Abstract
Background
Lymphocyte subset analysis is essential to evaluate the engraftment status in hematopoietic stem cell transplantation (HSCT). Automated gating tools are widely used for flow cytometry analysis. Unlike healthy individuals, different cell populations and aberrant expressions may occur in HSCT samples. In the present study, we evaluated the applicability of automated gating in HSCT recipients by comparing it to expert-based manual gating.
Methods
Lymphocyte subset was performed using Beckman Coulter Navios (Beckman Coulter, USA) flow cytometry. Data files from 22 patients with hematologic malignancies were analyzed in parallel by manual gating and automated gating using Navios Tetra software. Quality control results and reproducibility were evaluated using IMMUNO-TROL controls.
Results
Spearman rank correlation coefficients between the two gating methods were >0.970 in all cell populations except CD8+ T cells. CD8+ T cell counts via automated gating were higher than those of manual gating in all cases due to the T cell populations with reduced CD8 expression. Automated gating program failed to identify CD4+CD8+ double-positive T cell population. Moreover, it excluded certain lymphocytes with low forward scatter (FSC) and high side scatter (SSC). Furthermore, two HSCT recipients revealed a high percentage of CD56−CD16+ NK cells, we found the need to add CD16 reagent to the Navios system. All coefficients of variation were <10% except for CD56+ NK cells via automated gating.
초록
배경
림프구 아형 검사는 조혈모세포 이식 환자에서 생착을 평가하는데 필수적인 검사이다. 자동 게이팅은 현재 유세포분석에서 널리 사용되고 있다. 건강인과 달리 조혈모세포 이식 환자는 예상치 못한 세포군이나 세포의 비특이적인 발현이 예상될 수 있다. 따라서 본 연구에서는 조혈모세포 이식 환자의 림프구 아형 검사에서 자동 게이팅의 적용 가능성을 수동 게이팅과 비교해서 평가했다.
방법
림프구 아형 검사는 Beckman Coulter Navios (Beckman Coulter, USA) 유세포분석기로 시행하였다. 22명의 혈액 종양 환자의 데이터 파일을 Navios Tetra 소프트웨어를 사용하여 수동 게이팅과 자동 게이팅으로 각각 분석했다. 정도관리와 재현성 평가는 IMMUNO-TROL control을 사용했다.
결과
두 게이팅 방법 사이의 Spearman 상관계수는 CD8+ T 세포를 제외한 모든 세포 집단에서 0.970 이상이었다. 자동 게이팅에서 CD8+ T 세포 수는 수동 게이팅보다 더 높았는데, 이는 CD8 low-expressing T 세포가 자동 게이팅에서만 CD8+ T 세포에 포함되었기 때문이었다. 자동 게이팅 프로그램은 CD4+CD8+ double-positive T 세포를 식별하지 못했다. 자동 게이팅은 림프구 중 FSC가 낮고 SSC가 높은 일부 부분을 제외시키고 식별했다. 두 명의 조혈모세포 이식 환자는 CD56-CD16+ NK 세포의 비율이 높았고 이를 위해서 Navios 시스템에 CD16 시약을 추가해야 했다. 림프구 아형 검사의 변이계수는 자동 게이팅에 의한 CD56+ NK 세포를 제외하고, 모두 10% 미만이었다.
Peripheral blood lymphocyte subsets have been routinely assessed via flow cytometry to define immune reconstitution in the post-hematopoietic stem cell transplantation (HSCT) period [1, 2]. Assessment of immune recovery is important to ensure successful engraftment, monitor the risk of infection or rejection, and appropriately guide interventions [3, 4]. Unlike healthy individuals, lymphocytes in HSCT recipients are presumed to exhibit aberrant phenotype expression or rare cell populations because recovery of the functional immune system is slow and often incomplete [5].
Gating in flow cytometry analysis refers to generation of reportable data such as cell counts, percentages, and mean fluorescence intensity (MFI), which is essential for maintaining the data consistency and improving reproducibility of flow cytometry data [6]. Traditional manual gating was performed via sequential manual process by experts; therefore, it is time-consuming and highly subjective [7]. Manual gating is performed based on a visual comparison of plots; moreover, determination of gating area boundaries is subjective as it is not guided by rules. Furthermore, as gating majorly works on two-dimensional plots, the information present in the multidimensional space can be missing [8, 9]. Major technological advances in flow cytometry have resulted in an increased number of parameters and cells to be analyzed, thereby leading to the development of automated gating algorithms and tools [10-12]. Automated gating overcomes the limitation of sequential gating and automatically deduces cell populations directly from the multidimensional datasets [7, 8]. Recently, several programs are widely applied in the clinical field [11-13]; however, each new algorithm has been evaluated using separate data sets and methods. Additionally, the methodology to compare the results among these approaches and to traditional manual gating, remains unclear [14].
To control the quality of lymphocyte subsets, appropriate gating technique must be used in order to select only the desired cells and the analysis needs to be appropriately calibrated based on the gate purity. The present study aimed to confirm the applicability of automated gating by comparing it to manual gating in HSCT recipients.
Peripheral blood samples from 22 patients with hematologic malignancies were included. Of these, 20 patients received HSCT. All samples were retrieved from the blood samples routinely requested for lymphocyte subset analysis at the laboratory of Seoul St. Mary’s Hospital. For the precision study, between-run variability was measured using IMMUNO-TROL cells and IMMUNO-TROL low cells (Beckman Coulter, Miami, FL, USA) on 10 different days. Both cell controls were analyzed in a similar manner as the patient samples. Informed consent was waived by the Institutional Review Board of Seoul St. Mary’s Hospital (KC18SESI0870) because the present study used leftover samples.
Peripheral blood was collected in tubes containing K2EDTA (Becton/Dickinson, San Jose, CA). For each sample, cells were stained using two ready-to-use mixtures of antibodies: CD45-FITC/CD4-RD1/CD8-ECD/CD3-PC5 (tube 1) and CD45-FITC/CD56-RD1/CD19-ECD/CD3-PC5 (tube 2) (Beckman Coulter, USA). Thereafter, the samples were evaluated via Navios flow cytometer (Beckman Coulter, USA) by analyzing at least 5,000 events within the lymphocyte gate. The following lymphocyte populations were enumerated by manual and automated gating: CD3+ T cells, CD3+CD4+ T cells (CD4+ T cells), CD3+CD8+ T cells (CD8+ T cells), CD3−CD19+ B cells (CD19+ B cells), and CD3−CD56+ NK cells (CD56+ NK cells). In some specific cases, CD8 low-expressing T cells, CD4+CD8+ double-positive T cells, and CD56−CD16+ NK cells were enumerated via manual gating. IMMUNO-TROL cells and IMMUNO-TROL low cells were assessed daily for internal quality control. Staining and data acquisition were processed within 24 hours after blood collection.
