Abstract
Purpose
Lynch syndrome, the commonest hereditary colorectal cancer syndrome, is caused by germline mutations in mismatch repair (MMR) genes. Three recently developed prediction models for MMR gene mutations based on family history and clinical features (MMRPredict, PREMM1,2,6, and MMRPro) have been validated only in Western countries. In this study, we propose validating these prediction models in the Korean population.
Materials and Methods
We collected MMR gene analysis data from 188 individuals in the Korean Hereditary Tumor Registry. The probability of gene mutation was calculated using three prediction models, and the overall diagnostic value of each model compared using receiver operator characteristic (ROC) curves and area under the ROC curve (AUC). Quantitative test characteristics were calculated at sensitivities of 90%, 95%, and 98%.
Results
Of the individuals analyzed, 101 satisfied Amsterdam criteria II, and 87 were suspected hereditary nonpolyposis colorectal cancer. MMR mutations were identified in 62 of the 188 subjects (33.0%). All three prediction models showed a poor predictive value of AUC (MMRPredict, 0.683; PREMM1,2,6, 0.709; MMRPro, 0.590). Within the range of acceptable sensitivity (> 90%), PREMM1,2,6 demonstrated higher specificity than the other models.
Conclusion
In the Korean population, overall predictive values of the three models (MMRPredict, PREMM1,2,6, MMRPro) for MMR gene mutations are poor, compared with their performance in Western populations. A new prediction model is therefore required for the Korean population to detect MMR mutation carriers, reflecting ethnic differences in genotype-phenotype associations.
Hereditary nonpolyposis colorectal cancer (HNPCC) is the most common form of hereditary colorectal cancer, comprising 3%-4% of colorectal cancer cases [1]. The lifetime risk of colorectal cancer (CRC) in these patients is as high as 80%, and HNPCC is associated with extra-colonic tumors of endometrium, ovary, urinary tract, small intestine, stomach, brain, and sebaceous glands [1]. Although HNPCC was formerly used as a synonym for Lynch syndrome, HNPCC can encompass a heterogeneous group of hereditary nonpolyposis CRCs with or without germline mutations of mismatch repair (MMR) genes. Clinically diagnosed HNPCC by the Amsterdam criteria can include not only Lynch syndrome but also Lynch-like syndrome or familial CRC type X [2,3]. In recognition of this problem, the latest World Health Organization (WHO) classification of tumors of the digestive system recommended “Lynch syndrome” as the standard term of a hereditary cancer syndrome caused by a germline mutation in one of the MMR genes [4]. Lynch syndrome is caused by germline mutations in MMR genes, including MLH1, MSH2, MSH6, and PMS2 [5]. Early identification of such mutation carriers is essential to facilitate counseling, intensive surveillance and prophylactic surgery or chemoprevention. Since universal genetic testing is not cost-effective considering the rarity of this condition and germline sequencing efforts, it is necessary to prescreen individuals with suspected Lynch syndrome prior to genetic testing [6].
Several clinical criteria and molecular tests are used to screen individuals with MMR mutations. However, strategies for identifying MMR mutation carriers require improvement. Amsterdam criteria I and II, originally developed to define families suspected of HNPCC [7], are commonly utilized to determine eligibility for testing. However, these criteria are too stringent for use as a screening tool, leading to lack of sensitivity and specificity [6]. The less strict Bethesda guidelines have been utilized for this purpose [8], but may also overlook up to 15% of mutation carriers [9]. Another available method to detect possible Lynch syndrome families is microsatellite instability (MSI) testing, the genetic hallmark of Lynch syndrome. However, about 15% of sporadic CRC patients exhibit MSI [10], which may result in unnecessary genetic evaluation. Immunohistochemical analysis (IHC) of the major MMR proteins, MLH1 and MSH2, is used to identify individuals with MMR mutations. However, given that up to 5% of patients with Lynch syndrome show microsatellite stable or normal IHC staining [11], those at high risk of MMR mutations may be undetected. The most critical shortcoming of these clinical criteria and molecular testing protocols is that they are not designed to determine the risk of MMR mutations in individuals [6].
