This article has been
cited by other articles in ScienceCentral.
Abstract
Background and Objectives
A mobile application (app)-based electrocardiogram (ECG) consultation system (InterMD Co., Ltd., Seoul, Korea) using the collective intelligence (CI) and the availability of large-scale digitized ECG data would extend the utility of ECGs beyond their current limitations, while at the same time preserving interpretability that remains critical to medical decision-making.
Methods
We developed a new mobile app-based ECG consultation system by CI for general practitioners. We compared the responses of ECG reading between the mobile app-based CI system and the conventional system in a tertiary referring hospital.
Results
We analyzed 376 consecutive ECGs between December 2017 and May 2019. Of these, 159 ECGs (42.3%) were interpreted by CI through the mobile app-based ECG consultation system and 217 ECGs (57.7%) were analyzed by cardiologists in the conventional systems based on electronic medical record data in a tertiary hospital. All ECG readings were confirmed by an electrophysiologist (EP). The time to an initial response by the CI system was faster than that of the conventional system (6.6 hours vs. 35.8 hours, p<0.0001). The number of responses of each ECG in CI system outnumbered those of the conventional system in the tertiary hospital (3.1 vs. 1.2, p<0.0001). The consensus of the ECG readings with EP was similar in both systems (98.6% vs. 100%, p=0.158).
Conclusions
The mobile app-based ECG consultation system by CI is as reliable method as the conventional referral system. It would expand the app of the 12-lead ECG with the collaboration of physicians in clinics and hospitals without time and space constraints.
Keywords: Electrocardiography, Mobile applications
INTRODUCTION
The electrocardiogram (ECG) has been a great tool for diagnosing arrhythmia and cardiac disease for over a century.
1) However, until a few decades ago, the use of ECGs was limited to experts.
2)3)4) Recent advances in mobile technology have made it easier for non-medical personnel to make use of ECGs. Automated ECG interpretation is further fueling this trend. Such interpretations involve the use of artificial intelligence (AI) using pattern recognition software and knowledge bases to automatically interpret ECG readings.
5) However, the accurate interpretation of ECGs is still confined to medical experts. Even physicians sometimes disagree on ECG readings. Therefore, many confusing algorithms have been reported.
6)7)8)9) In medical practice, first-contact medical care is the main entrance to health services, providing continuous and comprehensive personal attention to patients. Therefore, a proper communication channel between the first and second level of medical care is needed. Physicians use inter-consulting as the main element to build this communication channel.
Globally, there are
Figure 1, QuantiaMD, and Sermo for sharing medical Q&A among physicians. However, in Korea, there was no reliable medical consulting platform among doctors and many primary care physicians struggle with even a simple ECG reading. They need fast reconfirmation of their initial diagnosis and also want to know which of their patients need to be referred to a specialist.
Figure 1
InterMD is a mobile application-based electrocardiogram consultation system by collective intelligence.
Collective intelligence (CI) is a powerful option in the medical field where differences of opinions exist.
10) Heylighen
11) define CI as the ability of a group to solve more problems than its individual members can do separately. The basic idea is that a group of individuals can be smarter together than separated. CI in medical decision-making is gaining popularity to advance medical decision-making and holds promise to improve patient outcomes.
12) In a recent study, simple and highly transparent CI improved the accuracy of mammography screening.
10) CI may have the potential to improve ECG based decision-making in a much wider range of contexts that are based on the ECG and patient history. In addition, recent advancements in mobile technologies and high speed internet have allowed it easier to organize CI than before. ECG consultation system by CI may preserve the interpretability of ECG while expanding the important attributes for medical decision-making.
For CI based ECG consultations, we founded InterMD Co., Ltd. which supports a mobile app-based ECG consulting system to realize ubiquitous ECG tele-diagnosis for general practitioners in late 2017. In this system, primary physicians can easily share their own patients' ECG with a clinical history and receive responses from the experts in ECG interpretation (e.g., internal medicine specialist including cardiologists). The aim of this CI based ECG consultation platform is to help primary physicians’ clinical decision making.