All data files were analyzed automatically using Navios Tetra software (Beckman Coulter) and manually using the KALUZA program (Beckman Coulter) that focused on the lymphocyte population. All automated gating results were visually inspected, but no active intervention was allowed. When the flags and run notifications were presented on the result screen, we reviewed the results. Manual gating was performed by an experienced lab technician and further verified by a medical supervisor. To ensure tube-to-tube consistency, we limited the difference in CD3+ T cell counts between tube 1 and tube 2 to ≤2% as recommended in the previous guideline for performing single-platform absolute CD4+ T cell determinations [15]. The gating purity was adjusted to the criterion stating that the sum of CD3+ T cells, CD19+ B cells, and CD56+ NK cells exceeded 95%.
The absolute percentage difference for each cell population obtained from automated and manual gating strategies was compared. Spearman rank correlation was used to compare the results. The interlaboratory variability was calculated by the coefficient of variation (CV) for each cell population using both gating strategies. Statistical analyses were performed using MedCalc Statistical Software version 19.6 (MedCalc Software Ltd, Ostend, Belgium).
In total, 22 blood samples were compared via gating (Table 1). The median difference of cell percentages between automated and manual gating was <1% in CD3+ T cells, CD4+ T cells, CD19+ B cells, and CD56+ NK cells. Spearman rank correlation coefficients (Spearman r) between automated and manual gating was >0.970 (P<0.001) for all cell populations, except for CD8+ T cells (Spearman r=0.800, P<0.001; Table 2). Moreover, the number of CD8+ T cells analyzed via automated gating was higher than that obtained by manual gating in all cases (Y [automated gating result]=9.504+0.925 X [manual gating result]), r=0.862, P<0.001; Fig. 1A). Of these, four cases (18.2%) revealed questionable CD8+ T cell results in automated gating analysis. One case (case no. 18) failed to identify the CD8+ T cell population in an automated program, and three cases (case nos. 6, 15, and 16) exhibited a difference of 20% or more in the CD8+ T cell counts. After reviewing the plots, we found that the CD8+ T cells with reduced CD8 expression (CD8 low-expressing T cell) were included as CD8+ T cells in the automated gating program (Fig. 1B). In manual gating analysis, cells expressing high levels of CD8 intensity were considered as CD8+ (Fig. 1C). Four cases with abnormal CD8+ T cell results failed the compensation process in automated gating due to dim fractions of CD8 (Fig. 2); however, manual gating approach could confirm the exact CD8+ T cell counts in these cases.
We detected a sample (case no. 13) exhibiting the difference in CD4+ T cell results between the two gating strategies. This sample comprises 5% of CD4+CD8+ double-positive cells. Furthermore, the automated gating program did not allocate CD4+ CD8+ double-positive T cells as a separate population. Using manual gating, we identified CD4+CD8+ double-positive T cells in this case.
Two cases (case nos. 16, 17) revealed relatively higher differences in both CD3+ T cell and CD19+ B cell counts; both these cases presented increased CD3+ T cells (6%, 5.5% respectively) and decreased CD19+ B cells (5.9%, 7.5%, respectively) in automated gating compared to that in manual gating. In these cases (Fig. 3), all lymphocytes were not located in the lymphocyte adjust (LADJ) region in the automated gating plot. After reanalysis with manual gating, the escape area was included and the LADJ region was increased to recover the lost cells.
The CD56+ NK cells did not differ significantly between automated and manual gating; however, two cases (case nos. 21 and 22) revealed gating purity <95% regardless of the gating method. To analyze the cause, these samples were retested with CD16/56 reagent and reanalyzed using BD FACSCanto II (BD Bioscience, USA); we observed 9% and 10.3% of CD56-CD16+ NK cells in case nos. 21 and 22, respectively. Notably, the low gating purity was due to CD56-CD16 + NK cells, which are currently not measured in the Navios system.
In all 22 cases, the tube-to-tube consistency was analyzed by comparing the CD3+ T cell percentage in the first and second tubes. Our laboratory allowed the difference in CD3+ T cell percentage to be <2% while performing manual gating. All 22 cases exhibited a difference <2% without errors by manual gating; however, automated gating revealed a 3.8% difference in one case (case no. 6). After review, the cut-off line for measuring CD3+ T cell positivity in histogram was fixed in an automated gating program. Thus, as illustrated in Fig. 4, the CD3+ T cell was overestimated in tube 1 and the cut-off line should be adjusted.
Using IMMUNO-TROL cells, CVs of the percentages of both total lymphocytes and absolute counts for CD3+ T cell, CD3+CD4+ T cell, CD3+CD8+ T cells, and CD19+ B cells were <5% in manual and automated gating. Moreover, the CVs for CD56+ NK cells were <10% (8.9% for percentages of total lymphocytes, 8.7% for the absolute count, respectively) in automated analysis. The CVs for CD56+ NK cells were 5.2% for total lymphocytes and 3.9% for the absolute count in automated analysis. Considering IMMUNO-TROL low cells, all CVs for the percentage of total lymphocytes and absolute counts were <10% except for CD56+ NK cells (11.6% for absolute count) via automated gating.
It is difficult to define an appropriate gating method due to the limited reference standard and analyzed sample numbers [16]. In the present study, certain inconsistent results were observed from post-HSCT patients, presumably because post-HSCT patients are more likely to develop aberrant cell expression or unexpected phenotyping cells during the engraftment that are not observed in healthy individuals. Aberrant marker expressions pose challenges during data analysis, even for an experienced and expert technician.
