Abstract
Purpose
Both low cardiorespiratory fitness (CRF) and obesity may hasten vascular aging and increase atherosclerotic cardiovascular disease (ASCVD) risk. This study explored the joint association of CRF and obesity with ASCVD risk using the fitness fatness index (FFI) and measures of vascular aging derived from blood pressure in a large sample of United States adults.
Methods
Data from the 1999−2002 National Health and Nutrition Examination Survey were used (N=8,080 adults between the ages of 20−85 years, which is a weighted population equivalent of 174,277,151). The FFI was calculated as CRF divided by waist-to-height ratio. Measures of vascular aging included estimated pulse wave velocity (ePWV), pulse pressure (PP), and the vascular overload index (VOI), with ePWV being calculated from systolic and diastolic blood pressures and age while PP and VOI were calculated from blood pressure alone.
Results
After full adjustment for age, sex, race/ethnicity, education, body mass index, smoking, physical activity, diabetes, hypertension, cardiovascular disease, high-density lipoprotein cholesterol, and total cholesterol, FFI was inversely associated with ePWV (b=−.10; 95% confidence interval [CI], −0.11 to −0.09; p<0.001), PP (b=−.86; 95% CI, −1.03 to −0.68; p< 0.001) and the VOI (b=−.74; 95% CI, −0.95 to −0.053; p< 0.001).
Conclusion
FFI is associated with lower ePWV, PP, and VOI in a nationally representative sample of adults. Maintaining higher CRF concomitant with lower body fat may minimize vascular load and contribute to healthy vascular aging. Conversely, lower fitness and higher fatness may contribute to early/accelerated vascular aging.
Cardiorespiratory fitness (CRF) is a powerful predictor of atherosclerotic cardiovascular disease (ASCVD) risk, with higher CRF being cardioprotective and lower CRF serving as a potent incendiary for cardiometabolic disease, morbidity, and mortality1. While the prevalence of low CRF is high2, so too is the prevalence of obesity. Obesity levels have reached epidemic proportions, also serving to increase ASCVD risk and cardiovascular disease (CVD)- and all-cause mortality in the general population3. Thus, the consideration of both CRF and obesity together may offer more insight into ASCVD risk than either alone.
The fitness fatness index (FFI) combines measures of CRF and fatness into a single continuous metric. CRF is determined from a validated algorithm recently shown in a meta-analysis to be an independent predictor of cardiovascular- and all-cause mortality4. Fatness is assessed using the waist-to-height ratio (WHtR), overcoming many of the potential limitations of using body mass index (BMI) as a measure of fatness5. WHtR is an anthropometric index known to be universally appropriate across race, age, sex, and genetic predisposition to changes in body weight and CRF level6. Moreover, the WHtR may be more closely associated with cardiometabolic risk and may be a better predictor of visceral fat than other anthropometric measures such as BMI7. Thus, the FFI has been shown to be a better predictor of incident diabetes than either CRF or obesity alone8,9, with recent studies supporting the FFI as predictive of CVD-specific and all-cause mortality in adults with CVD and the general population alike10,11. Whether the FFI is sensitive to differences in subclinical ASCVD risk remains unexplored. Such an evaluation may provide insight into the explanation of the previously observed effects between FFI and CVD- and all-cause mortality.
Measures of vascular aging offer novel insight into ASCVD risk. With aging and/or the presence of disease, large (central) arteries, such as the aorta, stiffen, exposing target organs and the downstream microvasculature to potentially damaging pulsatile hemodynamic forces12. Excessive pulsatility contributes to vascular remodeling and increases in arteriolar resistance13, which augments pressure from wave reflections, leading to further increases in pressure pulsatility. The sum effect of this hemodynamic sequela (i.e., increased arterial stiffness and pulse pressure [PP]) has been referred to as vascular overload14. Increased arterial stiffness and PP are each associated with subclinical target organ damage and future CVD risk12. We and others have found that while higher CRF is associated with lower arterial stiffness15, obesity is associated with increased arterial stiffness16.
We sought to explore the joint association of CRF and body fatness with ASCVD risk. To this end, we examined the association of FFI with estimated pulse wave velocity (ePWV), PP, and the vascular overload index (VOI) as measures of vascular aging derived from brachial blood pressure (BP) that capture the overall burden of arterial stiffness and BP pulsatility on the cardiovascular system (i.e., vascular overload). We hypothesized that a higher FFI would be associated with lower ePWV, PP, and thus, a lower VOI. We tested this hypothesis in a large, representative sample of the United States adults from the 1999–2002 National Health and Nutrition Examination Survey (NHANES; N=8,080 which is a weighted population equivalent of 174,277,151).
