Abstract
Background
Methods
Results
ACKNOWLEDGEMENTS
Notes
AUTHOR CONTRIBUTIONS
Kim J participated in research design and in the writing of the paper. Kim DM participated in the performance of the research. Park YJ participated in the acquisition of data. Lee ST contributed provided technical and analytical support. Kim HS participated in advising research methodology. Kim MS participated in research design and collection of samples. Kim BS participated in critically revising the study. Choi JR supervised the full study and acquired financial support.
REFERENCES


































































Fig. 1
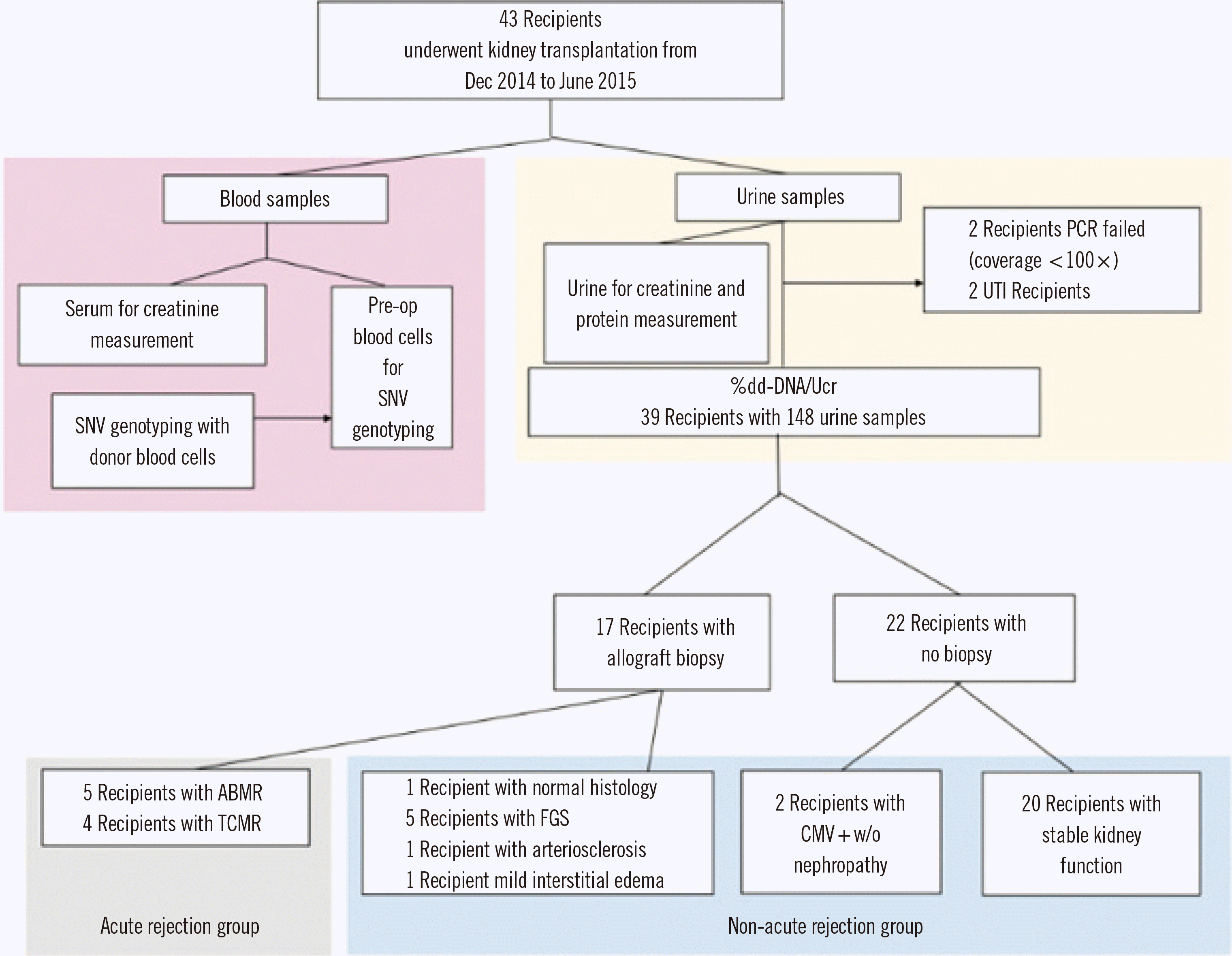
Fig. 2
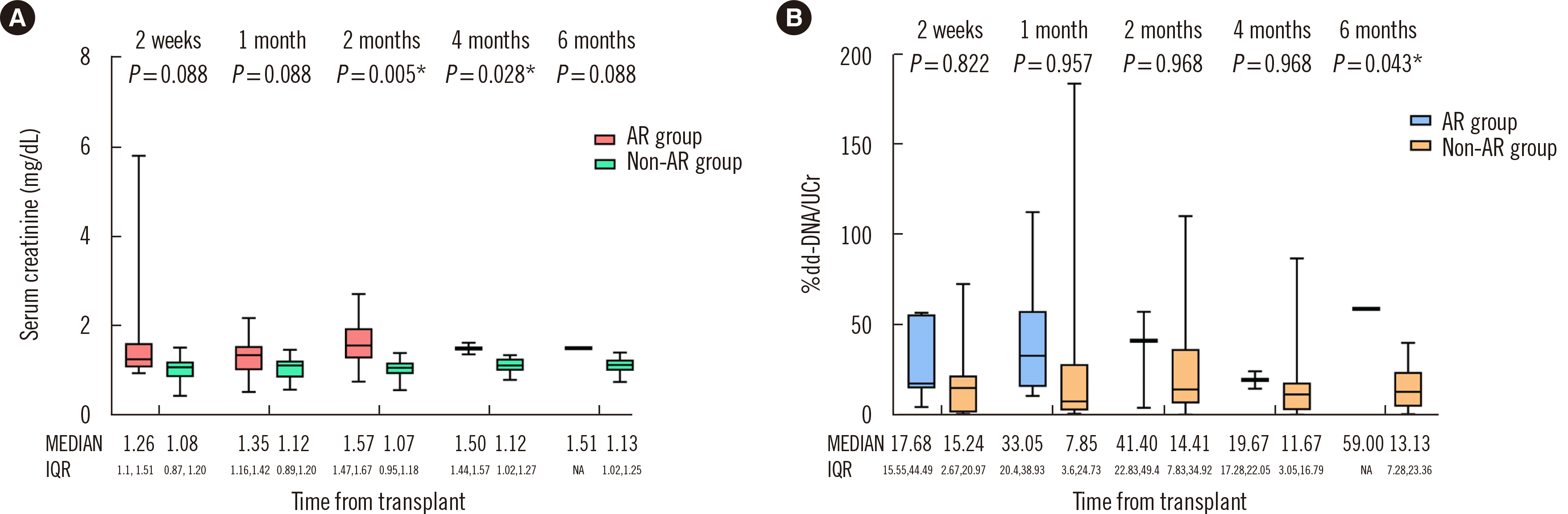
Table 1
Recipient number | N informative SNVs* | Outcome | Days after transplantation | % VAF of informative SNVs | Mean depth |
---|---|---|---|---|---|
KT01 | 11 | Non-AR | 28 | 0.315 | 8,156 |
105 | 0.262 | 13,898 | |||
168 | 1.476 | 10,231 | |||
KT02 | 7 | Non-AR | 168 | 0.885 | 11,317 |
196 | 6.545 | 9,866 | |||
KT03 | 11 | AR | 14 | 2.008 | 7,338 |
21 | 15.189 | 234 | |||
35 | 1.798 | 12,158 | |||
KT04 | 10 | Non-AR | 28 | 7.863 | 3,872 |
56 | 14.164 | 1,481 | |||
112 | 19.335 | 290 | |||
168 | 28.726 | 953 | |||
196 | 60.878 | 798 | |||
KT05 | 10 | AR | 14 | 18.449 | 687 |
28 | 60.199 | 859 | |||
98 | 41.693 | 1,059 | |||
KT06 | 6 | Non-AR | 21 | 0.15 | 1,170 |
84 | 0.22 | 1,181 | |||
224 | 27.358 | 379 | |||
KT07 | 16 | AR | 7 | 10.279 | 6,257 |
28 | 14.012 | 3,574 | |||
56 | 16.531 | 2,002 | |||
84 | 6.016 | 10,501 | |||
KT08 | 13 | AR | 14 | 71.87 | 215 |
49 | 74.835 | 7,369 | |||
140 | 74.182 | 6,140 | |||
168 | 87.5 | 667 | |||
KT09 | 12 | Non-AR | 7 | 22.247 | 200 |
14 | 3.045 | 9,065 | |||
KT10 | 6 | Non-AR | 14 | 12.29 | 253 |
28 | 3.69 | 5,120 | |||
168 | 60.259 | 953 | |||
196 | 50.038 | 2,836 | |||
KT11 | 7 | Non-AR | 56 | 15.27 | 198 |
168 | 53.733 | 197 | |||
196 | 66.709 | 570 | |||
