Abstract
Objectives
Physical distancing is a control measure against coronavirus disease 2019 (COVID-19). Lockdowns are a strategy to enforce physical distancing in urban areas, but they are drastic measures. Therefore, we assessed the effectiveness of the lockdown measures taken in the world’s second-most populous country, India, by exploring their relationship with community mobility patterns and the doubling time of COVID-19.
Methods
We conducted a retrospective analysis based on community mobility patterns, the stringency index of lockdown measures, and the doubling time of COVID-19 cases in India between February 15 and April 26, 2020. Pearson correlation coefficients were calculated between the stringency index, community mobility patterns, and the doubling time of COVID-19 cases. Multiple linear regression was applied to predict the doubling time of COVID-19.
Results
Community mobility drastically fell after the lockdown was instituted. The doubling time of COVID-19 cases was negatively correlated with population mobility patterns in outdoor areas (r = −0.45 to −0.58). The stringency index and outdoor mobility patterns were also negatively correlated (r = −0.89 to −0.95). Population mobility patterns (R2 = 0.67) were found to predict the doubling time of COVID-19, and the model’s predictive power increased when the stringency index was also added (R2 = 0.73).
Conclusions
Lockdown measures could effectively ensure physical distancing and reduce short-term case spikes in India. Therefore, lockdown measures may be considered for tailored implementation on an intermittent basis, whenever COVID-19 cases are predicted to exceed the health care system’s capacity to manage.
Coronavirus disease 2019 (COVID-19) is wreaking havoc worldwide, with 88.4 million cases and 1.9 million deaths recorded so far [1]. The infectivity of severe acute respiratory syndrome coronavirus-2 (SARS-CoV-2) is high, with a reproductive number (R0) ranging up to 5.8 [2]. Its mode of transmission (through aerosols and droplets) enables easy spread through direct or close contact between people. The mortality of COVID-19 is as high as 14.8% in patients older than 80 years of age [3], which has prompted urgent, active interventions to halt its spread. In order to tackle what appears to be the worst public health threat of the 21st century, governments are undertaking measures to break the transmission chain and control the case numbers. These include physical distancing, cough etiquette, universal face masks, aggressive tracing of contacts and quarantining, liberal testing strategies such that healthcare systems do not miss cases, prompt isolation, and treatment of cases in dedicated facilities [4]. Adherence to physical distancing in urban areas is a herculean task considering their higher population density than rural areas, burgeoning economic and industrial activity, and the resultant overcrowding. Lockdowns were one of the measures taken during the initial stages of the pandemic to enforce physical distancing in urban areas. Lockdowns aim to slow down the infection rate by statutory means to prevent direct and close contact. These measures are aimed at restricting the movements of people in public places to give health systems time to prepare to tackle the COVID-19 outbreak [5]. Lockdowns range from complete closure of all non-essential establishments, halting their activities and public transport, to partial relaxation of these restrictions. People are not allowed to travel or move freely in public, except for essential needs at specified times and emergencies. These measures restrict population mobility in public and social spaces. When explored in terms of time and geography, mobility may provide valuable information for understanding infectious disease dynamics [6,7]. How an individual moves and spends time in different places determines the individual’s activity space. This information can assist in studying the spatial and temporal risk of a disease for a person in places other than his or her place of residence. Various tools and data sources such as travel diaries, personal GPS trackers, census, remotely sensed night-time light data, social media, open mobile mapping applications, call data records, and Google location history (GLH) are used to analyze people’s mobility [6,7]. GLH is open-source data and an underused source of human mobility information that can be used in numerous research contexts [6].
Lockdowns are drastic measures that affect the masses’ social, political, and economic livelihood. They might also have a deleterious impact on routine non-COVID-19 healthcare services [8,9]. Hence, the dividends expected from such measures should also be high in terms of disease control. The effectiveness of lockdown measures has been under scrutiny, as studies across the globe have reported mixed results. Models explaining the effects of lockdown measures on case numbers have shown that the lockdowns have reduced the intensity of cases [10–12]. There are also countries like South Korea and Taiwan, which have efficiently controlled COVID-19 without lockdown measures [13]. India, a low-middle-income country with a population of 1.3 billion, recorded its first COVID-19 case on January 30, 2020. India is a union made up of 28 states and 8 union territories [14]. After the first week of March 2020, the COVID-19 epidemic curve of India started climbing up. The Union government of India announced a range of measures to tackle the COVID-19 outbreak. A complete nationwide lockdown was implemented from March 24, 2020 [15] to June 30, 2020. However, the lockdown, which was a strategy to reduce the public’s mobility and thus slow the transmission of COVID-19, was only as good as its implementation. The association of the lockdown measures with community mobility patterns and subsequent COVID-19 cases needs to be studied. Multiple vaccines against COVID-19 have been introduced, yet the emergence of new variants with high transmissibility poses a challenge [16]. Although the variants have not been reported to have significant impacts on the severity and outcomes of COVID-19 [16], they might do so in the future [17]. Therefore, further research on this topic will help us understand the effectiveness of lockdowns and whether they are a viable option for the future if waves of pandemics hit again. Hence, we assessed the effectiveness of the lockdown measures taken during the early phase of the COVID-19 pandemic in India by exploring community mobility patterns and their relationship with the doubling time of COVID-19.
We conducted a retrospective analysis based on data collected on community mobility patterns, the stringency index of lockdown measures, and the doubling time of COVID-19 cases in India for the period between February 15 and April 26, 2020, in order to include the first month of the lockdown.
