Abstract
Digital pathology (DP) is no longer an unfamiliar term for pathologists, but it is still difficult for many pathologists to understand the engineering and mathematics concepts involved in DP. Computer-aided pathology (CAP) aids pathologists in diagnosis. However, some consider CAP a threat to the existence of pathologists and are skeptical of its clinical utility. Implementation of DP is very burdensome for pathologists because technical factors, impact on workflow, and information technology infrastructure must be considered. In this paper, various terms related to DP and computer-aided pathologic diagnosis are defined, current applications of DP are discussed, and various issues related to implementation of DP are outlined. The development of computer-aided pathologic diagnostic tools and their limitations are also discussed.
Digital pathology (DP) is now one of the biggest issues facing the field of pathology. DP is a remarkable innovation that changes the paradigm of microscope-based pathology, which has existed for over 100 years. DP alters the way diagnostic tools represent pathologic images from the microscope to the computer screen and has changed storage media from glass slides to digitalized image files. Digitalized pathologic images stored in computer servers or cloud systems can be transmitted over the Internet, thus changing the temporal and spatial domain of pathologic diagnosis. In addition, machine learning allows software assisting in diagnosis to be developed and applied more actively and effectively.
This review describes various concepts related to DP and computer-aided pathologic diagnosis (CAPD), current applications of DP, and various issues related to the implementation of DP. It also briefly introduces the development of computer-aided diagnostic tools and their limitations.
DP, which initially delineated the process of digitizing whole slide images (WSIs) using advanced slide scanning technology, is now a generic term that includes artificial intelligence (AI)–based approaches for detection, segmentation, diagnosis, and analysis of digitalized images [1]. WSI indicates digital representation of an entire histopathologic glass slide at microscopic resolution [2]. Over the last two decades, WSI technology has evolved to encompass relatively high resolution, increased scanner capacity, faster scan speed, smaller image file sizes, and commercialization. The development of appropriate image management systems (IMS) and a seamless interface connection between existing hospital systems such as electronic medical records (EMR), picture archiving communication systems (PACS), and laboratory information systems (LIS) (also referred to as the pathology order communication system) has stabilized, cheaper storage systems have been established, and streaming technology for large image files has been developed [3,4]. Mukhopadhyay et al. [5] evaluated the diagnostic performance of digitalized images compared to microscopic images on specimens from 1,992 patients with different tumor types diagnosed by 16 surgical pathologists. Primary diagnostic performance with digitalized WSIs was not inferior to that achieved with light microscopy-based approaches (with a major discordance rate from the reference standard of 4.9% for WSI and 4.6% for microscopy) [5].
Computer-aided pathology (CAP, also referred to as computeraided pathologic diagnostics, computational pathology, and computer-assisted pathology) refers to a computational diagnosis system or a set of methodologies that utilizes computers or software to interpret pathologic images [2,6]. The Digital Pathology Association does not limit the definition of computational pathology to computer-based methodologies for image analysis, but rather as a field of pathology that uses AI methods to combine pathologic images and metadata from a variety of related sources to analyze patient specimens [2]. The performance of computeraided diagnostic tools has improved with the development of AI and computer vision technology. Digitalized WSIs facilitate the development of computer-aided diagnostic tools through AI applications of intelligent behavior modeled by machines [7].
Machine learning (ML) is a subfield within AI that develops algorithms and technologies. In 1959, Arthur Samuel defined ML as a “field of study that gives computers the ability to learn without being explicitly programmed” [8]. Artificial neural networks (ANNs) are a statistical learning algorithm inspired by biological neural networks such as the human neural architecture in ML. ANN refers to a general model of artificial neurons (nodes) that form a network by synapse binding and have the ability to solve problems by changing synapse strength through learning [9]. Deep learning (DL), a particular approach of ML, comprises multiple layers of neural networks that usually include an input layer, an output layer, and multiple hidden layers [10]. Convolutional neural networks (CNNs) are a type of deep, feedforward ANN used to analyze visual images. CNN is classified as a DL algorithm that is most commonly applied to image analysis [11]. Successful computer-aided pathologic diagnostic tools are being actively devised using AI techniques, particularly DL models [12].
DP covers all pathologic activities using digitalized pathologic images generated by digital scanners, and encompasses the primary diagnosis on the computer monitor screen, consultation by telepathology, morphometry by image analysis software, multidisciplinary conferences and student education, quality assurance activities, and enhanced diagnosis by CAP.
Recently, primary diagnosis on computer monitor screens using digitalized pathologic images has been practically approved by the Food and Drug Administrations (FDA) of the United States of America, the European Union, and Japan [13-15]. Dozens of validation studies have compared the diagnostic accuracy of DP and conventional microscopic diagnosis during the last decade [5,16]. Although most studies have demonstrated no inferiority of the diagnostic accuracy of whole slide imaging compared to conventional microscopy, study sample sizes were mostly limited, and the level of evidence was not high enough (only level III and IV). Therefore, an appropriate internal validation study for diagnostic concordance between whole slide imaging and conventional microscopic diagnosis should be performed before implementing DP into individual laboratories according to the guidelines suggested by major study groups and leading countries.
