Abstract
References










































Fig. 1
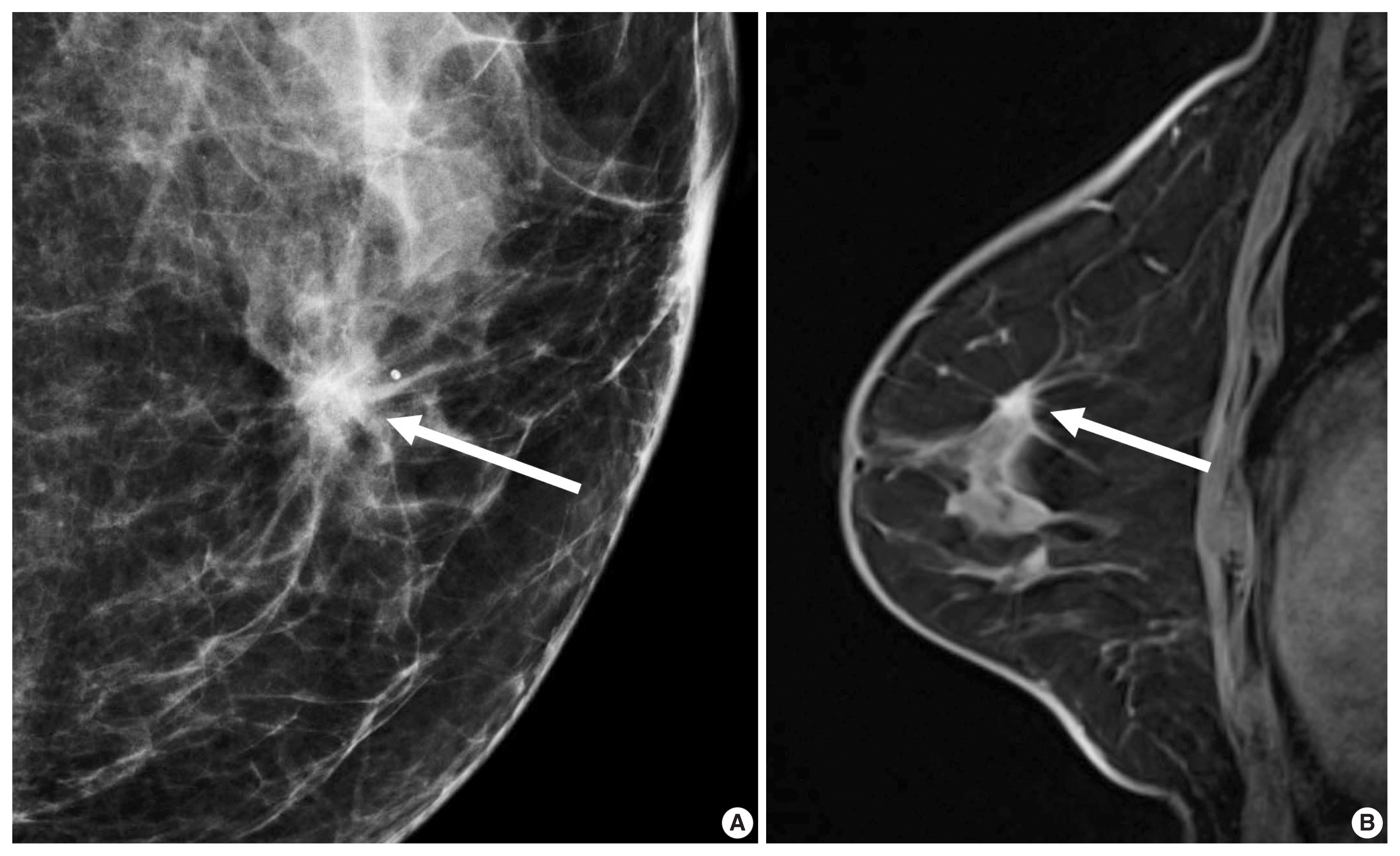
Fig. 2
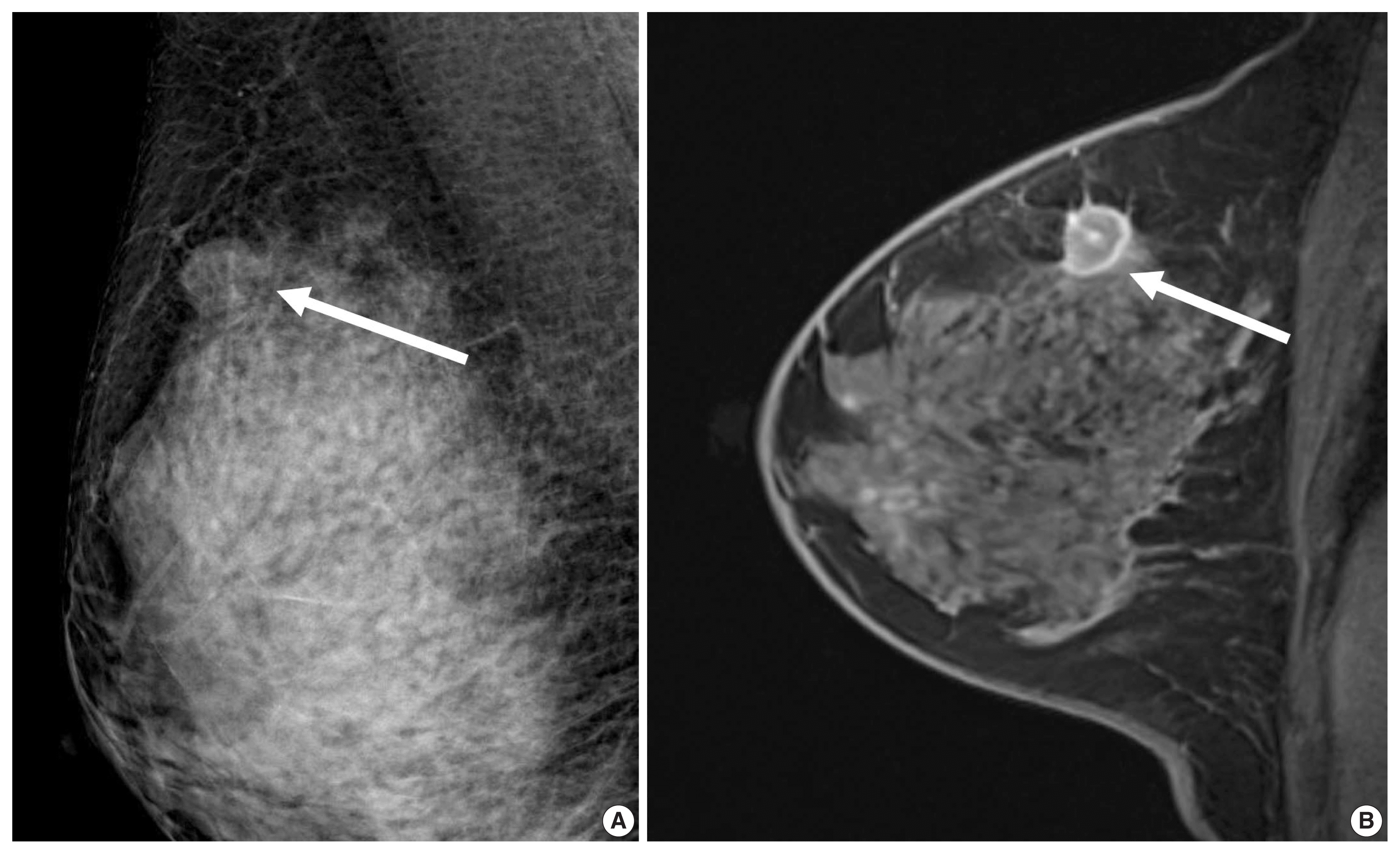
Fig. 3
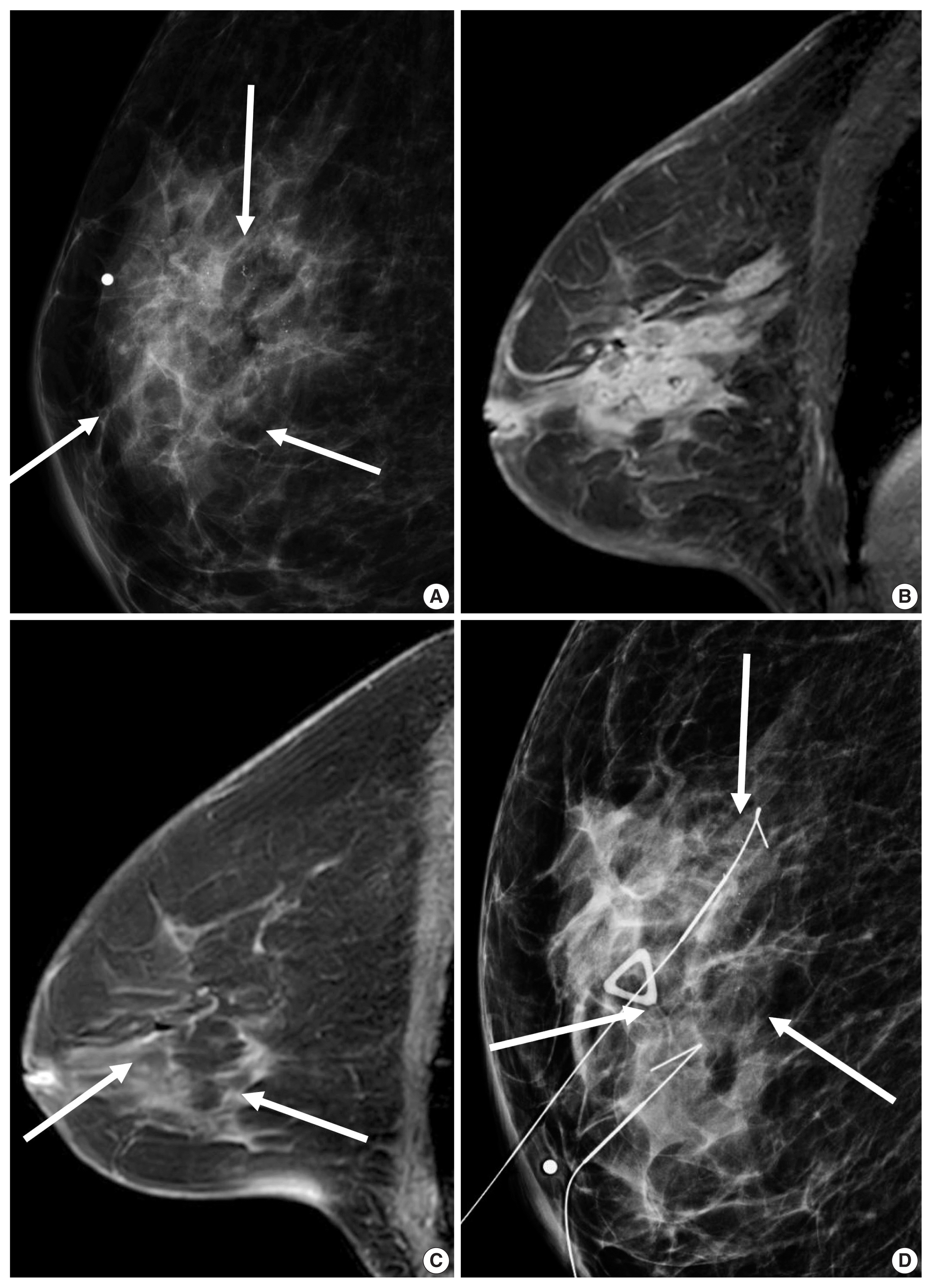
Fig. 4
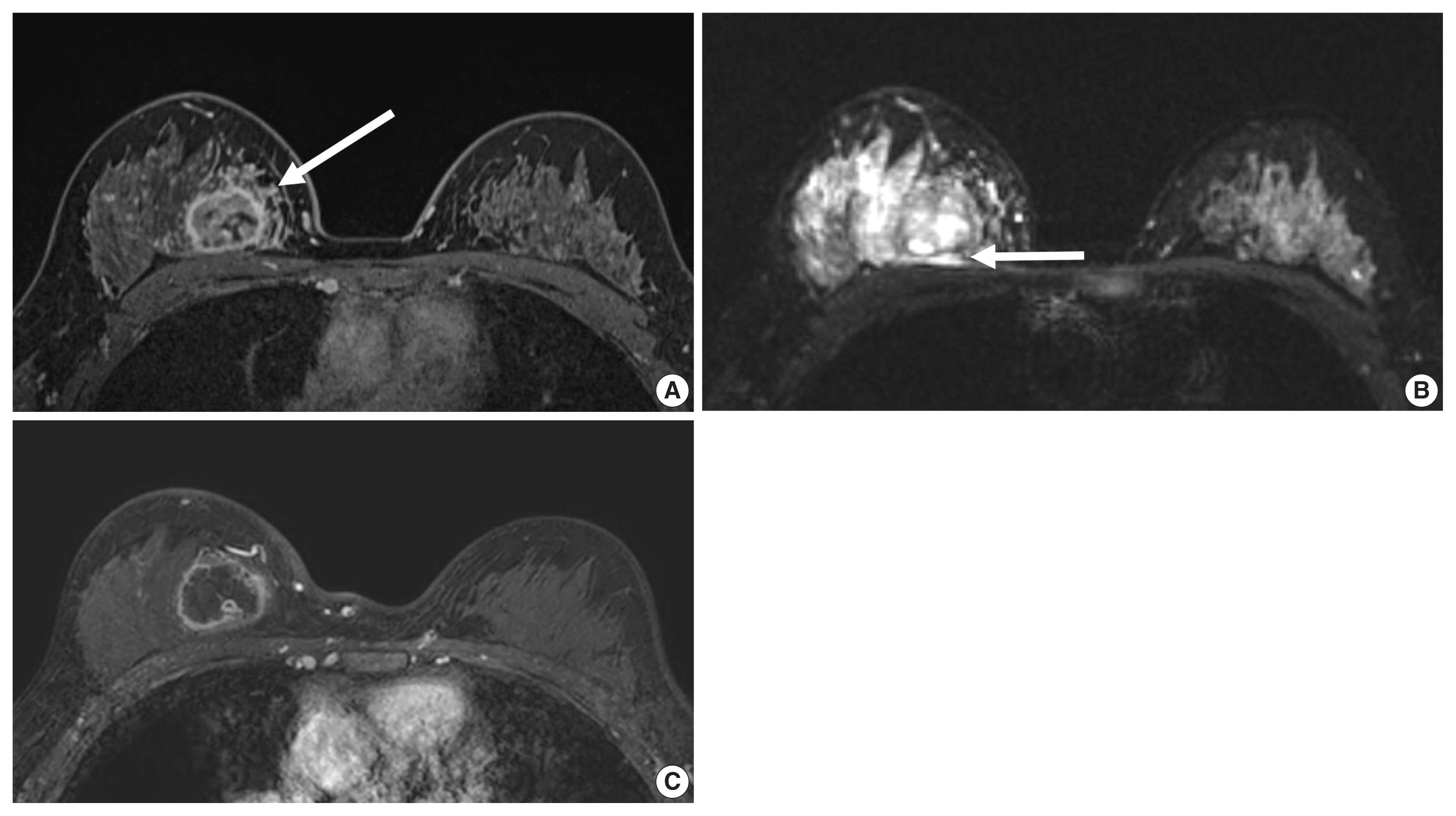
Table 1
Clinical grouping | Notes | Type of therapy |
---|---|---|
Triple-negative | Negative ER, PR, and HER2 |
Cytotoxic chemotherapy including anthracycline and taxane Consideration of cabecitabine for residual tumor after neoadjuvant chemotherapy |