Data from the 1999–2002 NHANES were extracted. Analyses are based on complete data from 8,080 male and female (aged 20–85 years). All NHANES procedures have been ethically approved by the National Center for Health Statistics review board. Prior to any data collection, participant consent was obtained from all individuals. Additional information on NHANES methodology and data collection can be obtained from the NHANES website (http://www.cdc.gov/nchs/nhanes.htm). Further, all NHANES survey response rates have been published elsewhere (https://wwwn.cdc.gov/nchs/nhanes/ResponseRates.aspx).
FFI was taken as the ratio of CRF to WHtR. The WHtR was assessed directly from measured height and waist circumference measured just above the ilium using standard procedures. CRF was determined from a prediction algorithm that uses non-exercise testing methods to estimate a CRF level. These algorithms, which demonstrate evidence of predictive validity, have been shown to inversely associated with all-cause and CVD-specific mortality. The specific algorithms used were as follows: estimated CRF for female (METs)=14.7873+(age×0.1159)–(age2×0.0017)–(BMI× 0.1534)–(WC×0.0085)–(RHR×0.0364)+(active×0.5987)–(smoker×0.2994); estimated CRF for male (MET)=21.2870+(age×0.1654)–(age2×0.0023)–(BMI×0.2318)–(WC×0.0337)–(RHR×0.0390)+(active×0.6351)–(smoker×0.4263).
In this algorithm, MET represents metabolic equivalent of task, RHR represents resting heart rate (bpm), and WC represents waist circumference (cm). Smoking status was determined based on self-report; current smoking was coded as 1, and otherwise, it was coded as 0. Activity status was determined from self-report and defined as ≥2,000 MVPA MET-min-month; MVPA represents moderate-to-vigorous physical activity. Those above this threshold were coded as 1 and others were coded as 0. The BMI (kg/m2) was determined here from measured height and weight.
ePWV was determined from the following algorithm17: 9.587–(0.402×age)+(4.560×0.001×age2)–(2.621×0.00001×age2×MBP)+(3.176×0.001×age×MBP)–(1.832×0.01×MBP). In this algorithm, age was expressed in years and mean BP (MBP) was calculated as [(DBP)+{0.4×(SBP–DBP)}], where DBP is diastolic BP and SBP is systolic BP. PP was calculated as SBP–DBP (above).
The VOI was calculated as follows14: 1.33×SBP–0.33×DBP–133.3.
BP was obtained with participants in the seated position following 5 minutes of quiet rest. NHANES technicians completed a thorough BP training program (Shared Care Research and Education Consulting) that involved a didactic section, audio-video observation, and measurement of BP against a certified, gold standard BP instructor. For the calculation of MBP, the average of up to four BP assessments was utilized.
Covariates included various demographic, behavioral, and CVD risk factors that could confound the association between FFI and measures of vascular aging. These included age (years), sex (male/female), race-ethnicity (Mexican American, other Hispanic, non-Hispanic white, non-Hispanic black, and multi-race), education (college graduate or not), self-reported smoking status (smoker vs. nonsmoker), self-reported physical activity (MET- min-month), measured BMI (kg/m2), physician-diagnosed diabetes/ use of diabetes medication (yes/no), physician-diagnosed hypertension/ use of anti-hypertensives (yes/no), physician-diagnosed CVD, and laboratory-assessed CVD biomarkers, including high-density lipoprotein cholesterol (mg/dL), total cholesterol (mg/dL), and C-reactive protein (CRP) (mg/dL). Specific details on the methodological assessment of these covariates can be found on the NHANES website (http://www.cdc.gov/nchs/nhanes.htm).
All statistical analyses were performed via complex survey analytical procedures using Stata version 12 (SAS Institute, Cary, NC, USA). To account for oversampling, nonresponse, noncoverage, and to provide nationally representative estimates, all analyses included the use of survey sample weights, clustering and primary sampling units.
Weighted multivariable linear regression models were used to examine the association between FFI as the independent variable with ePWV, PP, and the VOI as three separate dependent variables. Three specific models were explored, including (1) unadjusted, (2) minimally adjusted for demographic covariates (age, sex, race-ethnicity, and education), and (3) fully adjusted for demographic variables along with behavioral and CVD risk factors (BMI, smoking, physical activity, diabetes, hypertension, CVD, HDL cholesterol, total cholesterol, and CRP). To specifically explore the potential moderating impact of sex on our findings, we created a cross-product term (sex*FFI) and included it, along with its main effects, in a regression; and if significant, separate sex-specific models were explored in each of three separate regression models for ePWV, PP, and VOI. For all analyses, statistical significance was established as a nominal alpha of 0.05.