Google releases anonymized, aggregated data on the mobility of people using Android phones in various places categorized as retail and recreation, parks, grocery and pharmacy, transit stations, workplace, and residential areas [18]. After removing all identification details, the data are aggregated from the persons who have enabled GLH on their mobile devices. Google has released this area-wise, time-series dataset intending to help analyze mobility patterns and aid in assessing physical distancing efforts made in various locations during the COVID-19 times. These reports show how the density of visits to different places changes compared to baseline mobility. The baseline mobility is the median value for the corresponding day of the week during the 5 weeks between January 3 and February 6, 2020. The type of data included in the calculation depends on the user settings, connectivity, and whether it meets the Google privacy threshold. Mobility patterns may reflect the ground-level reality and the actual implementation of the lockdown measures as stipulated by the government as a policy decision. Data on mobility patterns for India, including all 28 states, from February 15 to April 26, 2020 were downloaded from Google. The values range from +100 to −100 relative to baseline mobility. These data sets can be accessed at google.com/covid19/mobility[18].
The measures taken as policy decisions by the governments to ensure physical distancing were quantified using a specialized index referred to as the stringency index [19]. It is formulated and calculated for more than 100 countries by a group of researchers at Oxford daily. This index helps to assess variation in governments’ containment and lockdown policies across time and geographic distance. The stringency index is calculated as a composite score of the following nine indicators: School closure, workplace closure, cancellation of public events, restrictions on gathering size, public transport closure, stay-at-home requirements, restrictions on internal movement, international travel, and public information campaigns. The score ranges from 0 to 100, with higher scores indicating stricter lockdown policy measures in the country. The stringency index for India was obtained from February 15, 2020, to April 26, 2020 from an open-source [19].
The doubling time of a disease refers to the time taken for the number of cases to double [20]. We calculated the doubling time for COVID-19, for each day from February 15 to April 26, 2020 based on the daily incidence of the cases and the growth rate by using the formula, T
d
= ln(2/r) [21]. The data on the cumulative number of daily COVID-19 cases in India from February 15 to April 26, 2020 weres obtained from the database published by Johns Hopkins University Center for Systems Science and Engineering (CSSE) (https://datahub.io/core/covid-19#readme). The data were verified with the daily World Health Organization (WHO) Situation Reports [1]. The doubling time on each day was calculated.
The statistical software package SPSS version 26.0 (trial version; IBM Corp., Armonk, NY, USA) was used for archiving and analysis. Scatterplots were used to visualize and analyze time-series data. Spatial variability maps were generated using an online tool (https://www.datawrapper.de/) to show changes in mobility patterns across the states of India. Pearson correlation coefficients were calculated between mobility patterns and the doubling time of COVID-19 cases, the stringency index, and the mobility pattern. Mobility patterns at various places and stringency index values were applied separately to obtain two different multiple linear regression models to predict the doubling time of COVID-19. Any extreme deviation in the doubling time on any particular day was removed as an outlier to meet the assumptions of the linear regression model. EViews 12 (trial version) [22] was used to test for autocorrelation using the Durbin-Watson test [23].
The changes in the mobility pattern of the population of India from February 15 to April 26, 2020, along with the landmark events, are shown in Figure 1. The mobility in all areas remained fairly consistent relative to the baseline until March 9, 2020. March 10 saw a considerable dip in the mobility in all outdoor and working areas, as it was a festival day in India. The mobility in parks, retail and recreation, transit stations, and workplaces decreased slightly from baseline after the WHO declared COVID-19 to be a pandemic on March 11, 2020. The fall in mobility gradually increased thereafter, and it steeply fell after March 23. The steep fall coincided with the day on which India implemented the nationwide lockdown. The category of retail and recreation had the highest fall (−87%), followed by grocery and pharmacy (−76%), transit stations (−74%), workplaces (−72%), and parks (−68%). The mobility in all these areas remained at or below 40% of the baseline values throughout the entire lockdown, thus indicating a ground-level implementation of the lockdown policy. The mobility in residential areas increased after March 23, as people were required to stay at their residences since outdoor movement was restricted. The spatial distribution of the mean percentage of the mobility changes across the states of India during the lockdown between March 24 and April 26, 2020 is depicted in Figure 2. Maharashtra had the highest decline among the states in community mobility in the categories of parks (−67%), transit stations (−77%), and workplaces (−72%), while Chandigarh was the best performer of the union territories in community mobility reductions (Figures 3
–5).
As the stringency index of India started to increase, community mobility in parks, retail and recreation, transit stations, and workplaces started decreasing. The relative mobility values dropped below 50% when the stringency index crossed 80 (Figure 6).
The mobility changes in all outdoor and social places, such as parks (r = −0.58) and retail and recreation areas (r = −0.57), were negatively correlated with the doubling time of COVID-19 cases. Mobility changes in grocery and pharmacy areas (r = −0.45), transit stations (r = −0.58), and workplaces (r = −0.48) also showed significant negative correlations with the doubling time. Mobility changes in residential places were positively correlated with the doubling time (r = 0.51). (Table 1) As mobility decreased in outdoor areas, the above findings indicate that the doubling time of COVID-19 cases increased.
The mobility changes in all outdoor and social places, such as grocery and pharmacy (r = −0.89), parks (r = −0.94), retail and recreation areas (r = −0.95), transit stations (r = −0.95), and workplaces (r = −0.92) had significant negative correlations with the stringency index (p < 0.001). However, mobility changes in residential places were positively correlated with the stringency index (r = 0.93) (Table 1). Thus, as the stringency index for India increased, outdoor mobility decreased and indoor mobility increased.