Telepathology primarily indicates a system that enables pathologic diagnosis by transmitting live pathology images through online connections using a microscopic system with a remotecontrolled, motorized stage. The limited meaning of “telediagnosis” can be used in certain clinical situations in which a pathologic diagnosis is made in a remote facility without pathologists. Due to recent advances in whole slide imaging technology, faster and more accurate acquisition and sharing of high quality digital images is now possible. Telepathology has evolved so that DP data can be easily used, shared, and exchanged on various systems and devices using cloud systems. Because of this ubiquitous accessibility, network security and deidentification of personal information have never been more important [13].
Morphometric analysis and CAPD techniques will be further accelerated by implementation of DP. Ki-67 labeling index is traditionally considered one of the most important prognostic markers in breast cancer, and various image analysis software programs based on ML have been developed for accurate and reproducible morphometric analysis. However, DL is more powerful for more complex pathologic tasks such as mitosis detection for breast cancers, microtumor metastasis detection in sentinel lymph nodes, and Gleason scoring for prostate biopsies. Furthermore, DP facilitates the use of DL in pathologic image analysis by providing an enormous source of training data.
DP is also a new opportunity for life-changing advances in education and multidisciplinary conferences. It enables easy sharing of pathologic data to simplify preparation of education and conference materials. DP mostly uses laboratory automation systems and tracking identification codes, which reduces potential human errors and contributes to patient safety. DP also simplifies pathologic review of archived slides. By adopting CAPD tools based on DL to review diagnosis, quality assurance activities can be performed quickly and with less effort. DL can be used to assess diagnostic errors and the staining quality of each histologic slide.
When combined with other medical information, such as the EMR, hospital information system (HIS), public health information and resources, medical imaging data systems like PACS, and genomic data such as next-generation sequencing, DP provides the basis for revolutionary innovation in medical technology.
Recent technological advances in WSI systems have accelerated the implementation of digital pathology system (DPS) in pathology. The use of WSI for clinical purposes includes primary diagnosis, expert consultation, intraoperative frozen section consultation, off-site diagnosis, clinicopathologic conferences, education, and quality assurance. The DPS for in vitro diagnostic use comprises whole digital slide scanners, viewing and archiving management systems, and integration with HIS and LIS. Image viewing software includes image analysis systems. Pathologists interpret WSIs and render diagnoses using the DPS set up with adequate hardware, software, and hospital networks.
Most recent WSI scanners permit high-speed digitization of whole glass slides and produce high-resolution WSI. However, there are still differences in scanning time, scan error rate, image resolution, and image quality among WSI scanners. WSI scanners differ with respect to their functionality and features, and most image viewers are provided by scanner vendors [4]. When selecting a WSI scanner for clinical diagnosis, it is important to consider the following factors: (1) volume of slides, (2) type of specimen (eg, tissue section slides, cytology slides, or hematopathology smears), (3) feasibility of z-stack scanning (focus stacking), (4) laboratory needs for oil-immersion scanning, (5) laboratory needs for both bright field and fluorescence scanning, (6) type of glass slides (e.g., wet slides, unusual size), (7) slide barcode readability, (8) existing space constraints in the laboratory, (9) functionality of image viewer and management system provided by vendor, (10) bidirectional integration with existing information systems, (11) communication protocol (e.g., XML, HL7) between DPS and LIS, (12) whether image viewer software is installed on the server or on the local hard disk of each client workstation, (13) whether the viewer works on mobile devices, and (14) open or closed system.
It is crucial to fully integrate the WSI system into the existing LIS to implement DPS in the workflow of a pathology department and decrease turn-around-time [14]. Therefore, information technology (IT) support is vital for successful implementation of DPS. Pathologists should work closely with IT staff, laboratory technicians, and vendors to integrate the DPS with the LIS and HIS. The implementation team should meet regularly to discuss progress on action items and uncover issues that could slow or impede progress.
Resistance to digital transformation can come from any level in the department. Documented processes facilitate staff training and allow smooth onboarding. Regular support and training should be provided until all staff understand the value of DPS and perform their tasks on a regular basis.
The earliest attempt at DP, so-called cell segmentation, detected cells via nuclei, cytoplasm, and structure. Because the cells are the basic units of histopathology images, identification of the color, intensity, and morphology of nuclei and cytoplasm through cell segmentation is the first and most important step in image analysis. Cell segmentation has been tried in immunohistochemistry (IHC) analysis and hematoxylin and eosin (H&E) staining and is a fundamental topic for computational image analysis to achieve quantitative histopathologic representation.
A number of cell segmentation algorithms have been developed for histopathologic image analysis [17,18]. Several classical ML studies reported that cellular features of H&E staining, nuclear and cytoplasmic texture, nuclear shape (e.g., perimeter, area, tortuosity, and eccentricity) and nuclear/cytoplasmic ratio carry prognostic significance [19-22]. Cell segmentation algorithms have usually been studied in IHC, which has a relatively simple color combination and limited analysis color channel compared to H&E staining [23]. For example, IHC staining for estrogen receptor (ER), progesterone receptor (PR), human epidermal growth factor receptor 2 (HER2), and Ki-67 is routinely performed in breast cancer diagnostics to determine adjuvant treatment strategy and predict prognosis [24,25]. Automated scoring algorithms for this IHC panel are the most developed and most commonly used, and some are FDA-approved [26-28]. Those automated algorithms are superior alternatives to manual biomarker scoring in most aspects. Many workflow steps can be automated or executed without pathologic expertise to reduce the use of a pathologist’s time.