In particular, a significant difference was observed in the number of CD8+ T cells between the two gating methods. Notably, CD8 low-expressing T cells were the major cause of this difference between automated and manual gating. To the best of our knowledge, no reference or guideline is available for appropriate gating of CD8 low-expressing T cells in the lymphocyte subset. The CD8 low-expressing T cells can be detected in approximately 0.3%–7.0% of all CD8+ T cells in healthy individuals [17, 18]; moreover, they can be increased in acute immune response after pathogen exposure or during chronic diseases [17-22]. These CD8 low-expressing T cells were associated with disease severity in chronic persistent infection; furthermore, they majorly produced interferon (IFN)-γ during inflammatory regulation [18, 19]. Therefore, we considered CD8 low-expressing T cells as a separate population, and did not include them in CD8 T cells while reporting the results.
In automated gating analysis, CD4+CD8+ double-positive T cells were not specified as a separate cell population. CD4+CD8+ double-positive T cells in peripheral blood have been described in multiple conditions such as viral infection, malignancies, and autoimmune diseases [23, 24]. This population exhibits potent immune suppressor function or highly cytotoxic potencies [23]; however, their exact function remains unclear. Laboratories should consider the likelihood of appearance of CD4+CD8+ double-positive T cells while analyzing post-HSCT samples with an automated gating approach.
In healthy individuals, CD56bright+ NK cells account for approximately 10% of peripheral NK cells, and these cells produce high levels of cytokines and exhibit low cytotoxicity; whereas, CD56dim+ NK cells, which account for approximately 90% of NK cells, produce less cytokines and exhibit high cytotoxicity [25]. CD56− NK cells were discovered 25 years ago [26] and have been reported as a dysfunctional subset compared to CD56+ NK cells, due to the low levels of cytotoxic receptor expression and cytokine production [25]. Moreover, CD56− NK cells were reported to increase in the cord blood of healthy neonates [27], as well as in patients with viral infection (HIV, hepatitis C virus, cytomegalovirus, hantavirus), autoimmune disease, and post-HSCT, particularly in T cell-depleted haploidentical HSCT and cord blood transplantation [28-31]. More recently, Forconi et al. [25] have described that CD56-CD16+ NK cells share similar morphologic features and transcriptional profiles with CD56dim CD16-NK cells. They reported that CD56-CD16+ NK cells could adapt to CD56dim CD16+ NK cells under chronic disease conditions. As presented in our data, the presence of CD56−CD16+ NK cells should be considered when the purity of the lymphocyte subset is <95%. Since CD56-CD16+ NK cells are likely to increase in post-HSCT conditions [28, 31], it is necessary to add CD16 reagents for NK cell markers in these cases. Furthermore, the Clinical and Laboratory Standards Institute guideline states that excluding CD16 marker prevents other normal CD16 monocytes/dendritic cell subsets that may be within the lymphocyte gate from leading to incorrect quantification of NK cells [32]. Nevertheless, while gating lymphocytes on CD45 and side scatter (SSC) plots, it is unlikely that monocytes/dendritic cells will be included in the lymphocyte gate; hence, addition of CD16 reagent has more advantages in accurate NK cell analysis.
The loss of lymphocytes via automated light scatter gating in automated gating software was previously mentioned [33]. The automated program is optimized for sample analysis considering specific conditions set by the manufacturer. These systems exhibit low tolerance, specifically when the light scatter parameters rely on gating, where sample integrity is compromised. Furthermore, automated software excludes lymphocytes in certain circumstances, such as processing delays or environmental factors. This issue was resolved using a modified protocol that repaired all lost lymphocytes by increasing the LADJ area [33]; similar protocol was applied in the present study as well. Additionally, the percentage of total lymphocytes in the light scatter LADJ gate needs to be evaluated via automated gating program. In patients presenting certain diseases, the operator needs to check the gating purity manually or optimize the setting.
As demonstrated in the present study, automated gating has a fixed baseline for determining cell positivity on a histogram. We observed that the fraction of CD3+ T cells in tube 1 and tube 2 may markedly differ than the laboratory acceptable levels. If the number of CD3+ T cells significantly differs between the two tubes, it is necessary to check the histogram manually.
In the present study, the CVs from automated gating and manual gating revealed no differences with <10% except for NK cells. According to the practice guideline from the International Council for Standardization of Haematology (ICSH) and International Clinical Cytometry Society (ICCS) a desirable assay imprecision indicated a CV <10% [34]; however, a CV <20% may be acceptable in cases with less abundant cell population, such as detection of fetal-maternal bleeding or minimal residual disease monitoring [34]. For samples with rare cell populations, higher CV may be tolerated depending on the disease status or intended use of the test. Large discrepancies could be expected in small cell populations, as the effects of small shifts in gate placement might be magnified. In these cases, it is recommended to use more replicates and samples for accurate evaluation.
The present study compared automated and manual gating in patients who revealed various cell populations in the engraftment process after HSCT. Collectively, the results of the two analyses were consistent; however, manual intervention was needed in some specific cases, in particular, CD8 low-expressing T cells, CD4+ CD8+ double-positive T cells, and CD56-CD16+ NK cells. It is recommended to visually assess the histogram and gating results while using automated gating in post-HSCT recipients to monitor the lymphocyte subset.
Acknowledgments
This study was supported by the 2017 Academic Research Fund of the Korean Society of Diagnostic Immunology.
REFERENCES
1. Peggs KS, Mackinnon S. 2004; Immune reconstitution following haematopoietic stem cell transplantation. Br J Haematol. 124:407–20. DOI: 10.1046/j.1365-2141.2003.04767.x. PMID: 14984491.