Table 1 displays the characteristics of the sample. Participants, on average, were middle age (46 years), predominately white (72.7%), and both sexes were equally represented (51.7% of females). A visual depiction of the relationship between FFI and ePWV is shown in Fig. 1. After adjusting for all the independent variables noted in Table 1, FFI was inversely associated with ePWV (b=–.10; 95% confidence interval [CI], –0.11 to –0.09; p<0.001). Results were similar in an unadjusted model (b=–.15; 95% CI, –0.16 to –0.13; p<0.001), as well as a minimally adjusted model controlling for age, sex, race-ethnicity, and education (b=–.028; 95% CI, –0.034 to –0.023; p<0.001). The sex*FFI interaction term was statistically significant (p<0.001); therefore, we computed separate sex-specific models (fully adjusting for all covariates) for male and female. In the fully adjusted models, FFI on ePWV for male was b=–.117 (95% CI, –0.13 to –0.10; p<0.001); and for female, it was b=–.175 (95% CI, –0.19 to –0.15; p<0.001).
A visual depiction of the relationship between FFI and PP is shown in Fig. 2. After adjusting for all the independent variables noted in Table 1, FFI was inversely associated with PP (b=–.86; 95% CI, –1.03 to –0.68; p<0.001). Results were similar in an unadjusted model (b=–.93; 95% CI, –1.01 to –0.85; p<0.001), as well as a minimally adjusted model controlling for age, sex, race-ethnicity, and education (b=–.28; 95% CI, –0.38 to –0.18; p<0.001). The sex*FFI interaction term was statistically significant, (p<0.001), therefore, we computed separate sex-specific models (fully adjusting for all covariates) for male and female. In the fully adjusted models, FFI on PP for male was b=–1.10 (95% CI, –1.30 to –0.90; p<0.001); and for female, it was b=–1.89 (95% CI, –2.22 to –1.56; p<0.001).
A visual depiction of the relationship between FFI and VOI is shown in Fig. 3. After adjusting for all the independent variables noted in Table 1, FFI was inversely associated with VOI (b=–.74; 95% CI, –0.95 to –0.53; p<0.001). Results were similar in an unadjusted model (b=–1.26; 95% CI, –1.35 to –1.17; p<0.001), as well as a minimally adjusted model controlling for age, sex, race-ethnicity, and education (b=–.60; 95% CI, –0.74 to –0.45; p<0.001). The sex*FFI interaction term was statistically significant (p<0.001); therefore, we computed separate sex- specific models (fully adjusting for all covariates) for males and females. In the fully adjusted models, FFI on the VOI for male was b=–1.09 (95% CI, –1.35 to –0.83; p<0.001); and for female, it was b=–1.67 (95% CI, –2.07 to –1.25; p<0.001).
Studies that explore the interaction between CRF and obesity as related to ASCVD risk typically categorize individuals into broad fit, unfit, obese, and/or nonobese groups (e.g., fat-but-fit) using somewhat arbitrary operational definitions and cut points. For example, body fatness is typically operationalized using BMI. BMI is regularly questioned as the most parsimonious way to appraise obesity status at the population level owing to concerns around it accurately reflecting adiposity and body composition (lean mass) across age, sex, race/ethnicity, etc.5. Moreover, using such an “and/or” categorical approach may obscure more subtle intraindividual variation in fitness-fatness interactions and only offer insight into more robust shifts in risk across/between categories9. Changes in fitness and fatness that may take place with lifestyle modification often occur concomitantly and on a continuum. A better understanding of the synergistic effects of CRF and obesity as continuous variables may be needed to inform more effective clinical practice. Thus, the FFI has been put forth as a metric to capture the joint contribution of CRF and obesity status on chronic disease risk and is emerging as a useful tool to predict cardiometabolic disease and mortality.
Our findings extend the utility of the FFI as a measure of ASCVD risk by noting associations with three separate measures of vascular aging. We found that higher FFI was independently and inversely associated with ePWV, PP, and the VOI in a large representative group of US adults from NHANES. Associations remained after adjusting for numerous covariates, including age, sex, race/ethnicity, and CVD risk factors like BMI, lipids, and CRP. Moreover, according to sex-specific analyses, while FFI was inversely associated with ePWV, PP, and VOI in both male and female, the magnitude of associations was stronger for female compared to male. These findings are taken to suggest that the combination of higher CRF and lower body fat is associated with more optimal vascular aging and by extension lower ASCVD risk.