Conventional ML algorithms for cell segmentation utilize combinations of median filtering, thresholds, watershed segmentation, contour models, shape dictionaries, and categorizing [26]. It is often necessary to adjust detailed settings when using these algorithms to avoid under- and over-segmentation (Fig. 1). Nuclear staining (e.g., ER, PR, and Ki-67) and membranous staining (e.g., HER2) clearly shows the boundary between nuclei or cells, whereas cytoplasmic staining has no clear cell boundary, limiting algorithm development. However, the recent development of technology using DL has resulted in algorithms showing better performance [29,30]. Quantitative analysis has recently been included in various tumor diagnosis, grading, and staging criteria. Neuroendocrine tumor grading requires a distinct mitotic count and/or Ki-67 labeling index [31,32]. With recent clinical applications of targeted therapy and immunotherapy, quantification of various biomarkers and tumor microenvironmental immune cells has become important [33-35]. Therefore, accurate cell segmentation algorithms will play an important role in diagnosis, predicting prognosis, and determining treatment strategy.
Color normalization is a standardization or scaling procedure often used in the data processing phase in preparation for ML. The quality of H&E stained slides varies by institution and is affected by dye concentration, staining time, formalin fixation time, freezing, cutting skill, type of glass slide, and fading color after staining (Fig. 2). H&E stained slides also show diversity within the same institution (Fig. 2A, D). The color of each slide is also affected by the slide scanner and settings. If excessive staining variability is present in a dataset, application of a threshold may produce different results due to different staining or imaging protocols rather than due to unique tissue characteristics. Therefore, color normalization for such algorithms improves their overall performance. A number of color normalization approaches have been developed that utilize intensity thresholds, histogram normalization, stain separation, color deconvolution, structurebased color classification, and generative adversarial networks [36-41]. However, it is important to evaluate and prevent the image distortion that can result from these techniques.
Pathologists play an important role in guiding algorithm development. Pathologists have expertise in the clinicopathologic purpose of algorithm development, technical knowledge of tissue processing, selecting data for use in an algorithm, and validating the quality of generated output to produce useful results. Region of interest (ROI) selection for algorithm development is usually performed by a pathologist. Depending on the subject, various ROI size assignments may be required. Finely defined ROIs allow an algorithm to focus on specific areas or elements, resulting in faster and more accurate output. Annotation is the process of communicating about ROIs with an algorithm. Annotations allow the algorithm to know that a particular slide area is important and focus on it for analysis. Annotation includes manual and automatic methods. In addition to ensuring the quality of annotations, pathologists can gain valuable insight into slide data and discover specific problems or potential pitfalls to consider during algorithm development.
Manual annotation involves drawing on the slide image with digital dots, lines, or faces to indicate the ROI of the algorithm. Both inclusion and exclusion annotations may be present depending on the algorithm analysis method. Inclusion and exclusion annotations may target necrosis, contamination, and any other type of artifact. However, manual annotation is costly and timeconsuming because it must be performed by skilled technicians with confirmation and approval by a pathologist. Various tools have been developed to overcome the shortcomings of manual annotations, including automated image annotation and predeveloped commercial software packages [42]. Because these tools may compromise accuracy, a pathologist must confirm the accuracy of automated annotations. Recently, several studies have tried to overcome the difficulties of the annotation step through weakly supervised learning from the label data of slides or mixing label data with detailed annotation data [43]. Crowdsourcing is also used, which involves engaging many people, including nonprofessionals, using web-based tools. Crowdsourcing has been used successfully for several goals [44,45]. While it is cheaper and faster than expert pathologist annotations, it is also more error-prone. Some detailed pathologic analyzes are available only to well-trained professionals.
Pathologists play an important role in CAPD tool development, from the data preparation stage to review of interpretations generated by the algorithm. Pathologists should play a role in identifying and resolving problems in every process of the algorithm during development [46]. If biomarker expression analysis via staining intensity is included in CAPD tool development, the final algorithm threshold should be reviewed and approved by a pathologist before each data generation. For example, pathologists also confirm and advise whether cells or other structures are correctly identified and enumerated, whether the target tissue can be correctly identified and analyzed separately from other structures, and whether staining intensity is properly classified. The algorithm does not have to be performed with 100% precision and accuracy, but a reasonable level of performance must be met that is relevant to the general goals of the analysis. Clinical studies that inform treatment decisions or prognosis may need to meet more stringent criteria for accurate classification, including staining methods and types of scanners. Certain samples that do not meet these predetermined criteria should be detected and excluded from the algorithm.
When the test CAPD tool meets the desired performance criteria, validation can be performed on a series of slides. Pathologists should review the resulting data derived from the algorithm to examine the CAPD tool’s performance. Analysis review should inform general questions, data interrogated through image analysis, and clinical impact on patients. Only results from CAPD tools that have passed pathologist and general performance criteria should be included in the final step. Quality assurance at different stages of slide creation is important to achieve optimal value in image analysis. Thus, humans are still required for quality assurance of digital images before they are processed by image analysis algorithms. Similarly, the technical aspects of slide digitization can affect the results of digital images and final image analysis. Color normalization techniques can help to solve these problems.