2. Storek J, Dawson MA, Storer B, Stevens-Ayers T, Maloney DG, Marr KA, et al. 2001; Immune reconstitution after allogeneic marrow transplantation compared with blood stem cell transplantation. Blood. 97:3380–9. DOI: 10.1182/blood.V97.11.3380. PMID: 11369627.


3. Savani BN, Rezvani K, Mielke S, Montero A, Kurlander R, Carter CS, et al. 2006; Factors associated with early molecular remission after T cell-depleted allogeneic stem cell transplantation for chronic myelogenous leukemia. Blood. 107:1688–95. DOI: 10.1182/blood-2005-05-1897. PMID: 16131570. PMCID: PMC1895415.


4. Kim DH, Sohn SK, Won DI, Lee NY, Suh JS, Lee KB. 2006; Rapid helper T-cell recovery above 200×106/l at 3 months correlates to successful transplant outcomes after allogeneic stem cell transplantation. Bone Marrow Transplant. 37:1119–28. DOI: 10.1038/sj.bmt.1705381. PMID: 16699530.
5. Williams KM, Gress RE. 2008; Immune reconstitution and implications for immunotherapy following haematopoietic stem cell transplantation. Best Pract Res Clin Haematol. 21:579–96. DOI: 10.1016/j.beha.2008.06.003. PMID: 18790456. PMCID: PMC2577193.


6. Brinkman RR. 2020; Improving the rigor and reproducibility of flow cytometry-based clinical research and trials through automated data analysis. Cytometry A. 97:107–12. DOI: 10.1002/cyto.a.23883. PMID: 31515945. PMCID: PMC8043840.


7. Montante S, Brinkman RR. 2019; Flow cytometry data analysis: Recent tools and algorithms. Int J Lab Hematol. 41(Suppl 1):56–62. DOI: 10.1111/ijlh.13016. PMID: 31069980.


8. Frelinger J, Ottinger J, Gouttefangeas C, Chan C. 2010; Modeling flow cytometry data for cancer vaccine immune monitoring. Cancer Immunol Immunother. 59:1435–41. DOI: 10.1007/s00262-010-0883-4. PMID: 20563720. PMCID: PMC2892609.


9. Meehan S, Walther G, Moore W, Orlova D, Meehan C, Parks D, et al. 2014; AutoGate: automating analysis of flow cytometry data. Immunol Res. 58:218–23. DOI: 10.1007/s12026-014-8519-y. PMID: 24825775. PMCID: PMC4464812.


10. Flores-Montero J, Grigore G, Fluxá R, Hernández J, Fernandez P, Almeida J, et al. 2019; EuroFlow Lymphoid Screening Tube (LST) data base for automated identification of blood lymphocyte subsets. J Immunol Methods. 475:112662. DOI: 10.1016/j.jim.2019.112662. PMID: 31454495.


11. Verschoor CP, Lelic A, Bramson JL, Bowdish DM. 2015; An introduction to automated flow cytometry gating tools and their implementation. Front Immunol. 6:380. DOI: 10.3389/fimmu.2015.00380. PMID: 26284066. PMCID: PMC4515551.


12. Mair F, Hartmann FJ, Mrdjen D, Tosevski V, Krieg C, Becher B. 2016; The end of gating? An introduction to automated analysis of high dimensional cytometry data. Eur J Immunol. 46:34–43. DOI: 10.1002/eji.201545774. PMID: 26548301.


13. Conrad VK, Dubay CJ, Malek M, Brinkman RR, Koguchi Y, Redmond WL. 2019; Implementation and validation of an automated flow cytometry analysis pipeline for human immune profiling. Cytometry A. 95:183–91. DOI: 10.1002/cyto.a.23664. PMID: 30570217.


14. Aghaeepour N, Finak G, Hoos H, Mosmann TR, Brinkman R, Gottardo R, et al. 2013; Critical assessment of automated flow cytometry data analysis techniques. Nat Methods. 10:228–38. DOI: 10.1038/nmeth.2365. PMID: 23396282. PMCID: PMC3906045.


15. Mandy FF, Nicholson JK, McDougal JS. 2003; Guidelines for performing single-platform absolute CD4+ T-cell determinations with CD45 gating for persons infected with human immunodeficiency virus. Centers for Disease Control and Prevention. MMWR Recomm Rep. 52:1–13. PMID: 12583540.
16. Linskens E, Diks AM, Neirinck J, Perez-Andres M, De Maertelaere E, Berkowska MA, et al. 2020; Improved standardization of flow cytometry diagnostic screening of primary immunodeficiency by software-based automated gating. Front Immunol. 11:584646. DOI: 10.3389/fimmu.2020.584646. PMID: 33224147. PMCID: PMC7667243.