Multiple linear regression to predict the doubling time based on population mobility patterns was performed. The Durbin-Watson statistic was found to be 2.131, which is very close to 2 and above the upper bound of the critical value, revealing an absence of autocorrelation [23]. A highly significant regression equation was found (F
(6, 63) = 8.44, p < 0.001), with an R
2 of 0.668. Upon adding stringency index to the model, R
2 increased to 0.726, with a significant regression equation (F
(7, 62) = 9.87, p < 0.001). This finding shows that the reduction in mobility accounted for about 60%–70% of the change in the doubling time of COVID-19 in India.
The time series for the stringency index and the doubling time were plotted (Figure 7). A positive correlation was found between the stringency index and the doubling time of COVID-19 cases (r = 0.62).
The mobility pattern in residential places had high negative correlations (r = −0.79 to −0.93) with the mobility patterns in all other outdoor areas. In contrast, the mobility changes in all outdoor areas had high positive correlations between themselves (r > 0.70) (Figure 8).
Lockdowns are a non-pharmaceutical intervention (NPI) used to control the pandemic by achieving physical distancing. Lockdowns have been predicted to reduce hospitalization rates drastically [10]. Lockdowns have also been suggested as a way to provide breathing time for the health system to prepare to tackle the upcoming surge of caseload [5,11]. They are seen as a suppression measure against COVID-19. The suppression strategy aims to reverse the epidemic growth by reducing the cases and keeping them at a diminished level indefinitely [24].
The stringency index, which was formulated to assess lockdown strictness, does not reveal the appropriateness or effectiveness of a country’s response. It only measures the political commitment and strictness of governmental policies. A higher stringency index value does not necessarily mean that a country’s response is better than that of those with lower values. The stringency index does not provide information on how well policies are enforced, nor does it capture demographic or cultural characteristics that may affect the spread of COVID-19 [19].
In order to assess the actual effectiveness of the lockdown on the ground level, the present study explored changes in people’s mobility patterns. We found that changes in population mobility in outdoor areas were negatively correlated with disease spread, and this model could predict 60%–70% of the change in the doubling time of COVID-19. Human mobility data using mobile phones have been explored to predict infectious disease dynamics in previous studies [6,25]. However, collecting data from individual mobile phones and dealing with privacy issues has been a challenge in creating population-level data on mobility patterns [25]. Using the community mobility patterns published by Google, we tried to address the lacunae in this area by providing anonymized population-level data. The mobility restrictions might have improved the COVID-19 doubling time, indicating the probable usefulness of India’s lockdown measures and mobility reduction in explaining disease control. However, the case numbers might still have increased through transmission within households [26].
Studies around the world have shown that strict lockdown measures have positive effects on disease control. Ruktanonchai et al. [27] in their analyses from Europe, found that a resurgence of the epidemic could occur as early as 5 weeks after premature termination of countries’ stringent interventions, such as mobility reduction. Strict physical distancing measures—in the form of lockdowns—were shown to have been most likely effective in reducing the incidence, mortality, and growth rate of COVID-19 in China, as well as increasing its doubling time [11,28]. To suppress COVID-19, restriction measures for physical distancing at the population level must be in place until a vaccine is found and distributed, with intermittent relaxation depending on the case incidence and transmission rates [24]. However, lockdowns as isolated interventions cannot contain COVID-19. Lockdowns should be combined with other NPIs such as aggressive contact tracing, testing, and isolation of cases for efficient containment and suppression [11,29].
The exact proportion or level of the strictness of lockdown measures or the reduction in community mobility required for adequately controlling COVID-19 could not be assessed in our study. Lai et al. [11] predicted that maintenance of 25% contact reduction by NPIs such as physical distancing would ensure control of the COVID-19 epidemic.
The results of our study must be cautiously interpreted, as it did not assess or adjust for other crucial factors or strategies for COVID-19 control, such as the testing policy, stage of transmission of COVID-19 in India, isolation and quarantining measures for cases and contacts, and the community awareness level. Aggressive testing and contact tracing have been advocated as one of the most effective measures to control COVID-19. Changes in mobility were calculated only based on data from Android mobile users who had enabled their location history for their Google account. In India, although the vast majority of smartphone users use Android devices [30], the data on the proportion of people with GLH enabled is unknown. A multi-country survey among 250 Android users revealed that 43%–72% of them enabled GLH, while 5.6%–17.5% disabled GLH [7]. Hence, mobility data do not include the entire population and their movements. Instead, this data source represents only a sample of Google location service users among Android users.
The mobility pattern of the population within a community or region impacts the infectious disease dynamics. Through statutory lockdown measures, mobility reduction ensured physical distancing, which could control the infection spread in a short time in India. Lockdowns may be considered for tailored implementation on an intermittent basis whenever COVID-19 cases are predicted to exceed—or actually do surpass—the health care system’s capacity to manage them. The social, political, and economic impact of lockdowns on the population must also be studied in detail and factored in, to reduce the collateral damage while tailoring lockdowns.
References
1. World Health Organization. Coronavirus disease (COVID-19) situation report [Internet]. Geneva, Switzerland: World Health Organization;2020. [cited at 2021 Oct 15]. Available from: https://www.who.int/docs/default-source/coronaviruse/situation-reports/20200921-weekly-epi-update-6.pdf?sfvrsn=d9cf9496_6
.
2. Muniz-Rodriguez K, Chowell G, Cheung CH, Jia D, Lai PY, Lee Y, et al. Doubling time of the COVID-19 epidemic by province, China. Emerg Infect Dis. 2020; 26(8):1912–4.