KT12 | 13 | Non-AR | 21 | 0.747 | 16,881 |
63 | 1.112 | 21,639 | |||
112 | 0.541 | 17,665 | |||
196 | 1.772 | 7,821 | |||
KT13 | 3 | Non-AR | 21 | 7.485 | 2,917 |
98 | 1.096 | 12,332 | |||
140 | 6.866 | 6,218 | |||
KT14 | 15 | Non-AR | 7 | 6.55 | 7,994 |
35 | 60.226 | 184 | |||
84 | 18.166 | 1,129 | |||
196 | 71.989 | 299 | |||
KT15 | 4 | Non-AR | 7 | 9.766 | 1,083 |
14 | 1.482 | 3,149 | |||
21 | 1.729 | 9,980 | |||
28 | 1.589 | 16,213 | |||
84 | 1.274 | 10,194 | |||
112 | 4.617 | 5,072 | |||
168 | 3.48 | 10,687 | |||
196 | 3.642 | 9,194 | |||
KT16 | 5 | AR | 7 | 47.212 | 4,863 |
14 | 30.175 | 11,197 | |||
28 | 38.849 | 326 | |||
KT17 | 6 | Non-AR | 7 | 14.444 | 1,131 |
14 | 0.877 | 10,340 | |||
21 | 1.427 | 8,684 | |||
28 | 0.545 | 9,756 | |||
56 | 0.279 | 8,548 | |||
KT18 | 14 | Non-AR | 14 | 14.217 | 1,374 |
21 | 7.008 | 2,124 | |||
56 | 6.775 | 986 | |||
168 | 10.675 | 544 | |||
KT19 | 10 | Non-AR | 21 | 7.199 | 6,390 |
28 | 15.113 | 2,201 | |||
56 | 21.449 | 9,504 | |||
140 | 30.468 | 1,179 | |||
168 | 24.242 | 690 | |||
KT20 | 6 | Non-AR | 14 | 5.373 | 7,910 |
28 | 2.724 | 6,112 | |||
56 | 10.671 | 4,680 | |||
84 | 7.544 | 1,307 | |||
KT21 | 11 | Non-AR | 14 | 1.104 | 6,741 |
21 | 0.773 | 6,372 | |||
28 | 1.302 | 6,693 | |||
56 | 2.892 | 3,462 | |||
112 | 1.416 | 1,820 | |||
140 | 2.39 | 1,972 | |||
168 | 2.231 | 1,138 | |||
KT22 | 10 | AR | 7 | 37.995 | 595 |
28 | 2.386 | 7,236 | |||
35 | 5.667 | 1,772 | |||
KT23 | 7 | Non-AR | 21 | 57.325 | 443 |
28 | 9.601 | 694 | |||
56 | 44.057 | 3,159 | |||
140 | 12.425 | 1,103 | |||
168 | 26.747 | 164 | |||
KT24 | 12 | Non-AR | 7 | 0.779 | 7,900 |
14 | 20.215 | 212 | |||
KT25 | 6 | Non-AR | 21 | 31.853 | 1,733 |
28 | 76.37 | 1,202 | |||
56 | 39.785 | 529 | |||
98 | 11.965 | 1,084 | |||
140 | 34.996 | 155 | |||
168 | 67.116 | 358 | |||
KT26 | 14 | Non-AR | 7 | 4.451 | 2,753 |
21 | 3.166 | 6,206 | |||
28 | 1.564 | 577 | |||
56 | 7.804 | 5,964 | |||
