 23% in the current dataset. The Harvard TEACHH patient population included 17% patients that were treated with > 2 prior palliative chemotherapy courses compared to 11% in this dataset. From these data, one can infer that patients in community practice tend to be older and may be less likely to receive greater than second-line chemotherapy than a major academic center with an extensive cancer clinical trial program. Further development of the NEAT model and other predictive models for stage IV cancer require external validation in diverse community and academic settings.
A limitation of the NEAT model is that a small minority of stage IV patients do not have recent albumin or extent of disease imaging available. If these tests have not been ordered, patients can still be evaluated with alternate models. Finally, currently available predictive models can only explain a modest percentage of observed survival due to the effect of unmeasured patient-specific prognostic factors. In the future, biomarkers and measures of antitumor immunity will supplement currently available clinical, radiologic, laboratory and pathologic prognostic factors to further refine existing models [29,30]. Although the accuracy of experienced clinicians in making unsupervised predictions in cancer is poor, the role of clinical intuition to further improve algorithmdefined predictions in a complex system such as oncology is worthy of investigation for both clinicians and data scientists.
References
1. Jones JA, Lutz ST, Chow E, Johnstone PA. Palliative radiotherapy at the end of life: a critical review. CA Cancer J Clin. 2014; 64:296–310.


2. Lamont EB, Christakis NA. Complexities in prognostication in advanced cancer: "to help them live their lives the way they want to". JAMA. 2003; 290:98–104.
3. Chow E, Davis L, Panzarella T, Hayter C, Szumacher E, Loblaw A, et al. Accuracy of survival prediction by palliative radiation oncologists. Int J Radiat Oncol Biol Phys. 2005; 61:870–3.


4. Hartsell WF, Desilvio M, Bruner DW, Scarantino C, Ivker R, Roach M 3rd, et al. Can physicians accurately predict survival time in patients with metastatic cancer? Analysis of RTOG 97-14. J Palliat Med. 2008; 11:723–8.


5. Kao J, Gold KD, Zarrili G, Copel E, Silverman AJ, Ramsaran SS, et al. Clinical predictors of survival for patients with stage IV cancer referred to radiation oncology. PLoS One. 2015; 10:e0124329.
6. Chow E, Abdolell M, Panzarella T, Harris K, Bezjak A, Warde P, et al. Predictive model for survival in patients with advanced cancer. J Clin Oncol. 2008; 26:5863–9.


7. Krishnan MS, Epstein-Peterson Z, Chen YH, Tseng YD, Wright AA, Temel JS, et al. Predicting life expectancy in patients with metastatic cancer receiving palliative radiotherapy: the TEACHH model. Cancer. 2014; 120:134–41.


8. Mack JW, Cronin A, Taback N, Huskamp HA, Keating NL, Malin JL, et al. End-of-life care discussions among patients with advanced cancer: a cohort study. Ann Intern Med. 2012; 156:204–10.
9. Morden NE, Chang CH, Jacobson JO, Berke EM, Bynum JP, Murray KM, et al. End-of-life care for medicare beneficiaries with cancer is highly intensive overall and varies widely. Health Aff (Millwood). 2012; 31:786–96.


10. Baek SK, Chang HJ, Byun JM, Han JJ, Heo DS. The association between end-of-life care and the time interval between provision of a do-not-resuscitate consent and death in cancer patients in Korea. Cancer Res Treat. 2017; 49:502–8.


11. Steyerberg EW, Vickers AJ, Cook NR, Gerds T, Gonen M, Obuchowski N, et al. Assessing the performance of prediction models: a framework for traditional and novel measures. Epidemiology. 2010; 21:128–38.
12. Harrell FE Jr, Lee KL, Mark DB. Multivariable prognostic models: issues in developing models, evaluating assumptions and adequacy, and measuring and reducing errors. Stat Med. 1996; 15:361–87.


13. Yourman LC, Lee SJ, Schonberg MA, Widera EW, Smith AK. Prognostic indices for older adults: a systematic review. JAMA. 2012; 307:182–92.
14. Newson RB. Comparing the predictive powers of survival models using Harrell's C or Somers' D. Stata J. 2010; 10:339–58.


15. Mulvenna P, Nankivell M, Barton R, Faivre-Finn C, Wilson P, McColl E, et al. Dexamethasone and supportive care with or without whole brain radiotherapy in treating patients with non-small cell lung cancer with brain metastases unsuitable for resection or stereotactic radiotherapy (QUARTZ): results from a phase 3, non-inferiority, randomised trial. Lancet. 2016; 388:2004–14.


16. Milano MT, Katz AW, Zhang H, Okunieff P. Oligometastases treated with stereotactic body radiotherapy: long-term followup of prospective study. Int J Radiat Oncol Biol Phys. 2012; 83:878–86.