Several prediction models to help assess the probability of MMR mutations in individuals have been developed [12-14]. The prediction tools, MMRPredict [12], PREMM1,2,6 [14], and MMRPro [13], are widely used to estimate the likelihood of harboring MMR gene mutations based on clinical information. A number of Western population-based studies have validated the feasibility of these models [6,11,15-19] in facilitating potential advancement in screening and diagnosis of Lynch syndrome. Considering the ethnic differences of Lynch syndrome patients evaluated previously [20-22], prediction models need to be validated before clinical application in Asian countries. Therefore, the current study was designed to assess the performance of prediction models for MMR gene mutations in the Korean population.
We retrospectively obtained data from the Korean Hereditary Tumor Registry, including clinical and molecular findings from HNPCC or suspected HNPCC families. HNPCC and suspected HNPCC were defined according to Amsterdam criteria II [7] and revised S-HNPCC I criteria [23]. One hundred and eighty-eight individuals from 142 families with available details on pedigree and results of mutation screening for MMR genes were included in the study.
Mutational risk scores were calculated for the three prediction models, MMRPredict, PREMM1,2,6 and MMRPro.
MMRPredict, developed by Barnetson et al. [12], is a web-based online calculator freely available at http://hnpccpredict.hgu.mrc.ac.uk/. Variables in this model include proband age, sex, tumor location, presence of synchronous or metachronous CRC, presence and age of first-degree relatives with CRC or endometrial cancer. The model is limited to CRC-related patients only, since it was developed based on data from patients under the age of 55 diagnosed with CRC. Therefore, data for only 149 individuals were calculated for this model.
PREMM1,2,6 [14] is also freely available at http://premm.dfci.harvard.edu/. This model consists of proband-specific and family member-related variables, including occurrence and age at diagnosis of CRC, endometrial cancer, and other Lynch-associated tumors.
MMRPro is calculated with CaGENE 5.2 software (Cancer-Gene, Dallas, TX) available at http://www4.utsouthwestern.edu/breasthealth/cagene. This model includes age at diagnosis of CRC or endometrial cancer of the proband, first- and second-degree relatives and current age of unaffected relatives.
Missing or unknown data were imputed by applying a practice similar to previous studies [11,17]. In unknown cases, age was estimated based on the age of relatives, assuming 30-year separation between generations. When only information about an individual’s age group was available, a mid-range age was used [11]. Unknown tumor location was entered into the prediction model as both proximal and distal locations, and the mean value of the two was used [17].
Individuals were given mutational screening for the MMR genes—MLH1, MSH2 and MSH6. Peripheral blood lymphocytes were isolated using Ficoll-Paque (Amersham Biosciences, Uppsala, Sweden), and genomic DNA was extracted using TRI reagent according to the manufacturer’s instructions (Molecular Research Center, Cincinnati, OH). Next, we screened all gene coding regions, exon/intron boundaries and core promoters using polymerase chain reaction–single strand conformation polymorphism analysis to detect germline mutations in MMR genes. The detailed methods are described in a previous report by our group [20].
The expected frequency of MMR mutations was compared with observed frequency to test the reliability of the three models [24]. Receiver-operator characteristic (ROC) curves were constructed to evaluate precision. Area under the ROC curve (AUC) was calculated as a measure of discrimination for each model [24]. We additionally estimated specificity, positive likelihood ratios and predicted risk scores at a range of sensitivities (90%, 95%, and 98%) to test model accuracy [24]. Chi-squared test and Student’s t test were employed to compare categorical and continuous data. We rejected null hypotheses of no difference if p-values were less than 0.05. Statistical analyses used SPSS ver. 19.0 (IBM Corp., Armonk, NY).