3. Worldometer. Coronavirus age, sex, demographics (COVID-19) [Internet]. [place unknown]: Worldometer;2020. [cited at 2021 Oct 15]. Available from: https://www.worldometers.info/coronavirus/coronavirus-age-sex-demographics/
.
4. World Health Organization. COVID-19: Infection prevention and control / WASH [Internet]. Geneva, Switzerland: World Health Organization;2020. [cited at 2021 Oct 15]. Available from: https://www.who.int/emergencies/diseases/novel-coronavirus-2019/technical-guidance-publications?publicationtypes=d198f134-5eed-400d-922e-1ac06462e676
.
5. The Lancet. India under COVID-19 lockdown. Lancet. 2020; 395(10233):1315.
6. Carrasco-Escobar G, Castro MC, Barboza JL, Ruiz-Cabrejos J, Llanos-Cuentas A, Vinetz JM, et al. Use of open mobile mapping tool to assess human mobility traceability in rural offline populations with contrasting malaria dynamics. PeerJ. 2019; 7:e6298.


7. Ruktanonchai NW, Ruktanonchai CW, Floyd JR, Tatem AJ. Using Google Location History data to quantify finescale human mobility. Int J Health Geogr. 2018; 17(1):28.


8. Webb L. COVID-19 lockdown: a perfect storm for older people's mental health. J Psychiatr Ment Health Nurs. 2021; 28(2):300.


9. Riley T, Sully E, Ahmed Z, Biddlecom A. Estimates of the potential impact of the COVID-19 pandemic on sexual and reproductive health in low- and middle-income countries. Int Perspect Sex Reprod Health. 2020; 46:73–6.
10. Ngonghala CN, Iboi E, Eikenberry S, Scotch M, MacIntyre CR, Bonds MH, et al. Mathematical assessment of the impact of non-pharmaceutical interventions on curtailing the 2019 novel Coronavirus. Math Biosci. 2020; 325:108364.


11. Lai S, Ruktanonchai NW, Zhou L, Prosper O, Luo W, Floyd JR, et al. Effect of non-pharmaceutical interventions to contain COVID-19 in China. Nature. 2020; 585(7825):410–3.


12. Chatterjee K, Chatterjee K, Kumar A, Shankar S. Healthcare impact of COVID-19 epidemic in India: a stochastic mathematical model. Med J Armed Forces India. 2020; 76(2):147–55.


13. Lu N, Cheng KW, Qamar N, Huang KC, Johnson JA. Weathering COVID-19 storm: Successful control measures of five Asian countries. Am J Infect Control. 2020; 48(7):851–2.