To clinically use a CAPD tool, it is important to know the intended use of the algorithm and ensure that the CAPD tool is properly validated for specific tissue conditions, such as frozen tissue, formalin-fixed tissue, or certain types of organs. In the future, commercial solutions for CAP will be available in the clinical field. However, CAPD tools must be internally validated by pathologists at each institution before they are used for clinical practice.
As many have already pointed out, the current AI-based CAP has several limitations. Tizhoosh and Liron have listed a number of problems that CAP should address, including vulnerability to adversarial attacks, capability limited to a certain diagnostic task, obscured diagnostic process, and lack of interpretability [47]. Specifically, lack of interpretability is unacceptable to the medical society. Other literature lists practical issues which may apply to pathologic AI: slow execution time of CAP, opacity of CAPD development process, insufficient clinical validation of CAP, and limited impact on health economics [48]. Another substantial issue mentioned was frequent workflow switching induced by limited integration of CAPD applications within the current pathology workflow. Beyond disease detection and grading, CAP should be able to provide an integrated diagnosis with a number of analyses on various data [49]. Rashidi et al. [50] emphasized the role of pathologists in developing CAPD tools, especially in dealing with CAP performance problems caused by lack of data and model limitations. These limitations can be summarized as follows: (1) lack of confidence in diagnosis results, (2) inconvenience in practical use, and (3) simplicity of function (Table 1). These limitations can be overcome by close collaboration among AI researchers, software engineers, and medical experts.
Confidence in diagnostic results can be improved by formalizing the CAP development and validation processes, expanding the size of validation data, and enhancing understanding of the CAPD.
CAPD products can only enter the market as medical devices with the approval of regulatory agencies. The approval process for medical devices requires validation of the medical device development process, including validation of CAPD algorithms if applicable [51]. It is notable that regulatory agencies are already preparing for regulation of adaptive medical devices that learn from information acquired in operation. To formalize the validation process, it is desirable to assess the performance change of the CAP based on input variation induced by several factors, such as quality of tissue samples, slide preparation process, staining protocol, and slide scanner characteristics. This is similar to the validation process for existing in vitro diagnostic devices such as reagents. Validation data that covers diverse demographics is desirable. The use of validation data covering multiple countries and institutions increases the credibility of validation results [43]. Regulatory agencies require data from two or more medical institutions in clinical trials for medical device approval, so CAPDs introduced in the market as approved medical devices should be sufficiently validated.
The ability to explain CAP is a hot topic in current AI research. According to a recently published book on the interpretability of ML, which comprise the majority of current AI, interpretation approaches to complex ML models are largely divided into two categories: visualization of important features in the decision process and example-based interpretation of decision output [52]. Important feature visualization in ML models includes Visualizing Activation Layers [53], SHAP [54], Grad-CAM [55], and LIME [56]. These methods can be used to interpret a model’s decision process by identifying parts of the input that have played a role. In applications to pathologic image analysis, an attention mechanism was used to visualize epithelial cell areas [57]. Arbitrary generated counterfactual examples can be used to describe how input change affects to model output [58]. Case-based reasoning [59,60] can be combined with the interpretation target model to demonstrate consistency between model output and reasoning output of the same input [61]. Similar image search can also be used to show that AI decisions are similar to human annotations on the same pathologic image, and systems such as SMILY can be used for this purpose [62].
Inconvenience in practical use can be solved by accelerating execution of AI and integrating it with other existing IT systems in medical institutions. In addition to hardware that provides sufficient computing power, software optimization must be achieved to accelerate AI execution. This includes removal of data processing bottlenecks and data access redundancy as well as model size reduction. Medical IT systems include HIS, EMR, and PACS, which are integrated with each other and operate within a workflow. CAPD systems, digital slide scanners, and slide IMS should participate in this integration so they can be easily utilized in a real-world workflow. The problem of CAPD systems being limited to specific data and tasks can be relieved by adding more data and more advanced AI technologies, including multi-task learning, continual learning, and reinforcement learning.
DP and CAP are revolutionary for pathology. If used properly, DP and CAP are expected to improve convenience and quality in pathology diagnosis and data management. However, a variety of challenges remain in implementation of DPS, and many aspects must be considered when applying CAP. Implementation is not limited to the pathology department and may involve the whole institution or even the entire healthcare system. CAP is becoming clinically available with the application of DL, but various limitations remain. The ability to overcome these limitations will determine the future of pathology.
Notes
Author contributions
Conceptualization: SN, YC, CKJ, TYK, HG.
Funding acquisition: JYL.
Investigation: SN, YC, CKJ, TYK, JYL, JP, MJR, HG.
Supervision: HG.
Writing—original draft: SN, YC, CKJ, TYK, HG.
Writing—review & editing: SN, YC, CKJ, TYK, HG.
REFERENCES
1. Bera K, Schalper KA, Rimm DL, Velcheti V, Madabhushi A. Artificial intelligence in digital pathology: new tools for diagnosis and precision oncology. Nat Rev Clin Oncol. 2019; 16:703–15.
2. Abels E, Pantanowitz L, Aeffner F, et al. Computational pathology definitions, best practices, and recommendations for regulatory guidance: a white paper from the Digital Pathology Association. J Pathol. 2019; 249:286–94.


