Abstract
Background
Methods
Results
Supplementary Materials
Supplementary Table 1.
Supplementary Table 2.
Supplementary Fig. 1.
Supplementary Fig. 2.
Supplementary Fig. 3.
Notes
ACKNOWLEDGMENTS
REFERENCES

































Fig. 1.
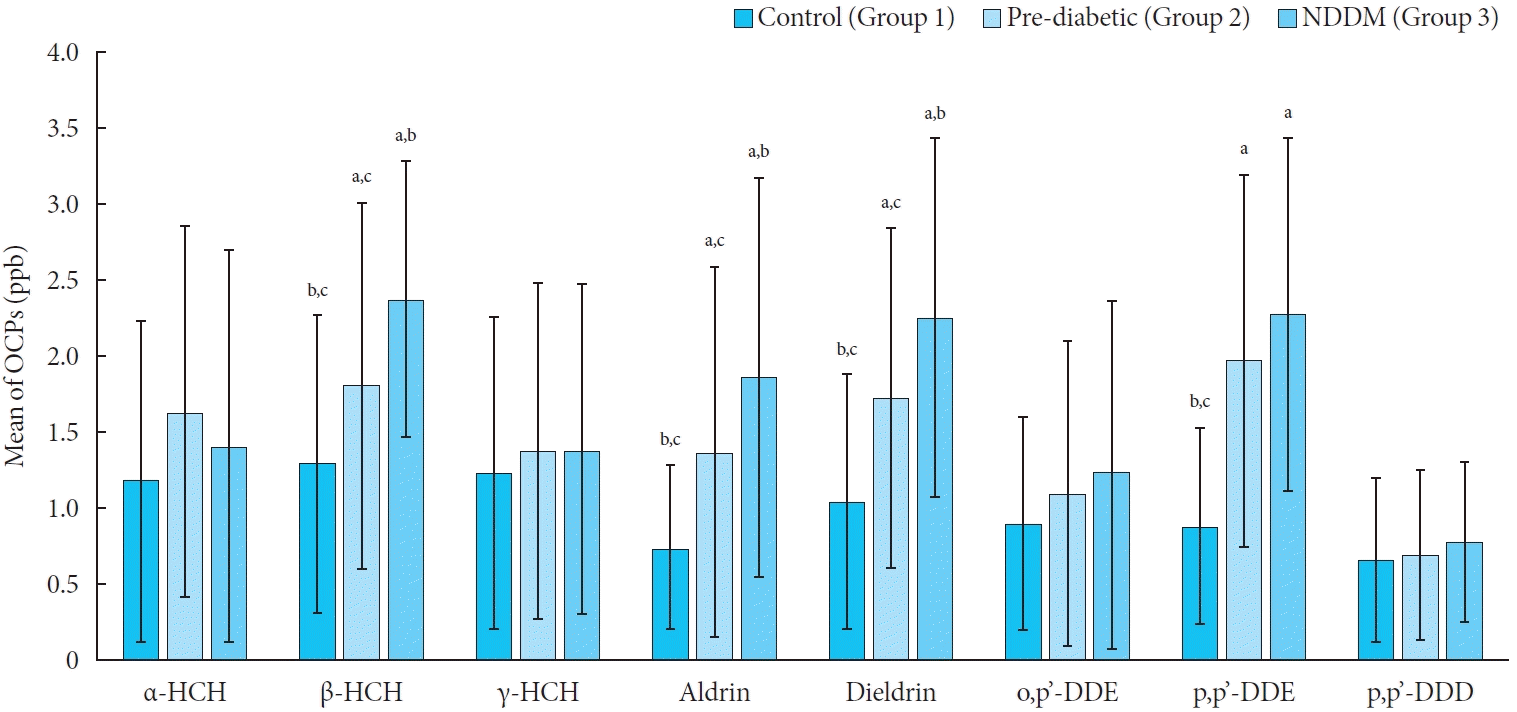
Table 1.