METHODS
This mobile-app based ECG consultation platform is available by any devices that are connected to internet; mobile phones, tablets, laptops and desktop computers. In this mobile environment, any doctors could see the ECG data and their interpretation similar to typical social media. Over 30,000 doctors in Korea are using this platform now. Main users of the platform are primary physicians and this was developed to reduce the gap between primary physicians and the experts in ECG readings. With our platform, we can reassure primary physician their initial diagnosis and finally reduce unnecessary referral from primary clinics to tertiary centers.
When an ECG with brief medical history but without any personal identifying information was registered from primary practice, two or more experts who connected to the system at the time can post the readings of the ECG (
Figure 1). If no experts are connected at that time, automatic alarm signal such as SNS alarm can be sent to the experts. CI, which is registered as an expert in ECG readings, consists of internal medicine, cardiology, emergency medicine and occupational environmental medicine specialists. CI was also defined as being formed only if any of two or more experts gave answers to the posted ECGs.
After the stabilization of mobile application (app), a retrospective registry study was conducted to determine the efficacy and the accuracy of the ECG reading in CI system compared to the existing conventional referral system.
Inclusion criteria for the CI system were the 12 leads ECG data posted in the mobile app through CI between December 1, 2017 and May 31, 2019. For the conventional system, ECG data from electronic medical records among patients consulted in cardiology department over the same period at the participation centers was also retrospectively collected. Exclusion criteria were in case there is no ECG data in the consulting patient and mobile app or it is inappropriate for ECG analysis due to noise, etc. Only minimum of baseline identifying characteristics, such as gender, age and clinical symptoms related to ECG data were achieved.
ECG readings by CI system was confined as two or more expert readings of ECG through the “InterMD” mobile app-based platform (1F, 104, Bukchon-ro, Jongno-gu, Seoul, Korea, and categorized as a Digital healthcare services company). ECG analysis by conventional system was specified as the ECG readings by a single cardiologist (prof. JHK or prof. YRK) with inter-department or inter-hospital face to face consultation in tertiary hospital.
Primary outcome of the study were the efficacy of the responses and the accuracy of ECG readings. The efficacy of ECG readings was defined as the time it took for the first response to run. Since the ECG readings using CI continued over the weekend, the time analysis was calculated not only during the week but also during the weekend which was not the regular working hours of the tertiary hospital. Investigators also calculated the time to last response as same manner, and the total number of responses to a single ECG reading. All ECG data were reviewed by an electrophysiologist (EP) (prof. YMH) who was blinded to the ECG readings in both systems. The accuracy of ECG readings was determined by comparing the readings of ECG extracted from each system with the consensus of the EP (
Figure 2). This study was approved by the Institutional Ethics Committee and Review Board at Dongguk University Ilsan Hospital (DUIH 2020-04-046). This work was supported by a research grant from The Korean Society of Cardiology (KCRF 201802-02).
Figure 2
Comparison and validation of ECG readings by collective intelligence (InterMD) vs. by conventionl system in a referrring hospital.
ECG = electrocardiogram; EP = electrophysiologist.
Statistical analysis
Statistical analysis was performed using R 3.6.3 statistical software (R Development Core Team, 2014). The continuous variables were examined by a t-test when appropriate and the results are expressed as the mean ± standard deviation. The continuous variables that were not normally distributed were analyzed using the Mann–Whitney U test and are described as the median±interquartile range. The categorical variables were analyzed by the χ2 test and are described as frequencies.
RESULTS
We analyzed data from 376 consecutive patients with consultations for ECG readings (age 68±13 years; 58.3% male) between December 1, 2017 and May 31, 2019. Of these, 159 ECGs (42.3%) were interpreted by CI in the mobile app (InterMD), and 217 ECGs (57.7%) were analyzed by two cardiologists in a tertiary-level hospital. All ECG data were confirmed by the cardiac EP, retrospectively. The patient characteristics and referral ECG of both systems are described in
Table 1. The proportion of primary physician referrals was higher in the CI system than that of the conventional system. The distribution of responders based on their specialties was different between the two systems (
Table 1). In the tertiary hospital, all of the ECG readings were performed by a cardiologist, especially the EP, whereas in the CI system, doctors from various departments actively participated in the ECG readings (Internal medicine including cardiologist, emergent medicine, occupational medicine, etc.). Medical emergent situations, including myocardial infarction or ventricular arrhythmia, were more frequent in the conventional system than in the CI system (1.5±5.6 vs. 3.6±2.4, p<0.041). Lastly, incidence of atrial fibrillation was also higher in conventional system than CI system (50.2% vs. 9.0%, p<0.0001). This is due to the fundamental difference between the patients of both systems. For the patients who were referred to cardiology outpatient clinics in tertiary hospital and including inter-department consults were bound to have higher severity of disease than the patients in primary practice.