3. he j, baxter sl, xu j, xu j, zhou x, zhang k. the practical implementation of artificial intelligence technologies in medicine. nat med. 2019; 25:30–6.


4. Evans AJ, Salama ME, Henricks WH, Pantanowitz L. Implementation of whole slide imaging for clinical purposes: issues to consider from the perspective of early adopters. Arch Pathol Lab Med. 2017; 141:944–59.


5. Mukhopadhyay S, Feldman MD, Abels E, et al. Whole slide imaging versus microscopy for primary diagnosis in surgical pathology: a multicenter blinded randomized noninferiority study of 1992 cases (pivotal study). Am J Surg Pathol. 2018; 42:39–52.
6. Niazi MK, Parwani AV, Gurcan MN. Digital pathology and artificial intelligence. Lancet Oncol. 2019; 20:e253–61.


8. Simon P. Too big to ignore: the business case for big data. Hoboken: John Wiley & Sons;2013.
9. Yao X. Evolving artificial neural networks. Proc IEEE. 1999; 87:1423–47.
10. Deng L, Yu D. Deep learning: methods and applications. Found Trends Signal Process. 2014; 7:197–387.


11. Collobert R, Weston J. A unified architecture for natural language processing: deep neural networks with multitask learning. In : Proceedings of the 25th International Conference on Machine Learning; 2008 Jul 5-9; Helsinki, Finland. New York: Association for Computing Machinery;2008. 160–7.


12. Wang S, Yang DM, Rong R, et al. Artificial intelligence in lung cancer pathology image analysis. Cancers (Basel). 2019; 11:E1673.


13. Garcia-Rojo M. International clinical guidelines for the adoption of digital pathology: a review of technical aspects. Pathobiology. 2016; 83:99–109.


14. Hanna MG, Reuter VE, Samboy J, et al. Implementation of digital pathology offers clinical and operational increase in efficiency and cost savings. Arch Pathol Lab Med. 2019; 143:1545–55.


15. Hanna MG, Pantanowitz L, Evans AJ. Overview of contemporary guidelines in digital pathology: what is available in 2015 and what still needs to be addressed? J Clin Pathol. 2015; 68:499–505.


16. Snead DR, Tsang YW, Meskiri A, et al. Validation of digital pathology imaging for primary histopathological diagnosis. Histopathology. 2016; 68:1063–72.


17. Irshad H, Veillard A, Roux L, Racoceanu D. Methods for nuclei detection, segmentation, and classification in digital histopathology: a review-current status and future potential. IEEE Rev Biomed Eng. 2014; 7:97–114.


18. Wang S, Yang DM, Rong R, Zhan X, Xiao G. Pathology image analysis using segmentation deep learning algorithms. Am J Pathol. 2019; 189:1686–98.


19. Gurcan MN, Pan T, Shimada H, Saltz J. Image analysis for neuroblastoma classification: segmentation of cell nuclei. Conf Proc IEEE Eng Med Biol Soc. 2006; 1:4844–7.


20. Ballaro B, Florena AM, Franco V, Tegolo D, Tripodo C, Valenti C. An automated image analysis methodology for classifying megakaryocytes in chronic myeloproliferative disorders. Med Image Anal. 2008; 12:703–12.
21. Korde VR, Bartels H, Barton J, Ranger-Moore J. Automatic segmentation of cell nuclei in bladder and skin tissue for karyometric analysis. Anal Quant Cytol Histol. 2009; 31:83–9.