Of the 188 subjects, 57 were diagnosed as HNPCC, 44 were their family members, 85 were suspected HNPCC probands, and the remaining two were from a suspected HNPCC family. Clinical characteristics of patients are summarized in Table 1. Sixty-two (33.0%) carried MMR gene mutations, 77.8% of which were MLH1. The majority of mutations identified were frameshift (53.7%) or missense (38.9%) mutations. Individuals with MMR mutations were significantly younger (40.2±13.7 years) than those without the mutations (47.7±14.1 years). We identified 40 men (35.1%) and 19 women (31.1%) with mutations; the proportions of men and women with mutations did not differ significantly. No association was evident between MMR mutation and cancer location.
The distribution of risk scores calculated for each model is depicted in Fig. 1. The three prediction models showed different distribution patterns. Risk scores of PREMM1,2,6 were positively skewed, i.e., concentrated in the lower range, while those of MMRPro were skewed to the left, being concentrated in the higher range. Median risk scores were 25.0% for MMRPredict, 14.2% for PREMM1,2,6, and 82.25% for MMRPro.
We compared the expected frequency of MMR mutations predicted by each model with observed frequency, with subjects divided into five groups by the calculated risk scores (Table 2). All three models underestimated the frequency of mutations in the < 50% risk score group. However, the models commonly overestimated the possibility of MMR mutations in the ≥ 50% risk score group. In general, PREMM1,2,6 underestimated the mutational frequency, with an expected to observed mutation ratio of 0.8, while MMRPredict and MMRPro tended to overestimate the possibility of mutations (expected/observed mutation carriers, 1.3 and 1.7, respectively).
The results of ROC analysis for three models are presented in Fig. 2. We observed an AUC of 0.709 (95% confidence interval [CI], 0.634 to 0.784) for PREMM1,2,6, 0.683 (95% CI, 0.592 to 0.773) for MMRPredict and 0.590 (95% CI, 0.493 to 0.688) for MMRPro. AUC from the PREMM1,2,6 model was the highest, although this difference was not statistically significant.
We additionally calculated specificity, positive likelihood ratio and risk score cutoff of the three prediction models in a range of sensitivities (Table 3). To achieve a sensitivity of 90%, a cutoff risk score of > 6.0% led to specificity of 34.3% and positive likelihood ratio of 1.4 for MMRPredict. Specificity decreased to 3.9% to obtain a sensitivity of 95%. For PREMM1,2,6, to gain a sensitivity of 90%, a cutoff risk score of > 7.4% provided specificity of 36.3% and positive likelihood ratio of 1.4, highest among the three models. Specificity remained 32.3% at a sensitivity of 95%. PREMM1,2,6 showed the highest specificity (25.8%) at a sensitivity of 98%.
To our knowledge, this is the first study to explore the performance of Lynch prediction models in an Asian population. All three models tended to underestimate the possibility of MMR mutation in low-risk individuals; but overestimated the possibility of mutation in high-risk individuals. AUCs of the three models were 0.709 for PREMM1,2,6, 0.683 for MMRPredict and 0.590 for MMRPro, signifying fair to poor discrimination. Within the range of acceptable sensitivity that reduces the number of missed mutant carriers (sensitivity > 90%), PREMM1,2,6 showed higher specificity (36.3%) than the other two models (34.3% for MMRPredict, 21.2% for MMRPro).
After the development of each Lynch syndrome prediction model [12-14], a number of studies explored performance in Western populations [6,11,15-19]. Some investigations used clinic-based samples similar to our study [6,11,17,18] and others were from population-based samples [15,16] or both [19]. Win et al. [25] reviewed prediction models for MMR gene mutations and performed a meta-analysis of AUCs from reviewed studies. Pooled AUCs were 0.84 for PREMM, 0.81 for MMRPredict, and 0.80 for MMRPro, indicative of good performance. Although some authors report that the Lynch prediction models provide no better discrimination than the revised Bethesda guidelines [16,18], they are generally considered a feasible strategy for further risk assessment and genetic testing [5].