17. Trautmann A, Rückert B, Schmid-Grendelmeier P, Niederer E, Bröcker EB, Blaser K, et al. 2003; Human CD8 T cells of the peripheral blood contain a low CD8 expressing cytotoxic/effector subpopulation. Immunology. 108:305–12. DOI: 10.1046/j.1365-2567.2003.01590.x. PMID: 12603596. PMCID: PMC1782903.


18. Ouyang L, Li X, Liang Z, Yang D, Gong F, Shen G, et al. 2013; CD8low T-cell subpopulation is increased in patients with chronic hepatitis B virus infection. Mol Immunol. 56:698–704. DOI: 10.1016/j.molimm.2013.07.003. PMID: 23933510.
19. Marins-Dos-Santos A, Olivieri BP, Ferreira-Reis R, de Meis J, Silva AA, de Araújo-Jorge TC, et al. 2020; CD8low T cells expanded following acute Trypanosoma cruzi infection and benznidazole treatment are a relevant subset of IFN-gamma producers. PLoS Negl Trop Dis. 14:e0008969. DOI: 10.1371/journal.pntd.0008969. PMID: 33347472. PMCID: PMC7785226.
20. Liu B, Ma Y, Zhang Y, Zhang C, Yi J, Zhuang R, et al. 2015; CD8low CD100- T cells identify a novel CD8 T cell subset associated with viral control during human Hantaan virus infection. J Virol. 89:11834–44. DOI: 10.1128/JVI.01610-15. PMID: 26378166. PMCID: PMC4645342.
21. Xu H, Wang X, Lackner AA, Veazey RS. 2013; CD8 down-regulation and functional impairment of SIV-specific cytotoxic T lymphocytes in lymphoid and mucosal tissues during SIV infection. J Leukoc Biol. 93:943–50. DOI: 10.1189/jlb.1112580. PMID: 23519937. PMCID: PMC3656336.


22. Favre D, Stoddart CA, Emu B, Hoh R, Martin JN, Hecht FM, et al. 2011; HIV disease progression correlates with the generation of dysfunctional naive CD8low T cells. Blood. 117:2189–99. DOI: 10.1182/blood-2010-06-288035. PMID: 21200021. PMCID: PMC3062328.
23. Overgaard NH, Jung JW, Steptoe RJ, Wells JW. 2015; CD4+/CD8+ double-positive T cells: more than just a developmental stage? J Leukoc Biol. 97:31–8. DOI: 10.1189/jlb.1RU0814-382. PMID: 25360000.
24. Alhaj Hussen K, Michonneau D, Biajoux V, Keita S, Dubouchet L, Nelson E, et al. 2020; CD4+CD8+ T-lymphocytes in xenogeneic and human graft-versus-host disease. Front Immunol. 11:579776. DOI: 10.3389/fimmu.2020.579776. PMID: 33329550. PMCID: PMC7732609.
25. Forconi CS, Oduor CI, Oluoch PO, Ong'echa JM, Münz C, Bailey JA, et al. 2020; A New Hope for CD56negCD16pos NK cells as unconventional cytotoxic mediators: An adaptation to chronic diseases. Front Cell Infect Microbiol. 10:162. DOI: 10.3389/fcimb.2020.00162. PMID: 32373555. PMCID: PMC7186373.


26. Hu PF, Hultin LE, Hultin P, Hausner MA, Hirji K, Jewett A, et al. 1995; Natural killer cell immunodeficiency in HIV disease is manifest by profoundly decreased numbers of CD16+CD56+ cells and expansion of a population of CD16dimCD56- cells with low lytic activity. J Acquir Immune Defic Syndr Hum Retrovirol. 10:331–40. DOI: 10.1097/00042560-199511000-00005. PMID: 7552495.
27. Jacobson A, Bell F, Lejarcegui N, Mitchell C, Frenkel L, Horton H. 2013; Healthy neonates possess a CD56-negative NK cell population with reduced anti-viral activity. PLoS One. 8:e67700. DOI: 10.1371/journal.pone.0067700. PMID: 23805324. PMCID: PMC3689709.


28. De Angelis C, Mancusi A, Ruggeri L, Capanni M, Urbani E, Velardi A, et al. 2011; Expansion of CD56-negative, CD16-positive, KIR-expressing natural killer cells after T cell-depleted haploidentical hematopoietic stem cell transplantation. Acta Haematol. 126:13–20. DOI: 10.1159/000323661. PMID: 21411985.


29. Milush JM, López-Vergès S, York VA, Deeks SG, Martin JN, Hecht FM, et al. 2013; CD56negCD16+ NK cells are activated mature NK cells with impaired effector function during HIV-1 infection. Retrovirology. 10:158. DOI: 10.1186/1742-4690-10-158. PMID: 24351015. PMCID: PMC3892122.


30. Van Acker HH, Capsomidis A, Smits EL, Van Tendeloo VF. 2017; CD56 in the immune system: more than a marker for cytotoxicity? Front Immunol. 8:892. DOI: 10.3389/fimmu.2017.00892. PMID: 28791027. PMCID: PMC5522883.


31. Lu X, Kondo Y, Takamatsu H, Ohata K, Yamazaki H, Takami A, et al. 2008; CD16+ CD56- NK cells in the peripheral blood of cord blood transplant recipients: a unique subset of NK cells possibly associated with graft-versus-leukemia effect. Eur J Haematol. 81:18–25. DOI: 10.1111/j.1600-0609.2008.01073.x. PMID: 18363874.
32. Clinical, Laboratory Standards Institute. 2007. Enumeration of immunologically defined cell populations by flow cytometry; Approved guideline-Second edition. CLSI document H42-A2. Clinical and Laboratory Standards Institute,;Wayne, PA: p. 18p. 21–22.
33. Diallo TO, Bergeron M, Seely P, Yang X, Ding T, Plews M, et al. 2017; Automation for clinical CD4 T-cell enumeration, a desirable tool in the hands of skilled operators. Cytometry B Clin Cytom. 92:445–50. DOI: 10.1002/cyto.b.21370. PMID: 26990810.