14. Know India. States and union territories [Internet]. New Delhi, India: Know India;c2020. [cited at 2021 Oct 15]. Available from: https://knowindia.india.gov.in/states-uts/
.
16. World Health Organization. SARS-CoV-2 variants [Internet]. Geneva, Switzerland: World Health Organization;[cited at 2021 Oct 15]. Available from: https://www.who.int/emergencies/disease-outbreak-news/item/2020-DON305
.
17. Callaway E. The coronavirus is mutating: does it matter? Nature. 2020; 585(7824):174–7.
18. Google. COVID-19 community mobility report [Internet]. Mountain View (CA): Google;2020. [cited at 2021 Oct 15]. Available from: https://www.google.com/covid19/mobility
.
19. Hale T, Petherick A, Phillips T, Webster S. Variation in government responses to COVID-19[Internet]. Oxford UK: Blavatnik School of Government;2020. [cited at 2021 Oct 15]. Available from: https://www.bsg.ox.ac.uk/sites/default/files/2020-04/BSG-WP-2020-032-v5.0.pdf
.
20. Galvani AP, Lei X, Jewell NP. Severe acute respiratory syndrome: temporal stability and geographic variation in case-fatality rates and doubling times. Emerg Infect Dis. 2003; 9(8):991–4.


21. Wallinga J, Lipsitch M. How generation intervals shape the relationship between growth rates and reproductive numbers. Proc Biol Sci. 2007; 274(1609):599–604.


22. EViews [Internet]. Irvine (CA): IHS Global Inc;2020. [cited at 2021 Oct 15]. Available from: https://www.eviews.com/home.html
.
23. Evans WN. Time Series Analysis: Durbin-Watson significance tables [Internet]. Notre Dame (IN): University of Notre Dame;2018. [cited at 2021 Oct 15]. Available from: https://www3.nd.edu/~wevans1/econ30331/Durbin_Watson_tables.pdf
.
24. Ferguson NM, Laydon D, Nedjati-Gilani G, Imai N, Ainslie K, Baguelin M, et al. Report 9: Impact of non-pharmaceutical interventions (NPIs) to reduce COVID19 mortality and healthcare demand [Internet]. London, UK: Imperial College COVID-19 Response Team, Imperial College London;2020. [cited at 2021 Oct 15]. Available from: https://doi.org/10.25561/77482
.


25. Wesolowski A, Buckee CO, Engo-Monsen K, Metcalf CJ. Connecting mobility to infectious diseases: the promise and limits of mobile phone data. J Infect Dis. 2016; 214(suppl_4):S414–S420.


26. Sjodin H, Wilder-Smith A, Osman S, Farooq Z, Rocklov J. Only strict quarantine measures can curb the coronavirus disease (COVID-19) outbreak in Italy, 2020. Euro Surveill. 2020; 25(13):2000280.


27. Ruktanonchai NW, Floyd JR, Lai S, Ruktanonchai CW, Sadilek A, Rente-Lourenco P, et al. Assessing the impact of coordinated COVID-19 exit strategies across Europe. Science. 2020; 369(6510):1465–70.


28. Ji T, Chen HL, Xu J, Wu LN, Li JJ, Chen K, et al. Lockdown contained the spread of 2019 novel coronavirus disease in Huangshi City, China: early epidemiological findings. Clin Infect Dis. 2020; 71(6):1454–60.


29. Kong Q, Jin H, Sun Z, Kao Q, Chen J. Non-pharmaceutical intervention strategies for outbreak of COVID-19 in Hangzhou, China. Public Health. 2020; 182:185–6.