However, our results indicate that the available prediction models are not broadly applicable to Asians, since the molecular characteristics of CRC between patients with distinct ethnic origins are substantially different. For instance, De Jesus-Monge et al. [22] reported wide variations (0.6%-47.1%) in negative MLH1 protein expression in CRC patients from different countries. Previously, we showed differences in MMR gene mutations in Korean HNPCC families [20] and a lower rate of MSI-high (5.5%) in Korean CRC patients than European ethnicities (10%-25%) but rates similar to Japanese patients (4.5%-5.9%) [21]. These ethnic differences may hinder the application of prediction models to Koreans, resulting in poor performance.
Each prediction model has distinct characteristics [11, 17,25]. Thus, clinical utilization of the three models may vary for different situations. MMRPredict is a web-based easily accessible model, but it includes only individuals diagnosed with CRC [17]. It can be useful for patients with early-onset CRC [11]. PREMM1,2,6 does not take into account MSI/IHC test results but is ideal for families with HNPCC-related cancers [11,17]. MMRPro needs a complete family history demanding a first-degree relative affected to estimate risk to proband [11,17]. The model is useful when MSI/IHC test results are both negative [11]. In our study population, the three models showed differences in reliability, accuracy, and even distribution. Considering both AUC and specificity within acceptable sensitivity, PREMM1,2,6 may have slightly better performance in Asian populations, despite lower reliability.
Our study has some limitations. Initially, data were collected from our registry database comprising HNPCC or suspected HNPCC families determined by Amsterdam criteria II or revised S-HNPCC I criteria. The registry-based retrospective study design prevented us from utilizing revised Bethesda guidelines, and resulted in lack of MSI testing or immunohistochemistry for MMR proteins, producing a bias. Nevertheless, AUCs of our study (0.590-0.709) were significantly lower than those of clinic-based studies on Western populations (0.77-0.82) [25]. In addition, the proportion of MLH1 mutations (77.8%) among all detected MMR mutations is higher than that of previous studies (25%-44%) [6,11,16-19], which could represent potential selection bias. However, a population-based study reported a surprisingly low proportion (5.6%) of MLH1 mutations among all detected mutations [15], suggesting that an unusually high proportion of MLH1 mutations might happen by chance. Another possibility is that individuals from 11 families in this study inherited a founder mutation (c.1757_1758insC) in the MLH1 gene from a common ancestor [20]. Because validation of a prediction model performance is individual-based, and therefore depends mainly on the precise mutational analysis and detailed family history of each individual, it seems unlikely that our results are severely biased.
Owing to the retrospective design, we were confronted with difficulties with missing data. Although methods similar to previous studies were used [11,17], we could not apply all models to all individuals due to missing values. Lastly, we did not examine mutations in PMS2 and EpCAM genes in this study. However, current prediction models were developed to predict the possibility of having mutations only in the MLH1, MSH2, and MSH6 genes, and mutations in PMS2 and EpCAM genes were not taken into account. This is a limitation of current prediction models, although mutations in PMS2 or EpCAM genes are very rare in Lynch syndrome. Therefore, we believe that the present study is of value despite the lack of PMS2 or EpCAM mutational results.
The overall performances of all three prediction models in our Korean population were poor compared to that in Western populations. We propose that a new prediction model is required for the Korean population to effectively detect MMR mutation carriers that reflects ethnic differences in genotype-phenotype associations.
ACKNOWLEDGMENTS
This study was supported by a grant from the National Cancer Center (number 1420180).
References
2. Lindor NM, Rabe K, Petersen GM, Haile R, Casey G, Baron J, et al. Lower cancer incidence in Amsterdam-I criteria families without mismatch repair deficiency: familial colorectal cancer type X. JAMA. 2005; 293:1979–85.
3. Mensenkamp AR, Vogelaar IP, van Zelst-Stams WA, Goossens M, Ouchene H, Hendriks-Cornelissen SJ, et al. Somatic mutations in MLH1 and MSH2 are a frequent cause of mismatch-repair deficiency in Lynch syndrome-like tumors. Gastroenterology. 2014; 146:643–6.