34. Wood B, Jevremovic D, Bene MC, Yan M, Jacobs P, Litwin V, et al. 2013; Validation of cell-based fluorescence assays: practice guidelines from the ICSH and ICCS - part V - assay performance criteria. Cytometry B Clin Cytom. 84:315–23. DOI: 10.1002/cyto.b.21108. PMID: 24022854.


Fig. 1
The difference in CD8+ T cells between automated and manual gating. (A) Correlation of CD8+ T cells between automated and manual gating. CD8+ T cells analyzed via automated gating were higher than those evaluated by manual gating (Y (automated gating)=9.504+0.925 X (manual gating)), r=0.862, P<0.001). The automated gating program (B) included CD8 dim population in CD8+ T cell, but we excluded them in manual gating (C) (data from Case 4).
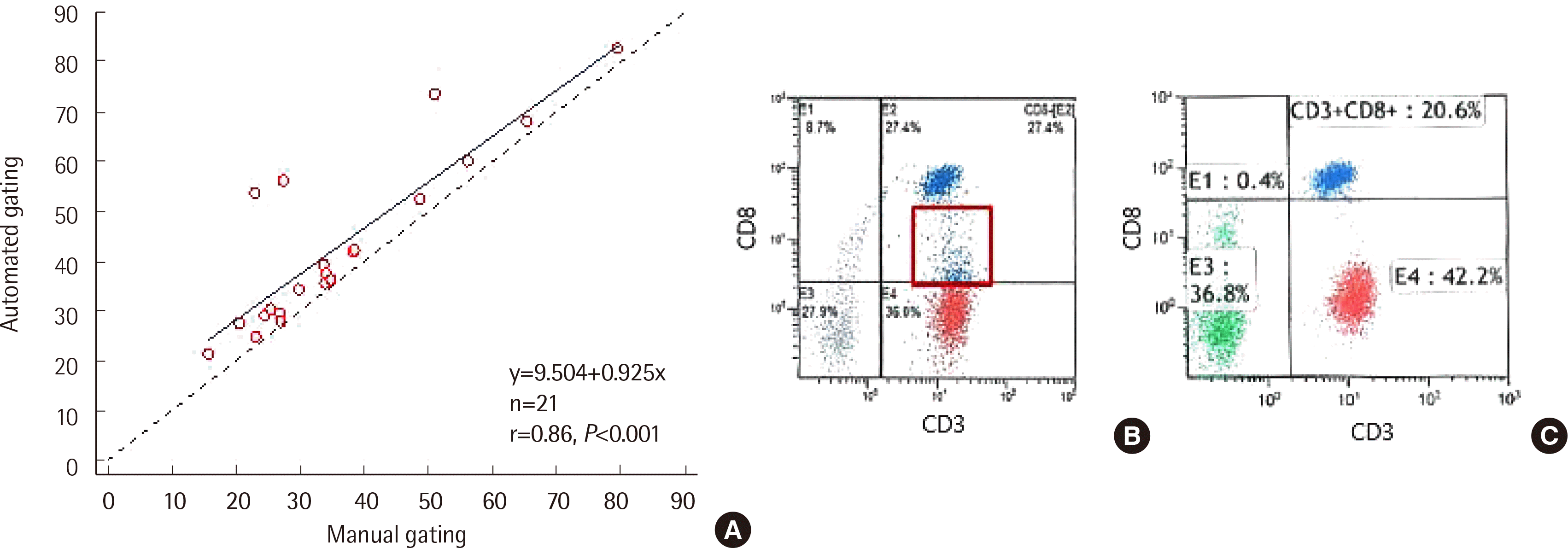
Fig. 2