Table 1
Basic characteristics of patients and ECGs in collective intelligence system and conventional system in a referrring hospital
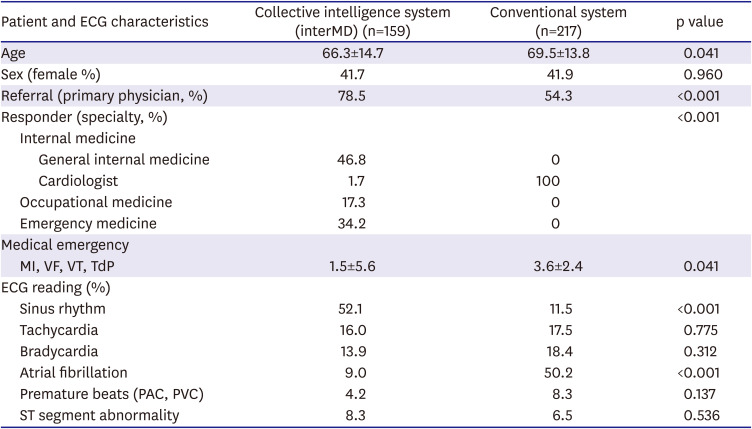
Patient and ECG characteristics |
Collective intelligence system (interMD) (n=159) |
Conventional system (n=217) |
p value |
Age |
66.3±14.7 |
69.5±13.8 |
0.041 |
Sex (female %) |
41.7 |
41.9 |
0.960 |
Referral (primary physician, %) |
78.5 |
54.3 |
<0.001 |
Responder (specialty, %) |
|
|
<0.001 |
|
Internal medicine |
|
|
|
|
General internal medicine |
46.8 |
0 |
|
|
Cardiologist |
1.7 |
100 |
|
Occupational medicine |
17.3 |
0 |
|
Emergency medicine |
34.2 |
0 |
Medical emergency |
|
|
|
|
MI, VF, VT, TdP |
1.5±5.6 |
3.6±2.4 |
0.041 |
ECG reading (%) |
|
|
|
|
Sinus rhythm |
52.1 |
11.5 |
<0.001 |
|
Tachycardia |
16.0 |
17.5 |
0.775 |
|
Bradycardia |
13.9 |
18.4 |
0.312 |
|
Atrial fibrillation |
9.0 |
50.2 |
<0.001 |
|
Premature beats (PAC, PVC) |
4.2 |
8.3 |
0.137 |
|
ST segment abnormality |
8.3 |
6.5 |
0.536 |
The efficacy and the accuracy of the ECG readings in both systems are shown in
Table 2. The time to an initial ECG reading by CI in the InterMD app was significantly faster than that of the ECG interpretations by cardiologists in the tertiary hospital using a conventional system (6.6 hours vs. 35.8 hours, p<0.0001). However, the time to the last response for the ECG readings was comparable in both systems (55.2 hours vs. 69.3 hours, p=0.07. The total number of responses for the ECG readings in the CI system outnumbered that of the conventional system (3.3±2.5 vs. 1.2±0.5, p<0 .0001). Unlike conventional systems which can no longer be answered after closing the consult, replies in CI system are continuously open. It allowed for continuous feedback and post correction in a comments system like social media. On the accuracy of ECG readings, the consensus rate with the EP was similar in both systems (98.6% vs. 100%, p=0.158). Only two cases of ECG readings in CI were not in consensus with the EP. One was incomplete RBBB which CI reading was Brugada syndrome and another was AF with interventricular conduction delay which was ventricular tachycardia in CI readings.