Arterial stiffening is considered a hallmark of the vascular aging process. The gold standard method for the assessment of aortic stiffness is carotid-femoral pulse wave velocity (cfPWV)12. cfPWV can be reasonably estimated from age and mean arterial pressure18 and this ePWV has been shown to predict CVD- and all-cause mortality independent of traditional CVD risk factors19. Age-associated reductions in arterial elasticity and loss of the intrinsic buffering capacity of large arteries owing to increased arterial stiffness contribute to an age-dependent widening of pressure pulsatility captured as PP. Elevated PP also increases ASCVD risk measured as vascular calcification20 and is an independent predictor of cardiovascular mortality. The VOI embraces both the steady (i.e. mean arterial pressure) and pulsatile (i.e., PP) components of BP and captures the effective incremental contribution of arterial stiffness, wave reflection, and arteriolar resistance to overall systolic BP14. The VOI has been shown to be associated with cfPWV21, detrimental vascular remodeling, and target organ damage (i.e. reduced brachial artery flow-mediated dilation and increased carotid intima-media thickness)21, and incident stroke22. Taken together, our findings demonstrate that FFI is independently and favorably associated with measures of vascular aging in a large sample of United States adults from NHANES.
Emerging studies note sexual dimorphism in the association of CRF and body fatness with morbidity and mortality23-26. Therefore, we explored sex-specific associations between FFI and our hemodynamic outcomes. Our findings suggest possible sex differences in the association of FFI with vascular aging. Although a higher FFI is associated with lower arterial stiffness and vascular overload in both male and female, the favorable effect of FFI on vascular aging may be stronger in female. This finding is important to note because compared to male: (1) age-associated increases in arterial stiffness and PP are greater in female; (2) the association between arterial stiffness and target organ damage, such as left ventricular hypertrophy, is greater in female; (3) the association between arterial stiffness and mortality is nearly twofold higher in female27,28. Thus, our findings may have important implications for sex differences in vascular aging and subsequent ASCVD risk. More research will be needed to examine optimal combinations of CRF and body fatness for preservation of vascular health in male and female.
The FFI has previously been shown to be inversely associated with CRP29, an important correlate of ASCVD. In our study, associations between FFI and vascular aging remained after adjusting for CRP, suggesting that favorable associations may be related to pathways extending beyond systemic inflammation, or perhaps, in the present study, CRP was not fully reflective of systemic inflammation. It is also interesting to note that associations remained after adjusting for BMI, confirming the unique contribution of WHtR to the strength of FFI in assessing ASCVD risk. Examination of the mechanisms responsible for individual contributions of high CRF, obesity status, and FFI to vascular aging are beyond the scope of this study. Higher CRF coupled with more ideal body habitus may be associated with favorable shifts in autonomic function, metabolic regulation, lipid metabolism, endothelial function, and vascular calcification; all of which may interact to favorably affect arterial stiffness and hemodynamic load30. Parenthetically, our results also support that those individuals with both low CRF, and high obesity status may be more susceptible to early vascular aging, which may drastically increase ASCVD risk.
Limitations of this study should be explored. We used an estimate of CRF derived from an established equation and not objectively measured VO2 from metabolic gas analysis. Nonetheless, estimated CRF has been shown to be a valid indirect proxy of directly measured CRF and is independently associated with CVD- and all-cause mortality4. Measures of vascular aging were all derived from systolic and diastolic BP and are likely capturing a similar hemodynamic epiphenomenon related to arterial stiffness and possibly not arterial stiffness per se. Future studies using cfPWV or brachial-ankle pulse wave velocity to measure arterial stiffness along with other established measures of vascular aging, such as carotid intima-media thickness, will be needed to confirm findings herein. The equations to estimate both FFI and ePWV included age. Although we additionally adjusted for age in our statistical models, care should be taken when interpreting our findings. To circumvent concern, we included two additional measures of vascular aging and ASCVD risk not derived from age. Associations between FFI and all three measures of vascular aging were comparable. Further, models without the control of age also produced similar findings.
In conclusion, we found that FFI was inversely associated with ePWV, PP, and the VOI in a large representative sample of the United States adults from NHANES. Maintaining higher CRF and lower body fat promotes healthy vascular aging while having both lower CRF and higher obesity may contribute to early vascular aging. The FFI may be a useful index to assess ASCVD risk via its association with vascular aging in the general population.
REFERENCES
1. Barry VW, Caputo JL, Kang M. 2018; The joint association of fitness and fatness on cardiovascular disease mortality: a meta-analysis. Prog Cardiovasc Dis. 61:136–41. DOI: 10.1016/j.pcad.2018.07.004. PMID: 29981352.