30. Statista. Market share of mobile operating systems in India from 2012–2020 [Internet]. Arlington (VA): The Institute for Statistics Education;c2021. [cited at 2021 Oct 15]. Available from: https://www.statista.com/statistics/262157/market-share-held-by-mobile-operating-systems-in-india/
.
Figure 2
Spatial distribution map showing mean changes from baseline in the mobility pattern between March 24 and April 26, 2020 across the states of India.
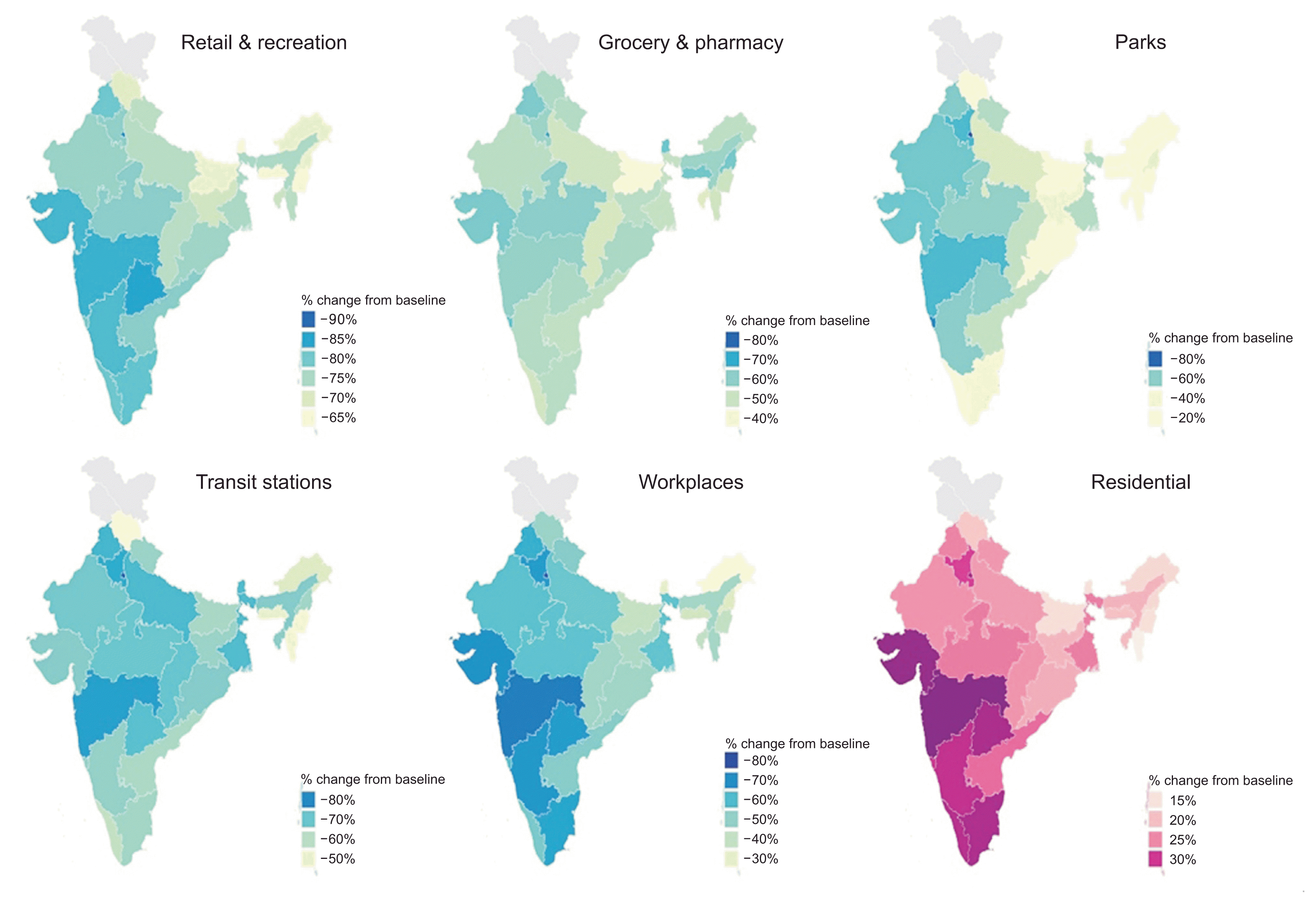
Figure 3
Statewise mean percentage changes in (A) retail and recreation and (B) grocery and pharmacy mobility patterns during the lockdown compared to baseline.
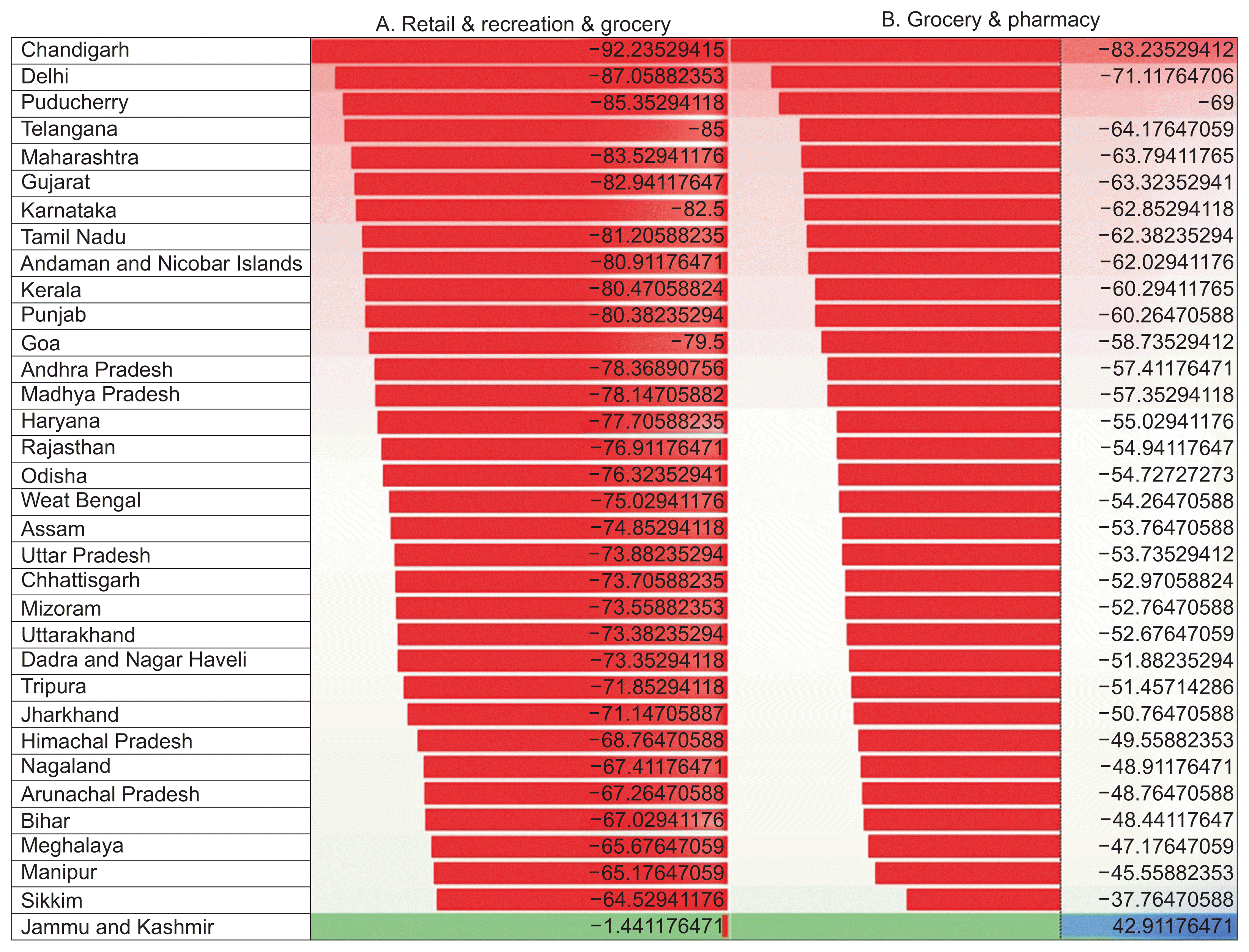
Figure 4
Statewise mean percentage changes in (A) park and (B) transit station mobility patterns during the lockdown compared to baseline.