Parameter | Control (Group 1) (n=100) | Prediabetes (Group 2) (n=100) | Newly detected DM (Group 3) (n=100) | P value |
---|---|---|---|---|
Sex | 0.621 | |||
Male | 47 | 56 | 43 | |
Female | 53 | 44 | 57 | |
Educational type | 0.040a | |||
Illiterate | 41 | 31 | 59 | |
0–5 | 5 | 8 | 11 | |
5–10 | 25 | 26 | 6 | |
10–12 | 29 | 22 | 17 | |
Graduate or above | 0 | 13 | 7 | |
Occupation | 0.001a | |||
Working | 63 | 57 | 36 | |
Non-working | 37 | 43 | 64 | |
Food habit | 0.018a | |||
Vegetarian | 47 | 49 | 30 | |
Non-vegetarian | 53 | 51 | 70 | |
Fat, /mo | 0.001a | |||
0–200 g | 28 | 33 | 56 | |
200–500 g | 3 | 5 | 8 | |
600–1,500 g | 25 | 31 | 17 | |
1,500 g and above | 44 | 31 | 19 | |
Source of drinking water | 0.041a | |||
Tap water | 74 | 64 | 59 | |
Ground water | 7 | 9 | 14 | |
Filter water | 19 | 27 | 27 |
Table 2.
Parameter | Control (Group 1) (n=100) | Prediabetes (Group 2) (n=100) | Newly detected DM (Group 3) (n=100) | P value |
---|---|---|---|---|
Age, yr | 43.90±6.79 | 44.50±6.47 | 43.78±7.67 | 1 vs. 2=0.639 |
1 vs. 3=0.927 | ||||
2 vs. 3=0.575 | ||||
BMI, kg/m2 | 28.23±6.02 | 31.05±5.72 | 34.1±0.72 | 1 vs. 2=0.025a |
1 vs. 3 <0.001a | ||||
2 vs. 3 <0.001a | ||||
Waist, cm | 92.85±16.38 | 95.78±15.51 | 101.70±12.42 | 1 vs. 2=0.281 |
1 vs. 3=0.001a | ||||
2 vs. 3=0.030a | ||||
Systolic blood pressure, mm Hg | 127.42±9.63 | 127.92±8.59 | 129.51±10.13 | 1 vs. 2=0.675 |
1 vs. 3=0.088 | ||||
2 vs. 3=0.425 | ||||
Diastolic blood pressure, mm Hg | 77.09±11 | 78.58±10.58 | 78.92±11 | 1 vs. 2=0.589 |
1 vs. 3=0.678 | ||||
2 vs. 3=0.485 | ||||
HbA1c, % | 5.24±0.31 | 5.51±0.52 | 7.14±1.75 | 1 vs. 2=0.171 |
1 vs. 3 <0.001a | ||||
2 vs. 3 <0.001a | ||||
Glucose fasting, mg/dL | 86.52±6.59 | 97.68±11.38 | 138.30±53.53 | 1 vs. 2=0.05a |
1 vs. 3 <0.001a | ||||
2 vs. 3 <0.001a | ||||
Glucose PP, mg/dL | 107.90±17.79 | 154.58±22.39 | 285.25±93.85 | 1 vs. 2 <0.001a |
1 vs. 3 <0.001a | ||||
2 vs. 3 <0.001a | ||||
Insulin, mIU/L | 11.44±5.77 | 14.52±11.47 | 30.38±10.60 | 1 vs. 2=0.081 |
1 vs. 3 <0.001a | ||||
2 vs. 3 <0.001a | ||||
HOMA-IR | 2.45±1.27 | 3.52±2.85 | 11.06±7.59 | 1 vs. 2=0.220 |
1 vs. 3 <0.001a | ||||
2 vs. 3 <0.001a | ||||
TC, mg/dL | 183.31 ±67.19 | 192.67±47.63 | 194.48±44.36 | 1 vs. 2=0.344 |
1 vs. 3=0.259 | ||||
2 vs. 3=0.854 | ||||
TG, mg/dL | 141.02±66.09 | 152.33±69.53 | 166.96±74.49 | 1 vs. 2=0.378 |
1 vs. 3=0.044a | ||||
2 vs. 3=0.255 | ||||
HDL-C, mg/dL | 44.85±10.52 | 43.92±13.16 | 42.53±9.06 | 1 vs. 2=0.644 |
1 vs. 3=0.252 | ||||
2 vs. 3=0.494 | ||||
LDL-C, mg/dL | 112.03±48.05 | 114.04±49.38 | 127.98±69.75 | 1 vs. 2=0.337 |
1 vs. 3=0.025a | ||||
2 vs. 3=0.196 |
Values are presented as mean±standard deviation.