17. Salama JK, Hasselle MD, Chmura SJ, Malik R, Mehta N, Yenice KM, et al. Stereotactic body radiotherapy for multisite extracranial oligometastases: final report of a dose escalation trial in patients with 1 to 5 sites of metastatic disease. Cancer. 2012; 118:2962–70.
18. Gripp S, Mjartan S, Boelke E, Willers R. Palliative radiotherapy tailored to life expectancy in end-stage cancer patients: reality or myth? Cancer. 2010; 116:3251–6.
19. Koshy M, Malik R, Mahmood U, Rusthoven CG, Sher DJ. Comparative effectiveness of aggressive thoracic radiation therapy and concurrent chemoradiation therapy in metastatic lung cancer. Pract Radiat Oncol. 2015; 5:374–82.


20. Gomez DR, Blumenschein GR Jr, Lee JJ, Hernandez M, Ye R, Camidge DR, et al. Local consolidative therapy versus maintenance therapy or observation for patients with oligometastatic non-small-cell lung cancer without progression after first-line systemic therapy: a multicentre, randomised, controlled, phase 2 study. Lancet Oncol. 2016; 17:1672–82.


21. Kao J, Chen CT, Tong CC, Packer SH, Schwartz M, Chen SH, et al. Concurrent sunitinib and stereotactic body radiotherapy for patients with oligometastases: final report of a prospective clinical trial. Target Oncol. 2014; 9:145–53.
22. Feliu J, Jimenez-Gordo AM, Madero R, Rodriguez-Aizcorbe JR, Espinosa E, Castro J, et al. Development and validation of a prognostic nomogram for terminally ill cancer patients. J Natl Cancer Inst. 2011; 103:1613–20.


23. Sperduto PW, Chao ST, Sneed PK, Luo X, Suh J, Roberge D, et al. Diagnosis-specific prognostic factors, indexes, and treatment outcomes for patients with newly diagnosed brain metastases: a multi-institutional analysis of 4,259 patients. Int J Radiat Oncol Biol Phys. 2010; 77:655–61.


24. Rades D, Fehlauer F, Schulte R, Veninga T, Stalpers LJ, Basic H, et al. Prognostic factors for local control and survival after radiotherapy of metastatic spinal cord compression. J Clin Oncol. 2006; 24:3388–93.


25. Chow E, James JL, Hartsell W, Scarantino CW, Ivker R, Roach M, et al. Validation of a predictive model for survival in patients with advanced cancer: secondary analysis of RTOG 9714. World J Oncol. 2011; 2:181–90.
26. Inui A. Cancer anorexia-cachexia syndrome: current issues in research and management. CA Cancer J Clin. 2002; 52:72–91.


27. Kao J, Packer S, Vu HL, Schwartz ME, Sung MW, Stock RG, et al. Phase 1 study of concurrent sunitinib and image-guided radiotherapy followed by maintenance sunitinib for patients with oligometastases: acute toxicity and preliminary response. Cancer. 2009; 115:3571–80.
28. Anderson F, Downing GM, Hill J, Casorso L, Lerch N. Palliative performance scale (PPS): a new tool. J Palliat Care. 1996; 12:5–11.