4. Bosman FT, Carneiro F, Hruban RH, Theise ND. WHO classification of tumours of the digestive system. 4th ed. Lyon: IARC Press;2010.
5. Kastrinos F, Balmana J, Syngal S. Prediction models in Lynch syndrome. Fam Cancer. 2013; 12:217–28.


6. Monzon JG, Cremin C, Armstrong L, Nuk J, Young S, Horsman DE, et al. Validation of predictive models for germline mutations in DNA mismatch repair genes in colorectal cancer. Int J Cancer. 2010; 126:930–9.


7. Vasen HF, Watson P, Mecklin JP, Lynch HT. New clinical criteria for hereditary nonpolyposis colorectal cancer (HNPCC, Lynch syndrome) proposed by the International Collaborative group on HNPCC. Gastroenterology. 1999; 116:1453–6.


8. Umar A, Boland CR, Terdiman JP, Syngal S, de la Chapelle A, Ruschoff J, et al. Revised Bethesda Guidelines for hereditary nonpolyposis colorectal cancer (Lynch syndrome) and microsatellite instability. J Natl Cancer Inst. 2004; 96:261–8.


9. Perez-Carbonell L, Ruiz-Ponte C, Guarinos C, Alenda C, Paya A, Brea A, et al. Comparison between universal molecular screening for Lynch syndrome and revised Bethesda guidelines in a large population-based cohort of patients with colorectal cancer. Gut. 2012; 61:865–72.


10. Parsons MT, Buchanan DD, Thompson B, Young JP, Spurdle AB. Correlation of tumour BRAF mutations and MLH1 methylation with germline mismatch repair (MMR) gene mutation status: a literature review assessing utility of tumour features for MMR variant classification. J Med Genet. 2012; 49:151–7.
11. Pouchet CJ, Wong N, Chong G, Sheehan MJ, Schneider G, Rosen-Sheidley B, et al. A comparison of models used to predict MLH1, MSH2 and MSH6 mutation carriers. Ann Oncol. 2009; 20:681–8.


12. Barnetson RA, Tenesa A, Farrington SM, Nicholl ID, Cetnarskyj R, Porteous ME, et al. Identification and survival of carriers of mutations in DNA mismatch-repair genes in colon cancer. N Engl J Med. 2006; 354:2751–63.


13. Chen S, Wang W, Lee S, Nafa K, Lee J, Romans K, et al. Prediction of germline mutations and cancer risk in the Lynch syndrome. JAMA. 2006; 296:1479–87.


14. Kastrinos F, Steyerberg EW, Mercado R, Balmana J, Holter S, Gallinger S, et al. The PREMM1,2,6 model predicts risk of MLH1, MSH2, and MSH6 germline mutations based on cancer history. Gastroenterology. 2011; 140:73–81.
15. Green RC, Parfrey PS, Woods MO, Younghusband HB. Prediction of Lynch syndrome in consecutive patients with colorectal cancer. J Natl Cancer Inst. 2009; 101:331–40.


16. Balmana J, Balaguer F, Castellvi-Bel S, Steyerberg EW, Andreu M, Llor X, et al. Comparison of predictive models, clinical criteria and molecular tumour screening for the identification of patients with Lynch syndrome in a population-based cohort of colorectal cancer patients. J Med Genet. 2008; 45:557–63.


17. Khan O, Blanco A, Conrad P, Gulden C, Moss TZ, Olopade OI, et al. Performance of Lynch syndrome predictive models in a multi-center US referral population. Am J Gastroenterol. 2011; 106:1822–7.


18. Tresallet C, Brouquet A, Julie C, Beauchet A, Vallot C, Menegaux F, et al. Evaluation of predictive models in daily practice for the identification of patients with Lynch syndrome. Int J Cancer. 2012; 130:1367–77.