DM, diabetes mellitus; BMI, body mass index; HbA1c, glycosylated hemoglobin; PP, postprandial; HOMA-IR, homeostasis model assessment of insulin resistance; TC, total cholesterol; TG, total triglycerides; HDL-C, high-density lipoprotein cholesterol; LDL-C, low-density lipoprotein cholesterol.
Table 3.
Parameter | Correlation | BMI | Waist | Hb1Ac | Fasting | PP | TC | TG | HDL-C | Insulin | HOMA-IR |
---|---|---|---|---|---|---|---|---|---|---|---|
α-HCH | P value | 0.001 | 0.39 | 0.48 | 0.84 | 0.79 | 0.73 | 0.77 | 0.30 | 0.97 | 0.90 |
r | 0.24a | –0.06 | 0.05 | 0.01 | 0.02 | 0.02 | 0.02 | –0.07 | –0.003 | –0.009 | |
β-HCH | P value | 0.005 | 0.66 | 0.22 | 0.01 | 0.00 | 0.83 | 0.50 | 0.03 | 0.00 | 0.002 |
r | 0.21a | 0.03 | 0.09 | 0.19b | 0.29a | –0.02 | –0.05 | –0.16b | 0.26a | 0.23a | |
γ-HCH | P value | 0.12 | 0.49 | 0.76 | 0.64 | 0.93 | 0.78 | 0.43 | 0.22 | 0.49 | 0.54 |
r | 0.11 | –0.05 | –0.02 | 0.03 | 0.006b | 0.021 | –0.05 | –0.09 | 0.05b | 0.05b | |
Aldrin | P value | 0.001 | 0.16 | 0.05 | 0.05 | 0.007 | 0.73 | 0.45 | 0.89 | 0.05 | 0.08 |
r | 0.23a | 0.10 | 0.15b | 0.14 | 0.20a | –0.02 | 0.06 | –0.01 | 0.14 | 0.13 | |
Dieldrin | P value | 0.88 | 0.82 | 0.00 | 0.00 | 0.00 | 0.89 | 0.85 | 0.04 | 0.008 | 0.003 |
r | 0.01 | 0.02 | 0.27a | 0.26a | 0.31a | –0.01 | –0.01 | –0.15b | 0.19a | 0.22a | |
o,p’-DDE | P value | 0.31 | 0.84 | 0.56 | 0.85 | 0.63 | 0.55 | 0.30 | 0.33 | 0.04 | 0.34 |
r | –0.08 | 0.01 | 0.04 | 0.01 | 0.04 | –0.04 | 0.07 | –0.07 | 0.15b | 0.07 | |
p,p’-DDE | P value | 0.96 | 0.47 | 0.006 | 0.22 | 0.00 | 0.77 | 0.48 | 0.56 | 0.11 | 0.44 |
r | 0.004 | 0.05 | 0.20a | 0.09 | 0.26a | 0.02 | 0.05 | 0.04 | 0.12 | 0.06 | |
p,p’-DDD | P value | 0.43 | 0.96 | 0.44 | 0.84 | 0.49 | 0.46 | 0.37 | 0.37 | 0.73 | 0.93 |
r | 0.06 | 0.004 | –0.06 | 0.01 | 0.05 | –0.05 | –0.07 | –0.07 | –0.03 | –0.007 |
BMI, body mass index; HbA1c, glycosylated hemoglobin; PP, postprandial; TC, total cholesterol; TG, total triglycerides; HDL-C, high-density lipoprotein cholesterol; HOMA-IR, homeostasis model assessment of insulin resistance; HCH, hexachlorocyclohexane; DDE, dichloro-diphenyl-dichloroethylene; DDD, dichloro-diphenyl-dichloroethane.
Table 4.