29. Sperduto PW, Yang TJ, Beal K, Pan H, Brown PD, Bangdiwala A, et al. Estimating survival in patients with lung cancer and brain metastases: an update of the graded prognostic assessment for lung cancer using molecular markers (Lung-mol-GPA). JAMA Oncol. 2017; 3:827–31.
Fig. 2.
Survival probability based on groups according to the NEAT model (A), Odette Cancer Center model (B), and TEACHH model (C) developed from the training cohort. Survival probability based on groups according to the NEAT model (D), Odette Cancer Center model (E), and TEACHH model (F) developed from the validation cohort. Survival estimated using the Cox’s model (dashed lines) and the actual survival calculated by the Kaplan-Meier method (solid lines) are shown.
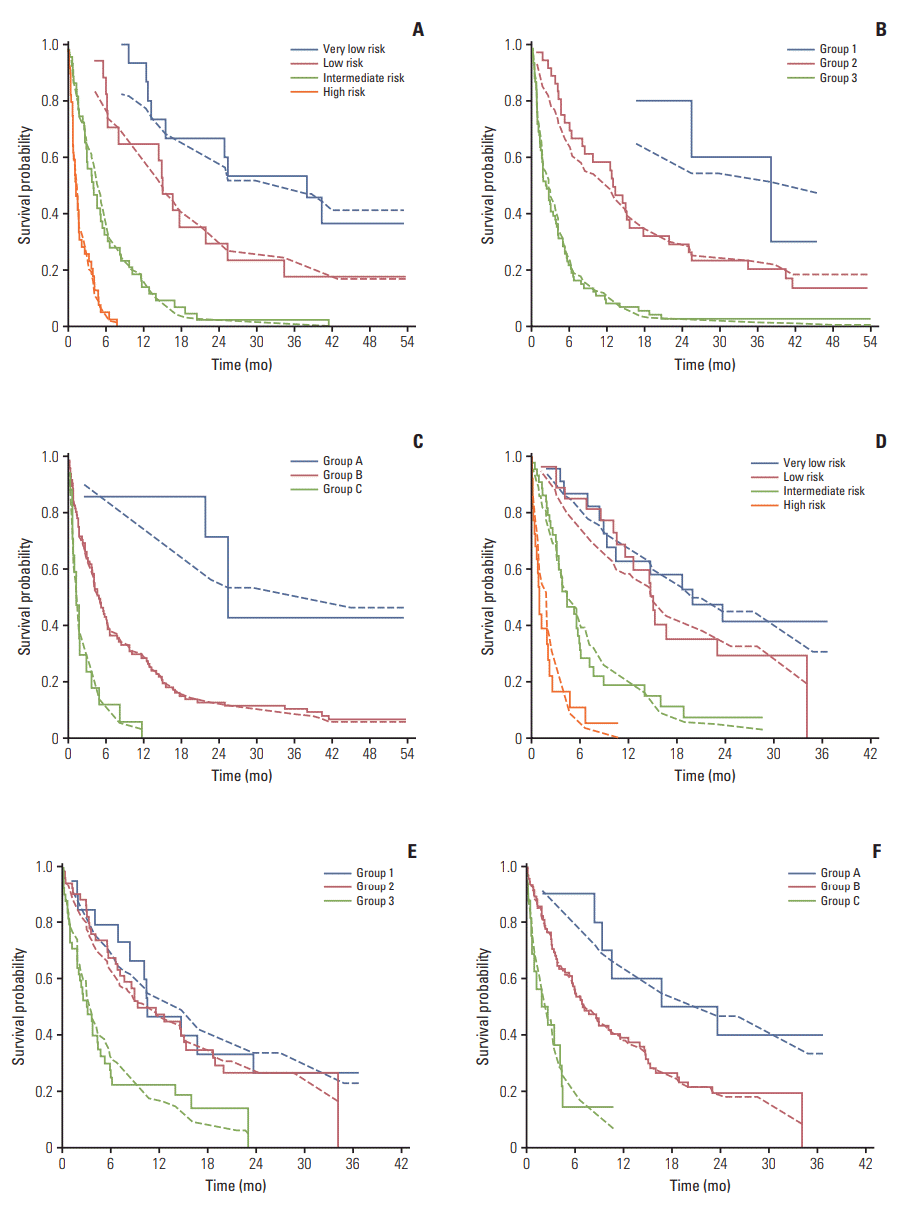
Fig. 3.
Overall survival based on groups according to the NEAT model (A), Odette Cancer Center model (B), and TEACHH model (C) developed from the combined cohort.
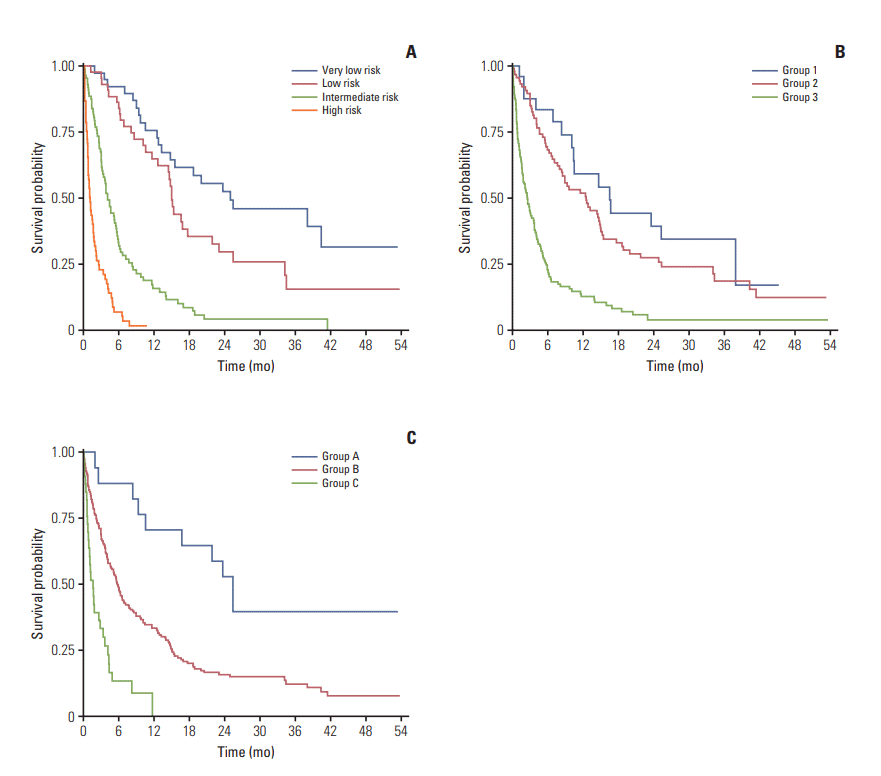
Table 1.
Risk group calculations by previously identified models
Table 2.
Patient characteristics
Table 3.
Univariate and multivariate analysis of predictors of overall survival for each model (n=235)
Table 4.
Comparing model performance