ER negative and HER2 positive | American Society of Clinical Oncology (ASCO)/College of American Pathologists (CAP) guideline 2018a |
Stage 1: paclitaxel+trastuzumab as adjuvant therapy Stage 2 or 3: Neoadjuvant anthracycline, alkylator-, and taxane-based chemotherapy + trastuzumab− and pertuzumab-based treatment (dual anti-HER2 therapy) Trastuzumab ematansine therapy for residual tumor after neoadjuvant chemotherapy in adjuvant setting |
ER positive and HER2 positive | ASCO/CAP guidelines 2018a | As above + endocrine therapy appropriate to menopausal status |
ER positive and HER2 negative | ER and/or PR-positive ≥ 1%b | |
Luminal A-like (high receptor, low proliferation, low grade) |
Multi-parameter molecular marker ‘good’ if availablec High ER/PR and clearly low Ki-67 or grade |
Endocrine therapy alone according to menopausal status |
Intermediate |
Multi-parameter molecular marker ‘intermediate’ if available Uncertainty persists about degree of risk and responsiveness to endocrine and cytotoxic therapies |
Endocrine therapy + adjuvant chemotherapy |
Luminal B-like (low receptor, high proliferation, high grade) | Multi-parameter molecular marker ‘bad’ if available. Lower ER/PR with clearly high Ki-67, high histological grade 3 | Endocrine therapy + adjuvant chemotherapy |
Modified from Coates AS et al. Ann Oncol 2015;26:1533–46 [4] and Burstein HJ et al. Ann Oncol 2019;30:1542–57 [6], according to the Creative Commons license.