Parameter |
Model 1 |
Model 2 |
Model 3 |
Model 4 |
||||
---|---|---|---|---|---|---|---|---|
OR (95% CI) | P value | OR (95% CI) | P value | OR (95% CI) | P value | OR (95% CI) | P value | |
α-HCH | 1.19 (0.64–2.19) | 0.579 | 1.17 (0.63–2.19) | 0.685 | 1.12 (0.60–2.08) | 0.719 | 1.31 (0.67–2.52) | 0.427 |
β-HCH | 2.63 (1.35–5.12) | 0.004a | 2.70 (1.38–5.29) | 0.004a | 2.88 (1.45–5.71) | 0.002a | 2.40 (1.18–4.87) | 0.015b |
γ-HCH | 1.14 (0.62–2.12) | 0.674 | 1.22 (0.64–2.32) | 0.545 | 1.08 (0.58–2.02) | 0.806 | 0.92 (0.48–1.75) | 0.398 |
Aldrin | 1.45 (0.76–2.76) | 0.263 | 1.60 (0.81–3.17) | 0.176 | 1.49 (0.77–2.86) | 0.232 | 0.97 (0.50–1.88) | 0.934 |
Dieldrin | 2.83 (1.46–5.49) | 0.002a | 2.83 (1.46–5.52) | 0.002a | 2.71 (1.39–5.31) | 0.004a | 2.18 (1.10–4.33) | 0.025b |
o,p’-DDE | 1.23 (0.67–2.27) | 0.506 | 1.23 (0.66–2.27) | 0.520 | 1.31 (0.70–2.43) | 0.398 | 1.16 (0.61–2.23) | 0.643 |
p,p’-DDE | 2.49 (1.28–4.85) | 0.007a | 2.55 (1.29–5.02) | 0.007a | 2.63 (1.33–5.19) | 0.005a | 1.76 (0.89–3.51) | 0.050b |
p,p’DDD | 0.77 (0.42–1.42) | 0.398 | 0.81 (0.43–1.51) | 0.506 | 0.81 (0.43–1.49) | 0.494 | 1.14 (0.59–2.19) | 0.698 |
Model 1: crude ratio; Model 2: adjusted for age and body mass index; Model 3: adjusted for triglycerides and cholesterol; Model 4: adjusted for demographic data.
OR, odds ratio; CI, confidence interval; HCH, hexachlorocyclohexane; DDE, dichloro-diphenyl-dichloroethylene; DDD, dichloro-diphenyl-dichloroethane.
Table 5.
Parameter |
BMI <23 kg/m2 |
BMI ≥23 and ≤25 kg/m2 |
BMI >25 kg/m2 |
|||
---|---|---|---|---|---|---|
OR (95% CI) | P value | OR (95% CI) | P value | OR (95% CI) | P value | |
α-HCH | 0.60 (0.09–3.98) | 0.597 | 1.78 (0.87–3.64) | 0.109 | 0.36 (0.06–2.15) | 0.262 |
β-HCH | 1.80 (0.85–3.80) | 0.012a | 4.00 (0.50–24.98) | 0.191 | 10.05 (1.36–43.05) | 0.024a |
γ-HCH | 1.50 (0.23–9.79) | 0.672 | 0.97 (0.48–1.97) | 0.947 | 0.52 (0.067–4.0) | 0.529 |
Aldrin | 1.50 (0.37–12.8) | 0.347 | 0.80 (0.38–1.66) | 0.550 | 0.40 (0.03–5.25) | 0.485 |
Dieldrin | 1.40 (0.19–2.43) | 0.738 | 1.95 (0.95–3.98) | 0.046a | 7.00 (0.97–30.57) | 0.050a |
o,p’-DDE | 0.90 (0.13–6.08) | 0.914 | 1.50 (0.74–6.05) | 0.262 | 0.375 (0.61–4.30) | 0.290 |
p,p’-DDE | 1.33 (0.14–7.37) | 0.800 | 1.29 (0.59–8.82) | 0.050a | 10.66 (1.38–36.03) | 0.013a |
p,p’-DDD | 2.40 (0.29–10.78) | 0.416 | 1.21 (0.59–3.45) | 0.601 | 1.78 (0.28–11.1) | 0.538 |