CD8 T cell errors in automated gating. Automated gating (A and B) failed to identify CD8+ T cells. In manual gating (C and D), we could identify the exact number of CD8+ T cells after compensation. (A) and (C) represent Case 16; (B) and (D) represent Case 18.
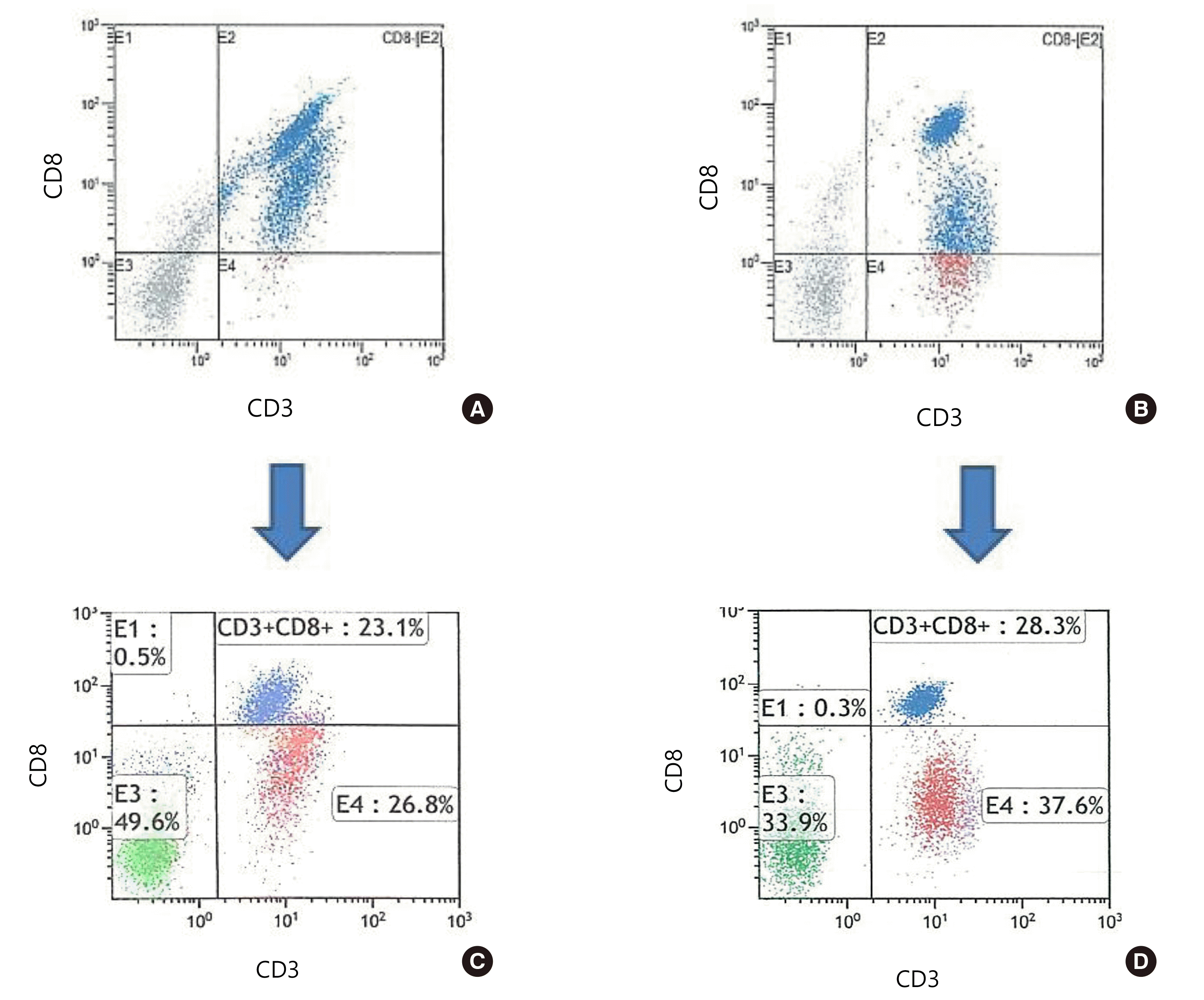
Fig. 3
Gating of total lymphocytes via automated gating by excluding some lymphocytes. Certain proportions of total lymphocytes were excluded in gating (A) and (B) (data from Case 16).
Abbreviations: GADJ, gate adjust; LADJ, lymphocyte adjust; FSC, forward scatter; SSC, side scatter.
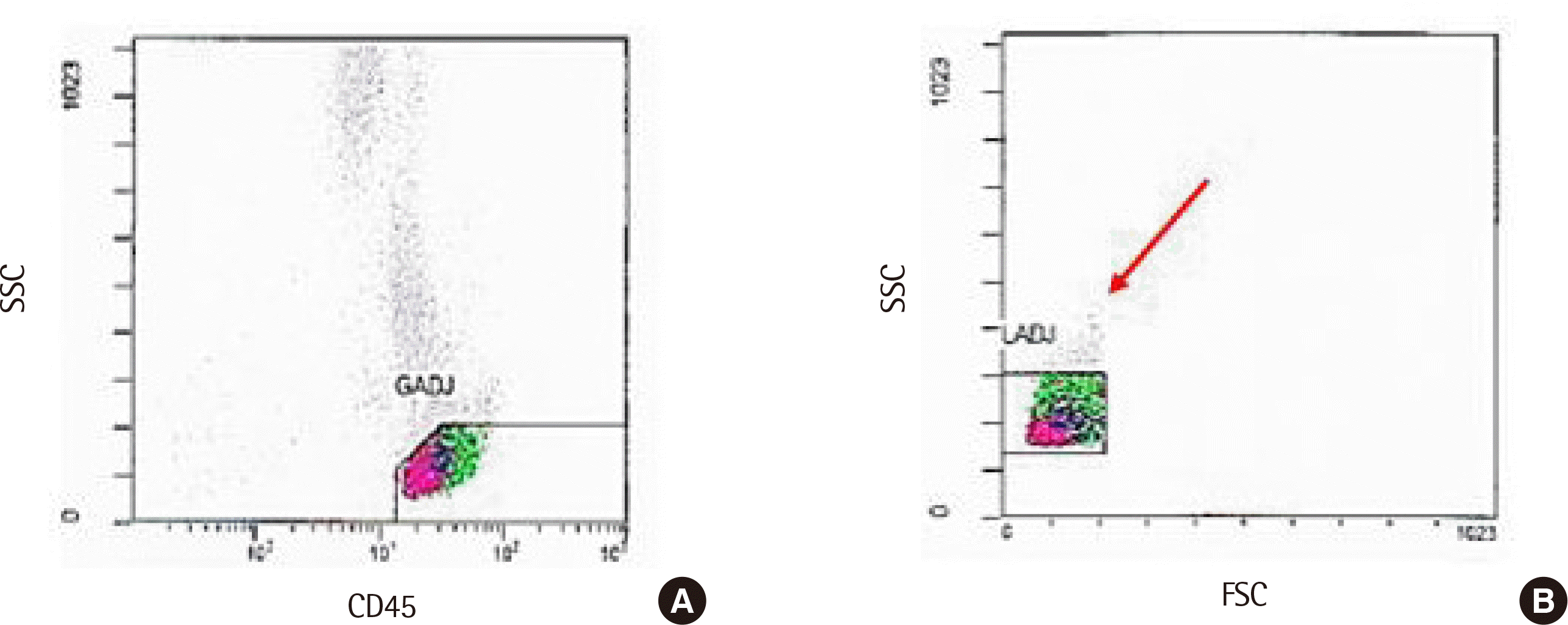
Fig. 4
CD3+ T cell difference in a case with tube-to-tube variability. In automated gating, CD3+ T cell fraction from tube 1 (A) was overestimated compared to that in tube 2 (B), as certain CD3− cell fraction was included in the analysis of tube 1 (data from Case 6).
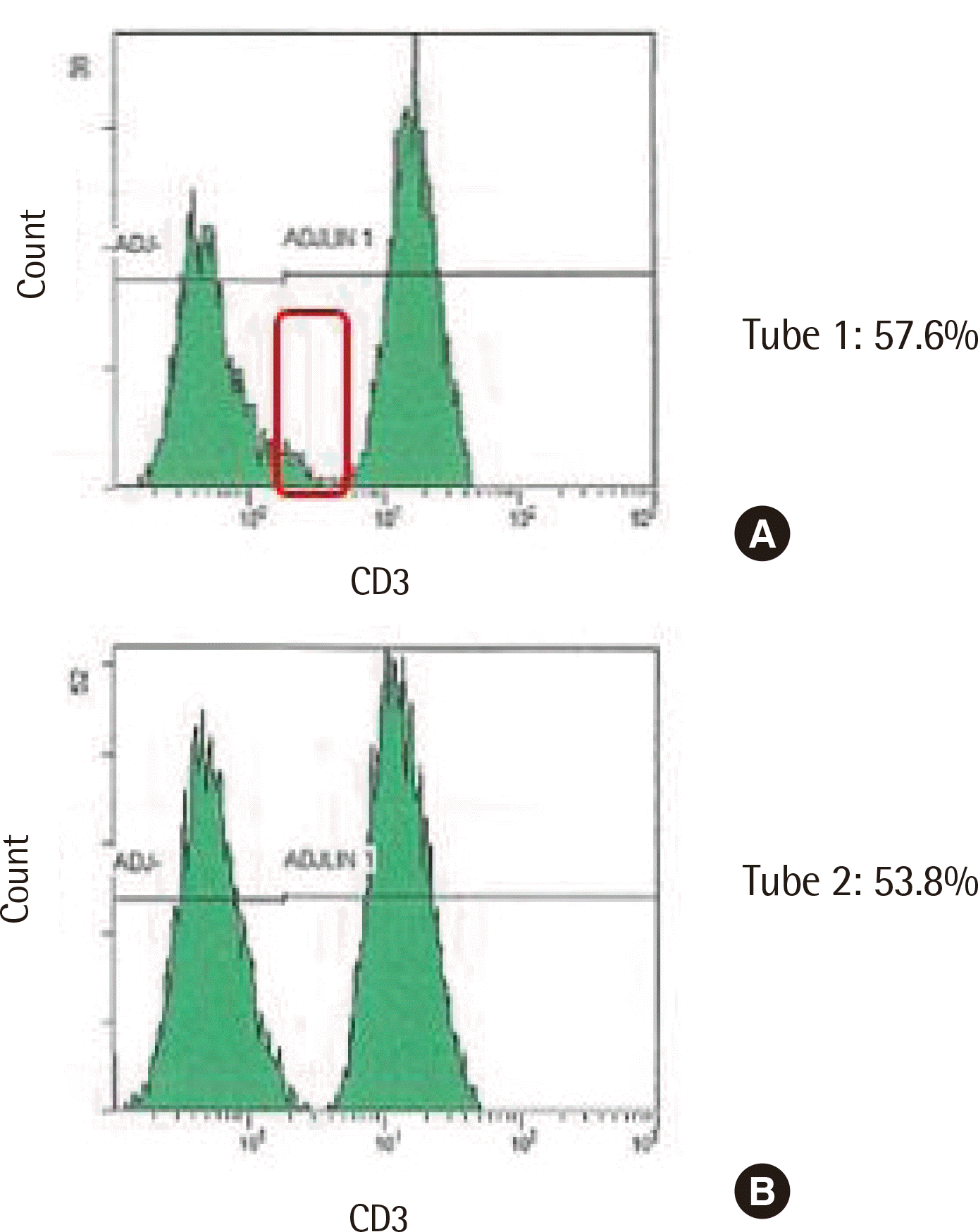