ER, estrogen receptor; PR, progesterone receptor; HER2, human epidermal growth factor receptor 2; TNBC, triple-negative breast cancer.
a An immunohistochemistry of c-erbB-2 staining 3+ score was defined as HER2-positive, while a 0 or 1+ score was negative. For tumors with 2+ score, HER2 gene copies to the centromeric region of chromosome 17 ratios < 2.0 by fluorescence in situ hybridization must be interpreted as negative due to the lack of evidence for any benefit from HER2 targeted therapy;
b If ER values fall between 1% and 9%, the term equivocal should not be used, suggesting response to endocrine therapy even in low ER (1%–9%). Low HR expression is associated with higher Ki-67, higher grade, and loss PR positivity, as well as higher recurrence score and higher chemo-sensitivity. Chemotherapy should be given following guidelines for TNBC. Endocrine therapy should be recommended despite the likely extremely small benefit;
Table 2
Study | No. | Imaging Parameters | Outcomes | Results |
---|---|---|---|---|
Leithner et al. (2019) [9] | 143 | First order histogram, co-occurrence matrix | Luminal A, luminal B, TNBC, HER2-enriched | Accuracy: luminal B vs. luminal A, 84.2%; luminal B vs. TNBC, 83.9%; luminal B vs. all others, 89%; HER2-enriched vs. all others, 81.3% |
Kim et al. (2020) [10] | 228 | Radiomics score from 5 MRI features | Disease free survival outcome of TNBC | Combined clinicopathological and radiomics feature model showed highest AUC (0.844) in prediction of DFS |
Song et al. (2020) [11] | 92 | GLCM features | HER2-positive vs. −negative tumors determined by FISH test | Among 3 machine learning methods, LRA, QDA, and SVM, AUC of SVM was the best (0.890) |
Mazurowski et al. (2014) [12] | 48 | Semiautomatically extracted MRI features | TCGA database contains full genomic sequencing | Higher ratio of lesion enhancement rate to background parenchymal enhancement was more likely to be luminal B subtype |
Grimm et al. (2015) [13] | 275 | 56 Features (morphologic, texture, and dynamic features) | luminal A, luminal B, HER2, basal | Luminal A and luminal B molecular subtype were associated with semiautomatically extracted imaging features |
Bae et al. (2015) [14] | 280 | Tumor roundness score | ER, PR, Ki-67 | ER score and Ki-67 index were independent factors determining tumor roundness. TNBC showed the highest mean roundness scores compared with the other subtypes |
Sutton et al. (2015) [15] | 95 | Morphology, histogram features, GLCM features | Oncotype DX recurrence score of Luminal A tumor | An increased kurtosis was found to be a statistically significant factor correlating with Oncotype DX recurrence score |
Waugh et al. (2016) [16] | 221 | Texture features | Hormone receptor–positive and −negative cancers demonstrated significantly different entropy features | Textural differences on contrast-enhanced MR images might reflect underlying lesion subtypes |
Li et al. (2016) [17] | 91 | Texture features | Luminal A, luminal B, HER2-enriched, and basal-like subtype | Enhancement texture (entropy) and molecular subtypes were related. AUC of ER+ vs. ER−: 0.89, PR+ vs. PR−: 0.69, HER2+ vs. HER2−: 0.65, TNBC vs. others: 0.67 |
Agner et al. (2014) [18] | 76 | Morphology, kinetic intensity, histogram features, GLCM features | TNBC, HER2, ER-positive tumors | AUC of texture features (more heterogeneity) for TNBC vs. other subtypes: 0.73 to 0.74 |
Chamming’s et al. (2018) [19] | 85 | Fine, medium, and coarse texture for mean, standard deviation, mean proportion of positive pixels, entropy, skewness, and kurtosis | pCR of TNBC | kurtosis appears to be associated with pCR to neoadjuvant chemotherapy in non–TNBC and may be a promising biomarker for the identification of TNBC |
Braman et al. (2017) [20] | 117 | Intratumoral and peritumoral texture features | Prediction of pCR | Combined intratumoral and peritumoral radiomics for prediction of pCR yielded AUC 0.83 for HR+ HER2−. Non-pCR was characterized by elevated peritumoral heterogeneity during initial phase. For TNBC/HER+ tumors were best characterized by a peritumoral speckled enhancement. |
TNBC, triple-negative breast cancer; HER2, human epidermal growth factor receptor 2; MRI, magnetic resonance imaging; AUC, area under the receiver operating characteristic curve; DFS, disease-free survival; GLCM, gray-level co-occurrence matrix; FISH, fluorescence in situ hybridization; LRA, logistic regression analysis; QDA, quadratic discriminant analysis; SVM, support vector machine; TCGA, The Cancer Genome Atlas; ER, estrogen receptor; PR, progesterone receptor; pCR, pathological complete response.