2. Carnethon MR, Gulati M, Greenland P. 2005; Prevalence and cardiovascular disease correlates of low cardiorespiratory fitness in adolescents and adults. JAMA. 294:2981–8. DOI: 10.1001/jama.294.23.2981. PMID: 16414945.


3. Dwivedi AK, Dubey P, Cistola DP, Reddy SY. 2020; Association between obesity and cardiovascular outcomes: updated evidence from meta-analysis studies. Curr Cardiol Rep. 22:25. DOI: 10.1007/s11886-020-1273-y. PMID: 32166448.


4. Qiu S, Cai X, Sun Z, Wu T, Schumann U. 2021; Is estimated cardiorespiratory fitness an effective predictor for cardiovascular and all-cause mortality? A meta-analysis. Atherosclerosis. 330:22–8. DOI: 10.1016/j.atherosclerosis.2021.06.904. PMID: 34225102.


5. Donini LM, Pinto A, Giusti AM, Lenzi A, Poggiogalle E. 2020; Obesity or BMI paradox? Beneath the tip of the iceberg. Front Nutr. 7:53. DOI: 10.3389/fnut.2020.00053. PMID: 32457915. PMCID: PMC7221058.


6. Schneider HJ, Friedrich N, Klotsche J, et al. 2010; The predictive value of different measures of obesity for incident cardiovascular events and mortality. J Clin Endocrinol Metab. 95:1777–85. DOI: 10.1210/jc.2009-1584. PMID: 20130075.


7. Ashwell M, Gunn P, Gibson S. 2012; Waist-to-height ratio is a better screening tool than waist circumference and BMI for adult cardiometabolic risk factors: systematic review and meta-analysis. Obes Rev. 13:275–86. DOI: 10.1111/j.1467-789X.2011.00952.x. PMID: 22106927.


8. Sloan RA, Sawada SS, I-Min L, et al. 2018; The association of Fit-Fat Index with incident diabetes in Japanese men: a prospective cohort study. Sci Rep. 8:569. DOI: 10.1038/s41598-017-18898-3. PMID: 29330373. PMCID: PMC5766556.


9. Sloan RA, Haaland BA, Sawada SS, et al. 2016; A Fit-Fat Index for predicting incident diabetes in apparently healthy men: a prospective cohort study. PLoS One. 11:e0157703. DOI: 10.1371/journal.pone.0157703. PMID: 27340824. PMCID: PMC4920380.


10. Edwards MK, Addoh O, Loprinzi PD. 2017; Predictive validity of a fitness fatness index in predicting cardiovascular disease and all-cause mortality. Mayo Clin Proc. 92:851. DOI: 10.1016/j.mayocp.2017.02.013. PMID: 28473044.


11. Frith E, Loprinzi PD. 2017; The protective effects of a novel fitness-fatness index on all-cause mortality among adults with cardiovascular disease. Clin Cardiol. 40:469–73. DOI: 10.1002/clc.22679. PMID: 28295468. PMCID: PMC6490318.


12. Townsend RR, Wilkinson IB, Schiffrin EL, et al. 2015; Recommendations for improving and standardizing vascular research on arterial stiffness: a scientific statement from the American Heart Association. Hypertension. 66:698–722. DOI: 10.1161/HYP.0000000000000033. PMID: 26160955. PMCID: PMC4587661.
13. Mitchell GF. 2008; Effects of central arterial aging on the structure and function of the peripheral vasculature: implications for end-organ damage. J Appl Physiol (1985). 105:1652–60. DOI: 10.1152/japplphysiol.90549.2008. PMID: 18772322. PMCID: PMC2584844.


14. Franklin SS, Weber MA. 1994; Measuring hypertensive cardiovascular risk: the vascular overload concept. Am Heart J. 128:793–803. DOI: 10.1016/0002-8703(94)90278-X. PMID: 7942450.


15. Augustine JA, Yoon ES, Choo J, Heffernan KS, Jae SY. 2016; The relationship between cardiorespiratory fitness and aortic stiffness in women with central obesity. J Womens Health (Larchmt). 25:680–6. DOI: 10.1089/jwh.2015.5314. PMID: 26595798.


16. Kappus RM, Fahs CA, Smith D, et al. 2014; Obesity and overweight associated with increased carotid diameter and decreased arterial function in young otherwise healthy men. Am J Hypertens. 27:628–34. DOI: 10.1093/ajh/hpt152. PMID: 24048148. PMCID: PMC3958602.


17. Vlachopoulos C, Terentes-Printzios D, Laurent S, et al. 2019; Association of estimated pulse wave velocity with survival: a secondary analysis of SPRINT. JAMA Netw Open. 2:e1912831. DOI: 10.1001/jamanetworkopen.2019.12831. PMID: 31596491. PMCID: PMC6802234.
18. Greve SV, Blicher MK, Kruger R, et al. 2016; Estimated carotid- femoral pulse wave velocity has similar predictive value as measured carotid-femoral pulse wave velocity. J Hypertens. 34:1279–89. DOI: 10.1097/HJH.0000000000000935. PMID: 27088638.
19. Heffernan KS, Jae SY, Loprinzi PD. 2020; Association between estimated pulse wave velocity and mortality in U.S. adults. J Am Coll Cardiol. 75:1862–4. DOI: 10.1016/j.jacc.2020.02.035. PMID: 32299599.


20. Heffernan KS, Barreira TV. 2020; Association between pulse pressure and aortic calcification: findings from the National Health and Nutrition Examination Survey 2013-2014. J Clin Hypertens (Greenwich). 22:879–85. DOI: 10.1111/jch.13853. PMID: 32249504.


21. Cai X, Xie L. 2018; A1275 relationship between vascular overload index and carotid-femoral pulse wave velocity in hypertensives. J Hypertens. 36:e118. DOI: 10.1097/01.hjh.0000548473.33676.37.


22. Yu Y, Huang Y, Liu L, et al. 2021; Association between vascular overload index and new-onset ischemic stroke in elderly population with hypertension. Clin Interv Aging. 16:1293–301. DOI: 10.2147/CIA.S312060. PMID: 34267509. PMCID: PMC8275168.