168 | 6.526 | 3,915 | |||
196 | 27.5 | 117 | |||
KT27 | 13 | Non-AR | 21 | 75.125 | 4,275 |
28 | 70.905 | 861 | |||
56 | 47.568 | 179 | |||
84 | 69.772 | 575 | |||
112 | 62.085 | 319 | |||
140 | 55.039 | 1,018 | |||
KT28 | 18 | Non-AR | 7 | 73.252 | 4,460 |
21 | 51.656 | 298 | |||
28 | 71.759 | 2,175 | |||
49 | 68.115 | 2,853 | |||
84 | 69.13 | 2,829 | |||
112 | 70.321 | 1,243 | |||
140 | 64.442 | 6,659 | |||
KT29 | 16 | Non-AR | 7 | 81.141 | 1,448 |
14 | 87.269 | 156 | |||
KT30 | 19 | Non-AR | 7 | 59.978 | 215 |
21 | 83.587 | 607 | |||
KT31 | 10 | Non-AR | 7 | 3.449 | 15,343 |
14 | 3.683 | 2,645 | |||
KT32 | 12 | Non-AR | 7 | 1.42 | 4,315 |
14 | 9.069 | 314 | |||
KT33 | 11 | AR | 7 | 22.03 | 7,999 |
21 | 5.062 | 19,788 | |||
56 | 4.564 | 20,496 | |||
KT34 | 7 | Non-AR | 14 | 9.213 | 293 |
21 | 6.532 | 13,266 | |||
28 | 9.941 | 2,271 | |||
56 | 10.483 | 5,422 | |||
KT35 | 9 | Non-AR | 14 | 10.816 | 517 |
28 | 11.187 | 2,630 | |||
56 | 23.901 | 177 | |||
84 | 17.485 | 271 | |||
KT36 | 14 | Non-AR | 14 | 27.191 | 814 |
28 | 14.659 | 1,620 | |||
56 | 18.461 | 201 | |||
KT37 | 13 | AR | 14 | 14.544 | 2,919 |
21 | 14.918 | 3,103 | |||
56 | 28.527 | 1,219 | |||
63 | 22.442 | 2,787 | |||
KT38 | 10 | Non-AR | 7 | 45.796 | 156 |
28 | 14.405 | 159 | |||
KT39 | 10 | AR | 14 | 2.542 | 7,328 |
28 | 49.321 | 402 | |||
35 | 4.727 | 499 |
*The following 39 SNV markers were used for the chimerism calculation: rs3738561, rs6480497, rs4757113, rs7983800, rs3745331, rs10426644, rs2540307, rs1358833, rs62270249, rs1436501, rs9386037, rs2159478, rs11023112, rs6589967, rs8022985, rs1202017, rs645107, rs6921313, rs4072990, rs6676162, rs72735619, rs11187560, rs10832201, rs7950719, rs6590643, rs1731550, rs10777988, rs4496026, rs12327492, rs1348784, rs281544, rs6445350, rs3819864, rs6863833, rs1423013, rs1561681, rs73230060, rs6995506, and rs16904057.
Table 2
Characteristics* | AR group (N = 9) | Non-AR group (N = 30) | P† |
---|---|---|---|