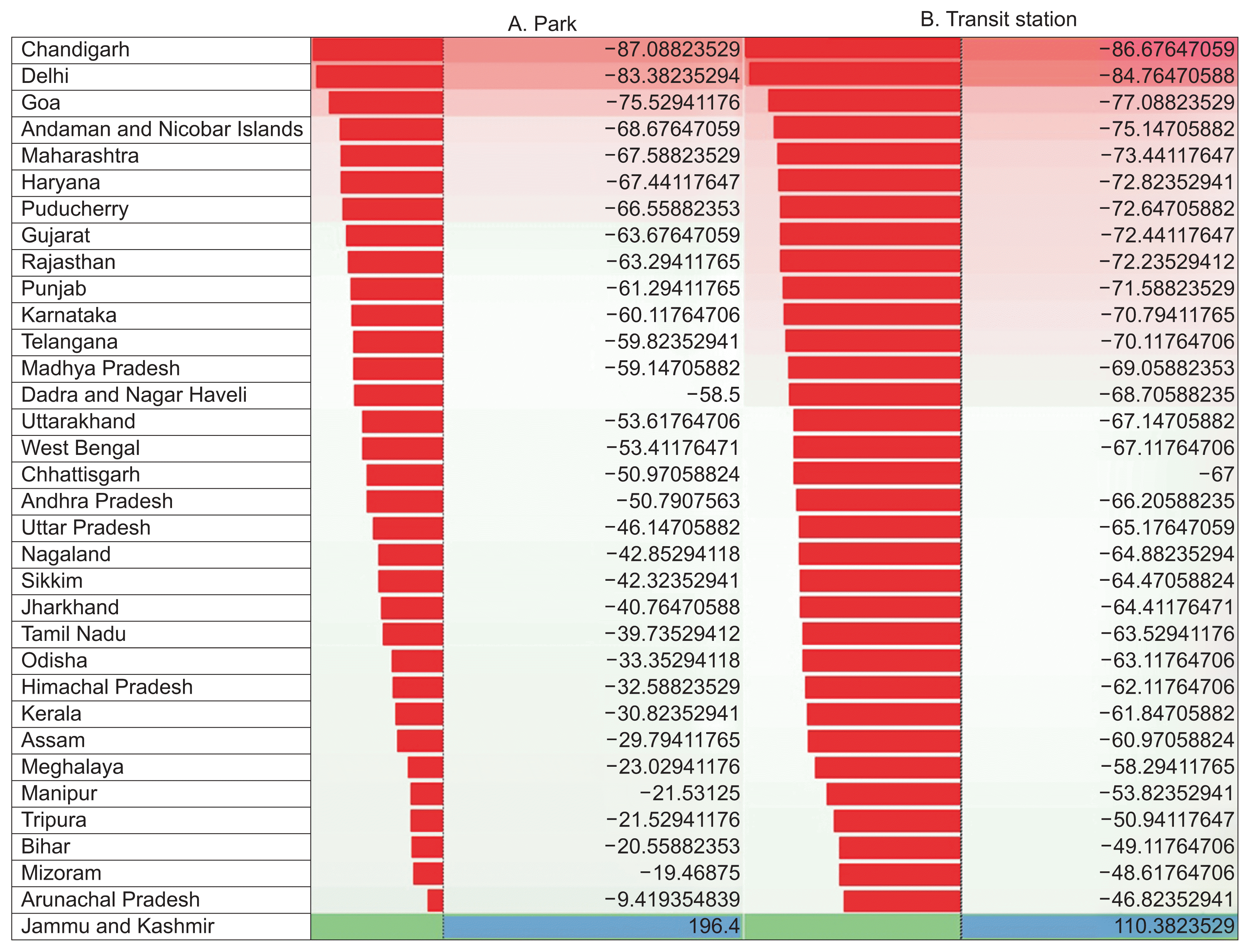
Figure 5
Statewise mean percentage changes in (A) workplace and (B) residential mobility patterns during the lockdown compared to baseline.
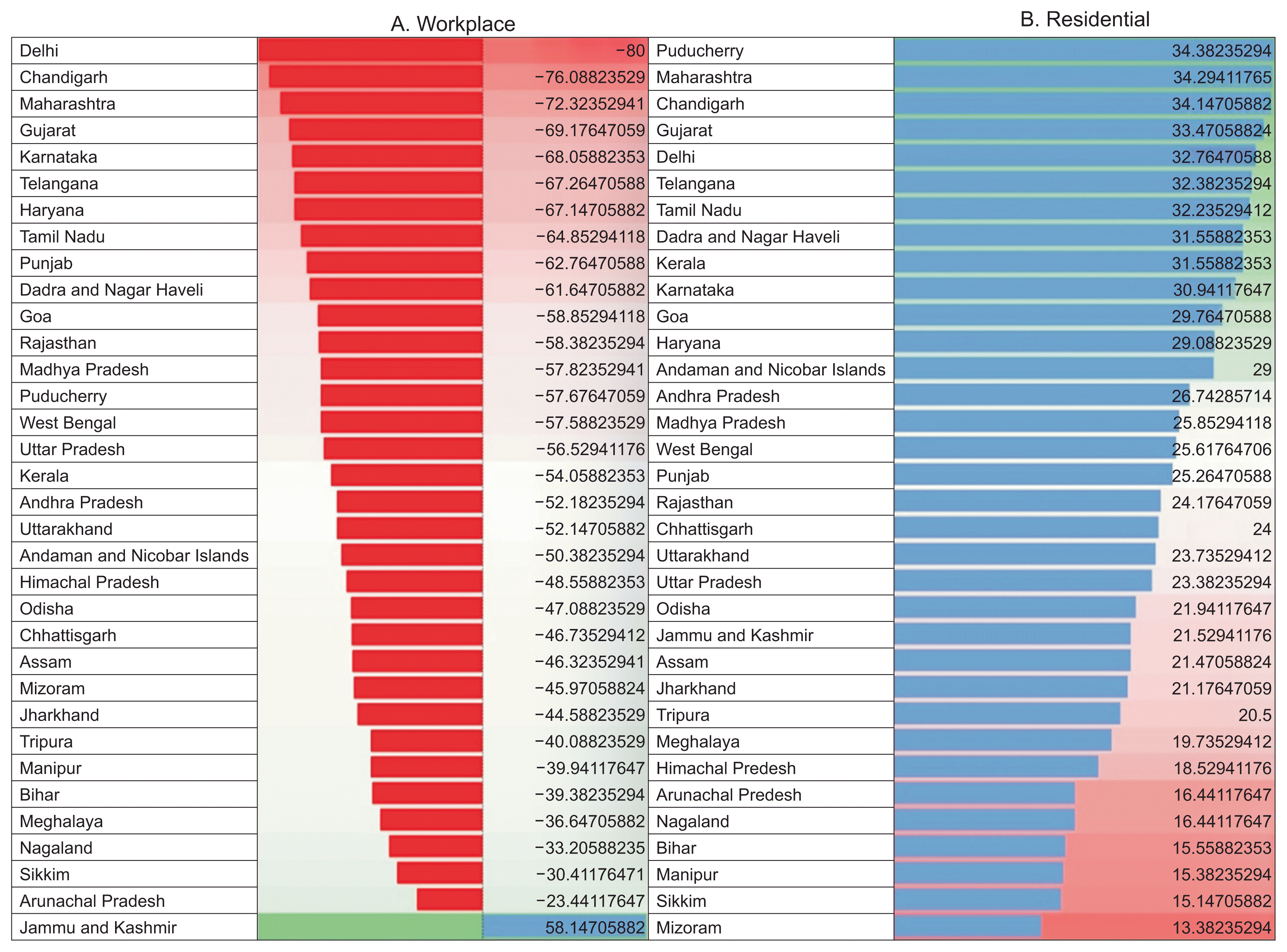
Figure 8
Correlation matrix of changes in mobility patterns between various place categories in India.

Table 1
Correlations between the doubling time, stringency index, and community mobility patterns