23. Al-Mallah MH, Juraschek SP, Whelton S, et al. 2016; Sex differences in cardiorespiratory fitness and all-cause mortality: the Henry Ford ExercIse Testing (FIT) Project. Mayo Clin Proc. 91:755–62. DOI: 10.1016/j.mayocp.2016.04.002. PMID: 27161032. PMCID: PMC5617114.
24. Srikanthan P, Horwich TB, Calfon Press M, Gornbein J, Watson KE. 2021; Sex differences in the association of body composition and cardiovascular mortality. J Am Heart Assoc. 10:e017511. DOI: 10.1161/JAHA.120.017511. PMID: 33619971. PMCID: PMC8174238.


25. Tarp J, Grøntved A, Sanchez-Lastra MA, Dalene KE, Ding D, Ekelund U. 2021; Fitness, fatness, and mortality in men and women from the UK Biobank: prospective cohort study. J Am Heart Assoc. 10:e019605. DOI: 10.1161/JAHA.120.019605. PMID: 33715383. PMCID: PMC8174221.


26. Loprinzi PD, Cardinal BJ. 2012; Interrelationships among physical activity, depression, homocysteine, and metabolic syndrome with special considerations by sex. Prev Med. 54:388–92. DOI: 10.1016/j.ypmed.2012.03.016. PMID: 22486943.


27. Coutinho T, Pellikka PA, Bailey KR, Turner ST, Kullo IJ. 2016; Sex differences in the associations of hemodynamic load with left ventricular hypertrophy and concentric remodeling. Am J Hypertens. 29:73–80. DOI: 10.1093/ajh/hpv071. PMID: 26031305. PMCID: PMC5014130.


28. Regnault V, Thomas F, Safar ME, et al. 2012; Sex difference in cardiovascular risk: role of pulse pressure amplification. J Am Coll Cardiol. 59:1771–7. DOI: 10.1016/j.jacc.2012.01.044. PMID: 22575315. PMCID: PMC3716253.
29. Loprinzi PD, Edwards MK. 2016; CVD-related Fit-Fat Index on inflammatory-based CVD biomarkers. Int J Cardiol. 223:284–5. DOI: 10.1016/j.ijcard.2016.08.194. PMID: 27541674.


30. Joyner MJ, Green DJ. 2009; Exercise protects the cardiovascular system: effects beyond traditional risk factors. J Physiol. 587(Pt 23):5551–8. DOI: 10.1113/jphysiol.2009.179432. PMID: 19736305. PMCID: PMC2805367.