19. Kastrinos F, Steyerberg EW, Balmana J, Mercado R, Gallinger S, Haile R, et al. Comparison of the clinical prediction model PREMM(1,2,6) and molecular testing for the systematic identification of Lynch syndrome in colorectal cancer. Gut. 2013; 62:272–9.
20. Shin YK, Heo SC, Shin JH, Hong SH, Ku JL, Yoo BC, et al. Germline mutations in MLH1, MSH2 and MSH6 in Korean hereditary non-polyposis colorectal cancer families. Hum Mutat. 2004; 24:351.
21. Oh JR, Kim DW, Lee HS, Lee HE, Lee SM, Jang JH, et al. Microsatellite instability testing in Korean patients with colorectal cancer. Fam Cancer. 2012; 11:459–66.


22. De Jesus-Monge WE, Gonzalez-Keelan C, Zhao R, Hamilton SR, Rodriguez-Bigas M, Cruz-Correa M. Mismatch repair protein expression and colorectal cancer in Hispanics from Puerto Rico. Fam Cancer. 2010; 9:155–66.


23. Park JG, Vasen HF, Park YJ, Park KJ, Peltomaki P, de Leon MP, et al. Suspected HNPCC and Amsterdam criteria II: evaluation of mutation detection rate, an international collaborative study. Int J Colorectal Dis. 2002; 17:109–14.