Table 2
Comparison between collective intelligence system and conventional system in a referrring hospital

Comparison variables |
Collective intelligence system (interMD) (n=159) |
Conventional system (n=217) |
p value |
Time to first response (hours) |
6.6±6.4 |
35.8±10.8 |
<0.001 |
Time to the last response (hours) |
55.2±34.7 |
69.3±50.7 |
0.075 |
Total number of ECG answers |
3.3±2.5 |
1.2±0.5 |
<0.001 |
Consensus with cardiac EP (%) |
98.6 |
100 |
0.158 |
DISCUSSION
In the current study, we compared the efficacy and the accuracy of ECG interpretations between the InterMD app by CI and the conventional system in a tertiary hospital. We found that ECG consultation platform in the InterMD app by CI was faster than those of the conventional system with comparable diagnostic accuracy, which demonstrates its usefulness. The proportion of primary physician referrals was higher in the CI system than that of the conventional system. This result was consistent with the original purpose of developing the mobile app to help doctors in primary practice. This is the first study to validate the app of CI in this area of cardiology. The biggest advantage of the ECG consultation in InterMD app is supporting the fast decision-making process in primary practice by the rapid sharing of ECG data using CI. Currently, AI has become an important tool in various academic fields. In the same vein, AI-based ECG analysis using digitalized ECG data is actively being researched in the field of cardiology. AI is evolving at a rapid rate. However, there is still a long way to go before it can be applied to general practice.
13)14)15) The most critical limitation of digitized ECG analysis of individual patient for diagnosis is the lack of clinical background reflected in each ECG. This is reflected in the fall of IBM Watson, a question answering computer system which was developed to assist decision-making in oncology. IBM Watson had abundant clinical trial data and was able to search for results faster than any oncologist, but was not capable to grasp the clinical conditions of an individual patient.
16) AI is particularly vulnerable in practice when image processing is needed or where there are abundant normal variations, such as ECG. In this manner, the clinical symptom of individual patients is essential to interpreting ECGs in real practice. In this sense, the CI system with high speed internet and mobile technology is a powerful tool for the ECG readings and it help physicians' decision-making more efficiently and accurately. Collected ECG data in CI system which had consensus of EP may also be used as a potential educational resource for machine learning of AI system in the future. CI could act as a bridge between the conventional system and AI system. Eventually, the collaboration of CI and AI would greatly improve diagnostic and decision-making capability not only in cardiology but in all medical fields.
17)18)
Technically, the ECG data between the CI system and conventional system was not properly distributed. It was because all comer data were used within the same period of time between the two systems. ECG data was not from the same patients and there was a fundamental difference of disease severity between two systems. For objective evaluation of the ECG readings, all ECGs were analyzed by third party, who is an EP cardiologist and a first author. The EP cardiologist was blinded with the source of ECGs to analyze the accuracy of ECG readings of both systems. Basically, there were more medical emergent situations in the tertiary center referrals with more variable ECG categories than those of primary practice in CI system. Another limitation of this study was the broad range of referrals from primary clinics to inter-department of the tertiary hospital. If the ECG analysis was limited to primary referrals in conventional system, it would be a clearer comparison but it would take more time to read ECG in conventional system. Nevertheless, the aim of the study was to evaluate the effectiveness of the CI through the mobile technology, it can be said that current results demonstrate the efficacy sufficiently. In addition, app-based CI consultation system was developed to assist the medical decision making in primary or secondary clinic where there is a lack of ECG experts (esp. EP cardiologist). It could help reduce unnecessary referrals from the primary clinic to the tertiary centers. Since InterMD Co. is the world's first ECG reading system by CI, the design of the present study has its inherent limitation. We could not compare our system with other app. The important advantage of the mobile ECG reading system by CI seems to be that it is easy for all doctors to access the ECG data regardless of their location or time. In future studies, the cross-validation of CI and AI would be also essential for mutually complementary development. Therefore, ECG data from the system has been continuously accumulating for the future analysis.
CI could improve the quality and efficiency of ECG readings and assisting physicians’ decision making in primary practice. This ECG consultation system by CI was reliable compared to the conventional inter-hospital or intra-hospital referral system. The InterMD, mobile app-based ECG consultation system by CI can expand the traditional apps of 12-lead ECG with the collaboration of clinicians without time and space constraints.