Fig. 1
Scatterplot illustrating the relationship between fitness fatness index (FFI) and estimated pulse wave velocity (ePWV) (N=8,080). CI: confidence interval.
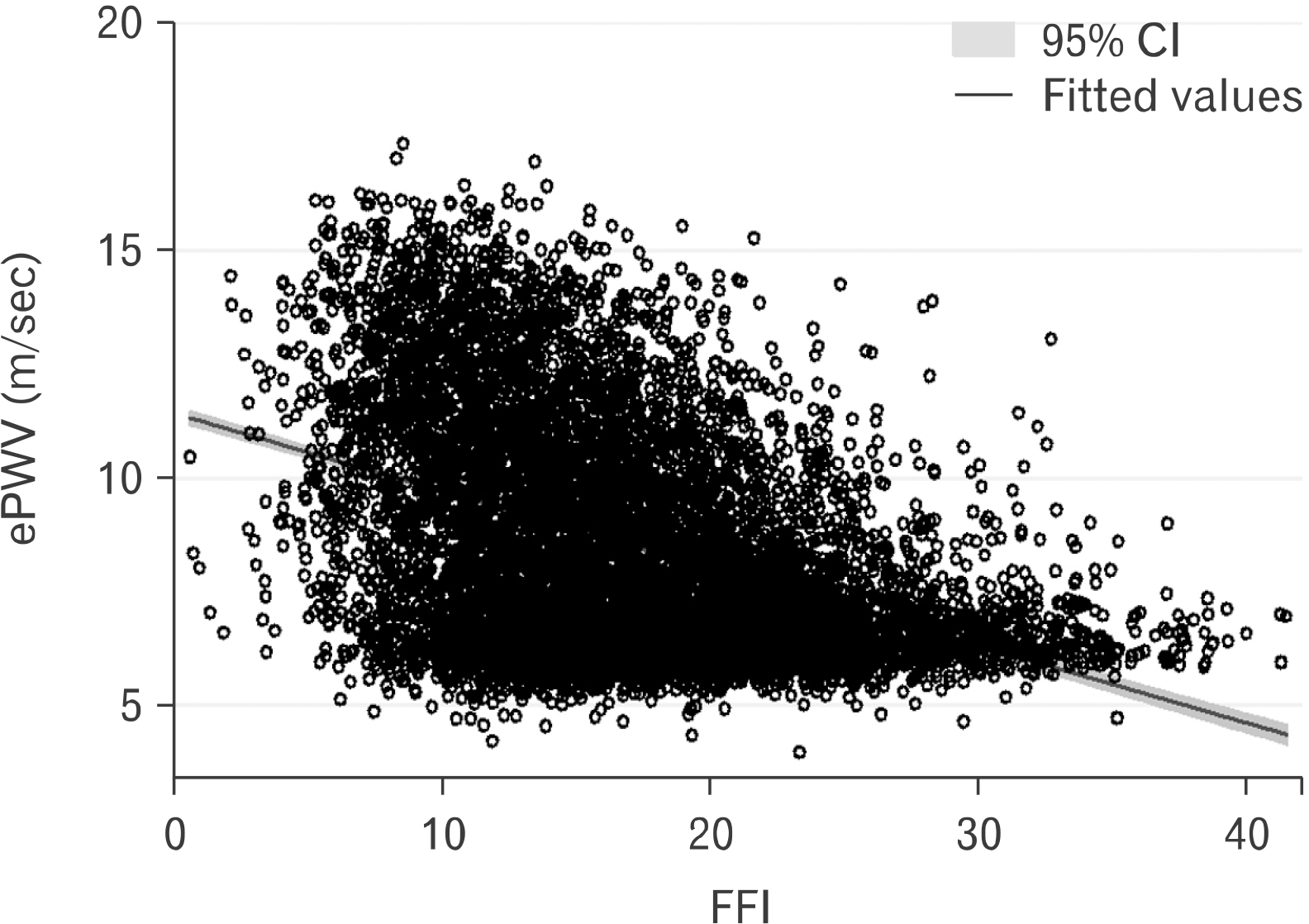
Fig. 2
Scatterplot illustrating the relationship between fitness fatness index (FFI) and pulse pressure (N=8,080). CI: confidence interval.