Table 1
Raw data from study population (N=22)
Table 2
Difference between automated and manual gating in patient samples (N=22)
Absolute percentage difference (%) (automated % - manual %) | Spearman rank correlation | ||||||
---|---|---|---|---|---|---|---|
|
|
||||||
Median | 95% CI | Min | Max | Spearman rho | 95% CI | P value | |
CD3+ T cell (tube 1) | 0.50 | 0.07–1.10 | -2.70 | 4.50 | 0.978 | 0.947–0.991 | < 0.001 |
CD3+ T cell (tube 2) | 0.80 | -0.10–1.91 | -2.20 | 6.00 | 0.980 | 0.951–0.992 | < 0.001 |
CD4+ T cell | 0.35 | -0.30–1.11 | -1.40 | 5.70 | 0.983 | 0.959–0.993 | < 0.001 |
CD8+ T cell (N = 21)* | -3.60 | -4.82– (-3.07) | -30.40 | -0.50 | 0.800 | 0.563–0.916 | < 0.001 |
CD19+ B cell | -0.30 | -0.51–0.20 | -7.50 | 1.10 | 0.991 | 0.977–0.996 | < 0.001 |
CD56+ NK cell | 0.00 | -0.30– (-1.10) | -1.10 | 3.00 | 0.999 | 0.997–1.000 | < 0.001 |