22. Veta M, van Diest PJ, Kornegoor R, Huisman A, Viergever MA, Pluim JP. Automatic nuclei segmentation in H&E stained breast cancer histopathology images. PLoS One. 2013; 8:e70221.
23. Ruifrok AC, Katz RL, Johnston DA. Comparison of quantification of histochemical staining by hue-saturation-intensity (HSI) transformation and color-deconvolution. Appl Immunohistochem Mol Morphol. 2003; 11:85–91.


24. Flanagan MB, Dabbs DJ, Brufsky AM, Beriwal S, Bhargava R. Histopathologic variables predict Oncotype DX recurrence score. Mod Pathol. 2008; 21:1255–61.


25. Hammond ME, Hayes DF, Dowsett M, et al. American Society of Clinical Oncology/College of American Pathologists guideline recommendations for immunohistochemical testing of estrogen and progesterone receptors in breast cancer (unabridged version). Arch Pathol Lab Med. 2010; 134:e48–72.
26. Al-Kofahi Y, Lassoued W, Lee W, Roysam B. Improved automatic detection and segmentation of cell nuclei in histopathology images. IEEE Trans Biomed Eng. 2010; 57:841–52.


27. stalhammar g, fuentes martinez n, lippert m, et al. digital image analysis outperforms manual biomarker assessment in breast cancer. mod pathol. 2016; 29:318–29.


28. Zarrella ER, Coulter M, Welsh AW, et al. Automated measurement of estrogen receptor in breast cancer: a comparison of fluorescent and chromogenic methods of measurement. Lab Invest. 2016; 96:1016–25.


29. Madabhushi A, Lee G. Image analysis and machine learning in digital pathology: Challenges and opportunities. Med Image Anal. 2016; 33:170–5.


30. Williams BJ, Bottoms D, Treanor D. Future-proofing pathology: the case for clinical adoption of digital pathology. J Clin Pathol. 2017; 70:1010–8.


31. Kloppel G, La Rosa S. Ki67 labeling index: assessment and prognostic role in gastroenteropancreatic neuroendocrine neoplasms. Virchows Arch. 2018; 472:341–9.


32. Chai SM, Brown IS, Kumarasinghe MP. Gastroenteropancreatic neuroendocrine neoplasms: selected pathology review and molecular updates. Histopathology. 2018; 72:153–67.


33. Mok TS, Wu YL, Kudaba I, et al. Pembrolizumab versus chemotherapy for previously untreated, PD-L1-expressing, locally advanced or metastatic non-small-cell lung cancer (KEYNOTE-042): a randomised, open-label, controlled, phase 3 trial. Lancet. 2019; 393:1819–30.
35. Yang Y. Cancer immunotherapy: harnessing the immune system to battle cancer. J Clin Invest. 2015; 125:3335–7.


36. Khan AM, Rajpoot N, Treanor D, Magee D. A nonlinear mapping approach to stain normalization in digital histopathology images using image-specific color deconvolution. IEEE Trans Biomed Eng. 2014; 61:1729–38.


37. Bejnordi BE, Litjens G, Timofeeva N, et al. Stain specific standardization of whole-slide histopathological images. IEEE Trans Med Imaging. 2016; 35:404–15.
38. Vahadane A, Peng T, Sethi A, et al. Structure-preserving color normalization and sparse stain separation for histological images. IEEE Trans Med Imaging. 2016; 35:1962–71.


39. Zarella MD, Yeoh C, Breen DE, Garcia FU. An alternative reference space for H&E color normalization. PLoS One. 2017; 12:e0174489.
40. Miyato T, Kataoka T, Koyama M, Yoshida Y. pectral normalization for generative adversarial networks. Preprint at: https://arxiv.org/abs/1802.05957 (2018).
41. Shaban MT, Baur C, Navab N, Albarqouni S. StainGAN: stain style transfer for digital histological images. Preprint at: https://arxiv.org/abs/1804.01601 (2018).


42. Aeffner F, Zarella MD, Buchbinder N, et al. Introduction to digital image analysis in whole-slide imaging: a white paper from the Digital Pathology Association. J Pathol Inform. 2019; 10:9.


43. Campanella G, Hanna MG, Geneslaw L, et al. Clinical-grade computational pathology using weakly supervised deep learning on whole slide images. Nat Med. 2019; 25:1301–9.


44. Albarqouni S, Baur C, Achilles F, Belagiannis V, Demirci S, Navab N. AggNet: deep learning from crowds for mitosis detection in breast cancer histology images. IEEE Trans Med Imaging. 2016; 35:1313–21.


45. Irshad H, Montaser-Kouhsari L, Waltz G, et al. Crowdsourcing image annotation for nucleus detection and segmentation in computational pathology: evaluating experts, automated methods, and the crowd. Pac Symp Biocomput. 2015; 294–305.