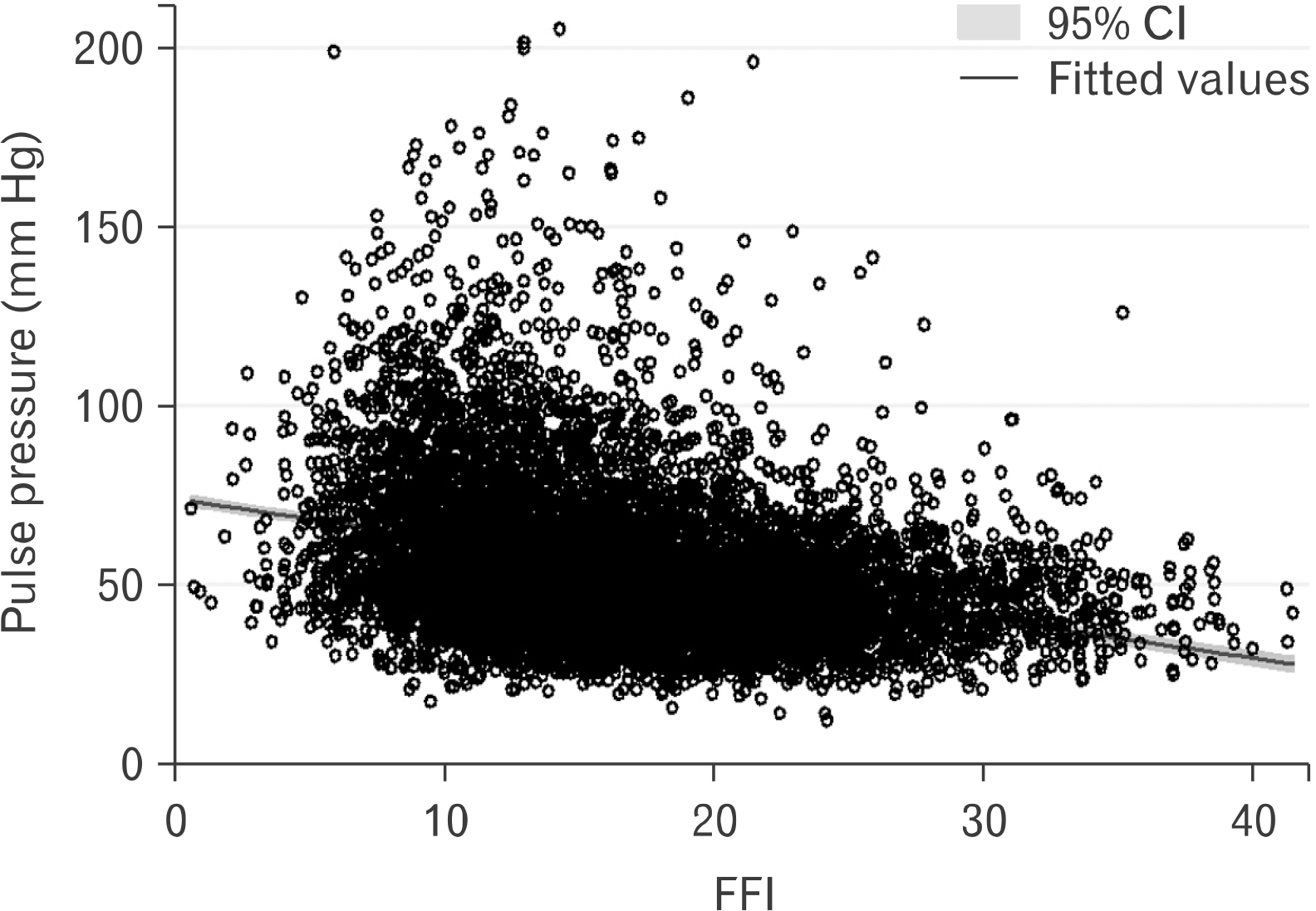
Fig. 3
Scatterplot illustrating the relationship between fitness fatness index (FFI) and vascular overload index (VOI) (N=8,080). CI: confidence interval.
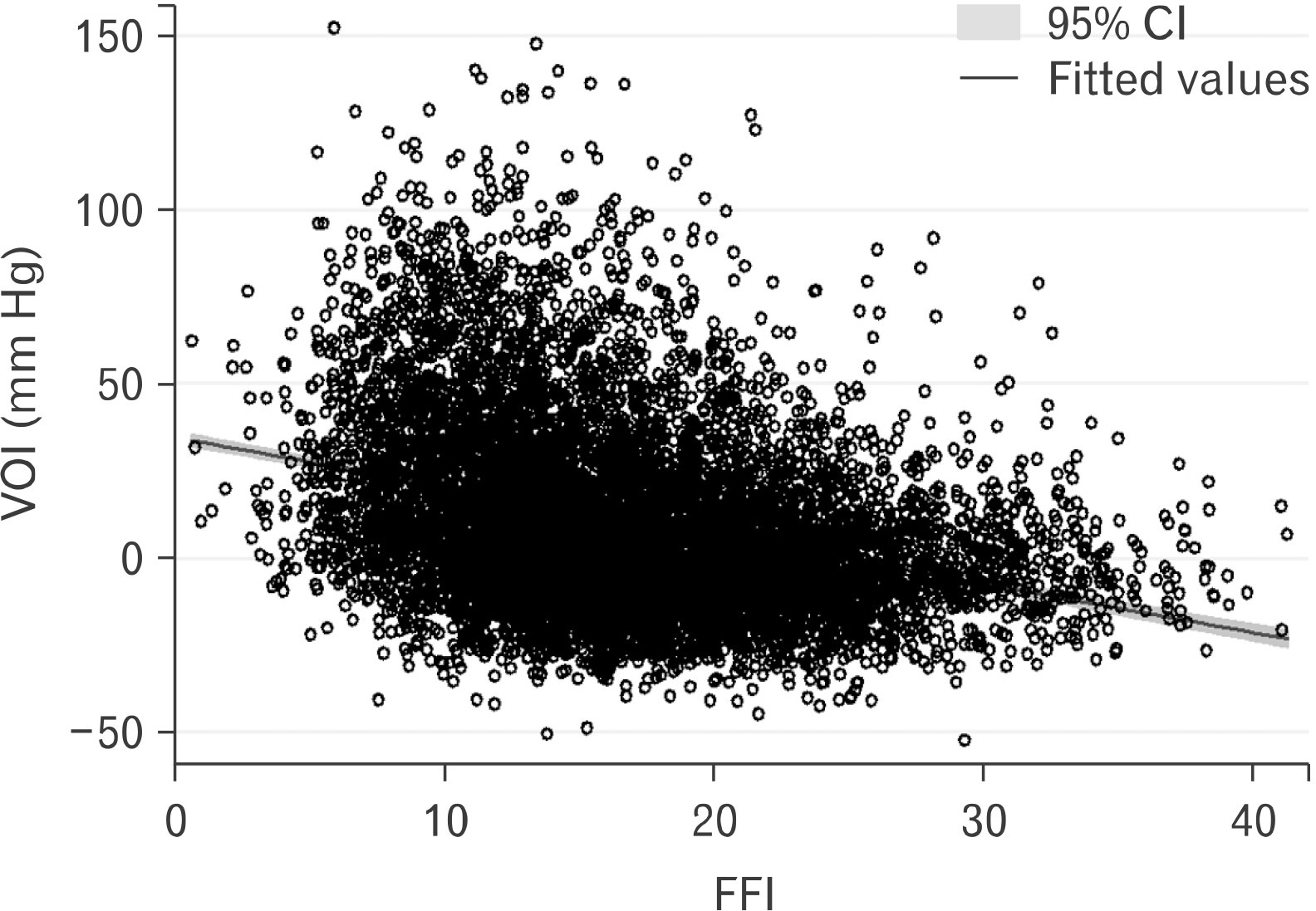
Table 1
Univariate characteristics of the study variables among American Participants*