24. Steyerberg EW, Vickers AJ, Cook NR, Gerds T, Gonen M, Obuchowski N, et al. Assessing the performance of prediction models: a framework for traditional and novel measures. Epidemiology. 2010; 21:128–38.
Fig. 1.
Distribution of risk scores of mismatch repair (MMR) gene mutations calculated for the three prediction models. (A) MMRPredict. (B) PREMM1,2,6. (C) MMRPro. The models show different distribution patterns and skewness.
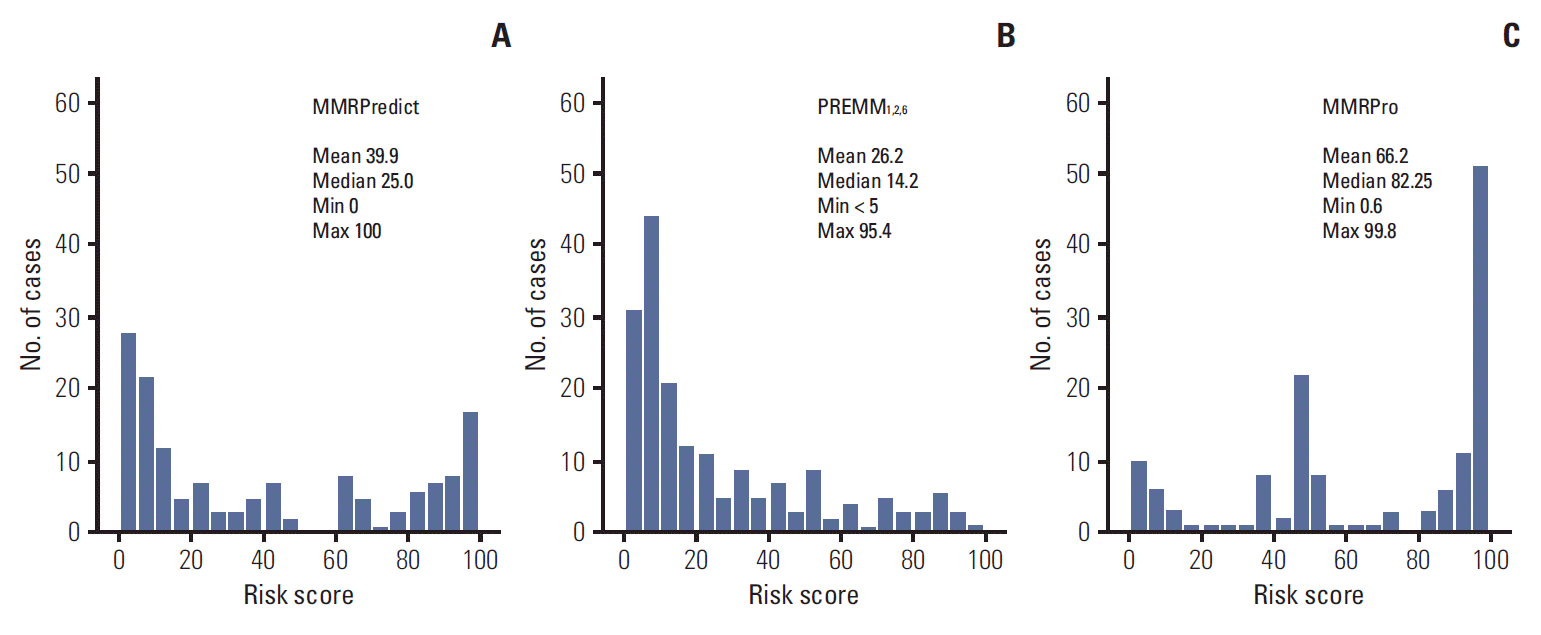
Fig. 2.
Receiver-operator characteristic curves for MMRPredict, PREMM1,2,6, and MMRPro. Area under the ROC curves (AUCs) for each model are shown.
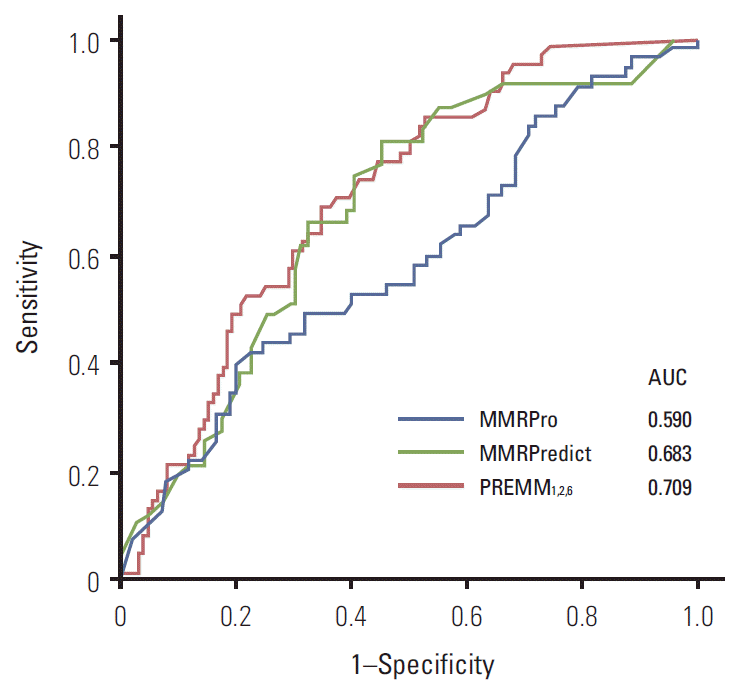
Table 1.
Clinical characteristics by mutation status
Characteristic | Mutation positivea) (n=62) | Mutation negative (n=126) | p-value |
---|---|---|---|
Age (yr) | 40.2±13.7 | 47.7±14.1 | 0.001 |
Sex | |||
Male | 40 (35.1) | 74 (64.9) | 0.599 |
Female | 19 (31.1) | 42 (68.9) | |
Probands | |||
No | 23 (50.0) | 23 (50.0) | 0.005 |
Yes | 39 (27.5) | 103 (72.5) | |
Cancer | |||
No | 13 (38.2) | 21 (61.8) | 0.518 |
Yes | 47 (32.4) | 98 (67.6) | |
Cancer location | |||
Proximal colon | 17 (34.7) | 32 (65.3) | 0.635 |
Distal colon | 21 (28.0) | 54 (72.0) | |
Entire colon | 7 (36.8) | 12 (63.2) | |
Mutation gene | |||
hMLH1 | 42 (77.8) | - | - |
hMSH2 | 9 (16.7) | - | |
hMSH6 | 3 (5.6) | - | |
Mutation type | |||
Frameshift | 29 (53.7) | - | - |
Missense | 21 (38.9) | - | |
Splicing | 4 (7.4) | - |
Table 2.
Comparison of expected and observed frequency of DNA mismatch repair gene mutations
Table 3.
Testing characteristics of the three prediction models at sensitivities of 90%, 95%, and 98%