46. Aeffner F, Wilson K, Bolon B, et al. Commentary: roles for pathologists in a high-throughput image analysis Team. Toxicol Pathol. 2016; 44:825–34.
47. Tizhoosh HR, Pantanowitz L. Artificial intelligence and digital pathology: challenges and opportunities. J Pathol Inform. 2018; 9:38.


48. Serag A, Ion-Margineanu A, Qureshi H, et al. Translational AI and deep learning in diagnostic pathology. Front Med (Lausanne). 2019; 6:185.


49. Chang HY, Jung CK, Woo JI, et al. Artificial intelligence in pathology. J Pathol Transl Med. 2019; 53:1–12.


50. Rashidi HH, Tran NK, Betts EV, Howell LP, Green R. Artificial intelligence and machine learning in pathology: the present landscape of supervised methods. Acad Pathol. 2019; 6:2374289519873088.


51. U.S. Food and Drug Administration. Artificial intelligence and machine learning in software as a medical device. Silverspring: U.S. Food and Drug Administration;2019.
52. Molnar C. Interpretable machine learning [Internet]. The Author, 2018 [cited 2019 Nov 2]. Available from: https://christophm.github.io/interpretable-ml-book/.
53. Kahng M, Andrews PY, Kalro A, Polo Chau DH. ACTIVIS: visual exploration of industry-scale deep neural network models. IEEE Trans Vis Comput Graph. 2018; 24:88–97.


54. Lundberg SM, Lee SI. A unified approach to interpreting model predictions. In : Advances in Neural Information Processing Systems. Proceedings of the 31st Neural Information Processing Systems (NIPS 2017); 2017 Dec 4-9; Long Beach, CA, USA. Red Hook: Curran Associates Inc;2017. 4765–74.
55. Selvaraju RR, Cogswell M, Das A, Vedantam R, Parikh D, Batra D. Grad-CAM: visual explanations from deep networks via gradientbased localization. Int J Comput Vision. 2019 Oct 11 [Epub]. https:// doi.org/10.1007/s11263-019-01228-7.


56. Ribeiro MT, Singh S, Guestrin C. Why should I trust you? Explaining the predictions of any classifier. New York: Association for Computing Machinery;2016. p. 1135–44.
57. Ilse M, Tomczak JM, Welling M. Attention-based deep multiple instance learning. In : Proceedings of the 35th International Conference on Machine Learning; 2018 Jul 10-15; Stockholm, Sweden. Cambridge: Proceedings of Machine Learning Research;2018. 3376–91.
58. Mothilal RK, Sharma A, Tan C. Explaining machine learning classifiers through diverse counterfactual explanations. Preprint at: https://arxiv.org/abs/1905.07697 (2019).