Age at transplantation (yr) | |||
Recipient | 49.0 (44.0–53.0) | 44.0 (36.0–54.0) | 0.385 |
Donor | 47.0 (45.0–53.0) | 40.0 (33.0–49.0) | 0.054 |
Sex | |||
Female/Male | 2/7 (28.6%) | 9/21 (42.9%) | 0.595 |
Body mass index (kg/m2) | |||
Recipient | 22.4 (20.6–24.2) | 22.5 (18.7–25.9) | 0.958 |
Donor | 22.3 (21.0–23.5) | 22.5 (20.4–24.7) | 0.741 |
Allograft length (cm) | 10.5 (10.3–11.4) | 11.0 (10.3–11.3) | 0.446 |
Donor type (living) | |||
Genetically related | 2/9 (22.2%) | 22/30 (73.3%) | 0.001 |
Parent | 0 | 12 | |
Sibling | 2 | 10 | |
Genetically unrelated (Spouse) | 7/9 (77.8%) | 8/30 (26.7%) | |
Tacrolimus trough level (ng/mL) | 3.60 (3.10–4.85) | 4.73 (3.8–5.82) | 0.110 |
ABO incompatibilities, N (%) (donor→recipient) | 1 (11.1%) | 10 (33.3%) | 0.421 |
HLA mismatch (HLA-A, B, DR) | 5 (3–6) | 3 (2–4) | 0.044 |
PRA Screening (%) (average) | 0.0 (0.0–0.0) | 0.0 (0.0–0.75) | 0.208 |
Table 3
Table 4
AUC (95% CI) | Cut-off* | Sensitivity (%) | Specificity (%) | Positive predictive value (%) | Negative predictive value (%) | |
---|---|---|---|---|---|---|
%dd-DNA/UCr† | 0.58 (0.42–0.75) | 47.50% | 53.6 | 77.8 | 15.2 | 95.7 |
SCr (mg/dL) | 0.79 (0.60–0.98) | 1.3 | 78.3 | 83.3 | 0.58 | 96.6 |
UPtn/UCr | 0.78 (0.57–0.98) | 58.4 | 57.9 | 100.0 | 33.3 | 100.0 |
SCr and UPtn/UCr | 0.91 (0.76–1.00) | 0.2 | 86.7 | 100.0 | 60.0 | 97.7 |
%dd-DNA/UCr, SCr and UPtn/UCr | 0.93 (0.81–1.00) | 0.2 | 86.7 | 100.0 | 60.0 | 100.0 |
*The cut-off values for %dd-DNA/UCr, SCr, and UPtn/UCr were individually selected to yield the highest Youden index, whereas the cut-offs for their combinations were determined using a non-parametric general linear model. †The diagnostic performance of %dd-DNA/UCr, evaluated by ROC curve analysis, were values of maximal %dd-DNA/UCr, which is defined as the highest %dd-DNA/UCr level among serial %dd-DNA/UCr values measured for each recipient (in both the AR and non-AR groups) at a certain time point.