59. Richter MM, Weber RO. Case-based reasoning: a textbook. Berlin: Springer-Verlag;2013. p. 546.
61. Keane MT, Kenny EM. How case based reasoning explained neural networks: an XAI survey of post-hoc explanation-by-example in ANN-CBR twins. Preprint at: https://arxiv.org/abs/1905.07186 (2019).
Fig. 1.
Under- (A) and over-nuclear (B) nuclear segmentation. Under- and over-nuclear segmentation results in ImageJ (open-source tool). Immunohistochemical staining images for Ki-67 antibody shows nuclear presentation. As the settings for range of diameter, area eccentricity and staining intensity change, segmentation algorithm shows different results. (A) algorithm failed to detect some positive nuclei. (B) algorithm showed that too many small non-specific stains were counted in the positive nucleus, resulting in over-nuclear counting. As a result, the count of (A) algorithm was 88, and the count of (B) algorithm was 130.
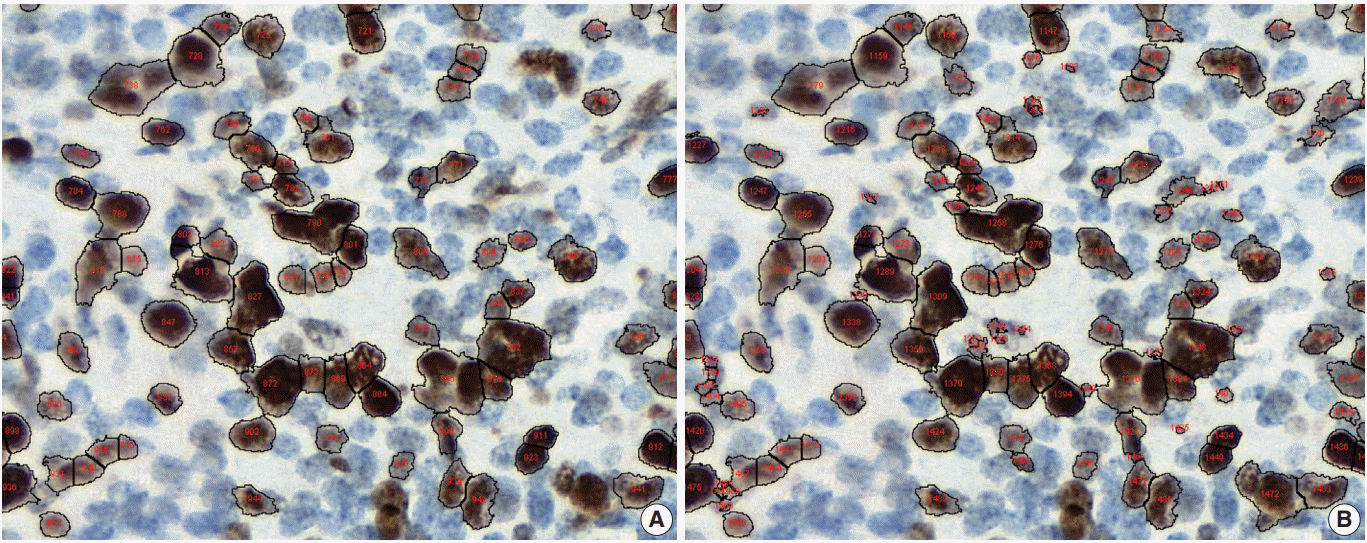
Fig. 2.
Various hematoxylin and eosin (H&E) staining color attributes. (A–F) Six H&E staining images for colonic mucosa represent color properties that usually occur in various laboratory settings or image scanning methods. Panels A and D were stained in the same laboratory.
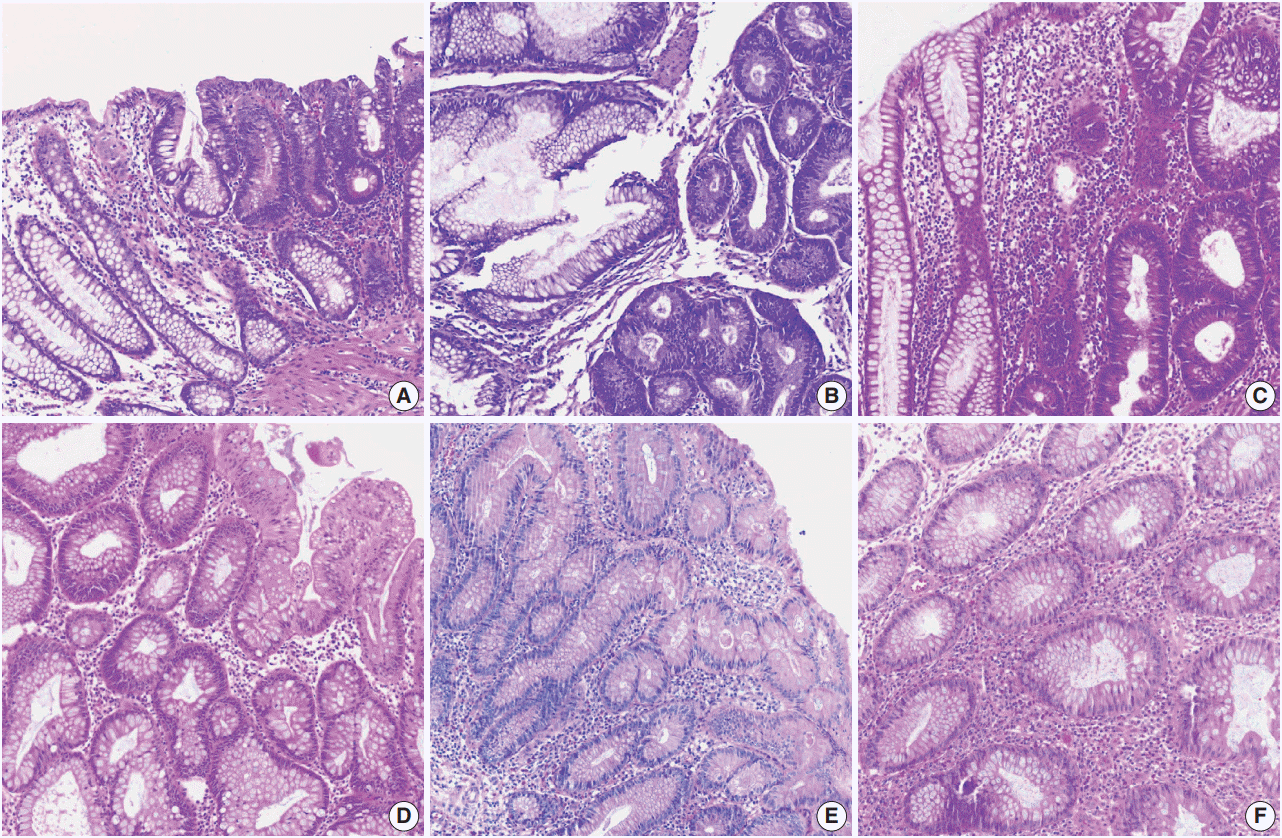
Table 1.
Current limitations of AI-based computer-assisted pathological diagnosis